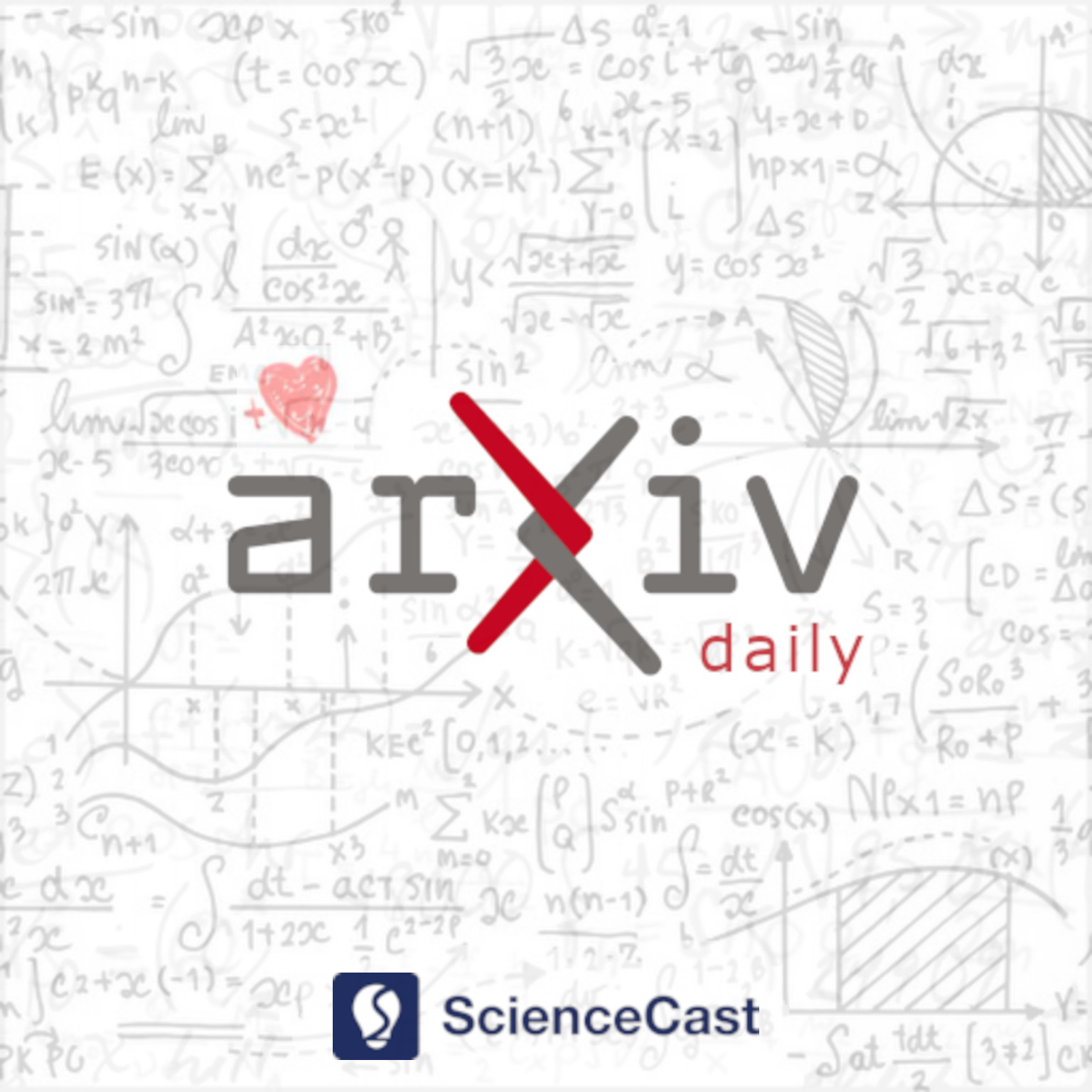
Optimization and Control (math.OC)
Fri, 30 Jun 2023
1.Calm local optimality for nonconvex-nonconcave minimax problems
Authors:Xiaoxiao Ma, Wei Yao, Jane J. Ye, Jin Zhang
Abstract: Nonconvex-nonconcave minimax problems have found numerous applications in various fields including machine learning. However, questions remain about what is a good surrogate for local minimax optimum and how to characterize the minimax optimality. Recently Jin, Netrapalli, and Jordan (ICML 2020) introduced a concept of local minimax point and derived optimality conditions for the smooth and unconstrained case. In this paper, we introduce the concept of calm local minimax point, which is a local minimax point with a calm radius function. With the extra calmness property we obtain first and second-order sufficient and necessary optimality conditions for a very general class of nonsmooth nonconvex-nonconcave minimax problem. Moreover we show that the calm local minimax optimality and the local minimax optimality coincide under a weak sufficient optimality condition for the maximization problem. This equivalence allows us to derive stronger optimality conditions under weaker assumptions for local minimax optimality.
