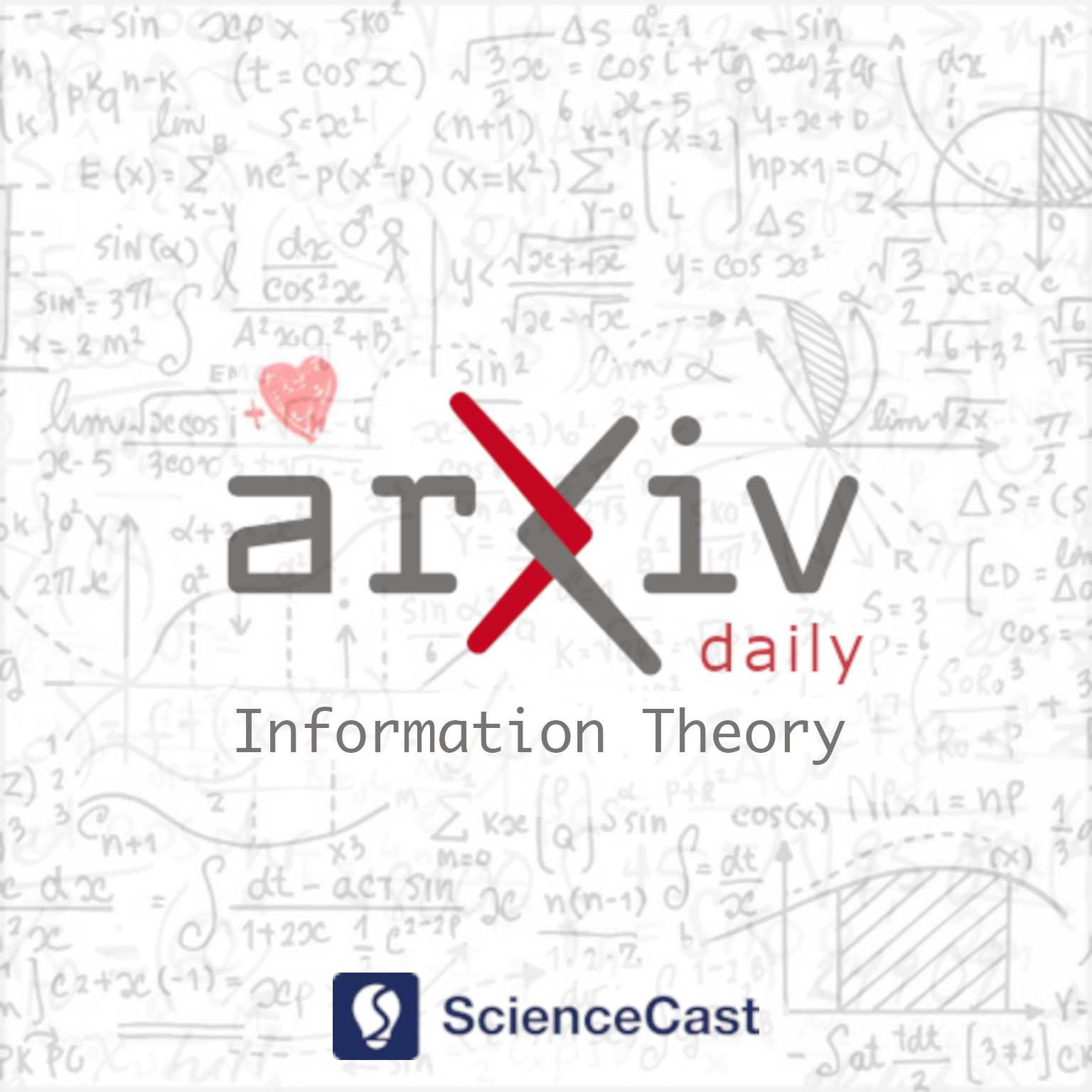
Information Theory (cs.IT)
Thu, 06 Jul 2023
1.Massive MIMO with Cauchy Noise: Channel Estimation, Achievable Rate and Data Decoding
Authors:Ziya Gulgun, Erik G. Larsson
Abstract: We consider massive multiple-input multiple-output (MIMO) systems in the presence of Cauchy noise. First, we focus on the channel estimation problem. In the standard massive MIMO setup, the users transmit orthonormal pilots during the training phase and the received signal at the base station is projected onto each pilot. This processing is optimum when the noise is Gaussian. We show that this processing is not optimal when the noise is Cauchy and as a remedy propose a channel estimation technique that operates on the raw received signal. Second, we derive uplink-downlink achievable rates in the presence of Cauchy noise for perfect and imperfect channel state information. Finally, we derive log-likelihood ratio expressions for soft bit detection for both uplink and downlink, and simulate coded bit-error-rate curves. In addition to this, we derive and compare the symbol detectors in the presence of both Gaussian and Cauchy noises. An important observation is that the detector constructed for Cauchy noise performs well with both Gaussian and Cauchy noises; on the other hand, the detector for Gaussian noise works poorly in the presence of Cauchy noise. That is, the Cauchy detector is robust against heavy-tailed noise, whereas the Gaussian detector is not.
