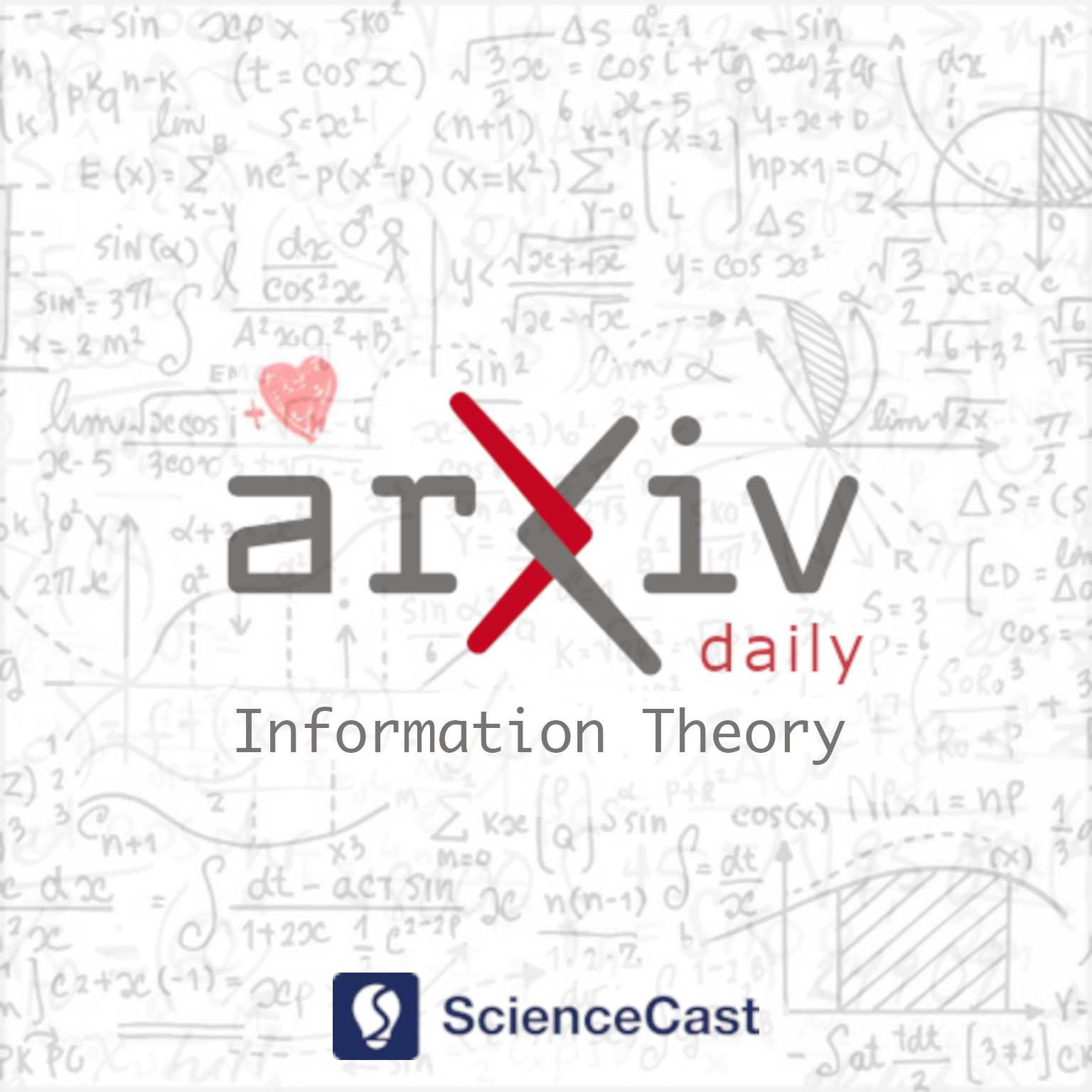
Information Theory (cs.IT)
Mon, 31 Jul 2023
1.Traffic Offloading Probability for Integrated LEO Satellite-Terrestrial Networks
Authors:Hossein Akhlaghpasand, Vahid Shah-Mansouri
Abstract: In this paper, we consider traffic offloading of integrated low earth orbit (LEO) satellite-terrestrial network. We first derive traffic offloading probability from the terrestrial network to the LEO satellite network based on the instantaneous radio signal strength. Then to overcome limited coverage and also traffic congestion of the terrestrial network, we design an optimal satellite network in which the minimum number of LEO satellites maximizes the traffic offloading probability, while probability of a generic LEO satellite being idle is close to zero. Since the satellite network is optimized regarding the intensities of the base stations (BSs) and the users, it can control the terrestrial traffic. Numerical results show that an appropriate number of LEO satellites overcomes the limited coverage in a region with low intensity of the BSs and also the traffic congestion by controlling the traffic in a region that the intensity of the users increases.
