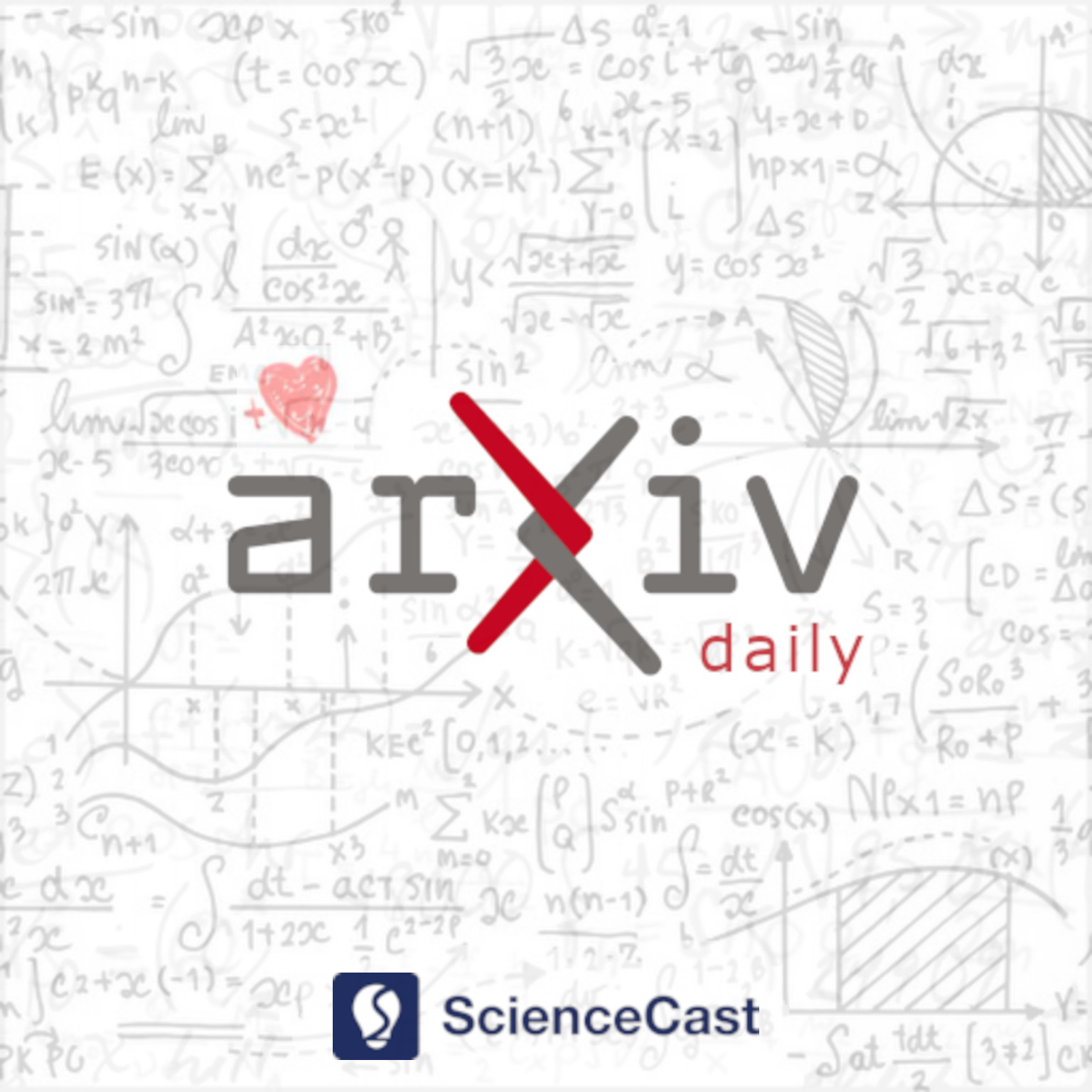
Optimization and Control (math.OC)
Thu, 10 Aug 2023
1.Existence theorems for optimal solutions in semi-algebraic optimization
Authors:Jae Hyoung Lee, Gue Myung Lee, Tien Son Pham
Abstract: Consider the problem of minimizing a lower semi-continuous semi-algebraic function $f \colon \mathbb{R}^n \to \mathbb{R} \cup \{+\infty\}$ on an unbounded closed semi-algebraic set $S \subset \mathbb{R}^n.$ Employing adequate tools of semi-algebraic geometry, we first establish some properties of the tangency variety of the restriction of $f$ on $S.$ Then we derive verifiable necessary and sufficient conditions for the existence of optimal solutions of the problem as well as the boundedness from below and coercivity of the restriction of $f$ on $S.$ We also present a computable formula for the optimal value of the problem.
