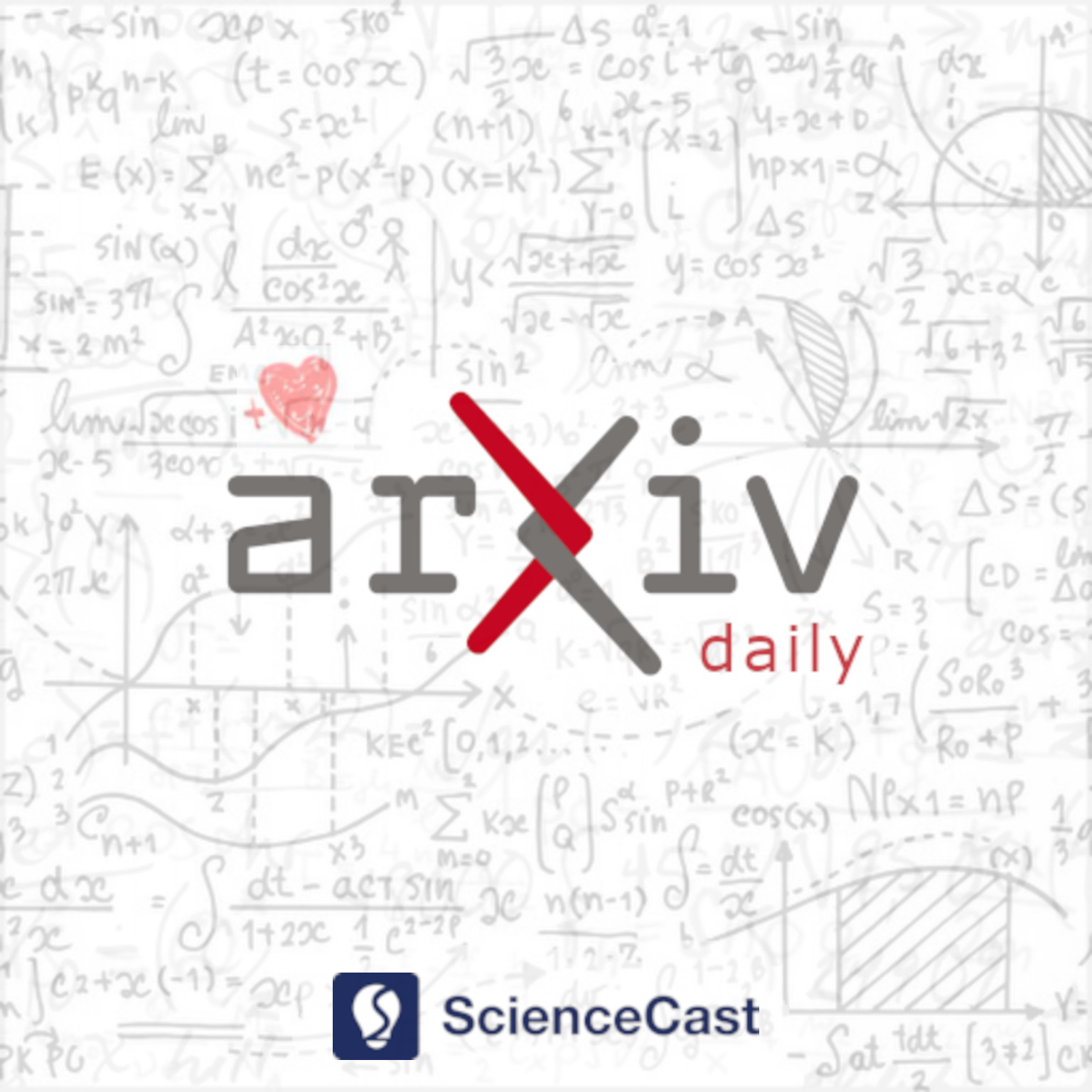
Methodology (stat.ME)
Wed, 19 Jul 2023
1.Communication-Efficient Distribution-Free Inference Over Networks
Authors:Mehrdad Pournaderi, Yu Xiang
Abstract: Consider a star network where each local node possesses a set of distribution-free test statistics that exhibit a symmetric distribution around zero when their corresponding null hypothesis is true. This paper investigates statistical inference problems in networks concerning the aggregation of this general type of statistics and global error rate control under communication constraints in various scenarios. The study proposes communication-efficient algorithms that are built on established non-parametric methods, such as the Wilcoxon and sign tests, as well as modern inference methods such as the Benjamini-Hochberg (BH) and Barber-Candes (BC) procedures, coupled with sampling and quantization operations. The proposed methods are evaluated through extensive simulation studies.
2.Correlation networks, dynamic factor models and community detection
Authors:Shankar Bhamidi, Dhruv Patel, Vladas Pipiras, Guorong Wu
Abstract: A dynamic factor model with a mixture distribution of the loadings is introduced and studied for multivariate, possibly high-dimensional time series. The correlation matrix of the model exhibits a block structure, reminiscent of correlation patterns for many real multivariate time series. A standard $k$-means algorithm on the loadings estimated through principal components is used to cluster component time series into communities with accompanying bounds on the misclustering rate. This is one standard method of community detection applied to correlation matrices viewed as weighted networks. This work puts a mixture model, a dynamic factor model and network community detection in one interconnected framework. Performance of the proposed methodology is illustrated on simulated and real data.
3.Dynamic factor and VARMA models: equivalent representations, dimension reduction and nonlinear matrix equations
Authors:Shankar Bhamidi, Dhruv Patel, Vladas Pipiras
Abstract: A dynamic factor model with factor series following a VAR$(p)$ model is shown to have a VARMA$(p,p)$ model representation. Reduced-rank structures are identified for the VAR and VMA components of the resulting VARMA model. It is also shown how the VMA component parameters can be computed numerically from the original model parameters via the innovations algorithm, and connections of this approach to non-linear matrix equations are made. Some VAR models related to the resulting VARMA model are also discussed.
4.Entropy regularization in probabilistic clustering
Authors:Beatrice Franzolini, Giovanni Rebaudo
Abstract: Bayesian nonparametric mixture models are widely used to cluster observations. However, one major drawback of the approach is that the estimated partition often presents unbalanced clusters' frequencies with only a few dominating clusters and a large number of sparsely-populated ones. This feature translates into results that are often uninterpretable unless we accept to ignore a relevant number of observations and clusters. Interpreting the posterior distribution as penalized likelihood, we show how the unbalance can be explained as a direct consequence of the cost functions involved in estimating the partition. In light of our findings, we propose a novel Bayesian estimator of the clustering configuration. The proposed estimator is equivalent to a post-processing procedure that reduces the number of sparsely-populated clusters and enhances interpretability. The procedure takes the form of entropy-regularization of the Bayesian estimate. While being computationally convenient with respect to alternative strategies, it is also theoretically justified as a correction to the Bayesian loss function used for point estimation and, as such, can be applied to any posterior distribution of clusters, regardless of the specific model used.