2.Theoretical Bounds for the Size of Elementary Trapping Sets by Graphic Methods
Authors:Haoran Xiong, Zicheng Ye, Huazi Zhang, Jun Wang, Ke Liu, Dawei Yin, Guanghui Wang, Guiying Yan, Zhiming Ma
Abstract: Elementary trapping sets (ETSs) are the main culprits for the performance of LDPC codes in the error floor region. Due to the large quantity, complex structures, and computational difficulties of ETSs, how to eliminate dominant ETSs in designing LDPC codes becomes a pivotal issue to improve the error floor behavior. In practice, researchers commonly address this problem by avoiding some special graph structures to free specific ETSs in Tanner graph. In this paper, we deduce the accurate Tur\'an number of $\theta(1,2,2)$ and prove that all $(a,b)$-ETSs in Tanner graph with variable-regular degree $d_L(v)=\gamma$ must satisfy the bound $b\geq a\gamma-\frac{1}{2}a^2$, which improves the lower bound obtained by Amirzade when the girth is 6. For the case of girth 8, by limiting the relation between any two 8-cycles in the Tanner graph, we prove a similar inequality $b\geq a\gamma-\frac{a(\sqrt{8a-7}-1)}{2}$. The simulation results show that the designed codes have good performance with lower error floor over additive white Gaussian noise channels.
3.Large Language Models Empowered Autonomous Edge AI for Connected Intelligence
Authors:Yifei Shen, Jiawei Shao, Xinjie Zhang, Zehong Lin, Hao Pan, Dongsheng Li, Jun Zhang, Khaled B. Letaief
Abstract: The evolution of wireless networks gravitates towards connected intelligence, a concept that envisions seamless interconnectivity among humans, objects, and intelligence in a hyper-connected cyber-physical world. Edge AI emerges as a promising solution to achieve connected intelligence by delivering high-quality, low-latency, and privacy-preserving AI services at the network edge. In this article, we introduce an autonomous edge AI system that automatically organizes, adapts, and optimizes itself to meet users' diverse requirements. The system employs a cloud-edge-client hierarchical architecture, where the large language model, i.e., Generative Pretrained Transformer (GPT), resides in the cloud, and other AI models are co-deployed on devices and edge servers. By leveraging the powerful abilities of GPT in language understanding, planning, and code generation, we present a versatile framework that efficiently coordinates edge AI models to cater to users' personal demands while automatically generating code to train new models via edge federated learning. Experimental results demonstrate the system's remarkable ability to accurately comprehend user demands, efficiently execute AI models with minimal cost, and effectively create high-performance AI models through federated learning.
4.On the Spatial-Wideband Effects in Millimeter-Wave Cell-Free Massive MIMO
Authors:Seyoung Ahn, Soohyeong Kim, Yongseok Kwon, Joohan Park, Jiseung Youn, Sunghyun Cho
Abstract: In this paper, we investigate the spatial-wideband effects in cell-free massive MIMO (CF-mMIMO) systems in mmWave bands. The utilization of mmWave frequencies brings challenges such as signal attenuation and the need for denser networks like ultra-dense networks (UDN) to maintain communication performance. CF-mMIMO is introduced as a solution, where distributed access points (APs) transmit signals to a central processing unit (CPU) for joint processing. CF-mMIMO offers advantages in reducing non-line-of-sight (NLOS) conditions and overcoming signal blockage. We investigate the synchronization problem in CF-mMIMO due to time delays between APs. It proposes a minimum cyclic prefix length to mitigate inter-symbol interference (ISI) in OFDM systems. Furthermore, the spatial correlations of channel responses are analyzed in the frequency-phase domain. The impact of these correlations on system performance is examined. The findings contribute to improving the performance of CF-mMIMO systems and enhancing the effective utilization of mmWave communication.
