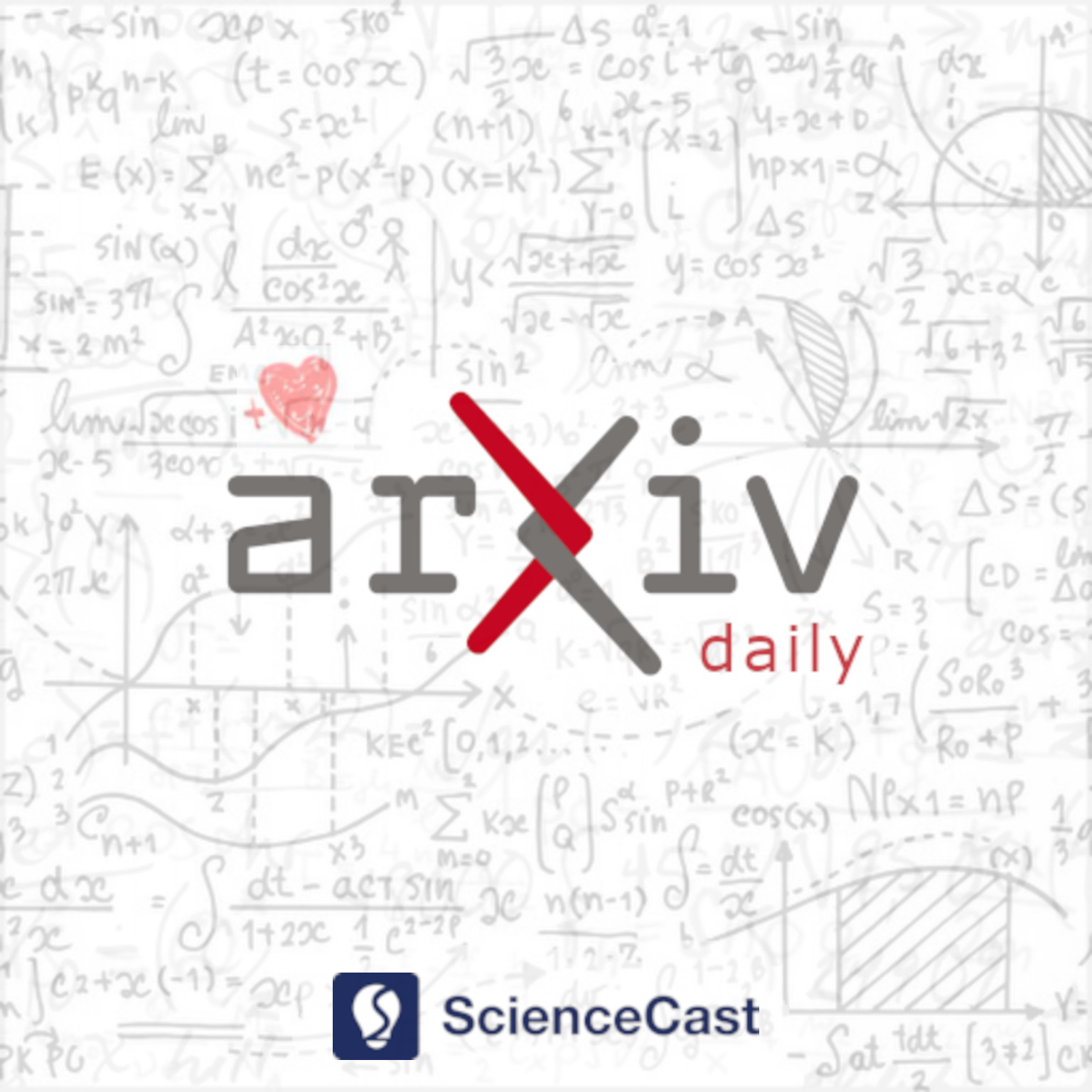
Methodology (stat.ME)
Wed, 31 May 2023
1.Testing Truncation Dependence: The Gumbel Copula
Authors:Anne-Marie Toparkus, Rafael Weißbach
Abstract: In the analysis of left- and double-truncated durations, it is often assumed that the age at truncation is independent of the duration. When truncation is a result of data collection in a restricted time period, the truncation age is equivalent to the date of birth. The independence assumption is then at odds with any demographic progress when life expectancy increases with time, with evidence e.g. on human demography in western civilisations. We model dependence with a Gumbel copula. Marginally, it is assumed that the duration of interest is exponentially distributed, and that births stem from a homogeneous Poisson process. The log-likelihood of the data, considered as truncated sample, is derived from standard results for point processes. Testing for positive dependence must include that the hypothetical independence is associated with the boundary of the parameter space. By non-standard theory, the maximum likelihood estimator of the exponential and the Gumbel parameter is distributed as a mixture of a two- and a one-dimensional normal distribution. For the proof, the third parameter, the unobserved sample size, is profiled out. Furthermore, verifying identification is simplified by noting that the score of the profile model for the truncated sample is equal to the score for a simple sample from the truncated population. In an application to 55 thousand double-truncated lifetimes of German businesses that closed down over the period 2014 to 2016, the test does not find an increase in business life expectancy for later years of the foundation. The $p$-value is $0.5$ because the likelihood has its maximum for the Gumbel parameter at the parameter space boundary. A simulation under the condition of the application suggests that the test retains the nominal level and has good power.
