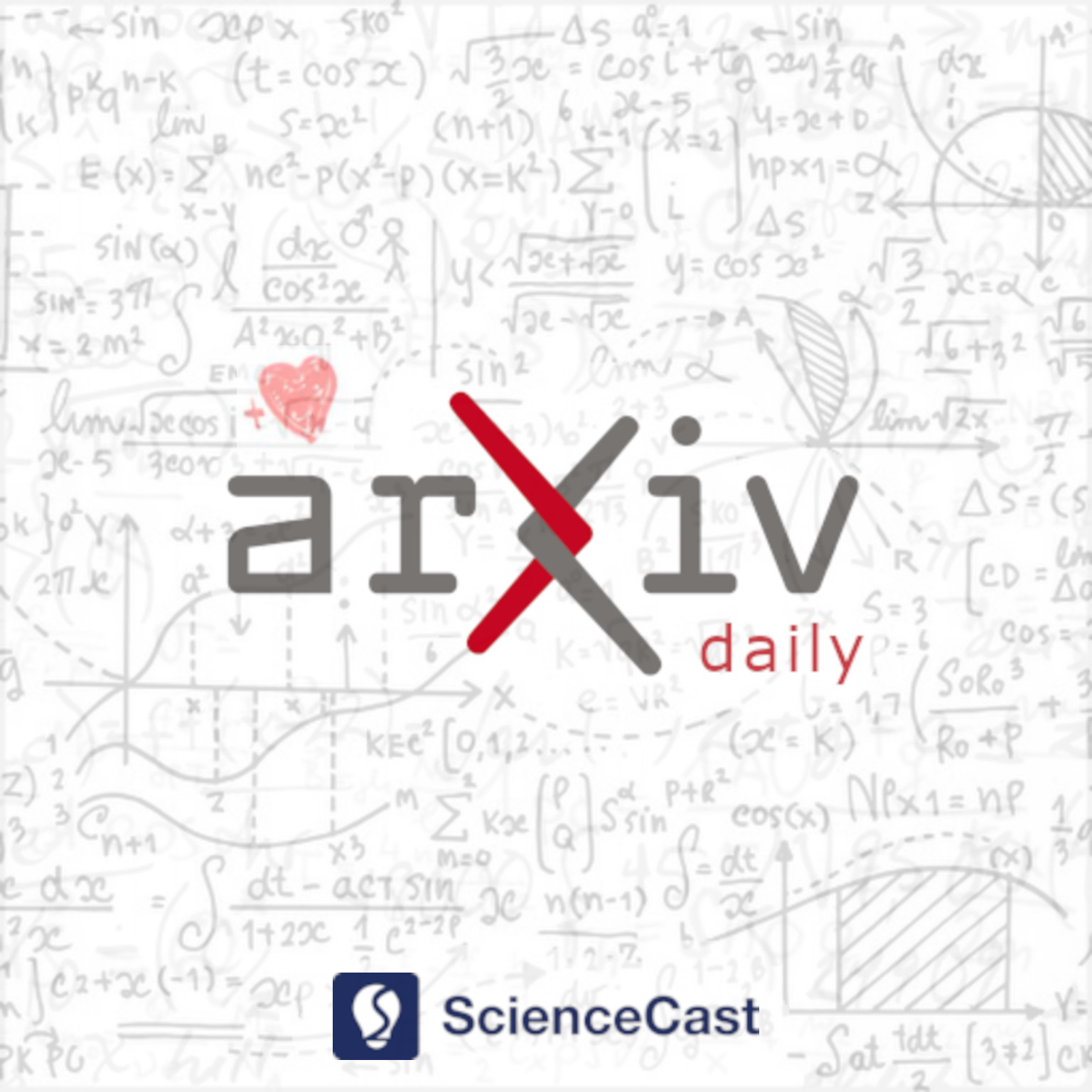
Optimization and Control (math.OC)
Tue, 30 May 2023
1.Stochastic Control/Stopping Problem with Expectation Constraints
Authors:Erhan Bayraktar, Song Yao
Abstract: We study a stochastic control/stopping problem with a series of inequality-type and equality-type expectation constraints in a general non-Markovian framework. We demonstrate that the stochastic control/stopping problem with expectation constraints (CSEC) is independent of a specific probability setting and is equivalent to the constrained stochastic control/stopping problem in weak formulation (an optimization over joint laws of Brownian motion, state dynamics, diffusion controls and stopping rules on an enlarged canonical space). Using a martingale-problem formulation of controlled SDEs in spirit of \cite{Stroock_Varadhan}, we characterize the probability classes in weak formulation by countably many actions of canonical processes, and thus obtain the upper semi-analyticity of the CSEC value function. Then we employ a measurable selection argument to establish a dynamic programming principle (DPP) in weak formulation for the CSEC value function, in which the conditional expected costs act as additional states for constraint levels at the intermediate horizon. This article extends the results of \cite{Elk_Tan_2013b} to the expectation-constraint case. We extend our previous work \cite{OSEC_stopping} to the more complicated setting where the diffusion is controlled. Compared to that paper the topological properties of diffusion-control spaces and the corresponding measurability are more technically involved which complicate the arguments especially for the measurable selection for the super-solution side of DPP in the weak formulation.
