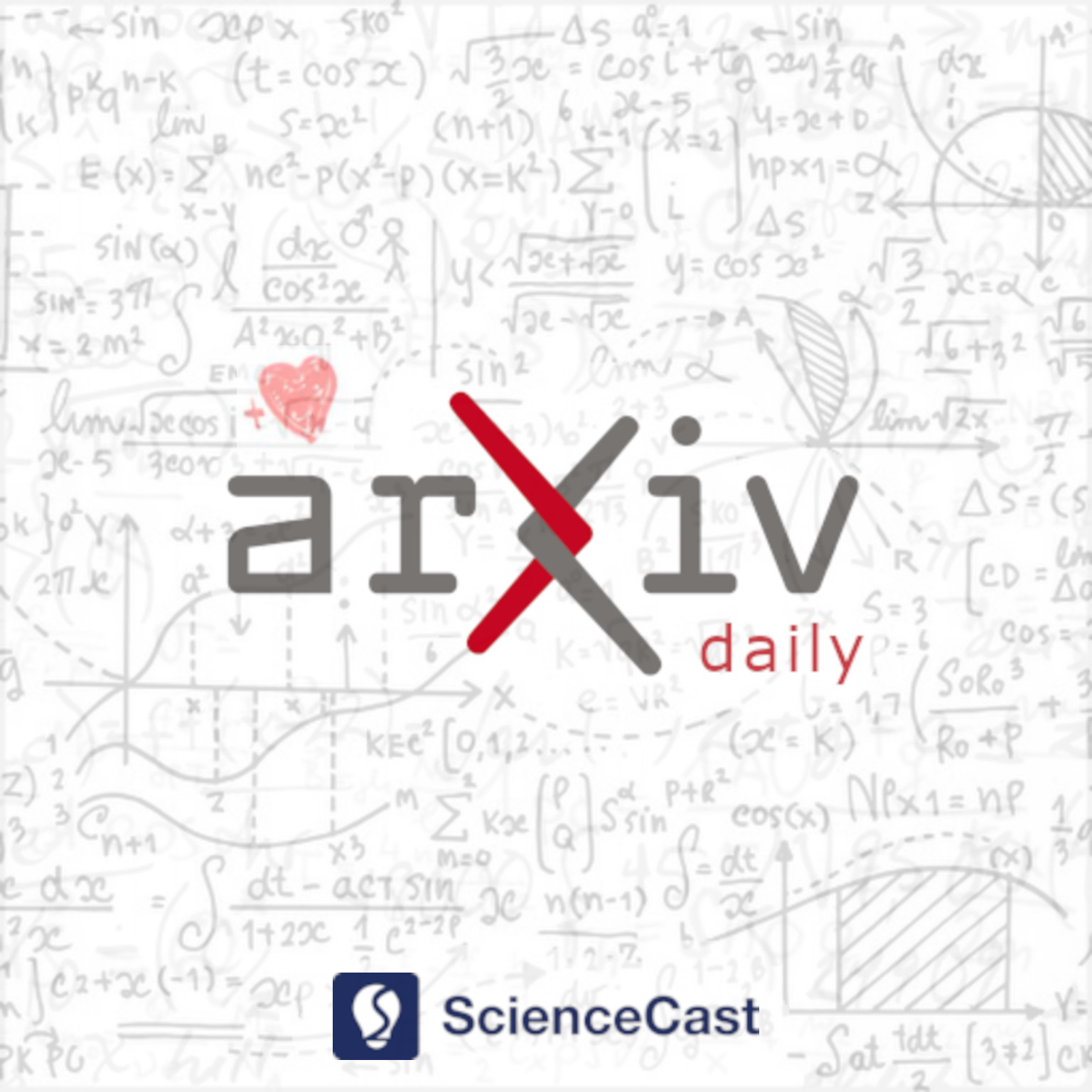
Methodology (stat.ME)
Tue, 20 Jun 2023
1.A Two-Stage Bayesian Small Area Estimation Method for Proportions
Authors:James Hogg, Jessica Cameron, Susanna Cramb, Peter Baade, Kerrie Mengersen
Abstract: With the rise in popularity of digital Atlases to communicate spatial variation, there is an increasing need for robust small-area estimates. However, current small-area estimation methods suffer from various modelling problems when data are very sparse or when estimates are required for areas with very small populations. These issues are particularly heightened when modelling proportions. Additionally, recent work has shown significant benefits in modelling at both the individual and area levels. We propose a two-stage Bayesian hierarchical small area estimation model for proportions that can: account for survey design; use both individual-level survey-only covariates and area-level census covariates; reduce direct estimate instability; and generate prevalence estimates for small areas with no survey data. Using a simulation study we show that, compared with existing Bayesian small area estimation methods, our model can provide optimal predictive performance (Bayesian mean relative root mean squared error, mean absolute relative bias and coverage) of proportions under a variety of data conditions, including very sparse and unstable data. To assess the model in practice, we compare modeled estimates of current smoking prevalence for 1,630 small areas in Australia using the 2017-2018 National Health Survey data combined with 2016 census data.
