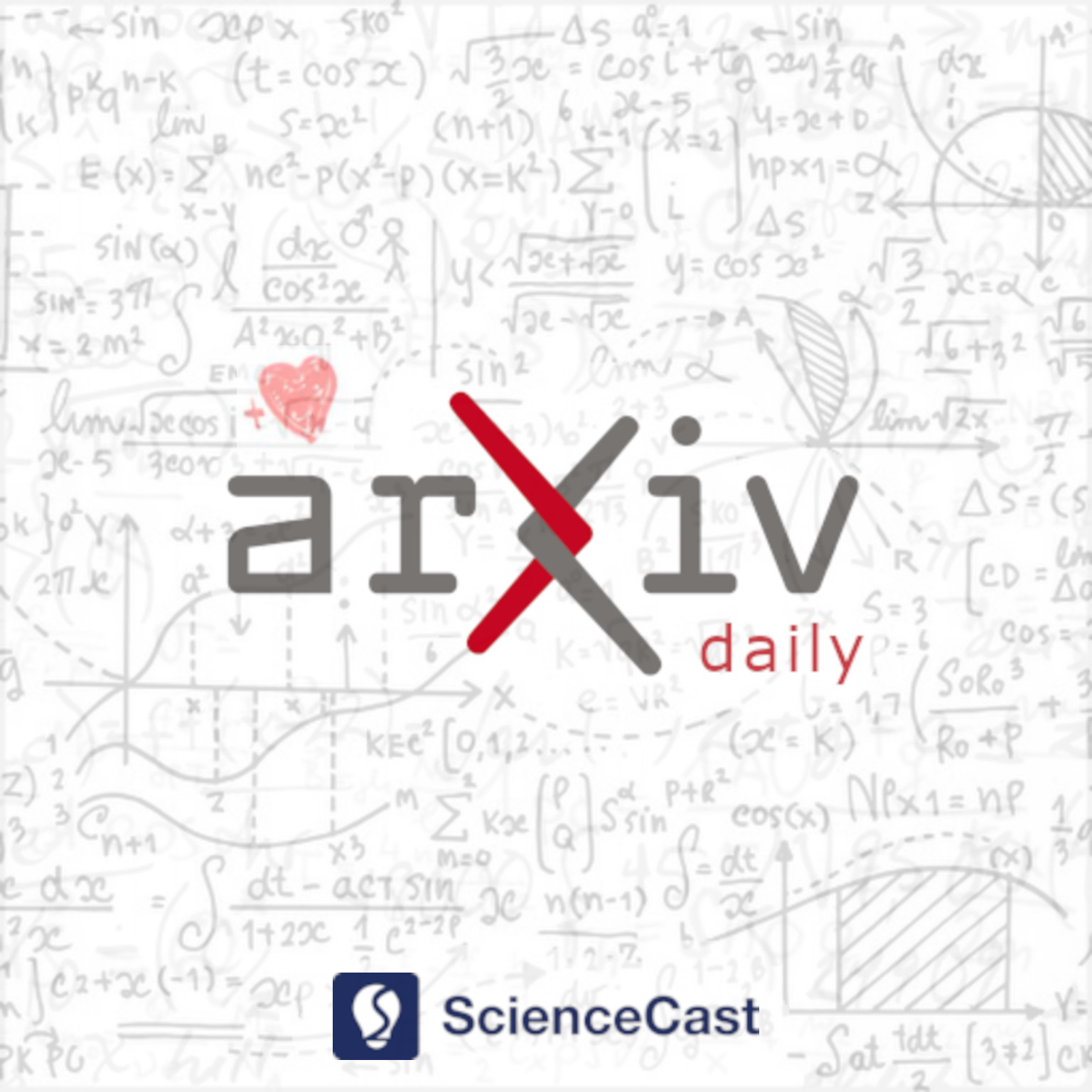
Optimization and Control (math.OC)
Tue, 25 Apr 2023
1.The logarithmic least squares priorities and ordinal violations in the best-worst method
Authors:László Csató
Abstract: The best-worst method is an increasingly popular approach to solving multi-criteria decision-making problems. Recently, the logarithmic least squares method has been proposed to derive priorities in the best-worst method since it has favourable properties such as the uniqueness of the weights, simple calculation, and the consideration of indirect comparisons. The current paper gives a sufficient condition for the logarithmic least squares method to guarantee the lack of ordinal violations in the best-worst method. It implies that the logarithmic least squares priorities are consistent with the preference order given by the decision-maker if the best alternative is at least two times more desirable than the others and the worst alternative is at least two times less desirable than the others, furthermore, the number of alternatives exceeds three. Our result provides another argument for using the logarithmic least squares priorities in the best-worst method.
