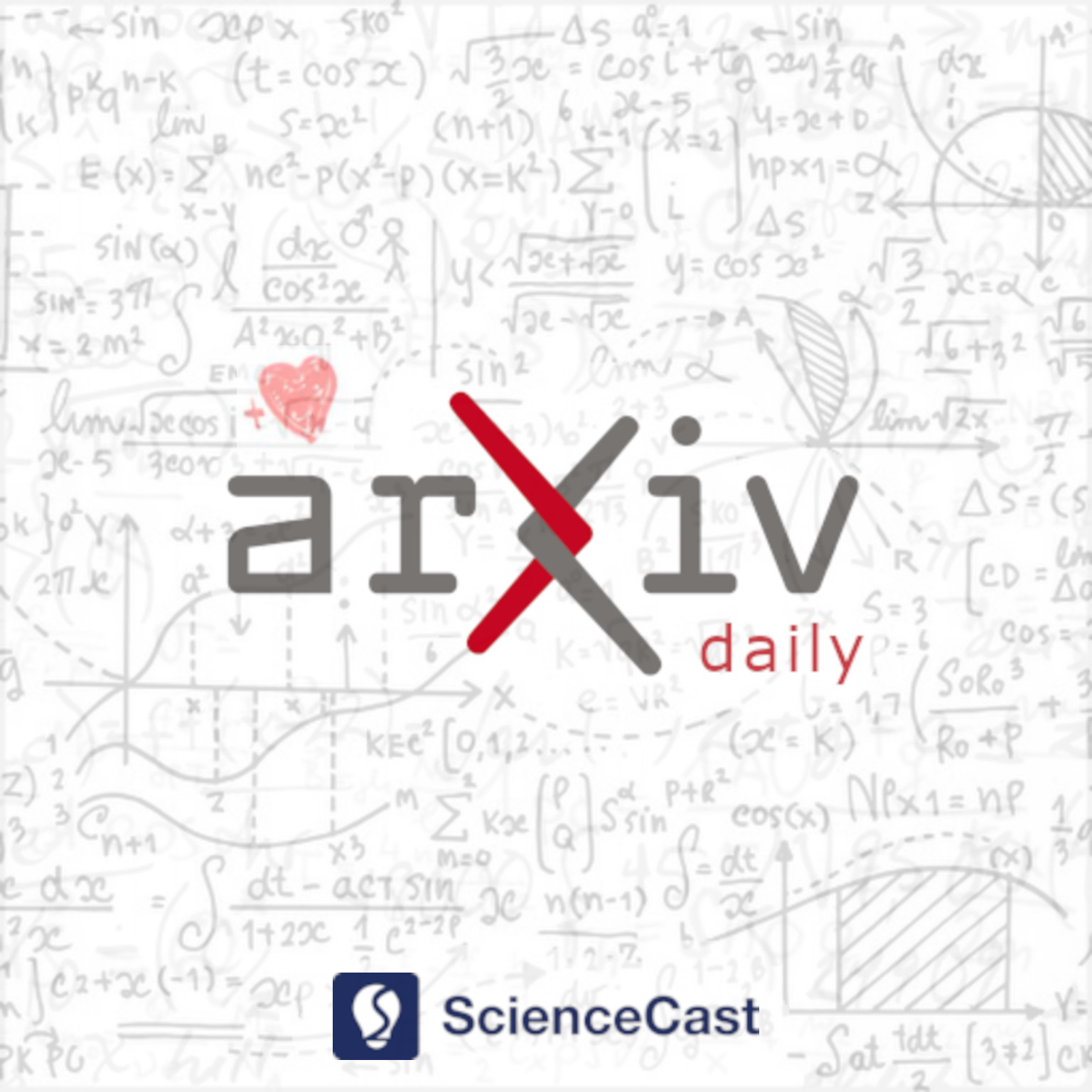
Optimization and Control (math.OC)
Thu, 18 May 2023
1.A New Perspective of Accelerated Gradient Methods: The Controlled Invariant Manifold Approach
Authors:Revati Gunjal, Sushama Wagh, Syed Shadab Nayyer, Alex Stankovic, Navdeep M. Singh
Abstract: Gradient Descent (GD) is a ubiquitous algorithm for finding the optimal solution to an optimization problem. For reduced computational complexity, the optimal solution $\mathrm{x^*}$ of the optimization problem must be attained in a minimum number of iterations. For this objective, the paper proposes a genesis of an accelerated gradient algorithm through the controlled dynamical system perspective. The objective of optimally reaching the optimal solution $\mathrm{x^*}$ where $\mathrm{\nabla f(x^*)=0}$ with a given initial condition $\mathrm{x(0)}$ is achieved through control.
2.On the Geometric Convergence of Byzantine-Resilient Distributed Optimization Algorithms
Authors:Kananart Kuwaranancharoen, Shreyas Sundaram
Abstract: The problem of designing distributed optimization algorithms that are resilient to Byzantine adversaries has received significant attention. For the Byzantine-resilient distributed optimization problem, the goal is to (approximately) minimize the average of the local cost functions held by the regular (non adversarial) agents in the network. In this paper, we provide a general algorithmic framework for Byzantine-resilient distributed optimization which includes some state-of-the-art algorithms as special cases. We analyze the convergence of algorithms within the framework, and derive a geometric rate of convergence of all regular agents to a ball around the optimal solution (whose size we characterize). Furthermore, we show that approximate consensus can be achieved geometrically fast under some minimal conditions. Our analysis provides insights into the relationship among the convergence region, distance between regular agents' values, step-size, and properties of the agents' functions for Byzantine-resilient distributed optimization.
3.Optimization Modeling for Pandemic Vaccine Supply Chain Management: A Review
Authors:Shibshankar Dey, Ali Kaan Kurbanzade, Esma S. Gel, Joseph Mihaljevic, Sanjay Mehrotra
Abstract: During various stages of the COVID-19 pandemic, countries implemented diverse vaccine management approaches, influenced by variations in infrastructure and socio-economic conditions. This article provides a comprehensive overview of optimization models developed by the research community throughout the COVID-19 era, aimed at enhancing vaccine distribution and establishing a standardized framework for future pandemic preparedness. These models address critical issues such as site selection, inventory management, allocation strategies, distribution logistics, and route optimization encountered during the COVID-19 crisis. A unified framework is employed to describe the models, emphasizing their integration with epidemiological models to facilitate a holistic understanding.
4.Maximal workload, minimal workload, maximal workload difference: optimizing all criteria at once
Authors:Sébastien Dechamps, Frédéric Meunier
Abstract: In a simple model of assigning workers to tasks, every solution that minimizes the load difference between the most loaded worker and the least loaded one actually minimizes the maximal load and maximizes the minimal load. This can be seen as a consequence of standard results of optimization over polymatroids. We show that similar phenomena still occur in close models, simple to state, and that do not enjoy any polymatroid structure.