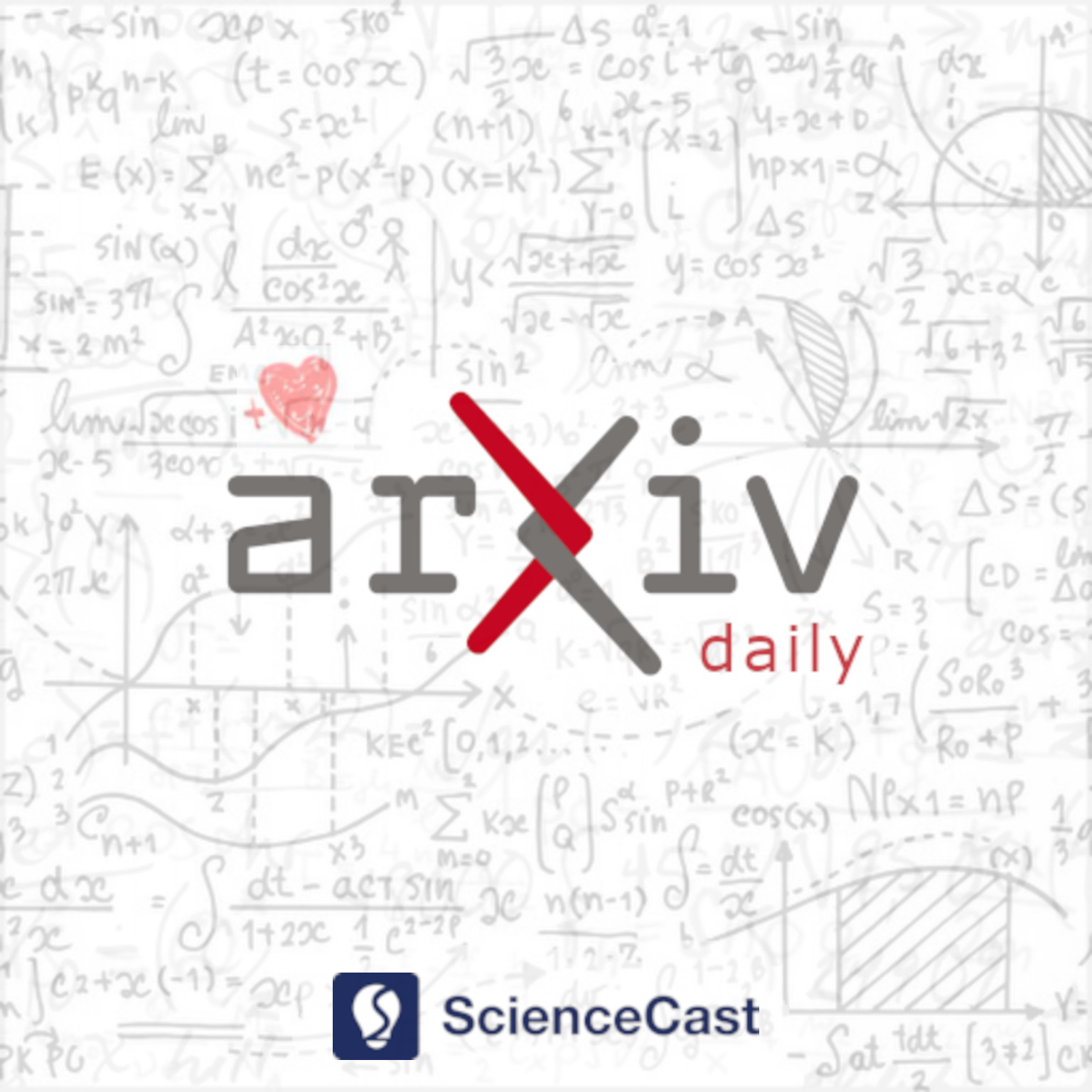
Optimization and Control (math.OC)
Mon, 17 Apr 2023
1.Accelerated Distributed Aggregative Optimization
Authors:Jiaxu Liu, Song Chen, Shengze Cai, Chao Xu
Abstract: In this paper, we investigate a distributed aggregative optimization problem in a network, where each agent has its own local cost function which depends not only on the local state variable but also on an aggregated function of state variables from all agents. To accelerate the optimization process, we combine heavy ball and Nesterov's accelerated methods with distributed aggregative gradient tracking, and propose two novel algorithms named DAGT-HB and DAGT-NES for solving the distributed aggregative optimization problem. We analyse that the DAGT-HB and DAGT-NES algorithms can converge to an optimal solution at a global $\mathbf{R}-$linear convergence rate when the objective function is smooth and strongly convex, and when the parameters (e.g., step size and momentum coefficients) are selected within certain ranges. A numerical experiment on the optimal placement problem is given to verify the effectiveness and superiority of our proposed algorithms.