5.Age-of-Information Dependent Random Access for Periodic Updating
Authors:Yuqing Zhu, Yiwen Zhu, Aoyu Gong, Yan Lin, Yijin Zhang
Abstract: This paper considers an uplink Internet of Things system with synchronous periodic traffic, where multiple devices generate their status updates at the beginning of each global frame and attempt to send them to a common access point. To achieve a low network-wide age of information (AoI) in an easily implementable manner, we require each device to adopt an age-dependent random access protocol, i.e., to transmit with a certain probability only when its corresponding AoI reaches a certain threshold. We analyze the time-average expected AoI by a multi-layer Markov model where an external infinite-horizon Markov chain manages the jumps between the beginnings of frames, while two internal finite-horizon Markov chains manage the evolution during an arbitrary frame for different cases. Simulation results verify the accuracy of the modeling and the AoI advantage over age-independent schemes.
6.Cell-Free XL-MIMO Meets Multi-Agent Reinforcement Learning: Architectures, Challenges, and Future Directions
Authors:Zhilong Liu, Jiayi Zhang, Ziheng Liu, Hongyang Du, Zhe Wang, Dusit Niyato, Mohsen Guizani, Bo Ai
Abstract: Cell-free massive multiple-input multiple-output (mMIMO) and extremely large-scale MIMO (XL-MIMO) are regarded as promising innovations for the forthcoming generation of wireless communication systems. Their significant advantages in augmenting the number of degrees of freedom have garnered considerable interest. In this article, we first review the essential opportunities and challenges induced by XL-MIMO systems. We then propose the enhanced paradigm of cell-free XL-MIMO, which incorporates multi-agent reinforcement learning (MARL) to provide a distributed strategy for tackling the problem of high-dimension signal processing and costly energy consumption. Based on the unique near-field characteristics, we propose two categories of the low-complexity design, i.e., antenna selection and power control, to adapt to different cell-free XL-MIMO scenarios and achieve the maximum data rate. For inspiration, several critical future research directions pertaining to green cell-free XL-MIMO systems are presented.
7.Performance Analysis and Approximate Message Passing Detection of Orthogonal Time Sequency Multiplexing Modulation
Authors:Zeping Sui, Shefeng Yan, Hongming Zhang, Sumei Sun, Yonghong Zeng, Lie-Liang Yang, Lajos Hanzo
Abstract: In orthogonal time sequency multiplexing (OTSM) modulation, the information symbols are conveyed in the delay-sequency domain upon exploiting the inverse Walsh Hadamard transform (IWHT). It has been shown that OTSM is capable of attaining a bit error ratio (BER) similar to that of orthogonal time-frequency space (OTFS) modulation at a lower complexity, since the saving of multiplication operations in the IWHT. Hence we provide its BER performance analysis and characterize its detection complexity. We commence by deriving its generalized input-output relationship and its unconditional pairwise error probability (UPEP). Then, its BER upper bound is derived in closed form under both ideal and imperfect channel estimation conditions, which is shown to be tight at moderate to high signal-to-noise ratios (SNRs). Moreover, a novel approximate message passing (AMP) aided OTSM detection framework is proposed. Specifically, to circumvent the high residual BER of the conventional AMP detector, we proposed a vector AMP-based expectation-maximization (VAMP-EM) detector for performing joint data detection and noise variance estimation. The variance auto-tuning algorithm based on the EM algorithm is designed for the VAMP-EM detector to further improve the convergence performance. The simulation results illustrate that the VAMP-EM detector is capable of striking an attractive BER vs. complexity trade-off than the state-of-the-art schemes as well as providing a better convergence. Finally, we propose AMP and VAMP-EM turbo receivers for low-density parity-check (LDPC)-coded OTSM systems. It is demonstrated that our proposed VAMP-EM turbo receiver is capable of providing both BER and convergence performance improvements over the conventional AMP solution.
8.On Distribution-Preserving Mitigation Strategies for Communication under Cognitive Adversaries
Authors:Soumita Hazra, J. Harshan
Abstract: In wireless security, cognitive adversaries are known to inject jamming energy on the victim's frequency band and monitor the same band for countermeasures thereby trapping the victim. Under the class of cognitive adversaries, we propose a new threat model wherein the adversary, upon executing the jamming attack, measures the long-term statistic of Kullback-Leibler Divergence (KLD) between its observations over each of the network frequencies before and after the jamming attack. To mitigate this adversary, we propose a new cooperative strategy wherein the victim takes the assistance for a helper node in the network to reliably communicate its message to the destination. The underlying idea is to appropriately split their energy and time resources such that their messages are reliably communicated without disturbing the statistical distribution of the samples in the network. We present rigorous analyses on the reliability and the covertness metrics at the destination and the adversary, respectively, and then synthesize tractable algorithms to obtain near-optimal division of resources between the victim and the helper. Finally, we show that the obtained near-optimal division of energy facilitates in deceiving the adversary with a KLD estimator.