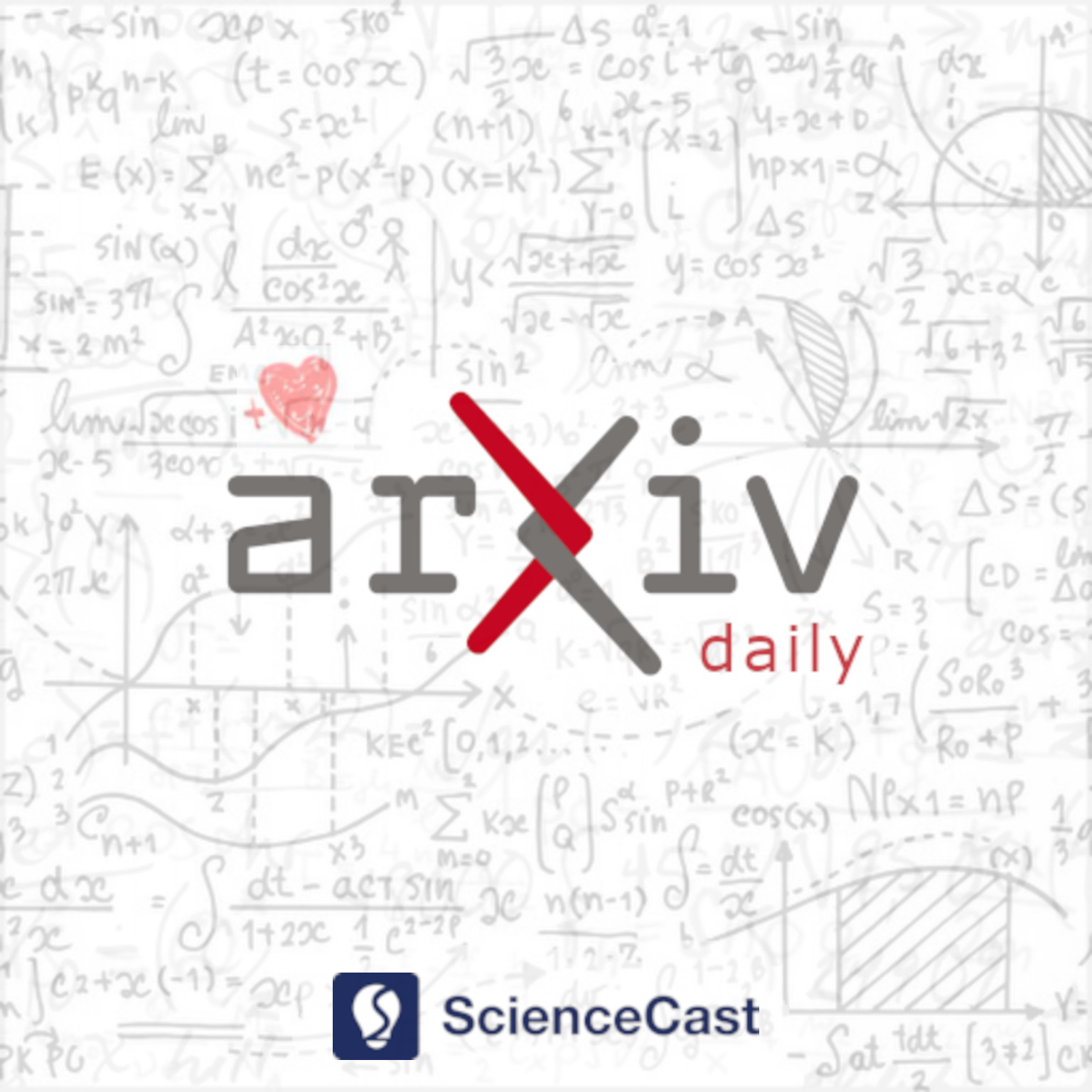
Optimization and Control (math.OC)
Mon, 10 Apr 2023
1.Closing Duality Gaps of SDPs through Perturbation
Authors:Takashi Tsuchiya, Bruno F. Lourenço, Masakazu Muramatsu, Takayuki Okuno
Abstract: Let $({\bf P},{\bf D})$ be a primal-dual pair of SDPs with a nonzero finite duality gap. Under such circumstances, ${\bf P}$ and ${\bf D}$ are weakly feasible and if we perturb the problem data to recover strong feasibility, the (common) optimal value function $v$ as a function of the perturbation is not well-defined at zero (unperturbed data) since there are ``two different optimal values'' $v({\bf P})$ and $v({\bf D})$, where $v({\bf P})$ and $v({\bf D})$ are the optimal values of ${\bf P}$ and ${\bf D}$ respectively. Thus, continuity of $v$ is lost at zero though $v$ is continuous elsewhere. Nevertheless, we show that a limiting version ${v_a}$ of $v$ is a well-defined monotone decreasing continuous bijective function connecting $v({\bf P})$ and $v({\bf D})$ with domain $[0, \pi/2]$ under the assumption that both ${\bf P}$ and ${\bf D}$ have singularity degree one. The domain $[0,\pi/2]$ corresponds to directions of perturbation defined in a certain manner. Thus, ${v_a}$ ``completely fills'' the nonzero duality gap under a mild regularity condition. Our result is tight in that there exists an instance with singularity degree two for which ${v_a}$ is not continuous.
