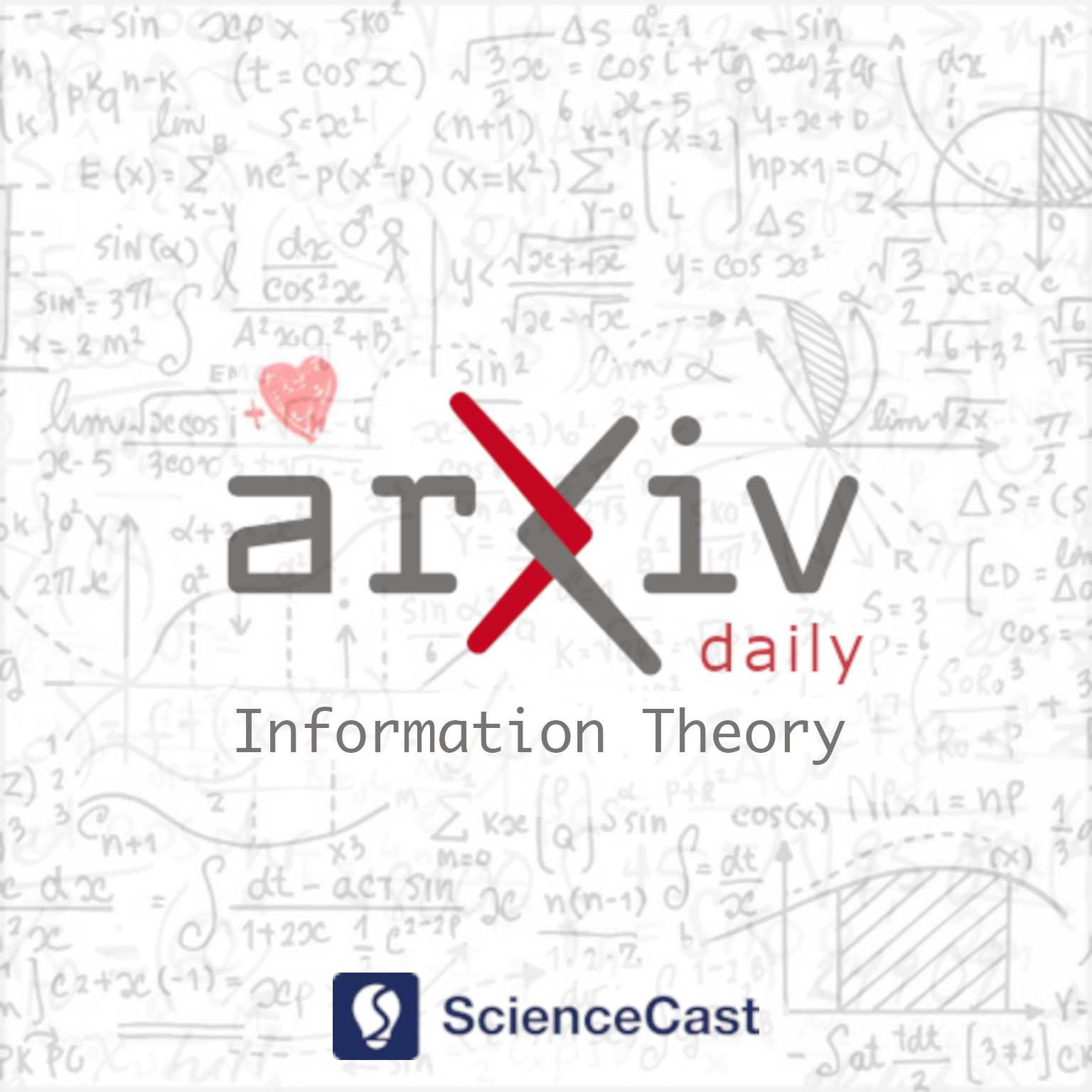
Information Theory (cs.IT)
Thu, 18 May 2023
1.Bounds on Size of Homopolymer Free Codes
Authors:Krishna Gopal Benerjee, Adrish Banerjee
Abstract: For any given alphabet of size $q$, a Homopolymer Free code (HF code) refers to an $(n, M, d)_q$ code of length $n$, size $M$ and minimum Hamming distance $d$, where all the codewords are homopolymer free sequences. For any given alphabet, this work provides upper and lower bounds on the maximum size of any HF code using Sphere Packing bound and Gilbert-Varshamov bound. Further, upper and lower bounds on the maximum size of HF codes for various HF code families are calculated. Also, as a specific case, upper and lower bounds are obtained on the maximum size of homopolymer free DNA codes.
