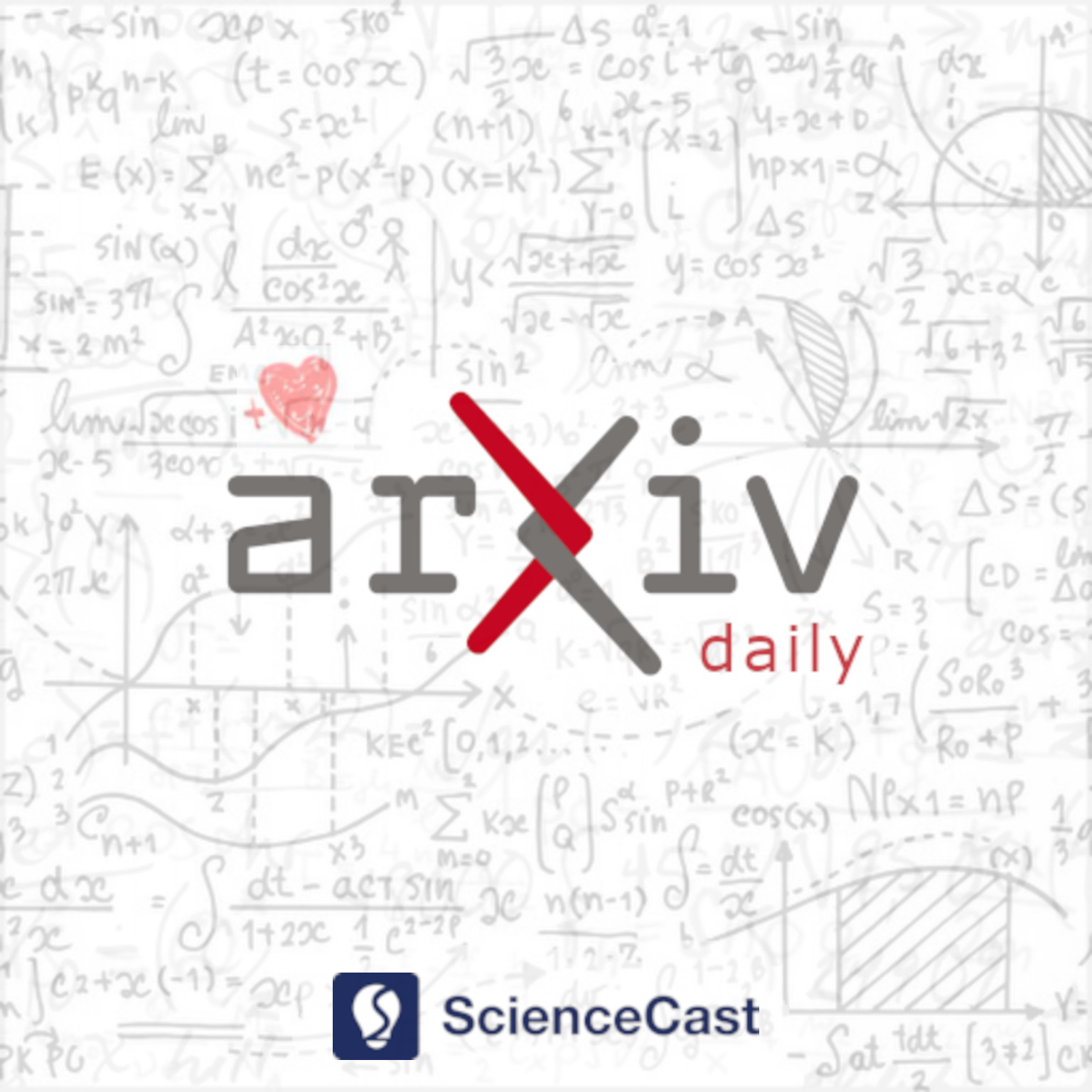
Optimization and Control (math.OC)
Mon, 05 Jun 2023
1.On the convergence of the $k$-point bound for topological packing graphs
Authors:Bram Bekker, Fernando Mário de Oliveira Filho
Abstract: We show that the $k$-point bound of de Laat, Machado, Oliveira, and Vallentin, a hierarchy of upper bounds for the independence number of a topological packing graph derived from the Lasserre hierarchy, converges to the independence number.
