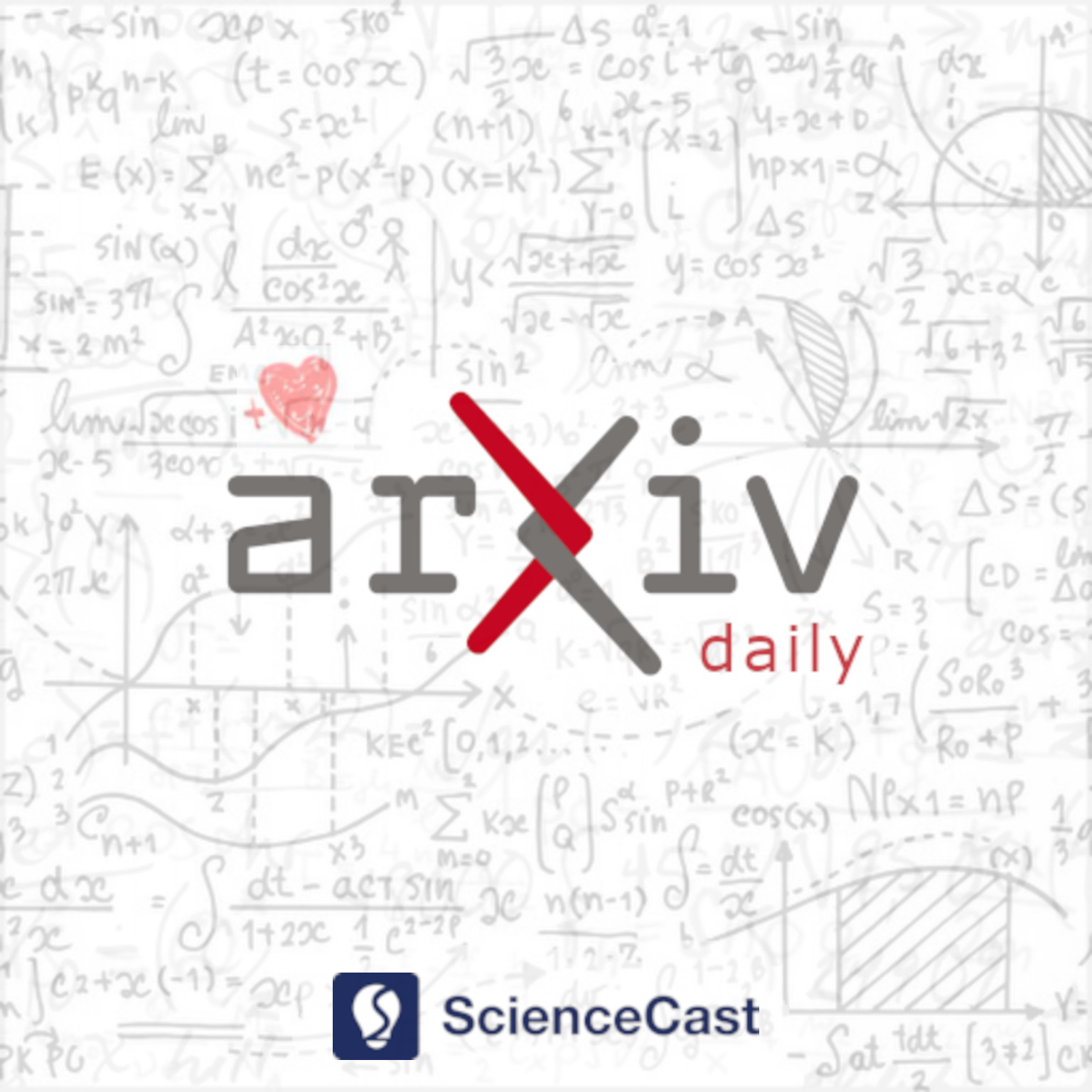
Optimization and Control (math.OC)
Fri, 04 Aug 2023
1.Optimization on Pareto sets: On a theory of multi-objective optimization
Authors:Abhishek Roy, Geelon So, Yi-An Ma
Abstract: In multi-objective optimization, a single decision vector must balance the trade-offs between many objectives. Solutions achieving an optimal trade-off are said to be Pareto optimal: these are decision vectors for which improving any one objective must come at a cost to another. But as the set of Pareto optimal vectors can be very large, we further consider a more practically significant Pareto-constrained optimization problem, where the goal is to optimize a preference function constrained to the Pareto set. We investigate local methods for solving this constrained optimization problem, which poses significant challenges because the constraint set is (i) implicitly defined, and (ii) generally non-convex and non-smooth, even when the objectives are. We define notions of optimality and stationarity, and provide an algorithm with a last-iterate convergence rate of $O(K^{-1/2})$ to stationarity when the objectives are strongly convex and Lipschitz smooth.