2.Joint BS Mode Selection and Beamforming Design for Cooperative Cell-Free ISAC Networks
Authors:Sifan Liu, Ming Li, Qian Liu
Abstract: Owing to the promising ability of saving hardware cost and spectrum resources, integrated sensing and communication (ISAC) is regarded as a revolutionary technology for future sixth-generation (6G) networks. The mono-static ISAC systems considered in most of existing works can only obtain limited sensing performance due to the single observation angle and easily blocked transmission links, which motivates researchers to investigate cooperative ISAC networks. In order to further improve the degrees of freedom (DoFs) of cooperative ISAC networks, the transmitter-receiver selection, i.e., BS mode selection problem, is meaningful to be studied. However, to our best knowledge, this crucial problem has not been extensively studied in existing works. In this paper, we consider the joint BS mode selection, transmit beamforming, and receive filter design for cooperative cell-free ISAC networks, where multi-base stations (BSs) cooperatively serve communication users and detect targets. We aim to maximize the sum of sensing signal-to-interference-plus-noise ratio (SINR) under the communication SINR requirements, total power budget, and constraints on the numbers of transmitters and receivers. An efficient joint beamforming design algorithm and three different heuristic BS mode selection methods are proposed to solve this non-convex NP-hard problem. Simulation results demonstrates the advantages of cooperative ISAC networks, the importance of BS mode selection, and the effectiveness of our proposed joint design algorithms.
3.Robust Hybrid Transceiver Designs for Linear Decentralized Estimation in mmWave MIMO IoT Networks in the Face of Imperfect CSI
Authors:Priyanka Maity, Kunwar Pritiraj Rajput, Suraj Srivastava, Naveen K. D. Venkategowda, Aditya K. Jagannatham, Lajos Hanzo
Abstract: Hybrid transceivers are designed for linear decentralized estimation (LDE) in a mmWave multiple-input multiple-output (MIMO) IoT network (IoTNe). For a noiseless fusion center (FC), it is demonstrated that the MSE performance is determined by the number of RF chains used at each IoT node (IoTNo). Next, the minimum-MSE RF transmit precoders (TPCs) and receive combiner (RC) matrices are designed for this setup using the dominant array response vectors, and subsequently, a closed-form expression is obtained for the baseband (BB) TPC at each IoTNo using Cauchy's interlacing theorem. For a realistic noisy FC, it is shown that the resultant mean squared error (MSE) minimization problem is non-convex. To address this challenge, a block-coordinate descent-based iterative scheme is proposed to obtain the fully digital TPC and RC matrices followed by the simultaneous orthogonal matching pursuit (SOMP) technique for decomposing the fully-digital transceiver into its corresponding RF and BB components. A theoretical proof of the convergence is also presented for the proposed iterative design procedure. Furthermore, robust hybrid transceiver designs are also derived for a practical scenario in the face of channel state information (CSI) uncertainty. The centralized MMSE lower bound has also been derived that benchmarks the performance of the proposed LDE schemes. Finally, our numerical results characterize the performance of the proposed transceivers as well as corroborate our various analytical propositions.
