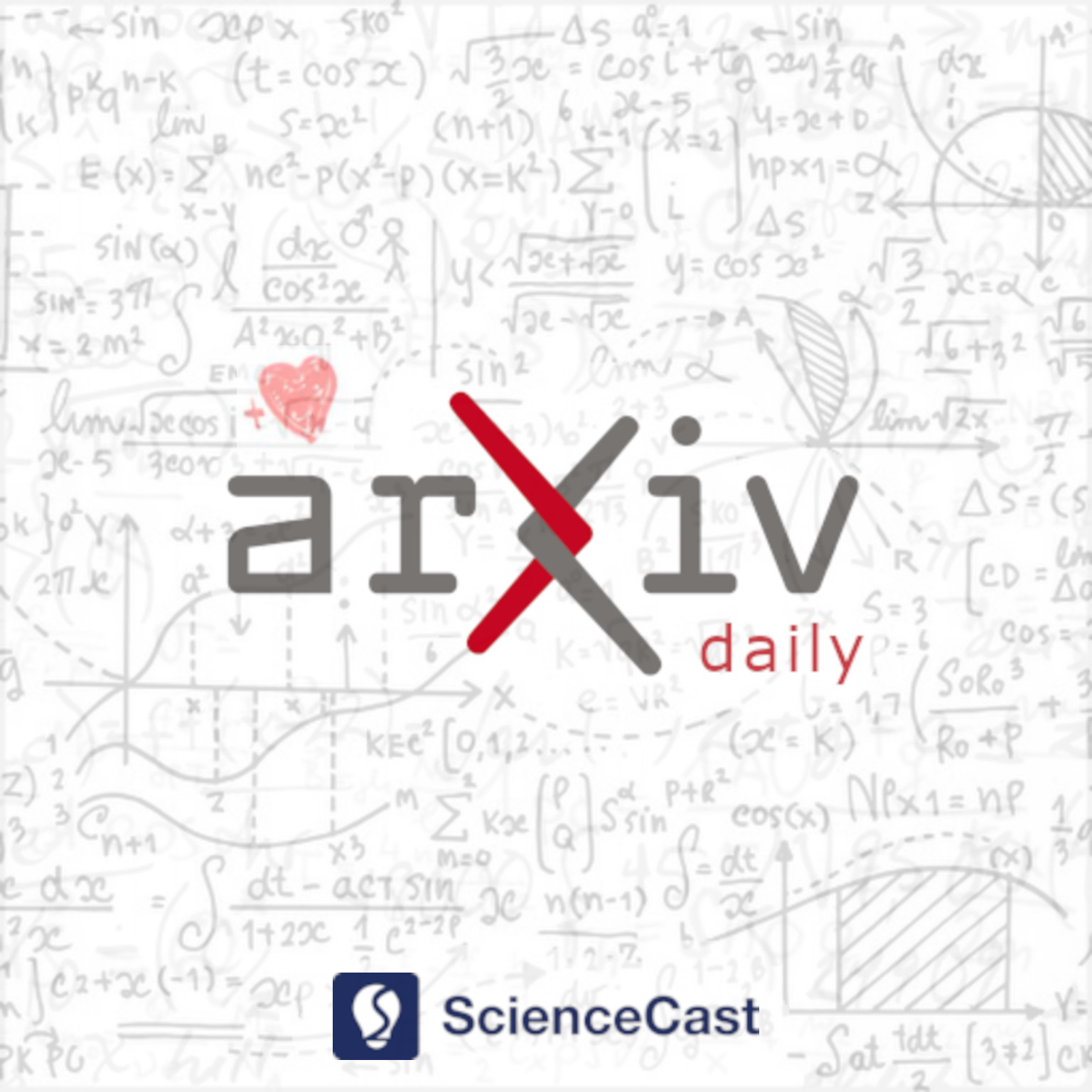
Optimization and Control (math.OC)
Wed, 13 Sep 2023
1.Maximum Principle for Mean Field Type Control Problems with General Volatility Functions
Authors:Alain Bensoussan, Ziyu Huang, Sheung Chi Phillip Yam
Abstract: In this paper, we study the maximum principle of mean field type control problems when the volatility function depends on the state and its measure and also the control, by using our recently developed method. Our method is to embed the mean field type control problem into a Hilbert space to bypass the evolution in the Wasserstein space. We here give a necessary condition and a sufficient condition for these control problems in Hilbert spaces, and we also derive a system of forward-backward stochastic differential equations.
2.Nonlinear network identifiability: The static case
Authors:Renato Vizuete, Julien M. Hendrickx
Abstract: We analyze the problem of network identifiability with nonlinear functions associated with the edges. We consider a static model for the output of each node and by assuming a perfect identification of the function associated with the measurement of a node, we provide conditions for the identifiability of the edges in a specific class of functions. First, we analyze the identifiability conditions in the class of all nonlinear functions and show that even for a path graph, it is necessary to measure all the nodes except by the source. Then, we consider analytic functions satisfying $f(0)=0$ and we provide conditions for the identifiability of paths and trees. Finally, by restricting the problem to a smaller class of functions where none of the functions is linear, we derive conditions for the identifiability of directed acyclic graphs. Some examples are presented to illustrate the results.
