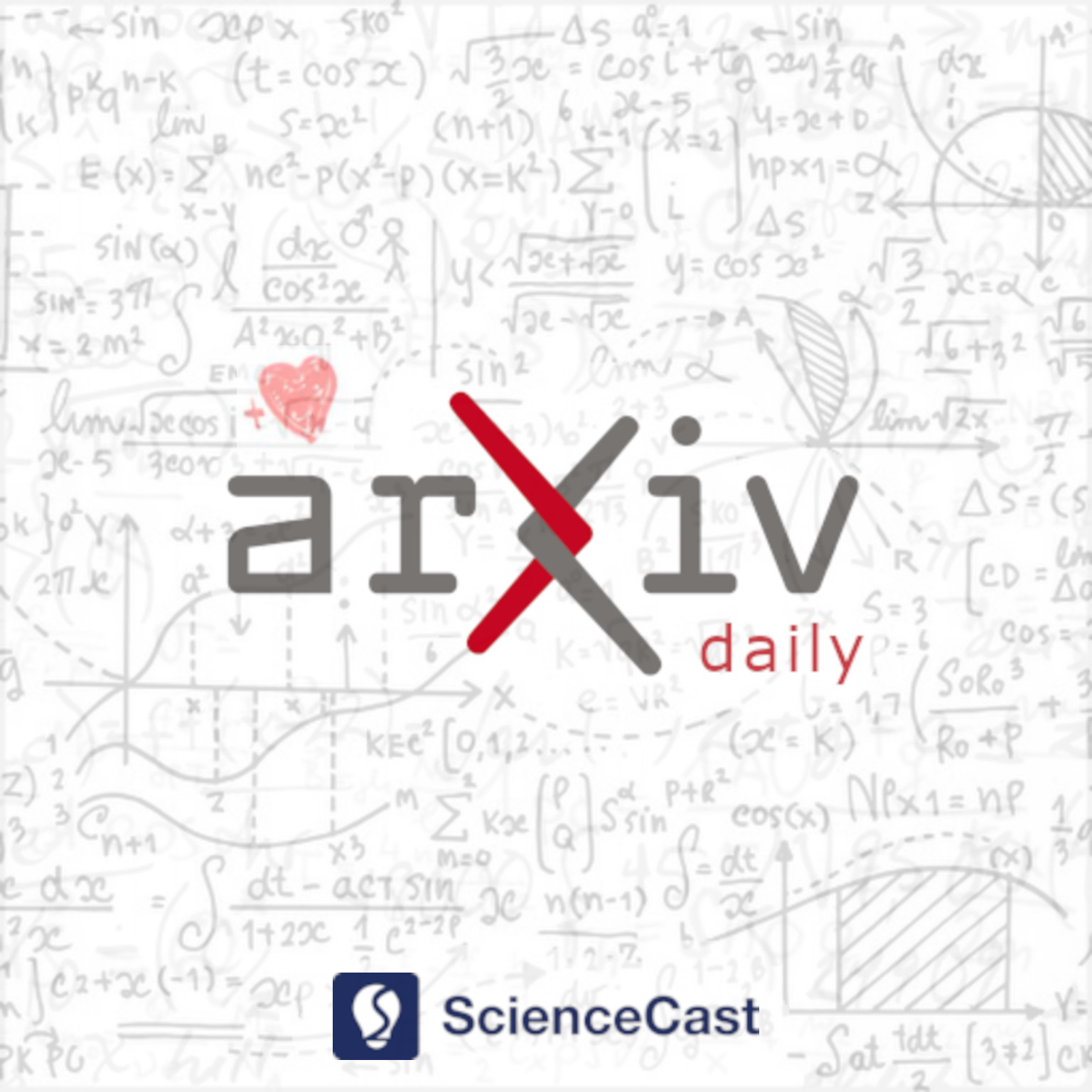
Methodology (stat.ME)
Fri, 14 Apr 2023
1.Efficient Estimation in Extreme Value Regression Models of Hedge Fund Tail Risks
Authors:Julien Hambuckers, Marie Kratz, Antoine Usseglio-Carleve
Abstract: We introduce a method to estimate simultaneously the tail and the threshold parameters of an extreme value regression model. This standard model finds its use in finance to assess the effect of market variables on extreme loss distributions of investment vehicles such as hedge funds. However, a major limitation is the need to select ex ante a threshold below which data are discarded, leading to estimation inefficiencies. To solve these issues, we extend the tail regression model to non-tail observations with an auxiliary splicing density, enabling the threshold to be selected automatically. We then apply an artificial censoring mechanism of the likelihood contributions in the bulk of the data to decrease specification issues at the estimation stage. We illustrate the superiority of our approach for inference over classical peaks-over-threshold methods in a simulation study. Empirically, we investigate the determinants of hedge fund tail risks over time, using pooled returns of 1,484 hedge funds. We find a significant link between tail risks and factors such as equity momentum, financial stability index, and credit spreads. Moreover, sorting funds along exposure to our tail risk measure discriminates between high and low alpha funds, supporting the existence of a fear premium.
2.Estimating Conditional Average Treatment Effects with Heteroscedasticity by Model Averaging and Matching
Authors:Pengfei Shi, Xinyu Zhang, Wei Zhong
Abstract: Causal inference is indispensable in many fields of empirical research, such as marketing, economic policy, medicine and so on. The estimation of treatment effects is the most important in causal inference. In this paper, we propose a model averaging approach, combined with a partition and matching method to estimate the conditional average treatment effect under heteroskedastic error settings. In