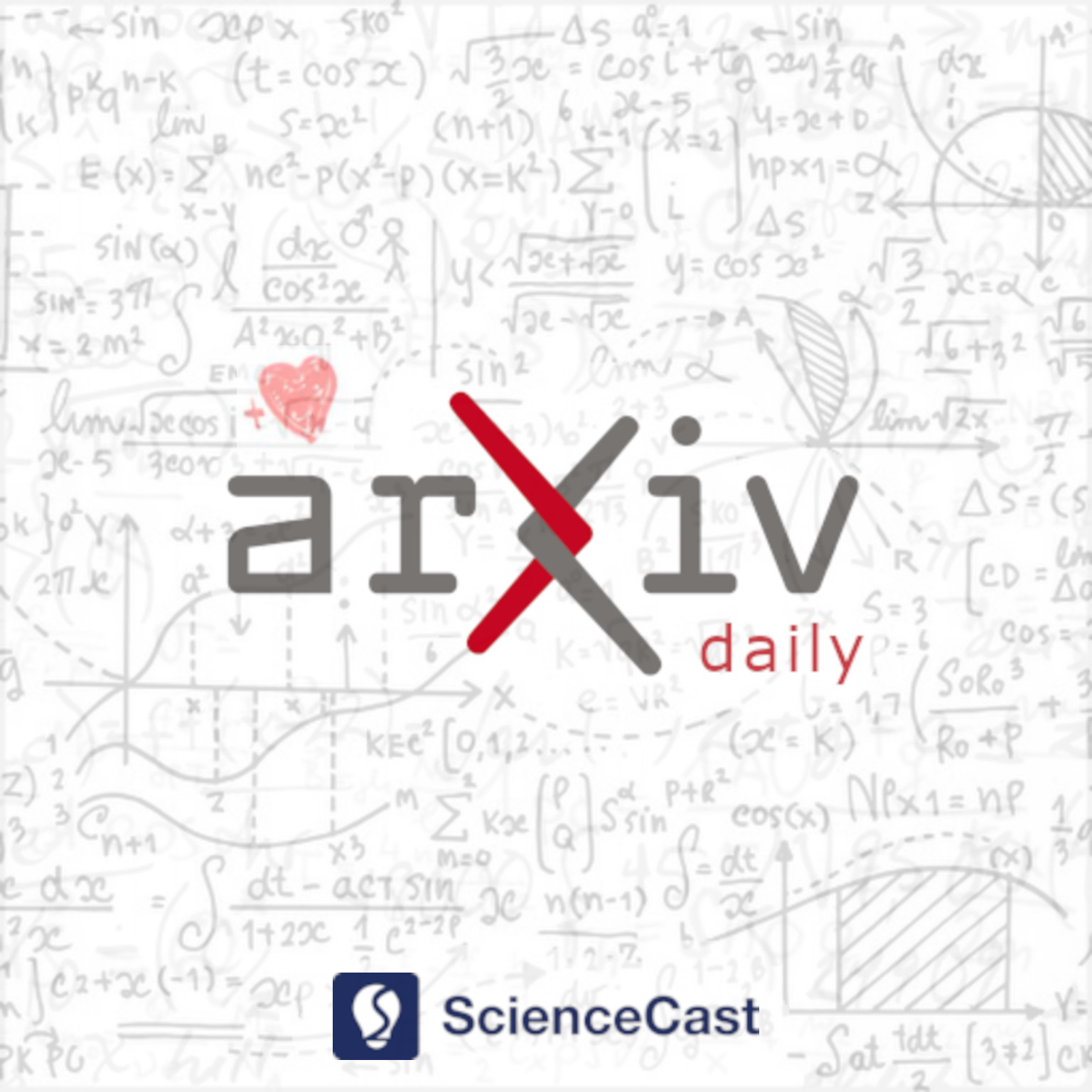
Optimization and Control (math.OC)
Thu, 11 May 2023
1.A Robust Control Approach to Asymptotic Optimality of the Heavy Ball Method for Optimization of Quadratic Functions
Authors:V. Ugrinovskii, I. R. Petersen, I. Shames
Abstract: Among first order optimization methods, Polyak's heavy ball method has long been known to guarantee the asymptotic rate of convergence matching Nesterov's lower bound for functions defined in an infinite-dimensional space. In this paper, we use results on the robust gain margin of linear uncertain feedback control systems to show that the heavy ball method is provably worst-case asymptotically optimal when applied to quadratic functions in a finite dimensional space.
2.Time-Reversed Dissipation Induces Duality Between Minimizing Gradient Norm and Function Value
Authors:Jaeyeon Kim, Asuman Ozdaglar, Chanwoo Park, Ernest K. Ryu
Abstract: In convex optimization, first-order optimization methods efficiently minimizing function values have been a central subject study since Nesterov's seminal work of 1983. Recently, however, Kim and Fessler's OGM-G and Lee et al.'s FISTA-G have been presented as alternatives that efficiently minimize the gradient magnitude instead. In this paper, we present H-duality, which represents a surprising one-to-one correspondence between methods efficiently minimizing function values and methods efficiently minimizing gradient magnitude. In continuous-time formulations, H-duality corresponds to reversing the time dependence of the dissipation/friction term. To the best of our knowledge, H-duality is different from Lagrange/Fenchel duality and is distinct from any previously known duality or symmetry relations. Using H-duality, we obtain a clearer understanding of the symmetry between Nesterov's method and OGM-G, derive a new class of methods efficiently reducing gradient magnitudes of smooth convex functions, and find a new composite minimization method that is simpler and faster than FISTA-G.
