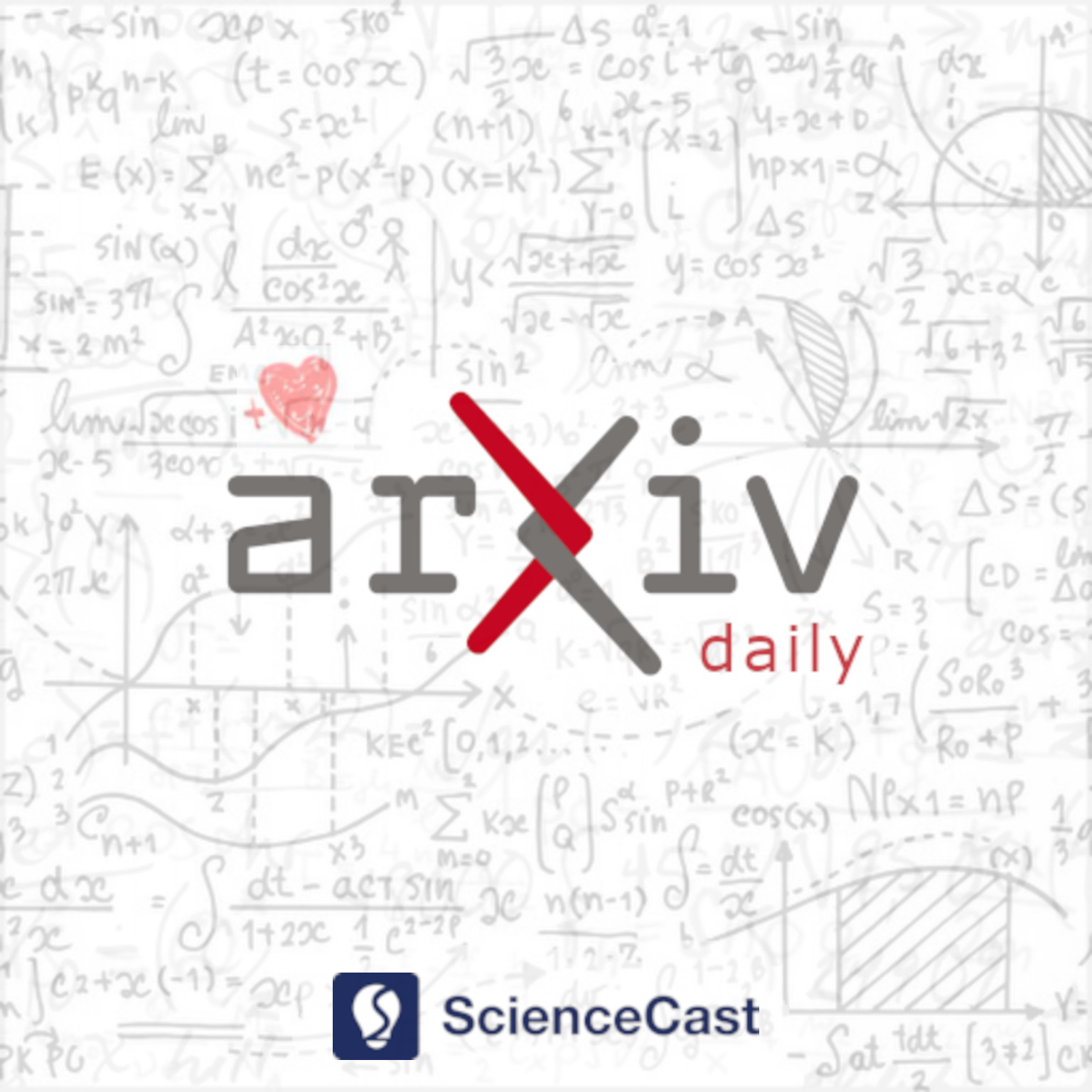
Optimization and Control (math.OC)
Thu, 17 Aug 2023
1.Convex Optimization-Based Model Predictive Control for the Guidance of Active Debris Removal Transfers
Authors:Minduli Wijayatunga, Roberto Armellin, Harry Holt, Laura Pirovano, Claudio Bombardelli
Abstract: Active debris removal (ADR) missions have garnered significant interest as means of mitigating collision risks in space. This work proposes a convex optimization-based model predictive control (MPC) approach to provide guidance for such missions. While convex optimization can obtain optimal solutions in polynomial time, it relies on the successive convexification of nonconvex dynamics, leading to inaccuracies. Here, the need for successive convexification is eliminated by using near-linear Generalized Equinoctial Orbital Elements (GEqOE) and by updating the reference trajectory through a new split-Edelbaum approach. The solution accuracy is then measured relative to a high-fidelity dynamics model, showing that the MPC-convex method can generate accurate solutions without iterations.