2.Acute Semigroups, the Order Bound on the Minimum Distance and the Feng-Rao Improvements
Authors:M. Bras-Amorós
Abstract: We introduce a new class of numerical semigroups, which we call the class of {\it acute} semigroups and we prove that they generalize symmetric and pseudo-symmetric numerical semigroups, Arf numerical semigroups and the semigroups generated by an interval. For a numerical semigroup $\Lambda=\{\lambda_0<\lambda_1<\dots\}$ denote $\nu_i=\#\{j\mid\lambda_i-\lambda_j\in\Lambda\}$. Given an acute numerical semigroup $\Lambda$ we find the smallest non-negative integer $m$ for which the order bound on the minimum distance of one-point Goppa codes with associated semigroup $\Lambda$ satisfies $d_{ORD}(C_i)(:=\min\{\nu_j\mid j>i\})=\nu_{i+1}$ for all $i\geq m$. We prove that the only numerical semigroups for which the sequence $(\nu_i)$ is always non-decreasing are ordinary numerical semigroups. Furthermore we show that a semigroup can be uniquely determined by its sequence $(\nu_i)$.
3.Continuous-Time Channel Prediction Based on Tensor Neural Ordinary Differential Equation
Authors:Mingyao Cui, Hao Jiang, Yuhao Chen, Yang Du, Linglong Dai
Abstract: Channel prediction is critical to address the channel aging issue in mobile scenarios. Existing channel prediction techniques are mainly designed for discrete channel prediction, which can only predict the future channel in a fixed time slot per frame, while the other intra-frame channels are usually recovered by interpolation. However, these approaches suffer from a serious interpolation loss, especially for mobile millimeter wave communications. To solve this challenging problem, we propose a tensor neural ordinary differential equation (TN-ODE) based continuous-time channel prediction scheme to realize the direct prediction of intra-frame channels. Specifically, inspired by the recently developed continuous mapping model named neural ODE in the field of machine learning, we first utilize the neural ODE model to predict future continuous-time channels. To improve the channel prediction accuracy and reduce computational complexity, we then propose the TN-ODE scheme to learn the structural characteristics of the high-dimensional channel by low dimensional learnable transform. Simulation results show that the proposed scheme is able to achieve higher intra-frame channel prediction accuracy than existing schemes.
4.Lossless Transformations and Excess Risk Bounds in Statistical Inference
Authors:László Györfi, Tamás Linder, Harro Walk
Abstract: We study the excess minimum risk in statistical inference, defined as the difference between the minimum expected loss in estimating a random variable from an observed feature vector and the minimum expected loss in estimating the same random variable from a transformation (statistic) of the feature vector. After characterizing lossless transformations, i.e., transformations for which the excess risk is zero for all loss functions, we construct a partitioning test statistic for the hypothesis that a given transformation is lossless and show that for i.i.d. data the test is strongly consistent. More generally, we develop information-theoretic upper bounds on the excess risk that uniformly hold over fairly general classes of loss functions. Based on these bounds, we introduce the notion of a delta-lossless transformation and give sufficient conditions for a given transformation to be universally delta-lossless. Applications to classification, nonparametric regression, portfolio strategies, information bottleneck, and deep learning, are also surveyed.
5.Distributed Signal Processing for Out-of-System Interference Suppression in Cell-Free Massive MIMO
Authors:Zakir Hussain Shaik, Erik G. Larsson
Abstract: Cell-free massive multiple-input-multiple-output (CF-mMIMO) is a next-generation wireless access technology that offers superior coverage and spectral efficiency compared to conventional MIMO. With many future applications in unlicensed spectrum bands, networks will likely experience and may even be limited by out-of-system (OoS) interference. The OoS interference differs from the in-system interference from other serving users in that for OoS interference, the associated pilot signals are unknown or non-existent, which makes estimation of the OoS interferer channel difficult. In this paper, we propose a novel sequential algorithm for the suppression of OoS interference for uplink CF-mMIMO with a stripe (daisy-chain) topology. The proposed method has comparable performance to that of a fully centralized interference rejection combining algorithm but has substantially less fronthaul load requirements.