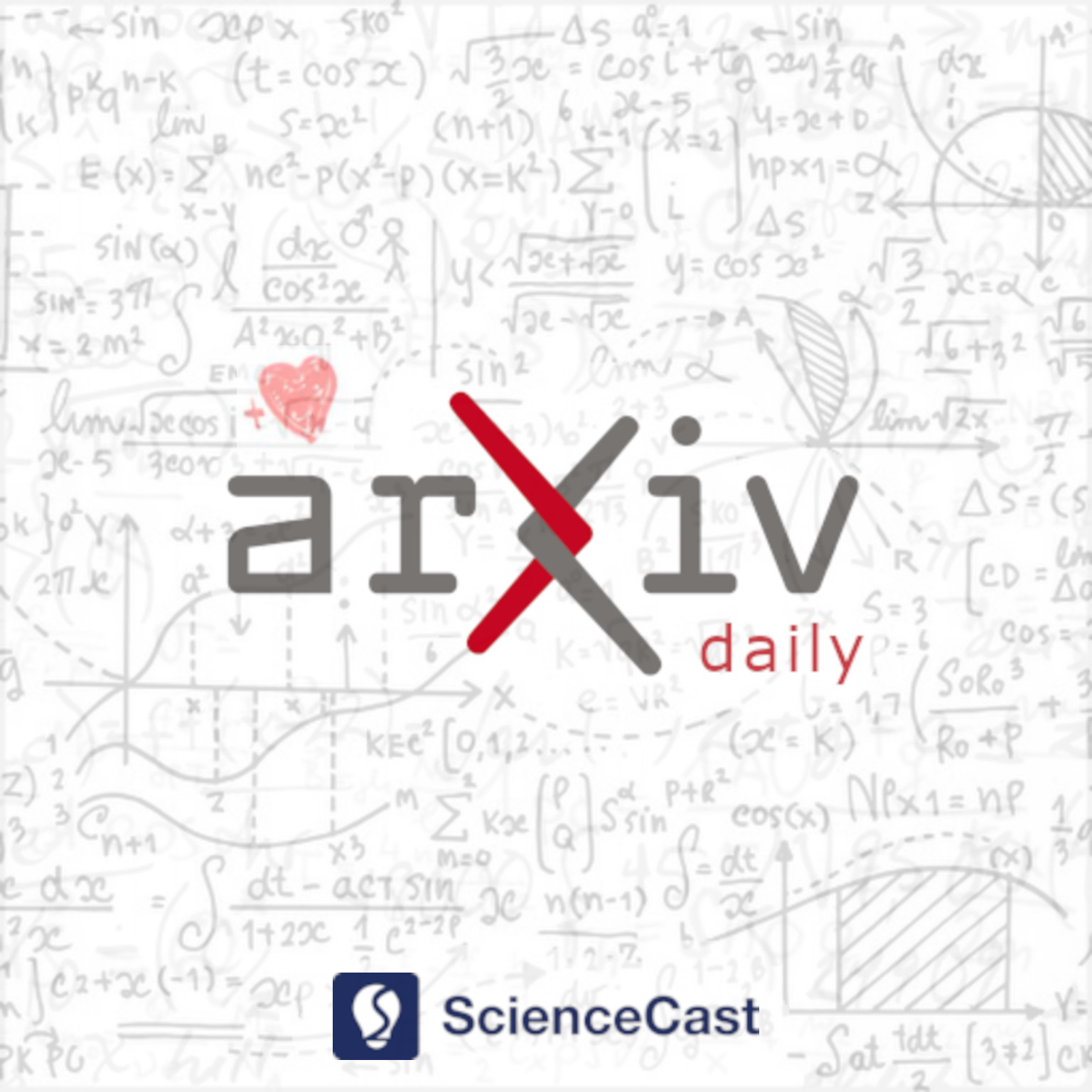
Optimization and Control (math.OC)
Tue, 18 Apr 2023
1.Constrained Assortment Optimization under the Cross-Nested Logit Model
Authors:Cuong Le, Tien Mai
Abstract: We study the assortment optimization problem under general linear constraints, where the customer choice behavior is captured by the Cross-Nested Logit model. In this problem, there is a set of products organized into multiple subsets (or nests), where each product can belong to more than one nest. The aim is to find an assortment to offer to customers so that the expected revenue is maximized. We show that, under the Cross-Nested Logit model, the assortment problem is NP-hard, even without any constraints. To tackle the assortment optimization problem, we develop a new discretization mechanism to approximate the problem by a linear fractional program with a performance guarantee of $\frac{1 - \epsilon}{1+\epsilon}$, for any accuracy level $\epsilon>0$. We then show that optimal solutions to the approximate problem can be obtained by solving mixed-integer linear programs. We further show that our discretization approach can also be applied to solve a joint assortment optimization and pricing problem, as well as an assortment problem under a mixture of Cross-Nested Logit models to account for multiple classes of customers. Our empirical results on a large number of randomly generated test instances demonstrate that, under a performance guarantee of 90%, the percentage gaps between the objective values obtained from our approximation methods and the optimal expected revenues are no larger than 1.2%.
