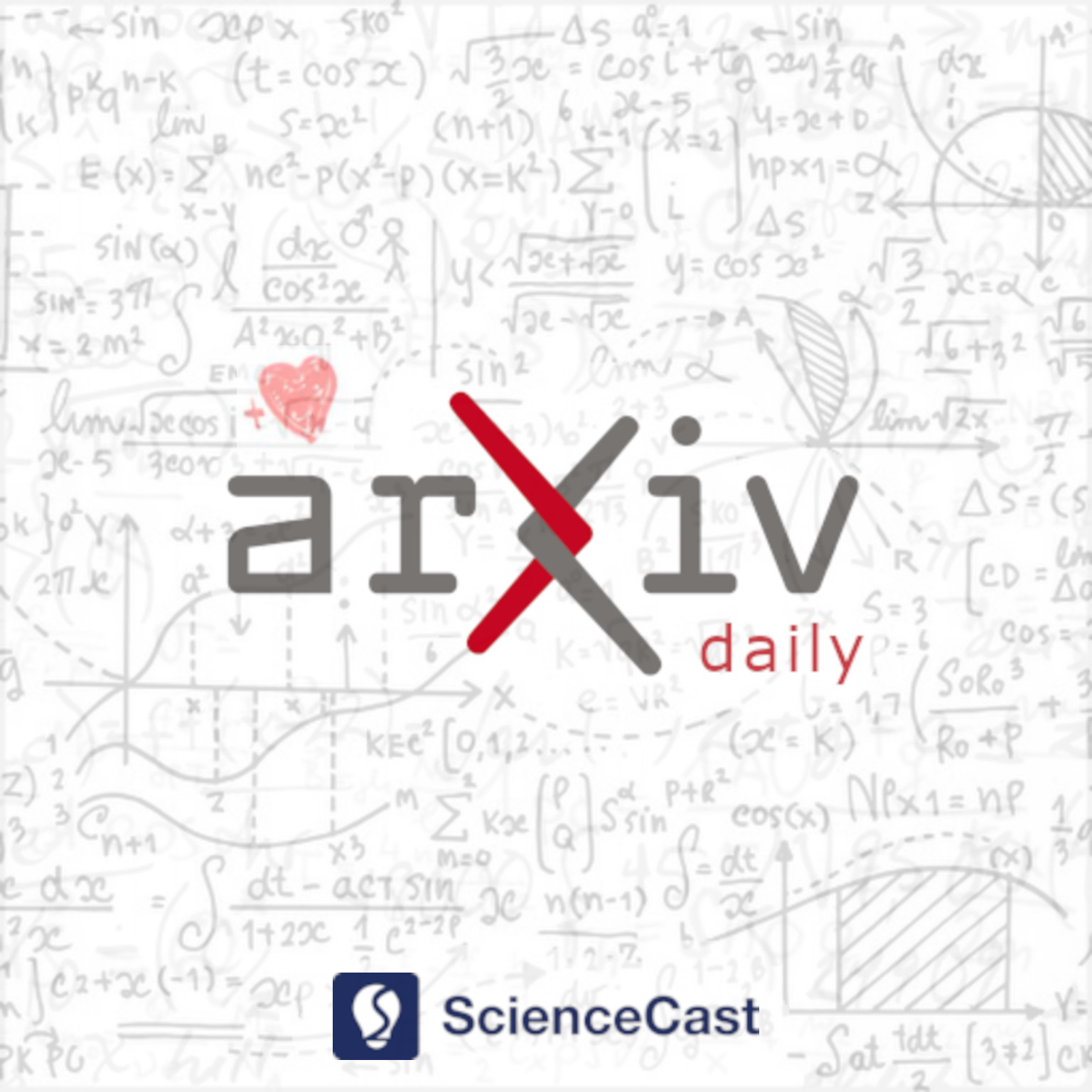
Methodology (stat.ME)
Mon, 24 Apr 2023
1.Faster estimation of the Knorr-Held Type IV space-time model
Authors:Fredrik Lohne Aanes, Geir Storvik
Abstract: In this paper we study the type IV Knorr Held space time models. Such models typically apply intrinsic Markov random fields and constraints are imposed for identifiability. INLA is an efficient inference tool for such models where constraints are dealt with through a conditioning by kriging approach. When the number of spatial and/or temporal time points become large, it becomes computationally expensive to fit such models, partly due to the number of constraints involved. We propose a new approach, HyMiK, dividing constraints into two separate sets where one part is treated through a mixed effect approach while the other one is approached by the standard conditioning by kriging method, resulting in a more efficient procedure for dealing with constraints. The new approach is easy to apply based on existing implementations of INLA. We run the model on simulated data, on a real data set containing dengue fever cases in Brazil and another real data set of confirmed positive test cases of Covid-19 in the counties of Norway. For all cases we get very similar results when comparing the new approach with the tradition one while at the same time obtaining a significant increase in computational speed, varying on a factor from 3 to 23, depending on the sizes of the data sets.
2.High-dimensional iterative variable selection for accelerated failure time models
Authors:Nilotpal Sanyal
Abstract: We propose an iterative variable selection method for the accelerated failure time model using high-dimensional survival data. Our method pioneers the use of the recently proposed structured screen-and-select framework for survival analysis. We use the marginal utility as the measure of association to inform the structured screening process. For the selection steps, we use Bayesian model selection based on non-local priors. We compare the proposed method with a few well-known methods. Assessment in terms of true positive rate and false discovery rate shows the usefulness of our method. We have implemented the method within the R package GWASinlps.
3.A Cheat Sheet for Bayesian Prediction
Authors:Bertrand Clarke, Yuling Yao
Abstract: This paper reviews the growing field of Bayesian prediction. Bayes point and interval prediction are defined and exemplified and situated in statistical prediction more generally. Then, four general approaches to Bayes prediction are defined and we turn to predictor selection. This can be done predictively or non-predictively and predictors can be based on single models or multiple models. We call these latter cases unitary predictors and model average predictors, respectively. Then we turn to the most recent aspect of prediction to emerge, namely prediction in the context of large observational data sets and discuss three further classes of techniques. We conclude with a summary and statement of several current open problems.