2.On the Split Closure of the Periodic Timetabling Polytope
Authors:Niels Lindner, Berenike Masing
Abstract: The Periodic Event Scheduling Problem (PESP) is the central mathematical tool for periodic timetable optimization in public transport. PESP can be formulated in several ways as a mixed-integer linear program with typically general integer variables. We investigate the split closure of these formulations and show that split inequalities are identical with the recently introduced flip inequalities. While split inequalities are a general mixed-integer programming technique, flip inequalities are defined in purely combinatorial terms, namely cycles and arc sets of the digraph underlying the PESP instance. It is known that flip inequalities can be separated in pseudo-polynomial time. We prove that this is best possible unless P $=$ NP, but also observe that the complexity becomes linear-time if the cycle defining the flip inequality is fixed. Moreover, introducing mixed-integer-compatible maps, we compare the split closures of different formulations, and show that reformulation or binarization by subdivision do not lead to stronger split closures. Finally, we estimate computationally how much of the optimality gap of the instances of the benchmark library PESPlib can be closed exclusively by split cuts, and provide better dual bounds for five instances.
3.Tight Big-Ms for Optimal Transmission Switching
Authors:Salvador Pineda, Juan Miguel Morales, Álvaro Porras, Concepción Domínguez
Abstract: This paper addresses the Optimal Transmission Switching (OTS) problem in electricity networks, which aims to find an optimal power grid topology that minimizes system operation costs while satisfying physical and operational constraints. Existing methods typically convert the OTS problem into a Mixed-Integer Linear Program (MILP) using big-M constants. However, the computational performance of these approaches relies significantly on the tightness of these big-Ms. In this paper, we propose an iterative tightening strategy to strengthen the big-Ms by efficiently solving a series of bounding problems that account for the economics of the OTS objective function through an upper-bound on the generating cost. We also discuss how the performance of the proposed tightening strategy is enhanced if reduced line capacities are considered. Using the 118-bus test system we demonstrate that the proposed methodology outperforms existing approaches, offering tighter bounds and significantly reducing the computational burden of the OTS problem.
4.Integer Programming Games: A Gentle Computational Overview
Authors:Margarida Carvalho, Gabriele Dragotto, Andrea Lodi, Sriram Sankaranarayan
Abstract: In this tutorial, we present a computational overview on computing Nash equilibria in Integer Programming Games ($IPG$s), $i.e.$, how to compute solutions for a class of non-cooperative and nonconvex games where each player solves a mixed-integer optimization problem. $IPG$s are a broad class of games extending the modeling power of mixed-integer optimization to multi-agent settings. This class of games includes, for instance, any finite game and any multi-agent extension of traditional combinatorial optimization problems. After providing some background motivation and context of applications, we systematically review and classify the state-of-the-art algorithms to compute Nash equilibria. We propose an essential taxonomy of the algorithmic ingredients needed to compute equilibria, and we describe the theoretical and practical challenges associated with equilibria computation. Finally, we quantitatively and qualitatively compare a sequential Stackelberg game with a simultaneous $IPG$ to highlight the different properties of their solutions.
5.Probabilistic Region-of-Attraction Estimation with Scenario Optimization and Converse Theorems
Authors:Torbjørn Cunis
Abstract: The region of attraction characterizes well-behaved and safe operation of a nonlinear system and is hence sought after for verification. In this paper, a framework for probabilistic region of attraction estimation is developed that combines scenario optimization and converse theorems. With this approach, the probability of an unstable condition being included in the estimate is independent of the system's complexity, while convergence in probability to the true region of attraction is proven. Numerical examples demonstrate the effectiveness for optimization-based control applications. Combining systems theory and sampling, the complexity of Monte--Carlo-based verification techniques can be reduced. The results can be extended to arbitrary level sets of which the defining function can be sampled, such as finite-horizon viability. Thus, the proposed approach is applicable and/or adaptable to verification of a wide range of safety-related properties for nonlinear systems including feedback laws based on optimization or learning.
6.Exact Two-Step Benders Decomposition for Two-Stage Stochastic Mixed-Integer Programs
Authors:Sifa Celik, Layla Martin, Albert H. Schrotenboer, Tom Van Woensel
Abstract: Many real-life optimization problems belong to the class of two-stage stochastic mixed-integer programming problems with continuous recourse. This paper introduces Two-Step Benders Decomposition with Scenario Clustering (TBDS) as a general exact solution methodology for solving such stochastic programs to optimality. The method combines and generalizes Benders dual decomposition, partial Benders decomposition, and Scenario Clustering techniques and does so within a novel two-step decomposition along the binary and continuous first-stage decisions. We use TBDS to provide the first exact solutions for the so-called Time Window Assignment Traveling Salesperson problem. This is a canonical optimization problem for service-oriented vehicle routing; it considers jointly assigning time windows to customers and routing a vehicle among them while travel times are stochastic. Extensive experiments show that TBDS is superior to state-of-the-art approaches in the literature. It solves instances with up to 25 customers to optimality. It provides better lower and upper bounds that lead to faster convergence than related methods. For example, Benders dual decomposition cannot solve instances of 10 customers to optimality. We use TBDS to analyze the structure of the optimal solutions. By increasing routing costs only slightly, customer service can be improved tremendously, driven by smartly alternating between high- and low-variance travel arcs to reduce the impact of delay propagation throughout the executed vehicle route.
