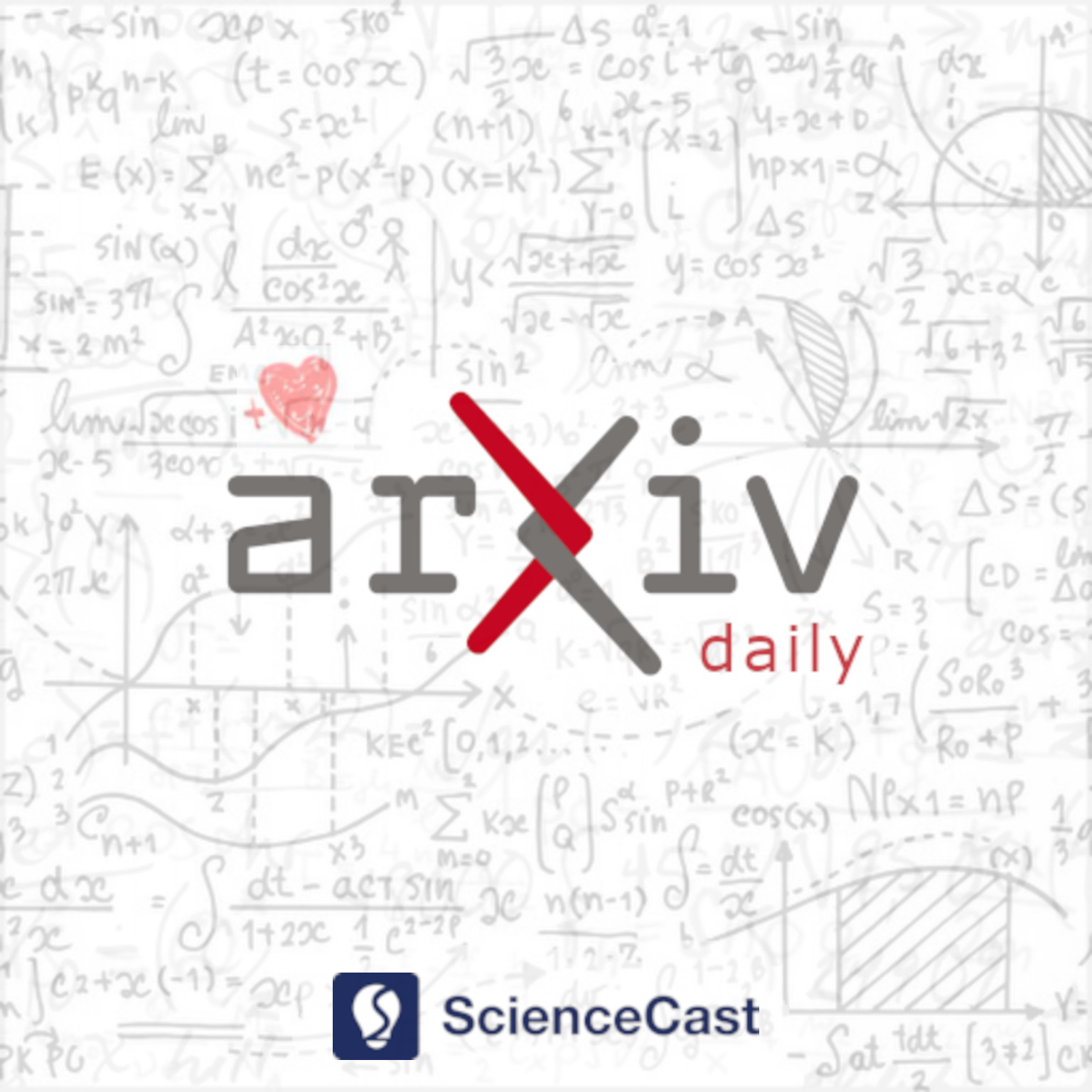
Optimization and Control (math.OC)
Tue, 16 May 2023
1.Push-LSVRG-UP: Distributed Stochastic Optimization over Unbalanced Directed Networks with Uncoordinated Triggered Probabilities
Authors:Jinhui Hu, Guo Chen, Huaqing Li, Zixiang Shen, Weidong Zhang
Abstract: Distributed stochastic optimization, arising in the crossing and integration of traditional stochastic optimization, distributed computing and storage, and network science, has advantages of high efficiency and a low per-iteration computational complexity in resolving large-scale optimization problems. This paper concentrates on resolving a large-scale convex finite-sum optimization problem in a multi-agent system over unbalanced directed networks. To tackle this problem in an efficient way, a distributed consensus optimization algorithm, adopting the push-sum technique and a distributed loopless stochastic variance-reduced gradient (LSVRG) method with uncoordinated triggered probabilities, is developed and named Push-LSVRG-UP. Each agent under this algorithmic framework performs only local computation and communicates only with its neighbors without leaking their private information. The convergence analysis of Push-LSVRG-UP is relied on analyzing the contraction relationships between four error terms associated with the multi-agent system. Theoretical results provide an explicit feasible range of the constant step-size, a linear convergence rate, and an iteration complexity of Push-LSVRG-UP when achieving the globally optimal solution. It is shown that Push-LSVRG-UP achieves the superior characteristics of accelerated linear convergence, fewer storage costs, and a lower per-iteration computational complexity than most existing works. Meanwhile, the introduction of an uncoordinated probabilistic triggered mechanism allows Push-LSVRG-UP to facilitate the independence and flexibility of agents in computing local batch gradients. In simulations, the practicability and improved performance of Push-LSVRG-UP are manifested via resolving two distributed learning problems based on real-world datasets.