our methods, we use the partition and matching method to approximate the true treatment effects and choose the weights by minimizing a leave-one-out cross validation criterion, which is also known as jackknife method. We prove that the model averaging estimator with weights determined by our criterion has asymptotic optimality, which achieves the lowest possible squared error. When there exist correct models in the candidate model set, we have proved that the sum of weights of the correct models converge to 1 as the sample size increases but with a finite number of baseline covariates. A Monte Carlo simulation study shows that our method has good performance in finite sample cases. We apply this approach to a National Supported Work Demonstration data set.
3.Detection and Estimation of Structural Breaks in High-Dimensional Functional Time Series
Authors:Degui Li, Runze Li, Han Lin Shang
Abstract: In this paper, we consider detecting and estimating breaks in heterogeneous mean functions of high-dimensional functional time series which are allowed to be cross-sectionally correlated and temporally dependent. A new test statistic combining the functional CUSUM statistic and power enhancement component is proposed with asymptotic null distribution theory comparable to the conventional CUSUM theory derived for a single functional time series. In particular, the extra power enhancement component enlarges the region where the proposed test has power, and results in stable power performance when breaks are sparse in the alternative hypothesis. Furthermore, we impose a latent group structure on the subjects with heterogeneous break points and introduce an easy-to-implement clustering algorithm with an information criterion to consistently estimate the unknown group number and membership. The estimated group structure can subsequently improve the convergence property of the post-clustering break point estimate. Monte-Carlo simulation studies and empirical applications show that the proposed estimation and testing techniques have satisfactory performance in finite samples.