2.Empirical prior distributions for Bayesian meta-analyses of binary and time to event outcomes
Authors:František Bartoš, Willem M. Otte, Quentin F. Gronau, Bram Timmers, Alexander Ly, Eric-Jan Wagenmakers
Abstract: Bayesian model-averaged meta-analysis allows quantification of evidence for both treatment effectiveness $\mu$ and across-study heterogeneity $\tau$. We use the Cochrane Database of Systematic Reviews to develop discipline-wide empirical prior distributions for $\mu$ and $\tau$ for meta-analyses of binary and time-to-event clinical trial outcomes. First, we use 50% of the database to estimate parameters of different required parametric families. Second, we use the remaining 50% of the database to select the best-performing parametric families and explore essential assumptions about the presence or absence of the treatment effectiveness and across-study heterogeneity in real data. We find that most meta-analyses of binary outcomes are more consistent with the absence of the meta-analytic effect or heterogeneity while meta-analyses of time-to-event outcomes are more consistent with the presence of the meta-analytic effect or heterogeneity. Finally, we use the complete database - with close to half a million trial outcomes - to propose specific empirical prior distributions, both for the field in general and for specific medical subdisciplines. An example from acute respiratory infections demonstrates how the proposed prior distributions can be used to conduct a Bayesian model-averaged meta-analysis in the open-source software R and JASP.
3.Conditional Independence Testing with Heteroskedastic Data and Applications to Causal Discovery
Authors:Wiebke Günther, Urmi Ninad, jonas Wahl, Jakob Runge
Abstract: Conditional independence (CI) testing is frequently used in data analysis and machine learning for various scientific fields and it forms the basis of constraint-based causal discovery. Oftentimes, CI testing relies on strong, rather unrealistic assumptions. One of these assumptions is homoskedasticity, in other words, a constant conditional variance is assumed. We frame heteroskedasticity in a structural causal model framework and present an adaptation of the partial correlation CI test that works well in the presence of heteroskedastic noise, given that expert knowledge about the heteroskedastic relationships is available. Further, we provide theoretical consistency results for the proposed CI test which carry over to causal discovery under certain assumptions. Numerical causal discovery experiments demonstrate that the adapted partial correlation CI test outperforms the standard test in the presence of heteroskedasticity and is on par for the homoskedastic case. Finally, we discuss the general challenges and limits as to how expert knowledge about heteroskedasticity can be accounted for in causal discovery.
4.Individual Treatment Effects in Extreme Regimes
Authors:Ahmed Aloui, Ali Hasan, Yuting Ng, Miroslav Pajic, Vahid Tarokh
Abstract: Understanding individual treatment effects in extreme regimes is important for characterizing risks associated with different interventions. This is hindered by the fact that extreme regime data may be hard to collect, as it is scarcely observed in practice. In addressing this issue, we propose a new framework for estimating the individual treatment effect in extreme regimes (ITE$_2$). Specifically, we quantify this effect by the changes in the tail decay rates of potential outcomes in the presence or absence of the treatment. Subsequently, we establish conditions under which ITE$_2$ may be calculated and develop algorithms for its computation. We demonstrate the efficacy of our proposed method on various synthetic and semi-synthetic datasets.
