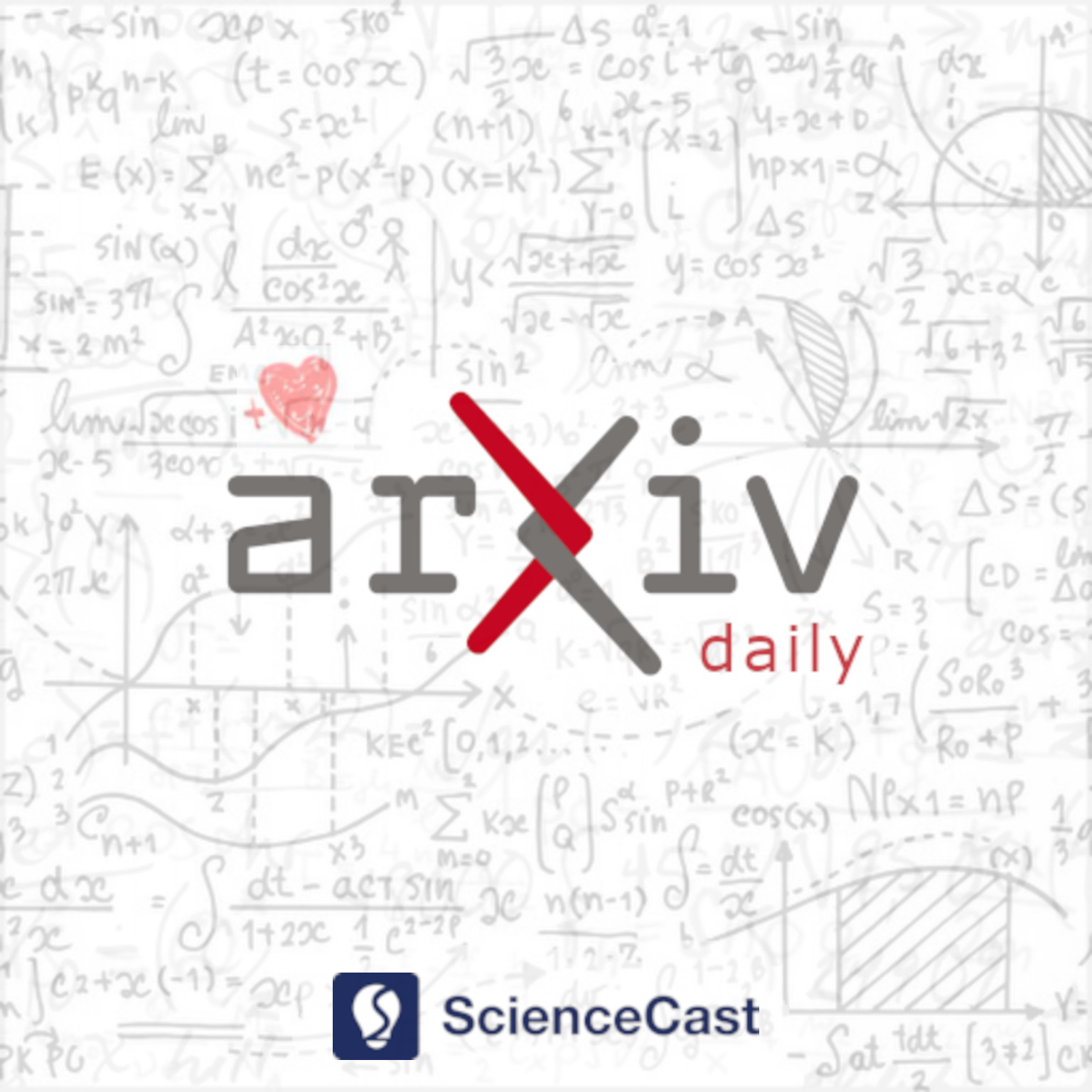
Optimization and Control (math.OC)
Tue, 11 Jul 2023
1.Control and estimation of multi-commodity network flow under aggregation
Authors:Yongxin Chen, Tryphon T. Georgiou, Michele Pavon
Abstract: A paradigm put forth by E. Schr\"odinger in 1931/32, known as Schr\"odinger bridges, represents a formalism to pose and solve control and estimation problems seeking a perturbation from an initial control schedule (in the case of control), or from a prior probability law (in the case of estimation), sufficient to reconcile data in the form of marginal distributions and minimal in the sense of relative entropy to the prior. In the same spirit, we consider traffic-flow and apply a Schr\"odinger-type dictum, to perturb minimally with respect to a suitable relative entropy functional a prior schedule/law so as to reconcile the traffic flow with scarce aggregate distributions on families of indistinguishable individuals. Specifically, we consider the problem to regulate/estimate multi-commodity network flow rates based only on empirical distributions of commodities being transported (e.g., types of vehicles through a network, in motion) at two given times. Thus, building on Schr\"odinger's large deviation rationale, we develop a method to identify {\em the most likely flow rates (traffic flow)}, given prior information and aggregate observations. Our method further extends the Schr\"odinger bridge formalism to the multi-commodity setting, allowing commodities to exit or enter the flow field as well (e.g., vehicles to enter and stop and park) at any time. The behavior of entering or exiting the flow field, by commodities or vehicles, is modeled by a Markov chains with killing and creation states. Our method is illustrated with a numerical experiment.