3.Linear System Analysis and Optimal Control of Natural Gas Dynamics in Pipeline Networks
Authors:Luke S. Baker, Sachin Shivakumar, Dieter Armbruster, Rodrigo B. Platte, Anatoly Zlotnik
Abstract: We derive a linear system of ordinary differential equations (ODEs) to approximate the dynamics of natural gas in pipeline networks. Although a closed-form expression of the eigenvalues of the state matrix does not generally exist, the poles of an irrational transfer function corresponding to the linearized partial differential equations are used to approximate the eigenvalues of the ODE system. Our analysis qualitatively demonstrates that the eigenvalues of the state matrix of the entire network system are "pipeline separable" in the sense that the eigenvalues are dominated by the individual pipeline parameters and not the incidence connectivity of the network graph. The linear system is used as the dynamic constraints of a linear optimal control problem (OCP) to design the control actions of compressor units to minimize the energy that they expend. The motivation of this work is to reduce the computational complexity of optimizing gas dynamics in large networks to meet the unpredictable and highly variable demand from electric generators. The linear and corresponding nonlinear OCPs are discretized in time to obtain linear and nonlinear optimization problems, which are demonstrated on a test network to illustrate the validity of linear programming. Moreover, an analytical bound on the error between the solutions of the linear and nonlinear flow dynamics is presented using Lyapunov functions and verified computationally by plotting the error against the size of the flow variation around the steady-state solution.
4.Regularization properties of dual subgradient flow
Authors:Vassilis Apidopoulos, Cesare Molinari, Lorenzo Rosasco, Silvia Villa
Abstract: Dual gradient descent combined with early stopping represents an efficient alternative to the Tikhonov variational approach when the regularizer is strongly convex. However, for many relevant applications, it is crucial to deal with regularizers which are only convex. In this setting, the dual problem is non smooth, and dual gradient descent cannot be used. In this paper, we study the regularization properties of a subgradient dual flow, and we show that the proposed procedure achieves the same recovery accuracy as penalization methods, while being more efficient from the computational perspective.
5.Lagrange Multipliers in locally convex spaces
Authors:Mohammed Bachir, Joel Blot
Abstract: We give a general Lagrange multiplier rule for mathematical programming problems in a Hausdorff locally convex space. We consider infinitely many inequality and equality constraints. Our results gives in particular a generalisation of the result of J. Jahn in \cite{Ja}, replacing Fr\'echet-differentiability assumptions on the functions by the Gateaux-differentiability. Moreover, the closed convex cone with a nonempty interior in the constraints is replaced by a strictly general class of closed subsets introduced in the paper and called {\it "admissible sets"}. Examples illustrating our results are given.
6.Multiplier rules for Dini-derivatives in a topological vector space
Authors:Mohammed Bachir, Rongzhen Lyu
Abstract: We provide new results of first-order necessary conditions of optimality problem in the form of John's theorem and in the form of Karush-Kuhn-Tucker's theorem. We establish our result in a topological vector space for problems with inequality constraints and in a Banach space for problems with equality and inequality constraints. Our contributions consist in the extension of the results known for the Fr\'echet and Gateaux-differentiable functions as well as for the Clarke's subdifferential of Lipschitz functions, to the more general Dini-differentiable functions. As consequences, we extend the result of B.H. Pourciau in \cite[Theorem 6, p. 445]{Po} from the convexity to the {\it "Dini-pseudoconvexity"}.
7.Alternating mixed-integer programming and neural network training for approximating stochastic two-stage problems
Authors:Jan Kronqvist, Boda Li, Jan Rolfes, Shudian Zhao
Abstract: The presented work addresses two-stage stochastic programs (2SPs), a broadly applicable model to capture optimization problems subject to uncertain parameters with adjustable decision variables. In case the adjustable or second-stage variables contain discrete decisions, the corresponding 2SPs are known to be NP-complete. The standard approach of forming a single-stage deterministic equivalent problem can be computationally challenging even for small instances, as the number of variables and constraints scales with the number of scenarios. To avoid forming a potentially huge MILP problem, we build upon an approach of approximating the expected value of the second-stage problem by a neural network (NN) and encoding the resulting NN into the first-stage problem. The proposed algorithm alternates between optimizing the first-stage variables and retraining the NN. We demonstrate the value of our approach with the example of computing operating points in power systems by showing that the alternating approach provides improved first-stage decisions and a tighter approximation between the expected objective and its neural network approximation.
8.Stochastic Variance-Reduced Majorization-Minimization Algorithms
Authors:Duy-Nhat Phan, Sedi Bartz, Nilabja Guha, Hung M. Phan
Abstract: We study a class of nonconvex nonsmooth optimization problems in which the objective is a sum of two functions: One function is the average of a large number of differentiable functions, while the other function is proper, lower semicontinuous and has a surrogate function that satisfies standard assumptions. Such problems arise in machine learning and regularized empirical risk minimization applications. However, nonconvexity and the large-sum structure are challenging for the design of new algorithms. Consequently, effective algorithms for such scenarios are scarce. We introduce and study three stochastic variance-reduced majorization-minimization (MM) algorithms, combining the general MM principle with new variance-reduced techniques. We provide almost surely subsequential convergence of the generated sequence to a stationary point. We further show that our algorithms possess the best-known complexity bounds in terms of gradient evaluations. We demonstrate the effectiveness of our algorithms on sparse binary classification problems, sparse multi-class logistic regressions, and neural networks by employing several widely-used and publicly available data sets.