2.Learning the hub graphical Lasso model with the structured sparsity via an efficient algorithm
Authors:Chengjing Wang, Peipei Tang, Wenling He, Meixia Lin
Abstract: Graphical models have exhibited their performance in numerous tasks ranging from biological analysis to recommender systems. However, graphical models with hub nodes are computationally difficult to fit, particularly when the dimension of the data is large. To efficiently estimate the hub graphical models, we introduce a two-phase algorithm. The proposed algorithm first generates a good initial point via a dual alternating direction method of multipliers (ADMM), and then warm starts a semismooth Newton (SSN) based augmented Lagrangian method (ALM) to compute a solution that is accurate enough for practical tasks. The sparsity structure of the generalized Jacobian ensures that the algorithm can obtain a nice solution very efficiently. Comprehensive experiments on both synthetic data and real data show that it obviously outperforms the existing state-of-the-art algorithms. In particular, in some high dimensional tasks, it can save more than 70\% of the execution time, meanwhile still achieves a high-quality estimation.
3.Stabilizability for nonautonomous linear parabolic equations with actuators as distributions
Authors:Karl Kunisch, Sérgio S. Rodrigues, Daniel Walter
Abstract: The stabilizability of a general class of abstract parabolic-like equations is investigated, with a finite number of actuators. This class includes the case of actuators given as delta distributions located at given points in the spatial domain of concrete parabolic equations. A stabilizing feedback control operator is constructed and given in explicit form. Then, an associated optimal control is considered and the corresponding Riccati feedback is investigated. Results of simulations are presented showing the stabilizing performance of both explicit and Riccati feedbacks.
4.Hitting the High-Dimensional Notes: An ODE for SGD learning dynamics on GLMs and multi-index models
Authors:Elizabeth Collins-Woodfin, Courtney Paquette, Elliot Paquette, Inbar Seroussi
Abstract: We analyze the dynamics of streaming stochastic gradient descent (SGD) in the high-dimensional limit when applied to generalized linear models and multi-index models (e.g. logistic regression, phase retrieval) with general data-covariance. In particular, we demonstrate a deterministic equivalent of SGD in the form of a system of ordinary differential equations that describes a wide class of statistics, such as the risk and other measures of sub-optimality. This equivalence holds with overwhelming probability when the model parameter count grows proportionally to the number of data. This framework allows us to obtain learning rate thresholds for stability of SGD as well as convergence guarantees. In addition to the deterministic equivalent, we introduce an SDE with a simplified diffusion coefficient (homogenized SGD) which allows us to analyze the dynamics of general statistics of SGD iterates. Finally, we illustrate this theory on some standard examples and show numerical simulations which give an excellent match to the theory.
5.Progressively Strengthening and Tuning MIP Solvers for Reoptimization
Authors:Krunal Kishor Patel
Abstract: This paper explores reoptimization techniques for solving sequences of similar mixed integer programs (MIPs) more effectively. Traditionally, these MIPs are solved independently, without capitalizing on information from previously solved instances. Our approach focuses on primal bound improvements by reusing the solutions of the previously solved instances as well as dual bound improvements by reusing the branching history and automating parameter-tuning. We also describe ways to improve the solver performance by extending ideas from reliability branching to generate better pseudocosts. Our reoptimization approach, which we developed for the computational competition of the MIP 2023 workshop, earned us the first prize. In this paper, we thoroughly analyze the performance of each technique and their combined impact on the solver's performance. Finally, we present ways to extend our techniques in practice for further improvements.
