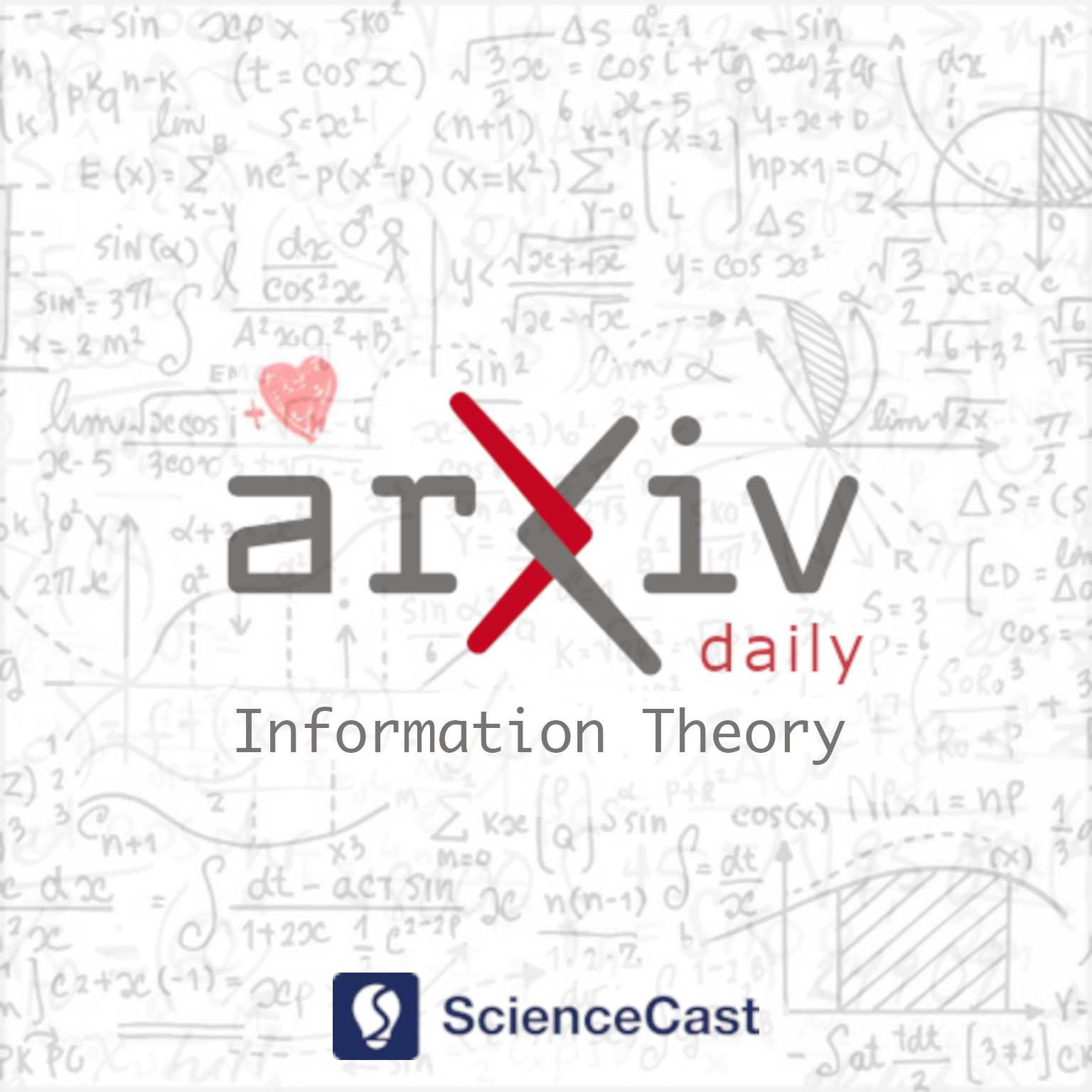
Information Theory (cs.IT)
Mon, 29 May 2023
1.The First and Second Order Asymptotics of Covert Communication over AWGN Channels
Authors:Xinchun Yu, Shuangqing Wei, Shao-Lun Huang, Xiao-Ping Zhang
Abstract: This paper investigates the asymptotics of the maximal throughput of communication over AWGN channels by $n$ channel uses under a covert constraint in terms of an upper bound $\delta$ of Kullback-Leibler divergence (KL divergence). It is shown that the first and second order asymptotics of the maximal throughput are $\sqrt{n\delta \log e}$ and $(2)^{1/2}(n\delta)^{1/4}(\log e)^{3/4}\cdot Q^{-1}(\epsilon)$, respectively. The technique we use in the achievability is quasi-$\varepsilon$-neighborhood notion from information geometry. We prove that if the generating distribution of the codebook is close to Dirac measure in the weak sense, then the corresponding output distribution at the adversary satisfies covert constraint in terms of most common divergences. This helps link the local differential geometry of the distribution of noise with covert constraint. For the converse, the optimality of Gaussian distribution for minimizing KL divergence under second order moment constraint is extended from dimension $1$ to dimension $n$. It helps to establish the upper bound on the average power of the code to satisfy the covert constraint, which further leads to the direct converse bound in terms of covert metric.
