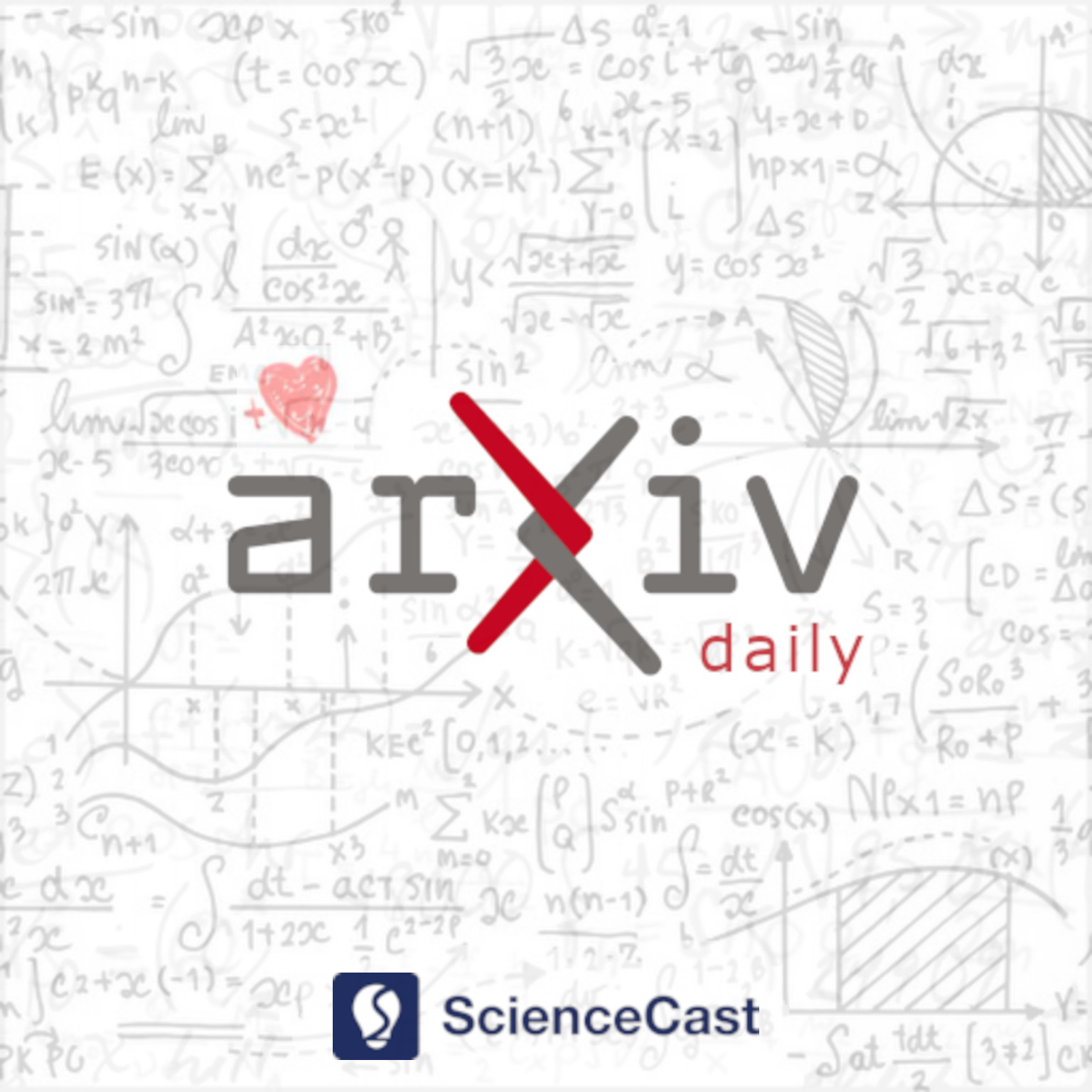
Optimization and Control (math.OC)
Wed, 12 Jul 2023
1.Outlier detection in regression: conic quadratic formulations
Authors:Andrés Gómez, José Neto
Abstract: In many applications, when building linear regression models, it is important to account for the presence of outliers, i.e., corrupted input data points. Such problems can be formulated as mixed-integer optimization problems involving cubic terms, each given by the product of a binary variable and a quadratic term of the continuous variables. Existing approaches in the literature, typically relying on the linearization of the cubic terms using big-M constraints, suffer from weak relaxation and poor performance in practice. In this work we derive stronger second-order conic relaxations that do not involve big-M constraints. Our computational experiments indicate that the proposed formulations are several orders-of-magnitude faster than existing big-M formulations in the literature for this problem.