4.Recursive Neyman Algorithm for Optimum Sample Allocation under Box Constraints on Sample Sizes in Strata
Authors:Jacek Wesołowski, Robert Wieczorkowski, Wojciech Wójciak
Abstract: The optimal sample allocation in stratified sampling is one of the basic issues of modern survey sampling methodology. It is a procedure of dividing the total sample among pairwise disjoint subsets of a finite population, called strata, such that for chosen survey sampling designs in strata, it produces the smallest variance for estimating a population total (or mean) of a given study variable. In this paper we are concerned with the optimal allocation of a sample, under lower and upper bounds imposed jointly on the sample strata-sizes. We will consider a family of sampling designs that give rise to variances of estimators of a natural generic form. In particular, this family includes simple random sampling without replacement (abbreviated as SI) in strata, which is perhaps, the most important example of stratified sampling design. First, we identify the allocation problem as a convex optimization problem. This methodology allows to establish a generic form of the optimal solution, so called optimality conditions. Second, based on these optimality conditions, we propose new and efficient recursive algorithm, named RNABOX, which solves the allocation problem considered. This new algorithm can be viewed as a generalization of the classical recursive Neyman allocation algorithm, a popular tool for optimal sample allocation in stratified sampling with SI design in all strata, when only upper bounds are imposed on sample strata-sizes. We implement the RNABOX in R as a part of our package stratallo, which is available from the Comprehensive R Archive Network (CRAN). Finally, in the context of the established optimality conditions, we briefly discuss two existing methodologies dedicated to the allocation problem being studied: the noptcond algorithm introduced in Gabler, Ganninger and M\"unnich (2012); and fixed iteration procedures from M\"unnich, Sachs and Wagner (2012).
5.Dynamic variable selection in high-dimensional predictive regressions
Authors:Mauro Bernardi, Daniele Bianchi, Nicolas Bianco
Abstract: We develop methodology and theory for a general Bayesian approach towards dynamic variable selection in high-dimensional regression models with time-varying parameters. Specifically, we propose a variational inference scheme which features dynamic sparsity-inducing properties so that different subsets of ``active'' predictors can be identified over different time periods. We compare our modeling framework against established static and dynamic variable selection methods both in simulation and within the context of two common problems in macroeconomics and finance: inflation forecasting and equity returns predictability. The results show that our approach helps to tease out more accurately the dynamic impact of different predictors over time. This translates into significant gains in terms of out-of-sample point and density forecasting accuracy. We believe our results highlight the importance of taking a dynamic approach towards variable selection for economic modeling and forecasting.
6.Causal inference with a functional outcome
Authors:Kreske Ecker, Xavier de Luna, Lina Schelin
Abstract: This paper presents methods to study the causal effect of a binary treatment on a functional outcome with observational data. We define a functional causal parameter, the Functional Average Treatment Effect (FATE), and propose a semi-parametric outcome regression estimator. Quantifying the uncertainty in the estimation presents a challenge since existing inferential techniques developed for univariate outcomes cannot satisfactorily address the multiple comparison problem induced by the functional nature of the causal parameter. We show how to obtain valid inference on the FATE using simultaneous confidence bands, which cover the FATE with a given probability over the entire domain. Simulation experiments illustrate the empirical coverage of the simultaneous confidence bands in finite samples. Finally, we use the methods to infer the effect of early adult location on subsequent income development for one Swedish birth cohort.
7.On the Generic Cut--Point Detection Procedure in the Binomial Group Testing
Authors:Ugnė Čižikovienė, Viktor Skorniakov
Abstract: Initially announced by Dorfman in 1943, (Binomial) Group Testing (BGT) was quickly recognized as a useful tool in many other fields as well. To apply any particular BGT procedure effectively, one first of all needs to know an important operating characteristic, the so called Optimal Cut-Point (OCP), describing the limits of its applicability. The determination of the latter is often a complicated task. In this work, we provide a generic algorithm suitable for a wide class of the BGT procedures and demonstrate its applicability by example. The way we do it exhibits independent interest since we link the BGT to seemingly unrelated field -- the bifurcation theory.
8.Weighted quantile estimators
Authors:Andrey Akinshin
Abstract: In this paper, we consider a generic scheme that allows building weighted versions of various quantile estimators, such as traditional quantile estimators based on linear interpolation of two order statistics, the Harrell-Davis quantile estimator and its trimmed modification. The obtained weighted quantile estimators are especially useful in the problem of estimating a distribution at the tail of a time series using quantile exponential smoothing. The presented approach can also be applied to other problems, such as quantile estimation of weighted mixture distributions.