7.Curvature and complexity: Better lower bounds for geodesically convex optimization
Authors:Christopher Criscitiello, Nicolas Boumal
Abstract: We study the query complexity of geodesically convex (g-convex) optimization on a manifold. To isolate the effect of that manifold's curvature, we primarily focus on hyperbolic spaces. In a variety of settings (smooth or not; strongly g-convex or not; high- or low-dimensional), known upper bounds worsen with curvature. It is natural to ask whether this is warranted, or an artifact. For many such settings, we propose a first set of lower bounds which indeed confirm that (negative) curvature is detrimental to complexity. To do so, we build on recent lower bounds (Hamilton and Moitra, 2021; Criscitiello and Boumal, 2022) for the particular case of smooth, strongly g-convex optimization. Using a number of techniques, we also secure lower bounds which capture dependence on condition number and optimality gap, which was not previously the case. We suspect these bounds are not optimal. We conjecture optimal ones, and support them with a matching lower bound for a class of algorithms which includes subgradient descent, and a lower bound for a related game. Lastly, to pinpoint the difficulty of proving lower bounds, we study how negative curvature influences (and sometimes obstructs) interpolation with g-convex functions.
8.Frequency Regulation with Storage: On Losses and Profits
Authors:Dirk Lauinger, François Vuille, Daniel Kuhn
Abstract: Low-carbon societies will need to store vast amounts of electricity to balance intermittent generation from wind and solar energy, for example, through frequency regulation. Here, we derive an analytical solution to the decision-making problem of storage operators who sell frequency regulation power to grid operators and trade electricity on day-ahead markets. Mathematically, we treat future frequency deviation trajectories as functional uncertainties in a receding horizon robust optimization problem. We constrain the expected terminal state-of-charge to be equal to some target to allow storage operators to make good decisions not only for the present but also the future. Thanks to this constraint, the amount of electricity traded on day-ahead markets is an implicit function of the regulation power sold to grid operators. The implicit function quantifies the amount of power that needs to be purchased to cover the expected energy loss that results from providing frequency regulation. We show how the marginal cost associated with the expected energy loss decreases with roundtrip efficiency and increases with frequency deviation dispersion. We find that the profits from frequency regulation over the lifetime of energy-constrained storage devices are roughly inversely proportional to the length of time for which regulation power must be committed.
9.Explicit feedback synthesis driven by quasi-interpolation for nonlinear robust model predictive control
Authors:Siddhartha Ganguly, Debasish Chatterjee
Abstract: We present QuIFS (Quasi-Interpolation driven Feedback Synthesis) -- an offline feedback synthesis algorithm for explicit nonlinear robust minmax model predictive control (MPC) problems with guaranteed quality of approximation. The underlying technique is driven by a particular type of grid-based quasi-interpolation scheme. The QuIFS algorithm departs drastically from conventional approximation algorithms that are employed in the MPC industry (in particular, it is neither based on multi-parametric programming tools nor does it involve kernel methods), and the essence of their point of departure is encoded in the following challenge-answer approach: Given an error margin $\varepsilon>0$, compute a feasible feedback policy that is uniformly $\varepsilon$-close to the optimal MPC feedback policy for a given nonlinear system subjected to hard constraints and bounded uncertainties. Conditions for closed-loop stability and recursive feasibility under the approximate feedback policy are also established. We provide a library of numerical examples to illustrate our results.
10.Entropic mean-field min-max problems via Best Response and Fisher-Rao flows
Authors:Razvan-Andrei Lascu, Mateusz B. Majka, Łukasz Szpruch
Abstract: We investigate convergence properties of two continuous-time optimization methods, the Mean-Field Best Response and the Fisher-Rao (Mean-Field Birth-Death) flows, for solving convex-concave min-max games with entropy regularization. We introduce suitable Lyapunov functions to establish exponential convergence to the unique mixed Nash equilibrium for both methods, albeit under slightly different conditions. Additionally, we demonstrate the convergence of the fictitious play flow as a by-product of our analysis.