2.Online Inventory Problems: Beyond the i.i.d. Setting with Online Convex Optimization
Authors:Massil Hihat, Stéphane Gaïffas, Guillaume Garrigos, Simon Bussy
Abstract: We study multi-product inventory control problems where a manager makes sequential replenishment decisions based on partial historical information in order to minimize its cumulative losses. Our motivation is to consider general demands, losses and dynamics to go beyond standard models which usually rely on newsvendor-type losses, fixed dynamics, and unrealistic i.i.d. demand assumptions. We propose MaxCOSD, an online algorithm that has provable guarantees even for problems with non-i.i.d. demands and stateful dynamics, including for instance perishability. We consider what we call non-degeneracy assumptions on the demand process, and argue that they are necessary to allow learning.
3.On the sharp Makai inequality
Authors:Francesca Prinari, Anna Chiara Zagati
Abstract: On a convex bounded open set, we prove that Poincar\'e-Sobolev constants for functions vanishing at the boundary can be bounded from below in terms of the norm of the distance function in a suitable Lebesgue space. This generalizes a result shown, in the planar case, by E. Makai, for the torsional rigidity. In addition, we compare the sharp Makai constants obtained in the class of convex sets with the optimal constants defined in other classes of open sets. Finally, an alternative proof of the Hersch-Protter inequality for convex sets is given.
4.Integrated supervisory control and fixed path speed trajectory generation for hybrid electric ships via convex optimization
Authors:Antti Ritari, Niklas Katzenburg, Fabricio Oliveira, Kari Tammi
Abstract: Battery-hybrid power source architectures can reduce fuel consumption and emissions for ships with diverse operation profiles. However, conventional control strategies may fail to improve performance if the future operation profile is unknown to the controller. This paper proposes a guidance, navigation, and control (GNC) function that integrates trajectory generation and hybrid power source supervisory control. We focus on time and fuel optimal path-constrained trajectory planning. This problem is a nonlinear and nonconvex optimal control problem, which means that it is not readily amenable to efficient and reliable solution onboard. We propose a nonlinear change of variables and constraint relaxations that transform the nonconvex planning problem into a convex optimal control problem. The nonconvex three-degree-of-freedom dynamics, hydrodynamic forces, fixed pitch propeller, battery, and general energy converter (e.g., fuel cell or generating set) dissipation constraints are expressed in convex functional form. A condition derived from Pontryagin's Minimum Principle guarantees that, when satisfied, the solution of the relaxed problem provides the solution to the original problem. The validity and effectiveness of this approach are numerically illustrated for a battery-hybrid vessel in model scale. First, the convex hydrodynamic hull and rudder force models are validated with towing tank test data. Second, optimal trajectories and supervisory control schemes are evaluated under varying mission requirements. The convexification scheme in this work lays the path for the employment of mature, computationally robust convex optimization methods and creates a novel possibility for real-time optimization onboard future smart and unmanned surface vehicles.
5.A preliminary model for optimal control of moisture content in unsaturated soils
Authors:Marco Berardi, Fabio V. Difonzo, Roberto Guglielmi
Abstract: In this paper we introduce an optimal control approach to Richards' equation in an irrigation framework, aimed at minimizing water consumption while maximizing root water uptake. We first describe the physics of the nonlinear model under consideration, and then develop the first-order necessary optimality conditions of the associated boundary control problem. We show that our model provides a promising framework to support optimized irrigation strategies, thus facing water scarcity in irrigation. The characterization of the optimal control in terms of a suitable relation with the adjoint state of the optimality conditions is then used to develop numerical simulations on different hydrological settings, that supports the analytical findings of the paper.
6.Further techniques on a polynomial positivity question of Collins, Dykema, and Torres-Ayala
Authors:Nathaniel K. Green, Edward D. Kim
Abstract: We prove that the coefficient of $t^2$ in $\mathsf{trace}((A+tB)^6)$ is a sum of squares in the entries of the symmetric matrices $A$ and $B$.
7.Provably Faster Gradient Descent via Long Steps
Authors:Benjamin Grimmer
Abstract: This work establishes provably faster convergence rates for gradient descent via a computer-assisted analysis technique. Our theory allows nonconstant stepsize policies with frequent long steps potentially violating descent by analyzing the overall effect of many iterations at once rather than the typical one-iteration inductions used in most first-order method analyses. We show that long steps, which may increase the objective value in the short term, lead to provably faster convergence in the long term. A conjecture towards proving a faster $O(1/T\log T)$ rate for gradient descent is also motivated along with simple numerical validation.