2.On the benefit of overparameterisation in state reconstruction: An empirical study of the nonlinear case
Authors:Jonas F. Haderlein, Andre D. H. Peterson, Parvin Zarei Eskikand, Anthony N. Burkitt, Iven M. Y. Mareels, David B. Grayden
Abstract: The empirical success of machine learning models with many more parameters than measurements has generated an interest in the theory of overparameterisation, i.e., underdetermined models. This paradigm has recently been studied in domains such as deep learning, where one is interested in good (local) minima of complex, nonlinear loss functions. Optimisers, like gradient descent, perform well and consistently reach good solutions. Similarly, nonlinear optimisation problems are encountered in the field of system identification. Examples of such high-dimensional problems are optimisation tasks ensuing from the reconstruction of model states and parameters of an assumed known dynamical system from observed time series. In this work, we identify explicit parallels in the benefits of overparameterisation between what has been analysed in the deep learning context and system identification. We test multiple chaotic time series models, analysing the optimisation process for unknown model states and parameters in batch mode. We find that gradient descent reaches better solutions if we assume more parameters to be unknown. We hypothesise that, indeed, overparameterisation leads us towards better minima, and that more degrees of freedom in the optimisation are beneficial so long as the system is, in principle, observable.
3.A Criterion for ${\rm Q}$-tensors
Authors:Sonali Sharma, K. Palpandi
Abstract: A tensor ${\mathcal A}$ of order $m$ and dimension $n$ is called a ${\rm Q}$-tensor if the tensor complementarity problem has a solution for all ${\bf q} \in {\mathbb R}^{n}$. This means that for every vector ${\bf q}$, there exists a vector ${\bf u}$ such that ${\bf u} \geq {\bf 0},{\bf w} = {\mathcal A}{\bf u}^{m-1}+{\bf q} \geq {\bf 0},~\text{and}~ {\bf u}^{T}{\bf w} = 0$. In this paper, we prove that within the class of rank one symmetric tensors, the ${\rm Q}$-tensors are precisely the positive tensors. Additionally, for a symmetric ${\mathrm Q}$-tensor ${\mathcal A}$ with $rank({\mathcal A})=2$, we show that ${\mathcal A}$ is an ${\mathrm R}_{0}$-tensor. The idea is inspired by the recent work of Parthasarathy et al. \cite{Parthasarathy} and Sivakumar et al. \cite{Sivakumar} on ${\rm Q}$-matrices.
4.Decentralized projected Riemannian gradient method for smooth optimization on compact submanifolds
Authors:Kangkang Deng, Jiang Hu
Abstract: We consider the problem of decentralized nonconvex optimization over a compact submanifold, where each local agent's objective function defined by the local dataset is smooth. Leveraging the powerful tool of proximal smoothness, we establish local linear convergence of the projected gradient descent method with unit step size for solving the consensus problem over the compact manifold. This serves as the basis for analyzing decentralized algorithms on manifolds. Then, we propose two decentralized methods, namely the decentralized projected Riemannian gradient descent (DPRGD) and the decentralized projected Riemannian gradient tracking (DPRGT) methods. We establish their convergence rates of $\mathcal{O}(1/\sqrt{K})$ and $\mathcal{O}(1/K)$, respectively, to reach a stationary point. To the best of our knowledge, DPRGT is the first decentralized algorithm to achieve exact convergence for solving decentralized optimization over a compact manifold. The key ingredients in the proof are the Lipschitz-type inequalities of the projection operator on the compact manifold and smooth functions on the manifold, which could be of independent interest. Finally, we demonstrate the effectiveness of our proposed methods compared to state-of-the-art ones through numerical experiments on eigenvalue problems and low-rank matrix completion.
5.Unrolled three-operator splitting for parameter-map learning in Low Dose X-ray CT reconstruction
Authors:Andreas Kofler, Fabian Altekrüger, Fatima Antarou Ba, Christoph Kolbitsch, Evangelos Papoutsellis, David Schote, Clemens Sirotenko, Felix Frederik Zimmermann, Kostas Papafitsoros
Abstract: We propose a method for fast and automatic estimation of spatially dependent regularization maps for total variation-based (TV) tomography reconstruction. The estimation is based on two distinct sub-networks, with the first sub-network estimating the regularization parameter-map from the input data while the second one unrolling T iterations of the Primal-Dual Three-Operator Splitting (PD3O) algorithm. The latter approximately solves the corresponding TV-minimization problem incorporating the previously estimated regularization parameter-map. The overall network is then trained end-to-end in a supervised learning fashion using pairs of clean-corrupted data but crucially without the need of having access to labels for the optimal regularization parameter-maps.
6.Beyond first-order methods for non-convex non-concave min-max optimization
Authors:Abhijeet Vyas, Brian Bullins
Abstract: We propose a study of structured non-convex non-concave min-max problems which goes beyond standard first-order approaches. Inspired by the tight understanding established in recent works [Adil et al., 2022, Lin and Jordan, 2022b], we develop a suite of higher-order methods which show the improvements attainable beyond the monotone and Minty condition settings. Specifically, we provide a new understanding of the use of discrete-time $p^{th}$-order methods for operator norm minimization in the min-max setting, establishing an $O(1/\epsilon^\frac{2}{p})$ rate to achieve $\epsilon$-approximate stationarity, under the weakened Minty variational inequality condition of Diakonikolas et al. [2021]. We further present a continuous-time analysis alongside rates which match those for the discrete-time setting, and our empirical results highlight the practical benefits of our approach over first-order methods.