5.Should I Stop or Should I Go: Early Stopping with Heterogeneous Populations
Authors:Hammaad Adam, Fan Yin, Mary Hu, Neil Tenenholtz, Lorin Crawford, Lester Mackey, Allison Koenecke
Abstract: Randomized experiments often need to be stopped prematurely due to the treatment having an unintended harmful effect. Existing methods that determine when to stop an experiment early are typically applied to the data in aggregate and do not account for treatment effect heterogeneity. In this paper, we study the early stopping of experiments for harm on heterogeneous populations. We first establish that current methods often fail to stop experiments when the treatment harms a minority group of participants. We then use causal machine learning to develop CLASH, the first broadly-applicable method for heterogeneous early stopping. We demonstrate CLASH's performance on simulated and real data and show that it yields effective early stopping for both clinical trials and A/B tests.
6.The Bayesian Regularized Quantile Varying Coefficient Model
Authors:Fei Zhou, Jie Ren, Shuangge Ma, Cen Wu
Abstract: The quantile varying coefficient (VC) model can flexibly capture dynamical patterns of regression coefficients. In addition, due to the quantile check loss function, it is robust against outliers and heavy-tailed distributions of the response variable, and can provide a more comprehensive picture of modeling via exploring the conditional quantiles of the response variable. Although extensive studies have been conducted to examine variable selection for the high-dimensional quantile varying coefficient models, the Bayesian analysis has been rarely developed. The Bayesian regularized quantile varying coefficient model has been proposed to incorporate robustness against data heterogeneity while accommodating the non-linear interactions between the effect modifier and predictors. Selecting important varying coefficients can be achieved through Bayesian variable selection. Incorporating the multivariate spike-and-slab priors further improves performance by inducing exact sparsity. The Gibbs sampler has been derived to conduct efficient posterior inference of the sparse Bayesian quantile VC model through Markov chain Monte Carlo (MCMC). The merit of the proposed model in selection and estimation accuracy over the alternatives has been systematically investigated in simulation under specific quantile levels and multiple heavy-tailed model errors. In the case study, the proposed model leads to identification of biologically sensible markers in a non-linear gene-environment interaction study using the NHS data.
7.XRRmeta: Exact Inference for Random-Effects Meta-Analyses with Rare Events
Authors:Jessica Gronsbell, Zachary R McCaw, Timothy Regis, Lu Tian
Abstract: Meta-analysis aggregates information across related studies to provide more reliable statistical inference and has been a vital tool for assessing the safety and efficacy of many high profile pharmaceutical products. A key challenge in conducting a meta-analysis is that the number of related studies is typically small. Applying classical methods that are asymptotic in the number of studies can compromise the validity of inference, particularly when heterogeneity across studies is present. Moreover, serious adverse events are often rare and can result in one or more studies with no events in at least one study arm. While it is common to use arbitrary continuity corrections or remove zero-event studies to stabilize or define effect estimates in such settings, these practices can invalidate subsequent inference. To address these significant practical issues, we introduce an exact inference method for comparing event rates in two treatment arms under a random effects framework, which we coin "XRRmeta". In contrast to existing methods, the coverage of the confidence interval from XRRmeta is guaranteed to be at or above the nominal level (up to Monte Carlo error) when the event rates, number of studies, and/or the within-study sample sizes are small. XRRmeta is also justified in its treatment of zero-event studies through a conditional inference argument. Importantly, our extensive numerical studies indicate that XRRmeta does not yield overly conservative inference. We apply our proposed method to reanalyze the occurrence of major adverse cardiovascular events among type II diabetics treated with rosiglitazone and in a more recent example examining the utility of face masks in preventing person-to-person transmission of severe acute respiratory syndrome coronavirus 2 (SARS-CoV-2) and coronavirus disease 2019 (COVID-19).