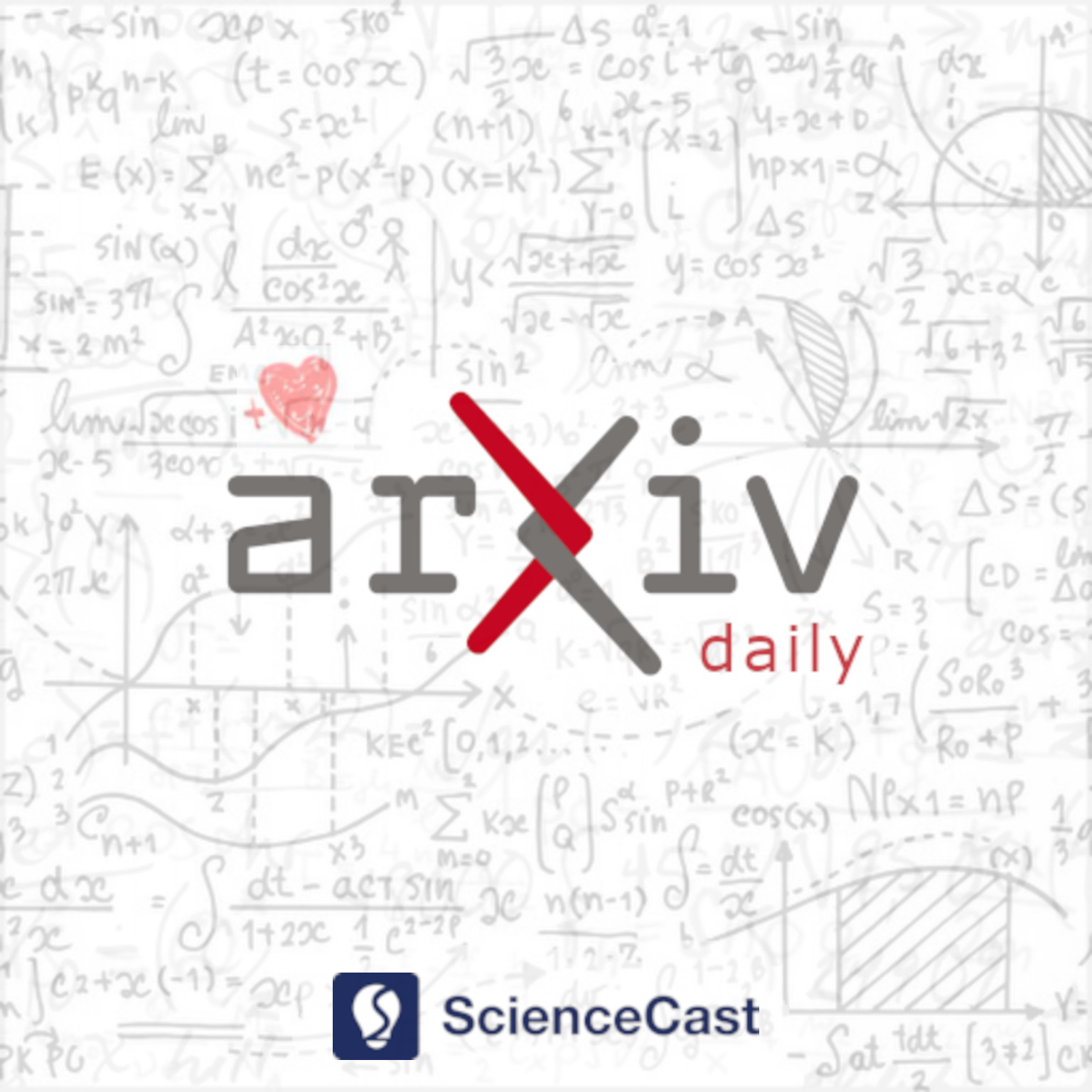
Optimization and Control (math.OC)
Mon, 08 May 2023
1.Nash equilibria for total expected reward absorbing Markov games: the constrained and unconstrained cases
Authors:François Dufour, Tomás Prieto-Rumeau
Abstract: We consider a nonzero-sum N-player Markov game on an abstract measurable state space with compact metric action spaces. The payoff functions are bounded Carath\'eodory functions and the transitions of the system are assumed to have a density function satisfying some continuity conditions. The optimality criterion of the players is given by a total expected payoff on an infinite discrete-time horizon. Under the condition that the game model is absorbing, we establish the existence of Markov strategies that are a noncooperative equilibrium in the family of all history-dependent strategies of the players for both the constrained and the unconstrained problems, We obtain, as a particular case of results, the existence of Nash equilibria for discounted constrained and unconstrained game models.
2.Optimal Battery Charge Scheduling For Revenue Stacking Under Operational Constraints Via Energy Arbitrage
Authors:Alban Puech IP Paris & DEIF, Gorazd Dimitrov IP Paris, Claudia D'Ambrosio LIX, CNRS
Abstract: As the share of variable renewable energy sources increases in the electricity mix, new solutions are needed to build a flexible and reliable grid. Energy arbitrage with battery storage systems supports renewable energy integration into the grid by shifting demand and increasing the overall utilization of power production systems. In this paper, we propose a mixed integer linear programming model for energy arbitrage on the day-ahead market, that takes into account operational and availability constraints of asset owners willing to get an additional revenue stream from their storage asset. This approach optimally schedules the charge and discharge operations associated with the most profitable trading strategy, and achieves between 80% and 90% of the maximum obtainable profits considering one-year time horizons using the prices of electricity in multiple European countries including Germany, France, Italy, Denmark, and Spain.
3.Accelerated Stochastic Optimization Methods under Quasar-convexity
Authors:Qiang Fu, Dongchu Xu, Ashia Wilson
Abstract: Non-convex optimization plays a key role in a growing number of machine learning applications. This motivates the identification of specialized structure that enables sharper theoretical analysis. One such identified structure is quasar-convexity, a non-convex generalization of convexity that subsumes convex functions. Existing algorithms for minimizing quasar-convex functions in the stochastic setting have either high complexity or slow convergence, which prompts us to derive a new class of stochastic methods for optimizing smooth quasar-convex functions. We demonstrate that our algorithms have fast convergence and outperform existing algorithms on several examples, including the classical problem of learning linear dynamical systems. We also present a unified analysis of our newly proposed algorithms and a previously studied deterministic algorithm.
4.Gradient descent with a general cost
Authors:Flavien Léger, Pierre-Cyril Aubin-Frankowski
Abstract: We present a new class of gradient-type optimization methods that extends vanilla gradient descent, mirror descent, Riemannian gradient descent, and natural gradient descent. Our approach involves constructing a surrogate for the objective function in a systematic manner, based on a chosen cost function. This surrogate is then minimized using an alternating minimization scheme. Using optimal transport theory we establish convergence rates based on generalized notions of smoothness and convexity. We provide local versions of these two notions when the cost satisfies a condition known as nonnegative cross-curvature. In particular our framework provides the first global rates for natural gradient descent and Newton's method.