2.Optimal Control of Dynamic District Heating Networks
Authors:Christian Jäkle, Lena Reichle, Stefan Volkwein
Abstract: In the present paper an optimal control problem for a system of differential-algebraic equations (DAEs) is considered. This problem arises in the dynamic optimization of unsteady district heating networks. Based on the Carath\'eodory theory existence of a unique solution to the DAE system is proved using specific properties of the district heating network model. Moreover, it is shown that the optimal control problem possesses optimal solutions. For the numerical experiments different networks are considered including also data from a real district heating network.
3.A Generalized Primal-Dual Correction Method for Saddle-Point Problems with the Nonlinear Coupling Operator
Authors:Sai Wang, Yi Gong
Abstract: Recently, the generalized primal-dual (GPD) method was developed for saddle-point problems (SPPs) with a linear coupling operator. However, the coupling operator in many engineering applications is nonlinear. In this letter, we propose a generalized primal-dual correction method (GPD-CM) to handle SPPs with a nonlinear coupling operator. To achieve this, we customize the proximal matrix and corrective matrix by adjusting the values of regularization factors. By the unified framework, the convergence of GPD-CM is directly obtained. Numerical results on a SPP with an exponential coupling operator support theoretical analysis.
4.Communication-efficient distributed optimization with adaptability to system heterogeneity
Authors:Ziyi Yu, Nikolaos M. Freris
Abstract: We consider the setting of agents cooperatively minimizing the sum of local objectives plus a regularizer on a graph. This paper proposes a primal-dual method in consideration of three distinctive attributes of real-life multi-agent systems, namely: (i)expensive communication, (ii)lack of synchronization, and (iii)system heterogeneity. In specific, we propose a distributed asynchronous algorithm with minimal communication cost, in which users commit variable amounts of local work on their respective sub-problems. We illustrate this both theoretically and experimentally in the machine learning setting, where the agents hold private data and use a stochastic Newton method as the local solver. Under standard assumptions on Lipschitz continuous gradients and strong convexity, our analysis establishes linear convergence in expectation and characterizes the dependency of the rate on the number of local iterations. We proceed a step further to propose a simple means for tuning agents' hyperparameters locally, so as to adjust to heterogeneity and accelerate the overall convergence. Last, we validate our proposed method on a benchmark machine learning dataset to illustrate the merits in terms of computation, communication, and run-time saving as well as adaptability to heterogeneity.
5.Unifying Distributionally Robust Optimization via Optimal Transport Theory
Authors:Jose Blanchet, Daniel Kuhn, Jiajin Li, Bahar Taskesen
Abstract: In the past few years, there has been considerable interest in two prominent approaches for Distributionally Robust Optimization (DRO): Divergence-based and Wasserstein-based methods. The divergence approach models misspecification in terms of likelihood ratios, while the latter models it through a measure of distance or cost in actual outcomes. Building upon these advances, this paper introduces a novel approach that unifies these methods into a single framework based on optimal transport (OT) with conditional moment constraints. Our proposed approach, for example, makes it possible for optimal adversarial distributions to simultaneously perturb likelihood and outcomes, while producing an optimal (in an optimal transport sense) coupling between the baseline model and the adversarial model.Additionally, the paper investigates several duality results and presents tractable reformulations that enhance the practical applicability of this unified framework.