2.Sensor Fault Detection and Isolation in Autonomous Nonlinear Systems Using Neural Network-Based Observers
Authors:John Cao, Muhammad Umar B. Niazi, Karl Henrik Johansson
Abstract: This paper presents a new observer-based approach to detect and isolate faulty sensors in industrial systems. Two types of sensor faults are considered: complete failure and sensor deterioration. The proposed method is applicable to general autonomous nonlinear systems without making any assumptions about its triangular and/or normal form, which is usually considered in the observer design literature. The key aspect of our approach is a learning-based design of the Luenberger observer, which involves using a neural network to approximate the injective map that transforms the nonlinear system into a stable linear system with output injection. This learning-based Luenberger observer accurately estimates the system's state, allowing for the detection of sensor faults through residual generation. The residual is computed as the norm of the difference between the system's measured output and the observer's predicted output vectors. Fault isolation is achieved by comparing each sensor's measurement with its corresponding predicted value. We demonstrate the effectiveness of our approach in capturing and isolating sensor faults while remaining robust in the presence of measurement noise and system uncertainty. We validate our method through numerical simulations of sensor faults in a network of Kuramoto oscillators.
3.Local Error Bounds for Affine Variational Inequalities on Hilbert Spaces
Authors:Tuan Ngoc Hoang, Lim Yongdo, Yend Dong Nguyen
Abstract: This paper gives some results related to the research problem about infinite-dimensional affine variational inequalities raised by N.D. Yen and X. Yang [Affine variational inequalities on normed spaces, J. Optim. Theory Appl., 178 (2018), 36--55]. Namely, we obtain local error bounds for affine variational inequalities on Hilbert spaces. To do so, we revisit two fundamental properties of polyhedral mappings. Then, we prove a locally upper Lipschitzian property of the inverse of the residual mapping of the infinite-dimensional affine variational inequality under consideration. Finally, we derive the desired local error bounds from that locally upper Lipschitzian property.
4.SOStab: a Matlab Toolbox for Approximating Regions of Attraction of Nonlinear Systems
Authors:Stéphane Drobot, Matteo Tacchi, Colin N. Jones
Abstract: This paper presents a novel Matlab toolbox, aimed at facilitating the use of polynomial optimization for stability analysis of nonlinear systems. Indeed, in the past decade several decisive contributions made it possible to recast the difficult problem of computing stability regions of nonlinear systems, under the form of convex optimization problems that are tractable in modest dimensions. However, these techniques combine sophisticated frameworks such as algebraic geometry, measure theory and mathematical programming, and existing software still requires their user to be fluent in Sum-of-Squares and Moment programming, preventing these techniques from being used more widely in the control community. To address this issue, SOStab entirely automates the writing and solving of optimization problems, and directly outputs relevant data for the user, while requiring minimal input. In particular, no specific knowledge of optimization is needed to use it.
5.On the Separation of Estimation and Control in Risk-Sensitive Investment Problems under Incomplete Observation
Authors:Sébastien Lleo, Wolfgang J. Runggaldier
Abstract: A typical approach to tackle stochastic control problems with partial observation is to separate the control and estimation tasks. However, it is well known that this separation generally fails to deliver an actual optimal solution for risk-sensitive control problems. This paper investigates the separability of a general class of risk-sensitive investment management problems when a finite-dimensional filter exists. We show that the corresponding separated problem, where instead of the unobserved quantities, one considers their conditional filter distribution given the observations, is strictly equivalent to the original control problem. We widen the applicability of the so-called Modified Zakai Equation (MZE) for the study of the separated problem and prove that the MZE simplifies to a PDE in our approach. Furthermore, we derive criteria for separability. We do not solve the separated control problem but note that the existence of a finite-dimensional filter leads to a finite state space for the separated problem. Hence, the difficulty is equivalent to solving a complete observation risk-sensitive problem. Our results have implications for existing risk-sensitive investment management models with partial observations in that they establish their separability. Their implications for future research on new applications is mainly to provide conditions to ensure separability.
6.Linear-quadratic-singular stochastic differential games and applications
Authors:Jodi Dianetti
Abstract: We consider a class of non-cooperative N-player non-zero-sum stochastic differential games with singular controls, in which each player can affect a linear stochastic differential equation in order to minimize a cost functional which is quadratic in the state and linear in the control. We call these games linear-quadratic-singular stochastic differential games. Under natural assumptions, we show the existence of open-loop Nash equilibria, which are characterized through a linear system of forward-backward stochastic differential equations. The proof is based on an approximation via a sequence of games in which players are restricted to play Lipschitz continuous strategies. We then discuss an application of these results to a model of capacity expansion in oligopoly markets.
7.A bilevel approach for compensation and routing decisions in last-mile delivery
Authors:Martina Cerulli, Claudia Archetti, Elena Fernandez, Ivana Ljubic
Abstract: In last-mile delivery logistics, peer-to-peer logistic platforms play an important role in connecting senders, customers, and independent carriers to fulfill delivery requests. Since the carriers are not under the platform's control, the platform has to anticipate their reactions, while deciding how to allocate the delivery operations. Indeed, carriers' decisions largely affect the platform's revenue. In this paper, we model this problem using bilevel programming. At the upper level, the platform decides how to assign the orders to the carriers; at the lower level, each carrier solves a profitable tour problem to determine which offered requests to accept, based on her own profit maximization. Possibly, the platform can influence carriers' decisions by determining also the compensation paid for each accepted request. The two considered settings result in two different formulations: the bilevel profitable tour problem with fixed compensation margins and with margin decisions, respectively. For each of them, we propose single-level reformulations and alternative formulations where the lower-level routing variables are projected out. A branch-and-cut algorithm is proposed to solve the bilevel models, with a tailored warm-start heuristic used to speed up the solution process. Extensive computational tests are performed to compare the proposed formulations and analyze solution characteristics.