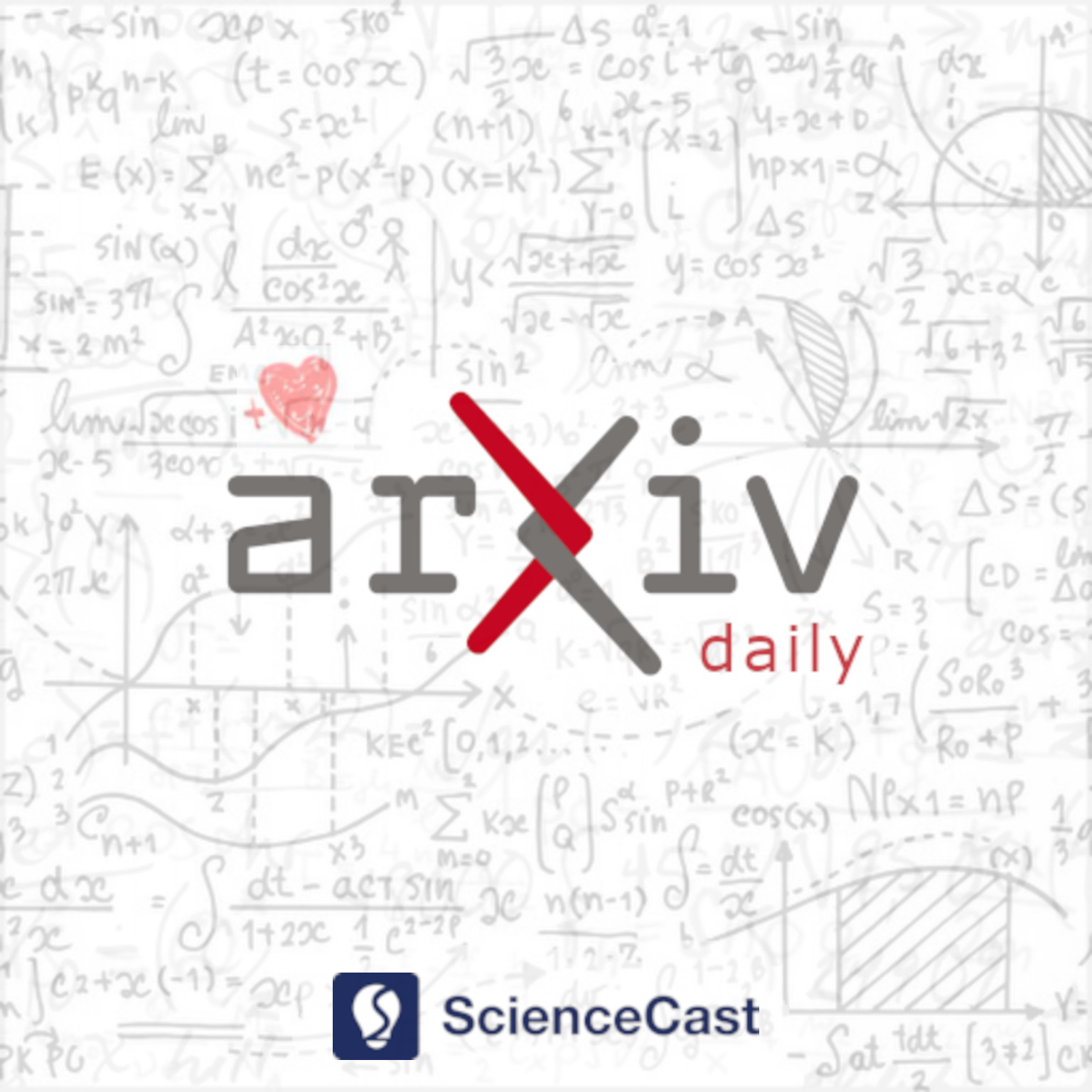
Methodology (stat.ME)
Fri, 19 May 2023
1.A general model-checking procedure for semiparametric accelerated failure time models
Authors:Dongrak Choi, Woojung Bae, Jun Yan, Sangwook Kang
Abstract: We propose a set of goodness-of-fit tests for the semiparametric accelerated failure time (AFT) model, including an omnibus test, a link function test, and a functional form test. This set of tests is derived from a multi-parameter cumulative sum process shown to follow asymptotically a zero-mean Gaussian process. Its evaluation is based on the asymptotically equivalent perturbed version, which enables both graphical and numerical evaluations of the assumed AFT model. Empirical p-values are obtained using the Kolmogorov-type supremum test, which provides a reliable approach for estimating the significance of both proposed un-standardized and standardized test statistics. The proposed procedure is illustrated using the induced smoothed rank-based estimator but is directly applicable to other popular estimators such as non-smooth rank-based estimator or least-squares estimator.Our proposed methods are rigorously evaluated using extensive simulation experiments that demonstrate their effectiveness in maintaining a Type I error rate and detecting departures from the assumed AFT model in practical sample sizes and censoring rates. Furthermore, the proposed approach is applied to the analysis of the Primary Biliary Cirrhosis data, a widely studied dataset in survival analysis, providing further evidence of the practical usefulness of the proposed methods in real-world scenarios. To make the proposed methods more accessible to researchers, we have implemented them in the R package afttest, which is publicly available on the Comprehensive R Archieve Network.
2.Structured factorization for single-cell gene expression data
Authors:Antonio Canale, Luisa Galtarossa, Davide Risso, Lorenzo Schiavon, Giovanni Toto
Abstract: Single-cell gene expression data are often characterized by large matrices, where the number of cells may be lower than the number of genes of interest. Factorization models have emerged as powerful tools to condense the available information through a sparse decomposition into lower rank matrices. In this work, we adapt and implement a recent Bayesian class of generalized factor models to count data and, specifically, to model the covariance between genes. The developed methodology also allows one to include exogenous information within the prior, such that recognition of covariance structures between genes is favoured. In this work, we use biological pathways as external information to induce sparsity patterns within the loadings matrix. This approach facilitates the interpretation of loadings columns and the corresponding latent factors, which can be regarded as unobserved cell covariates. We demonstrate the effectiveness of our model on single-cell RNA sequencing data obtained from lung adenocarcinoma cell lines, revealing promising insights into the role of pathways in characterizing gene relationships and extracting valuable information about unobserved cell traits.
3.Off-policy evaluation beyond overlap: partial identification through smoothness
Authors:Samir Khan, Martin Saveski, Johan Ugander
Abstract: Off-policy evaluation (OPE) is the problem of estimating the value of a target policy using historical data collected under a different logging policy. OPE methods typically assume overlap between the target and logging policy, enabling solutions based on importance weighting and/or imputation. In this work, we approach OPE without assuming either overlap or a well-specified model by considering a strategy based on partial identification under non-parametric assumptions on the conditional mean function, focusing especially on Lipschitz smoothness. Under such smoothness assumptions, we formulate a pair of linear programs whose optimal values upper and lower bound the contributions of the no-overlap region to the off-policy value. We show that these linear programs have a concise closed form solution that can be computed efficiently and that their solutions converge, under the Lipschitz assumption, to the sharp partial identification bounds on the off-policy value. Furthermore, we show that the rate of convergence is minimax optimal, up to log factors. We deploy our methods on two semi-synthetic examples, and obtain informative and valid bounds that are tighter than those possible without smoothness assumptions.