2.Causal discovery for time series with constraint-based model and PMIME measure
Authors:Antonin Arsac, Aurore Lomet, Jean-Philippe Poli
Abstract: Causality defines the relationship between cause and effect. In multivariate time series field, this notion allows to characterize the links between several time series considering temporal lags. These phenomena are particularly important in medicine to analyze the effect of a drug for example, in manufacturing to detect the causes of an anomaly in a complex system or in social sciences... Most of the time, studying these complex systems is made through correlation only. But correlation can lead to spurious relationships. To circumvent this problem, we present in this paper a novel approach for discovering causality in time series data that combines a causal discovery algorithm with an information theoretic-based measure. Hence the proposed method allows inferring both linear and non-linear relationships and building the underlying causal graph. We evaluate the performance of our approach on several simulated data sets, showing promising results.
3.Sensitivity analysis for publication bias on the time-dependent summary ROC analysis in meta-analysis of prognosis studies
Authors:Yi Zhou, Ao Huang, Satoshi Hattori
Abstract: In the analysis of prognosis studies with time-to-event outcomes, dichotomization of patients is often made. As the evaluations of prognostic capacity, the survivals of groups with high/low expression of the biomarker are often estimated by the Kaplan-Meier method, and the difference between groups is summarized via the hazard ratio (HR). The high/low expressions are usually determined by study-specific cutoff values, which brings heterogeneity over multiple prognosis studies and difficulty to synthesizing the results in a simple way. In meta-analysis of diagnostic studies with binary outcomes, the summary receiver operating characteristics (SROC) analysis provides a useful cutoff-free summary over studies. Recently, this methodology has been extended to the time-dependent SROC analysis for time-to-event outcomes in meta-analysis of prognosis studies. In this paper, we propose a sensitivity analysis method for evaluating the impact of publication bias on the time-dependent SROC analysis. Our proposal extends the recently introduced sensitivity analysis method for meta-analysis of diagnostic studies based on the bivariate normal model on sensitivity and specificity pairs. To model the selective publication process specific to prognosis studies, we introduce a trivariate model on the time-dependent sensitivity and specificity and the log-transformed HR. Based on the proved asymptotic property of the trivariate model, we introduce a likelihood based sensitivity analysis method based on the conditional likelihood constrained by the expected proportion of published studies. We illustrate the proposed sensitivity analysis method through the meta-analysis of Ki67 for breast cancer. Simulation studies are conducted to evaluate the performance of the proposed method.
4.Forecasting high-dimensional functional time series: Application to sub-national age-specific mortality
Authors:Cristian F. Jiménez-Varón, Ying Sun, Han Lin Shang
Abstract: We consider modeling and forecasting high-dimensional functional time series (HDFTS), which can be cross-sectionally correlated and temporally dependent. We present a novel two-way functional median polish decomposition, which is robust against outliers, to decompose HDFTS into deterministic and time-varying components. A functional time series forecasting method, based on dynamic functional principal component analysis, is implemented to produce forecasts for the time-varying components. By combining the forecasts of the time-varying components with the deterministic components, we obtain forecast curves for multiple populations. Illustrated by the age- and sex-specific mortality rates in the US, France, and Japan, which contain 51 states, 95 departments, and 47 prefectures, respectively, the proposed model delivers more accurate point and interval forecasts in forecasting multi-population mortality than several benchmark methods.
5.Reliability analysis of arbitrary systems based on active learning and global sensitivity analysis
Authors:Maliki Moustapha, Pietro Parisi, Stefano Marelli, Bruno Sudret
Abstract: System reliability analysis aims at computing the probability of failure of an engineering system given a set of uncertain inputs and limit state functions. Active-learning solution schemes have been shown to be a viable tool but as of yet they are not as efficient as in the context of component reliability analysis. This is due to some peculiarities of system problems, such as the presence of multiple failure modes and their uneven contribution to failure, or the dependence on the system configuration (e.g., series or parallel). In this work, we propose a novel active learning strategy designed for solving general system reliability problems. This algorithm combines subset simulation and Kriging/PC-Kriging, and relies on an enrichment scheme tailored to specifically address the weaknesses of this class of methods. More specifically, it relies on three components: (i) a new learning function that does not require the specification of the system configuration, (ii) a density-based clustering technique that allows one to automatically detect the different failure modes, and (iii) sensitivity analysis to estimate the contribution of each limit state to system failure so as to select only the most relevant ones for enrichment. The proposed method is validated on two analytical examples and compared against results gathered in the literature. Finally, a complex engineering problem related to power transmission is solved, thereby showcasing the efficiency of the proposed method in a real-case scenario.