2.Computation Offloading for Edge Computing in RIS-Assisted Symbiotic Radio Systems
Authors:Bin Li, Zhen Qian, Lei Liu, Yuan Wu, Dapeng Lan, Celimuge Wu
Abstract: In the paper, we investigate the coordination process of sensing and computation offloading in a reconfigurable intelligent surface (RIS)-aided base station (BS)-centric symbiotic radio (SR) systems. Specifically, the Internet-of-Things (IoT) devices first sense data from environment and then tackle the data locally or offload the data to BS for remote computing, while RISs are leveraged to enhance the quality of blocked channels and also act as IoT devices to transmit its sensed data. To explore the mechanism of cooperative sensing and computation offloading in this system, we aim at maximizing the total completed sensed bits of all users and RISs by jointly optimizing the time allocation parameter, the passive beamforming at each RIS, the transmit beamforming at BS, and the energy partition parameters for all users subject to the size of sensed data, energy supply and given time cycle. The formulated nonconvex problem is tightly coupled by the time allocation parameter and involves the mathematical expectations, which cannot be solved straightly. We use Monte Carlo and fractional programming methods to transform the nonconvex objective function and then propose an alternating optimization-based algorithm to find an approximate solution with guaranteed convergence. Numerical results show that the RIS-aided SR system outperforms other benchmarks in sensing. Furthermore, with the aid of RIS, the channel and system performance can be significantly improved.
3.Integrated Sensing and Communication Complex CNN CSI Enhancer for 6G Networks
Authors:Xu Chen, Zhiyong Feng, J. Andrew Zhang, Xin Yuan, Ping Zhang
Abstract: In this paper, we propose a novel integrated sensing and communication (ISAC) complex convolution neural network (CNN) CSI enhancer for 6G networks, which exploits the correlation between the sensing parameters, such as angle-of-arrival (AoA) and range, and the channel state information (CSI) to significantly improve the CSI estimation accuracy and further enhance the sensing accuracy. The ISAC complex CNN CSI enhancer uses the complex-value computation layers to form the CNN to better maintain the phase information of CSI. Furthermore, we incorporate the ISAC transform modules into the CNN enhancer to transform the CSI into the sparse angle-delay domain, which can be treated as images with prominent peaks and are suitable to be processed by CNN. Then, we further propose a novel biased FFT-based sensing scheme, where we actively add known phase bias terms to the original CSI to generate multiple estimation results using a simple FFT-based sensing method, and we finally calculate the average of all the debiased sensing results to obtain more accurate range estimates. The extensive simulation results show that the ISAC complex CNN CSI enhancer can converge within 30 training epochs. Its CSI estimation normalized mean square error (NMSE) is about 17 dB lower than the MMSE method, and the bit error rate (BER) of demodulation using the enhanced CSI approaches the perfect CSI. Finally, the range estimation MSE of the proposed biased FFT-based sensing method can approach the subspace-based method with much lower complexity.
4.Performance Analysis of Discrete-Phase-Shifter IRS-aided Amplify-and-Forward Relay Network
Authors:Rongen Dong, Zhongyi Xie, Feng Shu, Mengxing Huang, Jiangzhou Wang
Abstract: As a new technology to reconfigure wireless communication environment by signal reflection controlled by software, intelligent reflecting surface (IRS) has attracted lots of attention in recent years. Compared with conventional relay system, the relay system aided by IRS can effectively reduce the cost and energy consumption, and significantly enhance the system performance. However, the phase quantization error generated by IRS with discrete phase shifter may degrade the receiving performance of the receiver. To analyze the performance loss caused by IRS phase quantization error, based on the law of large numbers and Rayleigh distribution, the closed-form expressions for the signal-to-noise ratio (SNR) performance loss and achievable rate of the IRS-aided amplify-and-forward (AF) relay network, which are related to the number of phase shifter quantization bits, are derived under the line-of-sight (LoS) channels and Rayleigh channels, respectively. Moreover, their approximate performance loss closed-form expressions are also derived based on the Taylor series expansion. Simulation results show that the performance losses of SNR and achievable rate decrease with the number of quantization bits increases gradually. When the number of quantization bits is larger than or equal to 3, the SNR performance loss of the system is smaller than 0.23dB, and the achievable rate loss is less than 0.04bits/s/Hz, regardless of the LoS channels or Rayleigh channels.