4.Lyapunov-Driven Deep Reinforcement Learning for Edge Inference Empowered by Reconfigurable Intelligent Surfaces
Authors:Kyriakos Stylianopoulos, Mattia Merluzzi, Paolo Di Lorenzo, George C. Alexandropoulos
Abstract: In this paper, we propose a novel algorithm for energy-efficient, low-latency, accurate inference at the wireless edge, in the context of 6G networks endowed with reconfigurable intelligent surfaces (RISs). We consider a scenario where new data are continuously generated/collected by a set of devices and are handled through a dynamic queueing system. Building on the marriage between Lyapunov stochastic optimization and deep reinforcement learning (DRL), we devise a dynamic learning algorithm that jointly optimizes the data compression scheme, the allocation of radio resources (i.e., power, transmission precoding), the computation resources (i.e., CPU cycles), and the RIS reflectivity parameters (i.e., phase shifts), with the aim of performing energy-efficient edge classification with end-to-end (E2E) delay and inference accuracy constraints. The proposed strategy enables dynamic control of the system and of the wireless propagation environment, performing a low-complexity optimization on a per-slot basis while dealing with time-varying radio channels and task arrivals, whose statistics are unknown. Numerical results assess the performance of the proposed RIS-empowered edge inference strategy in terms of trade-off between energy, delay, and accuracy of a classification task.
5.Near-Field 3D Localization via MIMO Radar: Cramér-Rao Bound and Estimator Design
Authors:Haocheng Hua, Jie Xu
Abstract: Future sixth-generation (6G) networks are envisioned to provide both sensing and communications functionalities by using densely deployed base stations (BSs) with massive antennas operating in millimeter wave (mmWave) and terahertz (THz). Due to the large number of antennas and the high frequency band, the sensing and communications will operate within the near-field region, thus making the conventional designs based on the far-field channel models inapplicable. This paper studies a near-field multiple-input-multiple-output (MIMO) radar sensing system, in which the transceivers with massive antennas aim to localize multiple near-field targets in the three-dimensional (3D) space. In particular, we adopt a general wavefront propagation model by considering the exact spherical wavefront with both channel phase and amplitude variations over different antennas. Besides, we consider the general transmit signal waveforms and also consider the unknown cluttered environments. Under this setup, the unknown parameters to estimate include the 3D coordinates and the complex reflection coefficients of the multiple targets, as well as the noise and interference covariance matrix. Accordingly, we derive the Cram\'er-Rao bound (CRB) for estimating the target coordinates and reflection coefficients. Next, to facilitate practical localization, we propose an efficient estimator based on the 3D approximate cyclic optimization (3D-ACO), which is obtained following the maximum likelihood (ML) criterion. Finally, numerical results show that considering the exact antenna-varying channel amplitudes achieves more accurate CRB as compared to prior works based on constant channel amplitudes across antennas, especially when the targets are close to the transceivers. It is also shown that the proposed estimator achieves localization performance close to the derived CRB, thus validating its superior performance.
6.Convergence Analysis of Over-the-Air FL with Compression and Power Control via Clipping
Authors:Haifeng Wen, Hong Xing, Osvaldo Simeone
Abstract: One of the key challenges towards the deployment of over-the-air federated learning (AirFL) is the design of mechanisms that can comply with the power and bandwidth constraints of the shared channel, while causing minimum deterioration to the learning performance as compared to baseline noiseless implementations. For additive white Gaussian noise (AWGN) channels with instantaneous per-device power constraints, prior work has demonstrated the optimality of a power control mechanism based on norm clipping. This was done through the minimization of an upper bound on the optimality gap for smooth learning objectives satisfying the Polyak-{\L}ojasiewicz (PL) condition. In this paper, we make two contributions to the development of AirFL based on norm clipping, which we refer to as AirFL-Clip. First, we provide a convergence bound for AirFLClip that applies to general smooth and non-convex learning objectives. Unlike existing results, the derived bound is free from run-specific parameters, thus supporting an offline evaluation. Second, we extend AirFL-Clip to include Top-k sparsification and linear compression. For this generalized protocol, referred to as AirFL-Clip-Comp, we derive a convergence bound for general smooth and non-convex learning objectives. We argue, and demonstrate via experiments, that the only time-varying quantities present in the bound can be efficiently estimated offline by leveraging the well-studied properties of sparse recovery algorithms.