2.Completely Abstract Dynamic Programming
Authors:Thomas J. Sargent, John Stachurski
Abstract: We introduce a completely abstract dynamic programming framework in which dynamic programs are sets of policy operators acting on a partially ordered space. We provide an optimality theory based on high-level assumptions. We then study symmetric and asymmetric relationships between dynamic programs, and show how these relationships transmit optimality properties. Our formulation includes and extends applications of dynamic programming across many fields.
3.Optimal Control of Stationary Doubly Diffusive Flows on Two and Three Dimensional Bounded Lipschitz Domains: A Theoretical Study
Authors:Jai Tushar, Arbaz Khan, Manil T. Mohan
Abstract: In this work, a theoretical framework is developed to study the control constrained distributed optimal control of a stationary double diffusion model presented in [Burger, Mendez, Ruiz-Baier, SINUM (2019), 57:1318-1343]. For the control problem, as the source term belongs to a weaker space, a new solvability analysis of the governing equation is presented using Faedo- Galerkin approximation techniques. Some new minimal regularity results for the governing equation are established on two and three-dimensional bounded Lipschitz domains and are of independent interest. Moreover, we show the existence of an optimal control with quadratic type cost functional, study the Frechet differentiability properties of the control-to-state map and establish the first-order necessary optimality conditions corresponding to the optimal control problem.
4.Adaptive Proximal Gradient Method for Convex Optimization
Authors:Yura Malitsky, Konstantin Mishchenko
Abstract: In this paper, we explore two fundamental first-order algorithms in convex optimization, namely, gradient descent (GD) and proximal gradient method (ProxGD). Our focus is on making these algorithms entirely adaptive by leveraging local curvature information of smooth functions. We propose adaptive versions of GD and ProxGD that are based on observed gradient differences and, thus, have no added computational costs. Moreover, we prove convergence of our methods assuming only local Lipschitzness of the gradient. In addition, the proposed versions allow for even larger stepsizes than those initially suggested in [MM20].
5.Blessing of High-Order Dimensionality: from Non-Convex to Convex Optimization for Sensor Network Localization
Authors:Mingyu Lei, Jiayu Zhang, Yinyu Ye
Abstract: This paper investigates the Sensor Network Localization (SNL) problem, which seeks to determine sensor locations based on known anchor locations and partially given anchors-sensors and sensors-sensors distances. Two primary methods for solving the SNL problem are analyzed: the low-dimensional method that directly minimizes a loss function, and the high-dimensional semi-definite relaxation (SDR) method that reformulates the SNL problem as an SDP (semi-definite programming) problem. The paper primarily focuses on the intrinsic non-convexity of the loss function of the low-dimensional method, which is shown in our main theorem. The SDR method, via second-order dimension augmentation, is discussed in the context of its ability to transform non-convex problems into convex ones; while the first-order direct dimension augmentation fails. Additionally, we will show that more edges don't necessarily contribute to the better convexity of the loss function. Moreover, we provide an explanation for the success of the SDR+GD (gradient descent) method which uses the SDR solution as a warm-start of the minimization of the loss function by gradient descent. The paper also explores the parallels among SNL, max-cut, and neural networks in terms of the blessing of high-order dimension augmentation.
6.Approximation of deterministic mean field type control systems
Authors:Yurii Averboukh
Abstract: The paper is concerned with the approximation of the deterministic the mean field type control system by a mean field Markov chain. It turns out that the dynamics of the distribution in the approximating system is described by a system of ordinary differential equations. Given a strategy for the Markov chain, we explicitly construct a control in the deterministic mean field type control system. Our method is a realization of the model predictive approach. The converse construction is also presented. These results lead to an estimate of the Hausdorff distance between the bundles of motions in the deterministic mean field type control system and the mean field Markov chain. Especially, we pay the attention to the case when one can approximate the bundle of motions in the mean field type system by solutions of a finite systems of ODEs.