2.Blockwise Stochastic Variance-Reduced Methods with Parallel Speedup for Multi-Block Bilevel Optimization
Authors:Quanqi Hu, Zi-Hao Qiu, Zhishuai Guo, Lijun Zhang, Tianbao Yang
Abstract: In this paper, we consider non-convex multi-block bilevel optimization (MBBO) problems, which involve $m\gg 1$ lower level problems and have important applications in machine learning. Designing a stochastic gradient and controlling its variance is more intricate due to the hierarchical sampling of blocks and data and the unique challenge of estimating hyper-gradient. We aim to achieve three nice properties for our algorithm: (a) matching the state-of-the-art complexity of standard BO problems with a single block; (b) achieving parallel speedup by sampling $I$ blocks and sampling $B$ samples for each sampled block per-iteration; (c) avoiding the computation of the inverse of a high-dimensional Hessian matrix estimator. However, it is non-trivial to achieve all of these by observing that existing works only achieve one or two of these properties. To address the involved challenges for achieving (a, b, c), we propose two stochastic algorithms by using advanced blockwise variance-reduction techniques for tracking the Hessian matrices (for low-dimensional problems) or the Hessian-vector products (for high-dimensional problems), and prove an iteration complexity of $O(\frac{m\epsilon^{-3}\mathbb{I}(I<m)}{I\sqrt{I}} + \frac{m\epsilon^{-3}}{I\sqrt{B}})$ for finding an $\epsilon$-stationary point under appropriate conditions. We also conduct experiments to verify the effectiveness of the proposed algorithms comparing with existing MBBO algorithms.
3.Around a Farkas type Lemma
Authors:Nguyen Dinh, Miguel A. Goberna, M. Volle
Abstract: The first two authors of this paper asserted in Lemma 4 of "New Farkas-type constraint qualifications in convex infinite programming" (DOI: 10.1051/cocv:2007027) that a given reverse convex inequality is consequence of a given convex system satisfying the Farkas-Minkowski constraint qualification if and only if certain set depending on the data contains a particular point of the vertical axis. This paper identifies a hidden assumption in this reverse Farkas lemma which always holds in its applications to nontrivial optimization problems. Moreover, it shows that the statement remains valid when the Farkas-Minkowski constraint qualification fails by replacing the mentioned set by its closure. This hidden assumption is also characterized in terms of the data. Finally, the paper provides some applications to convex infinite systems and to convex infinite optimization problems.
4.Infinite-dimensional moment-SOS hierarchy for nonlinear partial differential equations
Authors:Didier Henrion, Maria Infusino, Salma Kuhlmann, Victor Vinnikov
Abstract: We formulate a class of nonlinear {evolution} partial differential equations (PDEs) as linear optimization problems on moments of positive measures supported on infinite-dimensional vector spaces. Using sums of squares (SOS) representations of polynomials in these spaces, we can prove convergence of a hierarchy of finite-dimensional semidefinite relaxations solving approximately these infinite-dimensional optimization problems. As an illustration, we report on numerical experiments for solving the heat equation subject to a nonlinear perturbation.
5.Global minimization of polynomial integral functionals
Authors:Giovanni Fantuzzi, Federico Fuentes
Abstract: We describe a `discretize-then-relax' strategy to globally minimize integral functionals over functions $u$ in a Sobolev space satisfying prescribed Dirichlet boundary conditions. The strategy applies whenever the integral functional depends polynomially on $u$ and its derivatives, even if it is nonconvex. The `discretize' step uses a bounded finite-element scheme to approximate the integral minimization problem with a convergent hierarchy of polynomial optimization problems over a compact feasible set, indexed by the decreasing size $h$ of the finite-element mesh. The `relax' step employs sparse moment-SOS relaxations to approximate each polynomial optimization problem with a hierarchy of convex semidefinite programs, indexed by an increasing relaxation order $\omega$. We prove that, as $\omega\to\infty$ and $h\to 0$, solutions of such semidefinite programs provide approximate minimizers that converge in $L^p$ to the global minimizer of the original integral functional if this is unique. We also report computational experiments that show our numerical strategy works well even when technical conditions required by our theoretical analysis are not satisfied.