6.Derivative-Free Global Minimization in One Dimension: Relaxation, Monte Carlo, and Sampling
Authors:Alexandra A. Gomes, Diogo A. Gomes
Abstract: We introduce a derivative-free global optimization algorithm that efficiently computes minima for various classes of one-dimensional functions, including non-convex, and non-smooth functions.This algorithm numerically approximates the gradient flow of a relaxed functional, integrating strategies such as Monte Carlos methods, rejection sampling, and adaptive techniques. These strategies enhance performance in solving a diverse range of optimization problems while significantly reducing the number of required function evaluations compared to established methods. We present a proof of the convergence of the algorithm and illustrate its performance by comprehensive benchmarking. The proposed algorithm offers a substantial potential for real-world models. It is particularly advantageous in situations requiring computationally intensive objective function evaluations.
7.A non-convex relaxed version of minimax theorems
Authors:M. I. A. Ghitri, A. Hantoute
Abstract: Given a subset $A\times B$ of a locally convex space $X\times Y$ (with $A$ compact) and a function $f:A\times B\rightarrow\overline{\mathbb{R}}$ such that $f(\cdot,y),$ $y\in B,$ are concave and upper semicontinuous, the minimax inequality $\max_{x\in A} \inf_{y\in B} f(x,y) \geq \inf_{y\in B} \sup_{x\in A_{0}} f(x,y)$ is shown to hold provided that $A_{0}$ be the set of $x\in A$ such that $f(x,\cdot)$ is proper, convex and lower semi-contiuous. Moreover, if in addition $A\times B\subset f^{-1}(\mathbb{R})$, then we can take as $A_{0}$ the set of $x\in A$ such that $f(x,\cdot)$ is convex. The relation to Moreau's biconjugate representation theorem is discussed, and some applications to\ convex duality are provided. Key words. Minimax theorem, Moreau theorem, conjugate function, convex optimization.
8.A DPG method for linear quadratic optimal control problems
Authors:Thomas Führer, Francisco Fuica
Abstract: The DPG method with optimal test functions for solving linear quadratic optimal control problems with control constraints is studied. We prove existence of a unique optimal solution of the nonlinear discrete problem and characterize it through first order optimality conditions. Furthermore, we systematically develop a priori as well as a posteriori error estimates. Our proposed method can be applied to a wide range of constrained optimal control problems subject to, e.g., scalar second-order PDEs and the Stokes equations. Numerical experiments that illustrate our theoretical findings are presented.
9.Linear Parameter Varying Power Regulation of Variable Speed Pitch Manipulated Wind Turbine in the Full Load Regime
Authors:T. Shaqarin, Mahmoud M. S. Al-Suod
Abstract: In a wind energy conversion system (WECS), changing the pitch angle of the wind turbine blades is a typical practice to regulate the electrical power generation in the full-load regime. Due to the turbulent nature of the wind and the large variations of the mean wind speed during the day, the rotary elements of the WECS are subjected to significant mechanical stresses and fatigue, resulting in conceivably mechanical failures and higher maintenance costs. Consequently, it is imperative to design a control system capable of handling continuous wind changes. In this work, Linear Parameter Varying (LPV) H_inf controller is used to cope with wind variations and turbulent winds with a turbulence intensity greater than 10%. The proposed controller is designed to regulate the rotational rotor speed and generator torque, thus, regulating the output power via pitch angle manipulations. In addition, a PI-Fuzzy control system is designed to be compared with the proposed control system. The closed-loop simulations of both controllers established the robustness and stability of the suggested LPV controller under large wind velocity variations, with minute power fluctuations compared to the PI-Fuzzy controller. The results show that in the presence of turbulent wind speed variations, the proposed LPV controller achieves improved transient and steady-state performance along with reduced mechanical loads in the above-rated wind speed region.