2.Fourier-Gegenbauer Pseudospectral Method for Solving Periodic Fractional Optimal Control Problems
Authors:Kareem T. Elgindy
Abstract: This paper introduces a new accurate model for periodic fractional optimal control problems (PFOCPs) using Riemann-Liouville (RL) and Caputo fractional derivatives (FDs) with sliding fixed memory lengths. The paper also provides a novel numerical method for solving PFOCPs using Fourier and Gegenbauer pseudospectral methods. By employing Fourier collocation at equally spaced nodes and Fourier and Gegenbauer quadratures, the method transforms the PFOCP into a simple constrained nonlinear programming problem (NLP) that can be treated easily using standard NLP solvers. We propose a new transformation that largely simplifies the problem of calculating the periodic FDs of periodic functions to the problem of evaluating the integral of the first derivatives of their trigonometric Lagrange interpolating polynomials, which can be treated accurately and efficiently using Gegenbauer quadratures. We introduce the notion of the {\alpha}th-order fractional integration matrix with index L based on Fourier and Gegenbauer pseudospectral approximations, which proves to be very effective in computing periodic FDs. We also provide a rigorous priori error analysis to predict the quality of the Fourier-Gegenbauer-based approximations to FDs. The numerical results of the benchmark PFOCP demonstrate the performance of the proposed pseudospectral method.
3.Inexact Online Proximal Mirror Descent for time-varying composite optimization
Authors:Woocheol Choi, Myeong-Su Lee, Seok-Bae Yun
Abstract: In this paper, we consider the online proximal mirror descent for solving the time-varying composite optimization problems. For various applications, the algorithm naturally involves the errors in the gradient and proximal operator. We obtain sharp estimates on the dynamic regret of the algorithm when the regular part of the cost is convex and smooth. If the Bregman distance is given by the Euclidean distance, our result also improves the previous work in two ways: (i) We establish a sharper regret bound compared to the previous work in the sense that our estimate does not involve $O(T)$ term appearing in that work. (ii) We also obtain the result when the domain is the whole space $\mathbb{R}^n$, whereas the previous work was obtained only for bounded domains. We also provide numerical tests for problems involving the errors in the gradient and proximal operator.
4.First-order methods for Stochastic Variational Inequality problems with Function Constraints
Authors:Digvijay Boob, Qi Deng
Abstract: The monotone Variational Inequality (VI) is an important problem in machine learning. In numerous instances, the VI problems are accompanied by function constraints which can possibly be data-driven, making the projection operator challenging to compute. In this paper, we present novel first-order methods for function constrained VI (FCVI) problem under various settings, including smooth or nonsmooth problems with a stochastic operator and/or stochastic constraints. First, we introduce the~{\texttt{OpConEx}} method and its stochastic variants, which employ extrapolation of the operator and constraint evaluations to update the variables and the Lagrangian multipliers. These methods achieve optimal operator or sample complexities when the FCVI problem is either (i) deterministic nonsmooth, or (ii) stochastic, including smooth or nonsmooth stochastic constraints. Notably, our algorithms are simple single-loop procedures and do not require the knowledge of Lagrange multipliers to attain these complexities. Second, to obtain the optimal operator complexity for smooth deterministic problems, we present a novel single-loop Adaptive Lagrangian Extrapolation~(\texttt{AdLagEx}) method that can adaptively search for and explicitly bound the Lagrange multipliers. Furthermore, we show that all of our algorithms can be easily extended to saddle point problems with coupled function constraints, hence achieving similar complexity results for the aforementioned cases. To our best knowledge, many of these complexities are obtained for the first time in the literature.