2.Impulse control with generalised discounting
Authors:Damian Jelito, Łukasz Stettner
Abstract: In this paper, we investigate the effects of applying generalised (non-exponential) discounting on a long-run impulse control problem for a Feller-Markov process. We show that the optimal value of the discounted problem is the same as the optimal value of its undiscounted version. Next, we prove that an optimal strategy for the undiscounted discrete time functional is also optimal for the discrete-time discounted criterion and nearly optimal for the continuous-time discounted one. This shows that the discounted problem, being time-inconsistent in nature, admits a time-consistent solution. Also, instead of a complex time-dependent Bellman equation one may consider its simpler time-independent version.
3.An Oblivious Stochastic Composite Optimization Algorithm for Eigenvalue Optimization Problems
Authors:Clément Lezane, Cristóbal Guzmán, Alexandre d'Aspremont
Abstract: In this work, we revisit the problem of solving large-scale semidefinite programs using randomized first-order methods and stochastic smoothing. We introduce two oblivious stochastic mirror descent algorithms based on a complementary composite setting. One algorithm is designed for non-smooth objectives, while an accelerated version is tailored for smooth objectives. Remarkably, both algorithms work without prior knowledge of the Lipschitz constant or smoothness of the objective function. For the non-smooth case with $\mathcal{M}-$bounded oracles, we prove a convergence rate of $ O( {\mathcal{M}}/{\sqrt{T}} ) $. For the $L$-smooth case with a feasible set bounded by $D$, we derive a convergence rate of $ O( {L^2 D^2}/{(T^{2}\sqrt{T})} + {(D_0^2+\sigma^2)}/{\sqrt{T}} )$, where $D_0$ is the starting distance to an optimal solution, and $ \sigma^2$ is the stochastic oracle variance. These rates had only been obtained so far by either assuming prior knowledge of the Lipschitz constant or the starting distance to an optimal solution. We further show how to extend our framework to relative scale and demonstrate the efficiency and robustness of our methods on large scale semidefinite programs.
4.Convergence property of the Quantized Distributed Gradient descent with constant stepsizes and an effective strategy for the stepsize selection
Authors:Woocheol Choi, Myeong-Su Lee
Abstract: In this paper, we establish new convergence results for the quantized distributed gradient descent and suggest a novel strategy of choosing the stepsizes for the high-performance of the algorithm. Under the strongly convexity assumption on the aggregate cost function and the smoothness assumption on each local cost function, we prove the algorithm converges exponentially fast to a small neighborhood of the optimizer whose radius depends on the stepsizes. Based on our convergence result, we suggest an effective selection of stepsizes which repeats diminishing the stepsizes after a number of specific iterations. Both the convergence results and the effectiveness of the suggested stepsize selection are also verified by the numerical experiments.
5.Homogeneous Second-Order Descent Framework: A Fast Alternative to Newton-Type Methods
Authors:Chang He, Yuntian Jiang, Chuwen Zhang, Dongdong Ge, Bo Jiang, Yinyu Ye
Abstract: This paper proposes a homogeneous second-order descent framework (HSODF) for nonconvex and convex optimization based on the generalized homogeneous model (GHM). In comparison to the Newton steps, the GHM can be solved by extremal symmetric eigenvalue procedures and thus grant an advantage in ill-conditioned problems. Moreover, GHM extends the ordinary homogeneous model (OHM) to allow adaptiveness in the construction of the aggregated matrix. Consequently, HSODF is able to recover some well-known second-order methods such as trust-region methods and gradient regularized methods while maintaining comparable iteration complexity bounds. We also study two specific realizations of HSODF. One is adptive HSODM, which has a parameter-free $O(\epsilon^{-3/2})$ global complexity bound for nonconvex second-order Lipschitz continuous functions. The other one is homotopy HSODM, which is proven to have a global linear rate of convergence without strong convexity. The efficiency of our appproach on ill-conditioned and high-dimensional problems are justified by some perlimiarny numerical results.
6.The risk-sensitive optimal stopping problem: geometric solution and algorithms
Authors:Tomasz Kosmala, John Moriarty
Abstract: We use the geometry of functions associated with martingales under a risk measure to solve risk-sensitive Markovian optimal stopping problems. Generalising the risk-neutral case due to Dynkin and Yushkievich (1969), the risk-sensitive value function is the pointwise infimum of those functions which dominate the gain function. The functions are not required to be differentiable, can explode to infinity, and form a three-dimensional set, and in the differentiable case the smooth fit principle holds. Only elementary properties of the driving Markov process $X$ are used. Algorithms are provided to construct the value function, with the computational cost of a two-dimensional search.