3.Barzilai-Borwein Descent Methods for Multiobjective Optimization Problems with Variable Trade-off Metrics
Authors:Jian Chen, Liping Tang, Xinmin Yang
Abstract: The imbalances and conditioning of the objective functions influence the performance of first-order methods for multiobjective optimization problems (MOPs). The latter is related to the metric selected in the direction-finding subproblems. Unlike single-objective optimization problems, capturing the curvature of all objective functions with a single Hessian matrix is impossible. On the other hand, second-order methods for MOPs use different metrics for objectives in direction-finding subproblems, leading to a high per-iteration cost. To balance per-iteration cost and better curvature exploration, we propose a Barzilai-Borwein descent method with variable metrics (BBDMO\_VM). In the direction-finding subproblems, we employ a variable metric to explore the curvature of all objectives. Subsequently, Barzilai-Borwein's method relative to the variable metric is applied to tune objectives, which mitigates the effect of imbalances. We investigate the convergence behaviour of the BBDMO\_VM, confirming fast linear convergence for well-conditioned problems relative to the variable metric. In particular, we establish linear convergence for problems that involve some linear objectives. These convergence results emphasize the importance of metric selection, motivating us to approximate the trade-off of Hessian matrices to better capture the geometry of the problem. Comparative numerical results confirm the efficiency of the proposed method, even when applied to large-scale and ill-conditioned problems.
4.On the Intelligent Proportional Controller Applied to Linear Systems
Authors:Mohamed Camil Belhadjoudja, Mohamed Maghenem, Emmanuel Witrant
Abstract: We analyze in this paper the effect of the well known intelligent proportional controller on the stability of linear control systems. Inspired by the literature on neutral time delay systems and advanced type systems, we derive sufficient conditions on the order of the control system, under which, the used controller fails to achieve exponential stability. Furthermore, we obtain conditions, relating the system s and the control parameters, such that the closed-loop system is either unstable or not exponentially stable. After that, we provide cases where the intelligent proportional controller achieves exponential stability. The obtained results are illustrated via numerical simulations, and on an experimental benchmark that consists of an electronic throttle valve.
5.Dynamical convergence analysis for nonconvex linearized proximal ADMM algorithms
Authors:Jiahong Guo, Xiao Wang, Xiantao Xiao
Abstract: The convergence analysis of optimization algorithms using continuous-time dynamical systems has received much attention in recent years. In this paper, we investigate applications of these systems to analyze the convergence of linearized proximal ADMM algorithms for nonconvex composite optimization, whose objective function is the sum of a continuously differentiable function and a composition of a possibly nonconvex function with a linear operator. We first derive a first-order differential inclusion for the linearized proximal ADMM algorithm, LP-ADMM. Both the global convergence and the convergence rates of the generated trajectory are established with the use of Kurdyka-\L{}ojasiewicz (KL) property. Then, a stochastic variant, LP-SADMM, is delved into an investigation for finite-sum nonconvex composite problems. Under mild conditions, we obtain the stochastic differential equation corresponding to LP-SADMM, and demonstrate the almost sure global convergence of the generated trajectory by leveraging the KL property. Based on the almost sure convergence of trajectory, we construct a stochastic process that converges almost surely to an approximate critical point of objective function, and derive the expected convergence rates associated with this stochastic process. Moreover, we propose an accelerated LP-SADMM that incorporates Nesterov's acceleration technique. The continuous-time dynamical system of this algorithm is modeled as a second-order stochastic differential equation. Within the context of KL property, we explore the related almost sure convergence and expected convergence rates.
6.Absorbing Markov Decision Processes
Authors:François Dufour, Tomás Prieto-Rumeau
Abstract: In this paper, we study discrete-time absorbing Markov Decision Processes (MDP) with measurable state space and Borel action space with a given initial distribution. For such models, solutions to the characteristic equation that are not occupation measures may exist. Several necessary and sufficient conditions are provided to guarantee that any solution to the characteristic equation is an occupation measure. Under the so-called continuity-compactness conditions, it is shown that the set of occupation measures is compact in the weak-strong topology if and only if the model is uniformly absorbing. Finally, it is shown that the occupation measures are characterized by the characteristic equation and an additional condition. Several examples are provided to illustrate our results.
7.Complexity analysis of regularization methods for implicitly constrained least squares
Authors:Akwum Onwunta, Clément W. Royer
Abstract: Optimization problems constrained by partial differential equations (PDEs) naturally arise in scientific computing, as those constraints often model physical systems or the simulation thereof. In an implicitly constrained approach, the constraints are incorporated into the objective through a reduced formulation. To this end, a numerical procedure is typically applied to solve the constraint system, and efficient numerical routines with quantifiable cost have long been developed. Meanwhile, the field of complexity in optimization, that estimates the cost of an optimization algorithm, has received significant attention in the literature, with most of the focus being on unconstrained or explicitly constrained problems. In this paper, we analyze an algorithmic framework based on quadratic regularization for implicitly constrained nonlinear least squares. By leveraging adjoint formulations, we can quantify the worst-case cost of our method to reach an approximate stationary point of the optimization problem. Our definition of such points exploits the least-squares structure of the objective, leading to an efficient implementation. Numerical experiments conducted on PDE-constrained optimization problems demonstrate the efficiency of the proposed framework.
8.Optimal adaptive control with separable drift uncertainty
Authors:Samuel N. Cohen, Christoph Knochenhauer, Alexander Merkel
Abstract: We consider a problem of stochastic optimal control with separable drift uncertainty in strong formulation on a finite horizon. The drift coefficient of the state $Y^{u}$ is multiplicatively influenced by an unknown random variable $\lambda$, while admissible controls $u$ are required to be adapted to the observation filtration. Choosing a control actively influences the state and information acquisition simultaneously and comes with a learning effect. The problem, initially non-Markovian, is embedded into a higher-dimensional Markovian, full information control problem with control-dependent filtration and noise. To that problem, we apply the stochastic Perron method to characterize the value function as the unique viscosity solution to the HJB equation, explicitly construct $\varepsilon$-optimal controls and show that the values of strong and weak formulations agree. Numerical illustrations show a significant difference between the adaptive control and the certainty equivalence control.