5.Dynamically adaptive networks for integrating optimal pressure management and self-cleaning controls
Authors:Bradley Jenks, Aly-Joy Ulusoy, Filippo Pecci, Ivan Stoianov
Abstract: This paper investigates the problem of integrating optimal pressure management and self-cleaning controls in dynamically adaptive water distribution networks. We review existing single-objective valve placement and control problems for minimizing average zone pressure (AZP) and maximizing self-cleaning capacity (SCC). Since AZP and SCC are conflicting objectives, we formulate a bi-objective design-for-control problem where locations and operational settings of pressure control and automatic flushing valves are jointly optimized. We approximate Pareto fronts using the weighted sum scalarization method, which uses a previously developed convex heuristic to solve the sequence of parametrized single-objective problems. The resulting Pareto fronts suggest that significant improvements in SCC can be achieved for minimal trade-offs in AZP performance. Moreover, we demonstrate that a hierarchical design strategy is capable of yielding good quality solutions to both objectives. This hierarchical design considers pressure control valves first placed for the primary AZP objective, followed by automatic flushing valves placed to augment SCC conditions. In addition, we investigate an adaptive control scheme for dynamically transitioning between AZP and SCC controls. We demonstrate these control challenges on case networks with both interconnected and branched topology.
6.Gradient and Hessian of functions with non-independent variables
Authors:Matieyendou Lamboni
Abstract: Mathematical models are sometime given as functions of independent input variables and equations or inequations connecting the input variables. A probabilistic characterization of such models results in treating them as functions with non-independent variables. Using the distribution function or copula of such variables that comply with such equations or inequations, we derive two types of partial derivatives of functions with non-independent variables (i.e., actual and dependent derivatives) and argue in favor of the latter. The dependent partial derivatives of functions with non-independent variables rely on the dependent Jacobian matrix of dependent variables, which is also used to define a tensor metric. The differential geometric framework allows for deriving the gradient, Hessian and Taylor-type expansion of functions with non-independent variables.
7.Iterative Singular Tube Hard Thresholding Algorithms for Tensor Completion
Authors:Rachel Grotheer, Shuang Li, Anna Ma, Deanna Needell, Jing Qin
Abstract: Due to the explosive growth of large-scale data sets, tensors have been a vital tool to analyze and process high-dimensional data. Different from the matrix case, tensor decomposition has been defined in various formats, which can be further used to define the best low-rank approximation of a tensor to significantly reduce the dimensionality for signal compression and recovery. In this paper, we consider the low-rank tensor completion problem. We propose a novel class of iterative singular tube hard thresholding algorithms for tensor completion based on the low-tubal-rank tensor approximation, including basic, accelerated deterministic and stochastic versions. Convergence guarantees are provided along with the special case when the measurements are linear. Numerical experiments on tensor compressive sensing and color image inpainting are conducted to demonstrate convergence and computational efficiency in practice.
8.Finite Element Error Analysis and Solution Stability of affine optimal control problems
Authors:Nicolai Jork
Abstract: We consider affine optimal control problems subject to semilinear elliptic PDEs. The results are two-fold; first, we continue the analysis of solution stability of control problems under perturbations appearing jointly in the objective functional and the PDE. For this, we consider a coercivity-type property that is common in the field of optimal control. The second result is concerned with the obtainment of error estimates for the numerical approximation for a finite element and a variational discretization scheme. The error estimates for the optimal controls and states are obtained under several conditions of different strengths that appeared recently in the context of solution stability. This includes an improvement of error estimates for the optimal controls and states under a H\"older-type growth condition.