2.Linearization via Ordering Variables in Binary Optimization for Ising Machines
Authors:Kentaro Ohno, Nozomu Togawa
Abstract: Ising machines are next-generation computers expected for efficiently sampling near-optimal solutions of combinatorial oprimization problems. Combinatorial optimization problems are modeled as quadratic unconstrained binary optimization (QUBO) problems to apply an Ising machine. However, current state-of-the-art Ising machines still often fail to output near-optimal solutions due to the complicated energy landscape of QUBO problems. Furthermore, physical implementation of Ising machines severely restricts the size of QUBO problems to be input as a result of limited hardware graph structures. In this study, we take a new approach to these challenges by injecting auxiliary penalties preserving the optimum, which reduces quadratic terms in QUBO objective functions. The process simultaneously simplifies the energy landscape of QUBO problems, allowing search for near-optimal solutions, and makes QUBO problems sparser, facilitating encoding into Ising machines with restriction on the hardware graph structure. We propose linearization via ordering variables of QUBO problems as an outcome of the approach. By applying the proposed method to synthetic QUBO instances and to multi-dimensional knapsack problems, we empirically validate the effects on enhancing minor embedding of QUBO problems and performance of Ising machines.
3.A regularized Interior Point Method for sparse Optimal Transport on Graphs
Authors:Stefano Cipolla, Jacek Gondzio, Filippo Zanetti
Abstract: In this work, the authors address the Optimal Transport (OT) problem on graphs using a proximal stabilized Interior Point Method (IPM). In particular, strongly leveraging on the induced primal-dual regularization, the authors propose to solve large scale OT problems on sparse graphs using a bespoke IPM algorithm able to suitably exploit primal-dual regularization in order to enforce scalability. Indeed, the authors prove that the introduction of the regularization allows to use sparsified versions of the normal Newton equations to inexpensively generate IPM search directions. A detailed theoretical analysis is carried out showing the polynomial convergence of the inner algorithm in the proposed computational framework. Moreover, the presented numerical results showcase the efficiency and robustness of the proposed approach when compared to network simplex solvers.
4.A stochastic two-step inertial Bregman proximal alternating linearized minimization algorithm for nonconvex and nonsmooth problems
Authors:Chenzheng Guo, Jing Zhao, Qiao-Li Dong
Abstract: In this paper, for solving a broad class of large-scale nonconvex and nonsmooth optimization problems, we propose a stochastic two step inertial Bregman proximal alternating linearized minimization (STiBPALM) algorithm with variance-reduced stochastic gradient estimators. And we show that SAGA and SARAH are variance-reduced gradient estimators. Under expectation conditions with the Kurdyka-Lojasiewicz property and some suitable conditions on the parameters, we obtain that the sequence generated by the proposed algorithm converges to a critical point. And the general convergence rate is also provided. Numerical experiments on sparse nonnegative matrix factorization and blind image-deblurring are presented to demonstrate the performance of the proposed algorithm.
5.Stochastic Nested Compositional Bi-level Optimization for Robust Feature Learning
Authors:Xuxing Chen, Krishnakumar Balasubramanian, Saeed Ghadimi
Abstract: We develop and analyze stochastic approximation algorithms for solving nested compositional bi-level optimization problems. These problems involve a nested composition of $T$ potentially non-convex smooth functions in the upper-level, and a smooth and strongly convex function in the lower-level. Our proposed algorithm does not rely on matrix inversions or mini-batches and can achieve an $\epsilon$-stationary solution with an oracle complexity of approximately $\tilde{O}_T(1/\epsilon^{2})$, assuming the availability of stochastic first-order oracles for the individual functions in the composition and the lower-level, which are unbiased and have bounded moments. Here, $\tilde{O}_T$ hides polylog factors and constants that depend on $T$. The key challenge we address in establishing this result relates to handling three distinct sources of bias in the stochastic gradients. The first source arises from the compositional nature of the upper-level, the second stems from the bi-level structure, and the third emerges due to the utilization of Neumann series approximations to avoid matrix inversion. To demonstrate the effectiveness of our approach, we apply it to the problem of robust feature learning for deep neural networks under covariate shift, showcasing the benefits and advantages of our methodology in that context.
6.Reliable optimal controls for SEIR models in epidemiology
Authors:Simone Cacace, Alessio Oliviero
Abstract: We present and compare two different optimal control approaches applied to SEIR models in epidemiology, which allow us to obtain some policies for controlling the spread of an epidemic. The first approach uses Dynamic Programming to characterise the value function of the problem as the solution of a partial differential equation, the Hamilton-Jacobi-Bellman equation, and derive the optimal policy in feedback form. The second is based on Pontryagin's maximum principle and directly gives open-loop controls, via the solution of an optimality system of ordinary differential equations. This method, however, may not converge to the optimal solution. We propose a combination of the two methods in order to obtain high-quality and reliable solutions. Several simulations are presented and discussed.
7.Stability and genericity of bang-bang controls in affine problems
Authors:Alberto Domínguez Corella, Gerd Wachsmuth
Abstract: We analyse the role of the bang-bang property in affine optimal control problems. We show that many essential stability properties of affine problems are only satisfied when minimizers have the bang-bang property. Moreover, we prove that almost any perturbation in an affine optimal control problem leads to a bang-bang strict global minimizer. We work in an abstract framework that allows to cover many problems in the literature of optimal control, this includes problems constrained by partial and ordinary differential equations. We give examples that show the applicability of our results to specific optimal control problems.