6.Policy Gradient Algorithms for Robust MDPs with Non-Rectangular Uncertainty Sets
Authors:Mengmeng Li, Tobias Sutter, Daniel Kuhn
Abstract: We propose a policy gradient algorithm for robust infinite-horizon Markov Decision Processes (MDPs) with non-rectangular uncertainty sets, thereby addressing an open challenge in the robust MDP literature. Indeed, uncertainty sets that display statistical optimality properties and make optimal use of limited data often fail to be rectangular. Unfortunately, the corresponding robust MDPs cannot be solved with dynamic programming techniques and are in fact provably intractable. This prompts us to develop a projected Langevin dynamics algorithm tailored to the robust policy evaluation problem, which offers global optimality guarantees. We also propose a deterministic policy gradient method that solves the robust policy evaluation problem approximately, and we prove that the approximation error scales with a new measure of non-rectangularity of the uncertainty set. Numerical experiments showcase that our projected Langevin dynamics algorithm can escape local optima, while algorithms tailored to rectangular uncertainty fail to do so.
7.Adaptive Quasi-Newton and Anderson Acceleration Framework with Explicit Global (Accelerated) Convergence Rates
Authors:Damien Scieur
Abstract: Despite the impressive numerical performance of quasi-Newton and Anderson/nonlinear acceleration methods, their global convergence rates have remained elusive for over 50 years. This paper addresses this long-standing question by introducing a framework that derives novel and adaptive quasi-Newton or nonlinear/Anderson acceleration schemes. Under mild assumptions, the proposed iterative methods exhibit explicit, non-asymptotic convergence rates that blend those of gradient descent and Cubic Regularized Newton's method. Notably, these rates are achieved adaptively, as the method autonomously determines the optimal step size using a simple backtracking strategy. The proposed approach also includes an accelerated version that improves the convergence rate on convex functions. Numerical experiments demonstrate the efficiency of the proposed framework, even compared to a fine-tuned BFGS algorithm with line search.
8.Fast global convergence of gradient descent for low-rank matrix approximation
Authors:Hengchao Chen, Xin Chen, Mohamad Elmasri, Qiang Sun
Abstract: This paper investigates gradient descent for solving low-rank matrix approximation problems. We begin by establishing the local linear convergence of gradient descent for symmetric matrix approximation. Building on this result, we prove the rapid global convergence of gradient descent, particularly when initialized with small random values. Remarkably, we show that even with moderate random initialization, which includes small random initialization as a special case, gradient descent achieves fast global convergence in scenarios where the top eigenvalues are identical. Furthermore, we extend our analysis to address asymmetric matrix approximation problems and investigate the effectiveness of a retraction-free eigenspace computation method. Numerical experiments strongly support our theory. In particular, the retraction-free algorithm outperforms the corresponding Riemannian gradient descent method, resulting in a significant 29\% reduction in runtime.
9.Learning for Robust Optimization
Authors:Irina Wang, Cole Becker, Bart Van Parys, Bartolomeo Stellato
Abstract: We propose a data-driven technique to automatically learn the uncertainty sets in robust optimization. Our method reshapes the uncertainty sets by minimizing the expected performance across a family of problems while guaranteeing constraint satisfaction. We learn the uncertainty sets using a novel stochastic augmented Lagrangian method that relies on differentiating the solutions of the robust optimization problems with respect to the parameters of the uncertainty set. We show sublinear convergence to stationary points under mild assumptions, and finite-sample probabilistic guarantees of constraint satisfaction using empirical process theory. Our approach is very flexible and can learn a wide variety of uncertainty sets while preserving tractability. Numerical experiments show that our method outperforms traditional approaches in robust and distributionally robust optimization in terms of out of sample performance and constraint satisfaction guarantees. We implemented our method in the open-source package LROPT.
10.Minimal Sparsity for Second-Order Moment-SOS Relaxations of the AC-OPF Problem
Authors:Adrien Le Franc LAAS-POP, Victor Magron LAAS-POP,IMT, Jean-Bernard Lasserre LAAS-POP, Manuel Ruiz, Patrick Panciatici
Abstract: AC-OPF (Alternative Current Optimal Power Flow)aims at minimizing the operating costs of a power gridunder physical constraints on voltages and power injections.Its mathematical formulation results in a nonconvex polynomial optimizationproblem which is hard to solve in general,but that can be tackled by a sequence of SDP(Semidefinite Programming) relaxationscorresponding to the steps of the moment-SOS (Sums-Of-Squares) hierarchy.Unfortunately, the size of these SDPs grows drastically in the hierarchy,so that even second-order relaxationsexploiting the correlative sparsity pattern of AC-OPFare hardly numerically tractable for largeinstances -- with thousands of power buses.Our contribution lies in a new sparsityframework, termed minimal sparsity, inspiredfrom the specific structure of power flowequations.Despite its heuristic nature, numerical examples show that minimal sparsity allows the computation ofhighly accurate second-order moment-SOS relaxationsof AC-OPF, while requiring far less computing time and memory resources than the standard correlative sparsity pattern. Thus, we manage to compute second-order relaxations on test caseswith about 6000 power buses, which we believe to be unprecedented.