2.A Moment-SOS Hierarchy for Robust Polynomial Matrix Inequality Optimization with SOS-Convexity
Authors:Feng Guo, Jie Wang
Abstract: We study a class of polynomial optimization problems with a robust polynomial matrix inequality constraint for which the uncertainty set is defined also by a polynomial matrix inequality (including robust polynomial semidefinite programs as a special case). Under certain SOS-convexity assumptions, we construct a hierarchy of moment-SOS relaxations for this problem to obtain convergent upper bounds of the optimal value by solving a sequence of semidefinite programs. To this end, we apply the Positivstellensatz for polynomial matrices and its dual matrix-valued moment theory to a conic reformulation of the problem. Most of the nice features of the moment-SOS hierarchy for the scalar polynomial optimization are generalized to the matrix case. In particular, the finite convergence of the hierarchy can be also certified if the flat extension condition holds. To extract global minimizers in this case, we develop a linear algebra approach to recover the representing matrix-valued measure for the corresponding truncated matrix-valued moment problem. As an application, we use this hierarchy to solve the problem of minimizing the smallest eigenvalue of a polynomial matrix subject to a polynomial matrix inequality. Finally, if SOS-convexity is replaced by convexity, we can still approximate the optimal value as closely as desired by solving a sequence of semidefinite programs, and certify global optimality in case that certain flat extension conditions hold true.
3.Minimal-time geodesics on a homogeneous spaces of the 2D Lie group
Authors:Victor Ayala, Adriano Da Silva, Maria Torreblanca
Abstract: Our main concern is to continue developing the theory of Linear Control Systems on homogeneous spaces of connected Lie groups. In this manuscript we solve a specific issue for this class of systems: time-optimal problem on a cylinder, a homogeneous space of the solvable Lie group of dimension two.
4.Faster than Fast: Accelerating the Griffin-Lim Algorithm
Authors:Rossen Nenov, Dang-Khoa Nguyen, Peter Balazs
Abstract: The phase retrieval problem is found in various areas of applications of engineering and applied physics. It is also a very active field of research in mathematics, signal processing and machine learning. In this paper, we present an accelerated version of the well known Fast Griffin-Lim algorithm (FGLA) for the phase retrieval problem in a general setting. It has increased the speed of convergence, and most importantly, the limit points of the generated sequence can reach a significantly smaller error than the ones generated by FGLA. We will give a motivation of the acceleration and compare it numerically to its predecessors and other algorithms typically used to solve similar problems.
5.Asymptotic Behaviors and Phase Transitions in Projected Stochastic Approximation: A Jump Diffusion Approach
Authors:Jiadong Liang, Yuze Han, Xiang Li, Zhihua Zhang
Abstract: In this paper we consider linearly constrained optimization problems and propose a loopless projection stochastic approximation (LPSA) algorithm. It performs the projection with probability $p_n$ at the $n$-th iteration to ensure feasibility. Considering a specific family of the probability $p_n$ and step size $\eta_n$, we analyze our algorithm from an asymptotic and continuous perspective. Using a novel jump diffusion approximation, we show that the trajectories connecting those properly rescaled last iterates weakly converge to the solution of specific stochastic differential equations (SDEs). By analyzing SDEs, we identify the asymptotic behaviors of LPSA for different choices of $(p_n, \eta_n)$. We find that the algorithm presents an intriguing asymptotic bias-variance trade-off and yields phase transition phenomenons, according to the relative magnitude of $p_n$ w.r.t. $\eta_n$. This finding provides insights on selecting appropriate ${(p_n, \eta_n)}_{n \geq 1}$ to minimize the projection cost. Additionally, we propose the Debiased LPSA (DLPSA) as a practical application of our jump diffusion approximation result. DLPSA is shown to effectively reduce projection complexity compared to vanilla LPSA.
6.Delayed impulsive stabilisation of discrete-time systems: a periodic event-triggering algorithm
Authors:Kexue Zhang, Elena Braverman
Abstract: This paper studies the problem of event-triggered impulsive control for discrete-time systems. A novel periodic event-triggering scheme with two tunable parameters is presented to determine the moments of updating impulsive control signals which are called event times. Sufficient conditions are established to guarantee asymptotic stability of the resulting impulsive systems. It is worth mentioning that the event times are different from the impulse times, that is, the control signals are updated at each event time but the actuator performs the impulsive control tasks at a later time due to time delays. The effectiveness of our theoretical result with the proposed scheme is illustrated by three examples.
7.Deep Reinforcement Learning in Finite-Horizon to Explore the Most Probable Transition Pathway
Authors:Jin Guo, Ting Gao, Peng Zhang, Jinqiao Duan
Abstract: This investigation focuses on discovering the most probable transition pathway for stochastic dynamical systems employing reinforcement learning. We first utilize Onsager-Machlup theory to quantify rare events in stochastic dynamical systems, and then convert the most likely transition path issue into a finite-horizon optimal control problem, because, in many instances, the transition path cannot be determined explicitly by variation. We propose the terminal prediction method and integrate it with reinforcement learning, develop our algorithm Finite Horizon Deep Deterministic Policy Gradient(FH-DDPG) to deal with the finite-horizon optimal control issue. Next, we present the convergence analysis of the algorithm for the value function estimation in terms of the neural network approximation error and the sampling error when estimating the network. Finally, experiments are conducted for the transition problem under Gaussian noise to verify the effectiveness of the algorithm.