7.Convex quartic problems: homogenized gradient method and preconditioning
Authors:Radu-Alexandru Dragomir, Yurii Nesterov
Abstract: We consider a convex minimization problem for which the objective is the sum of a homogeneous polynomial of degree four and a linear term. Such task arises as a subproblem in algorithms for quadratic inverse problems with a difference-of-convex structure. We design a first-order method called Homogenized Gradient, along with an accelerated version, which enjoy fast convergence rates of respectively $\mathcal{O}(\kappa^2/K^2)$ and $\mathcal{O}(\kappa^2/K^4)$ in relative accuracy, where $K$ is the iteration counter. The constant $\kappa$ is the quartic condition number of the problem. Then, we show that for a certain class of problems, it is possible to compute a preconditioner for which this condition number is $\sqrt{n}$, where $n$ is the problem dimension. To establish this, we study the more general problem of finding the best quadratic approximation of an $\ell_p$ norm composed with a quadratic map. Our construction involves a generalization of the so-called Lewis weights.
8.Algorithms for Shipping Container Delivery Scheduling
Authors:Anna Collins, Dimitrios Letsios, Gueorgui Mihaylov
Abstract: Motivated by distribution problems arising in the supply chain of Haleon, we investigate a discrete optimization problem that we call the "container delivery scheduling problem". The problem models a supplier dispatching ordered products with shipping containers from manufacturing sites to distribution centers, where orders are collected by the buyers at agreed due times. The supplier may expedite or delay item deliveries to reduce transshipment costs at the price of increasing inventory costs, as measured by the number of containers and distribution center storage/backlog costs, respectively. The goal is to compute a delivery schedule attaining good trade-offs between the two. This container delivery scheduling problem is a temporal variant of classic bin packing problems, where the item sizes are not fixed, but depend on the item due times and delivery times. An approach for solving the problem should specify a batching policy for container consolidation and a scheduling policy for deciding when each container should be delivered. Based on the available item due times, we develop algorithms with sequential and nested batching policies as well as on-time and delay-tolerant scheduling policies. We elaborate on the problem's hardness and substantiate the proposed algorithms with positive and negative approximation bounds, including the derivation of an algorithm achieving an asymptotically tight 2-approximation ratio.
9.Accelerating Inexact HyperGradient Descent for Bilevel Optimization
Authors:Haikuo Yang, Luo Luo, Chris Junchi Li, Michael I. Jordan
Abstract: We present a method for solving general nonconvex-strongly-convex bilevel optimization problems. Our method -- the \emph{Restarted Accelerated HyperGradient Descent} (\texttt{RAHGD}) method -- finds an $\epsilon$-first-order stationary point of the objective with $\tilde{\mathcal{O}}(\kappa^{3.25}\epsilon^{-1.75})$ oracle complexity, where $\kappa$ is the condition number of the lower-level objective and $\epsilon$ is the desired accuracy. We also propose a perturbed variant of \texttt{RAHGD} for finding an $\big(\epsilon,\mathcal{O}(\kappa^{2.5}\sqrt{\epsilon}\,)\big)$-second-order stationary point within the same order of oracle complexity. Our results achieve the best-known theoretical guarantees for finding stationary points in bilevel optimization and also improve upon the existing upper complexity bound for finding second-order stationary points in nonconvex-strongly-concave minimax optimization problems, setting a new state-of-the-art benchmark. Empirical studies are conducted to validate the theoretical results in this paper.
10.Convex Optimization in Legged Robots
Authors:Prathamesh Saraf, Mustafa Shaikh, Myron Phan
Abstract: Convex optimization is crucial in controlling legged robots, where stability and optimal control are vital. Many control problems can be formulated as convex optimization problems, with a convex cost function and constraints capturing system dynamics. Our review focuses on active balancing problems and presents a general framework for formulating them as second-order cone programming (SOCP) for robustness and efficiency with existing interior point algorithms. We then discuss some prior work around the Zero Moment Point stability criterion, Linear Quadratic Regulator Control, and then the feedback model predictive control (MPC) approach to improve prediction accuracy and reduce computational costs. Finally, these techniques are applied to stabilize the robot for jumping and landing tasks. Further research in convex optimization of legged robots can have a significant societal impact. It can lead to improved gait planning and active balancing which enhances their ability to navigate complex environments, assist in search and rescue operations and perform tasks in hazardous environments. These advancements have the potential to revolutionize industries and help humans in daily life.
11.Optimal Control of Chromate Removal via Enhanced Modeling using the Method of Moments
Authors:Fred Ghanem, Kirti M. Yenkie
Abstract: Single-use anion-exchange resins can reduce hazardous chromates to safe levels in drinking water. However, since most process control strategies monitor effluent concentrations, detection of any chromate leakage leads to premature resin replacement. Furthermore, variations in the inlet chromate concentration and other process conditions make process control a challenging step. In this work, we capture the uncertainty of the process conditions by applying the Ito process of Brownian motion with drift into a stochastic optimal control strategy. The ion exchange process is modeled using the method of moments which helps capture the process dynamics, later formulated into mathematical objectives representing desired chromate removal. We then solved our developed models as an optimal control problem via Pontryagin's maximum principle. The objectives enabled a successful control via flow rate adjustments leading to higher chromate extraction. Such an approach maximized the capacity of the resin and column efficiency to remove toxic compounds from water while capturing deviations in the process conditions.