6.Bounding the Difference between the Values of Robust and Non-Robust Markov Decision Problems
Authors:Ariel Neufeld, Julian Sester
Abstract: In this note we provide an upper bound for the difference between the value function of a distributionally robust Markov decision problem and the value function of a non-robust Markov decision problem, where the ambiguity set of probability kernels of the distributionally robust Markov decision process is described by a Wasserstein-ball around some reference kernel whereas the non-robust Markov decision process behaves according to a fixed probability kernel contained in the ambiguity set. Our derived upper bound for the difference between the value functions is dimension-free and depends linearly on the radius of the Wasserstein-ball.
7.Learning (With) Distributed Optimization
Authors:Aadharsh Aadhithya A, Abinesh S, Akshaya J, Jayanth M, Vishnu Radhakrishnan, Sowmya V, Soman K. P
Abstract: This paper provides an overview of the historical progression of distributed optimization techniques, tracing their development from early duality-based methods pioneered by Dantzig, Wolfe, and Benders in the 1960s to the emergence of the Augmented Lagrangian Alternating Direction Inexact Newton (ALADIN) algorithm. The initial focus on Lagrangian relaxation for convex problems and decomposition strategies led to the refinement of methods like the Alternating Direction Method of Multipliers (ADMM). The resurgence of interest in distributed optimization in the late 2000s, particularly in machine learning and imaging, demonstrated ADMM's practical efficacy and its unifying potential. This overview also highlights the emergence of the proximal center method and its applications in diverse domains. Furthermore, the paper underscores the distinctive features of ALADIN, which offers convergence guarantees for non-convex scenarios without introducing auxiliary variables, differentiating it from traditional augmentation techniques. In essence, this work encapsulates the historical trajectory of distributed optimization and underscores the promising prospects of ALADIN in addressing non-convex optimization challenges.
8.Disturbance attenuation in the Euler-Bernoulli beam using piezoelectric actuators
Authors:Anton Selivanov, Emilia Fridman
Abstract: We consider a simply-supported Euler-Bernoulli beam with viscous and Kelvin-Voigt damping. Our objective is to attenuate the effect of an unknown distributed disturbance using one piezoelectric actuator. We show how to design a suitable $H_\infty$ state-feedback controller based on a finite number of dominating modes. If the remaining (infinitely many) modes are ignored, the calculated $L^2$ gain is wrong. This happens because of the spillover phenomenon that occurs when the effect of the control on truncated modes is not accounted for in the feedback design. We propose a simple modification of the $H_\infty$ cost that prevents spillover. The key idea is to treat the control as a disturbance in the truncated modes and find the corresponding $L^2$ gains using the bounded real lemma. These $L^2$ gains are added to the control weight in the $H_\infty$ cost for the dominating modes, which prevents spillover. A numerical simulation of an aluminum beam with realistic parameters demonstrates the effectiveness of the proposed method.
9.Intercept Function and Quantity Bidding in Two-stage Electricity Market with Market Power Mitigation
Authors:Rajni Kant Bansal, Yue Chen, Pengcheng You, Enrique Mallada
Abstract: Electricity markets typically operate in two stages, day-ahead and real-time. Despite best efforts striving efficiency, evidence of price manipulation has called for system-level market power mitigation (MPM) initiatives that substitute noncompetitive bids with default bids. Implementing these policies with a limited understanding of participant behavior may lead to unintended economic losses. In this paper, we model the competition between generators and inelastic loads in a two-stage market with stage-wise MPM policies. The loss of Nash equilibrium and lack of guarantee of stable market outcome in the case of conventional supply function bidding motivates the use of an alternative market mechanism where generators bid an intercept function. A Nash equilibrium analysis for a day-ahead MPM policy leads to a Stackelberg-Nash game with loads exercising market power at the expense of generators. A comparison of the resulting equilibrium with the standard market (not implementing any MPM policy) shows that a day-ahead policy completely mitigates the market power of generators. On the other hand, the real-time MPM policy increases demand allocation to real-time, contrary to current market practice with most electricity trades in the day-ahead market. Numerical studies illustrate the impact of the slope of the intercept function on the standard market.