2.Optimal Control of McKean-Vlasov equations with controlled stochasticity
Authors:Luca Di Persio, Peter Kuchling
Abstract: In this article, we analyse the existence of an optimal feedback controller of stochastic optimal control problems governed by SDEs which have the control in the diffusion part. To this end, we consider the underlying Fokker-Planck equation to transform the stochastic optimal control problem into a deterministic problem with open-loop controller.
3.Local well-posedness of the Mortensen observer
Authors:Tobias Breiten, Jesper Schröder
Abstract: The analytical background of nonlinear observers based on minimal energy estimation is discussed. It is shown that locally the derivation of the observer equation based on a trajectory with pointwise minimal energy can be done rigorously. The result is obtained by a local sensitivity analysis of the value function based on Pontryagin's maximum principle and the Hamilton-Jacobi-Bellman equation. The consideration of a differential Riccati equation reveals that locally the second derivative of the value function is a positive definite matrix. The local convexity ensures existence of a trajectory minimizing the energy, which is then shown to satisfy the observer equation.
4.Optimizing over trained GNNs via symmetry breaking
Authors:Shiqiang Zhang, Juan S Campos Salazar, Christian Feldmann, David Walz, Frederik Sandfort, Miriam Mathea, Calvin Tsay, Ruth Misener
Abstract: Optimization over trained machine learning models has applications including: verification, minimizing neural acquisition functions, and integrating a trained surrogate into a larger decision-making problem. This paper formulates and solves optimization problems constrained by trained graph neural networks (GNNs). To circumvent the symmetry issue caused by graph isomorphism, we propose two types of symmetry-breaking constraints: one indexing a node 0 and one indexing the remaining nodes by lexicographically ordering their neighbor sets. To guarantee that adding these constraints will not remove all symmetric solutions, we construct a graph indexing algorithm and prove that the resulting graph indexing satisfies the proposed symmetry-breaking constraints. For the classical GNN architectures considered in this paper, optimizing over a GNN with a fixed graph is equivalent to optimizing over a dense neural network. Thus, we study the case where the input graph is not fixed, implying that each edge is a decision variable, and develop two mixed-integer optimization formulations. To test our symmetry-breaking strategies and optimization formulations, we consider an application in molecular design.
5.A BSDE approach to the asymmetric risk-sensitive optimization and its applications
Authors:Mingshang Hu, Shaolin Ji, Rundong Xu, Xiaole Xue
Abstract: In this paper, we propose a formulation to describe a risk-sensitive criterion involving asymmetric risk attitudes toward different risk sources. The introduced criterion can only be defined through quadratic backward stochastic differential equations (BSDE). Before uncovering the mean-variance representation for the introduced asymmetric risk-sensitive criterion by variational approach, some axioms to characterize a variance decomposition of square integrable random variables are provided for the first time. The control problems under the asymmetric risk-sensitive criterion are characterized as a kind of stochastic recursive control problem that includes quadratic BSDEs. Under bounded and unbounded (linear quadratic case) conditions, the stochastic recursive control problems are investigated.