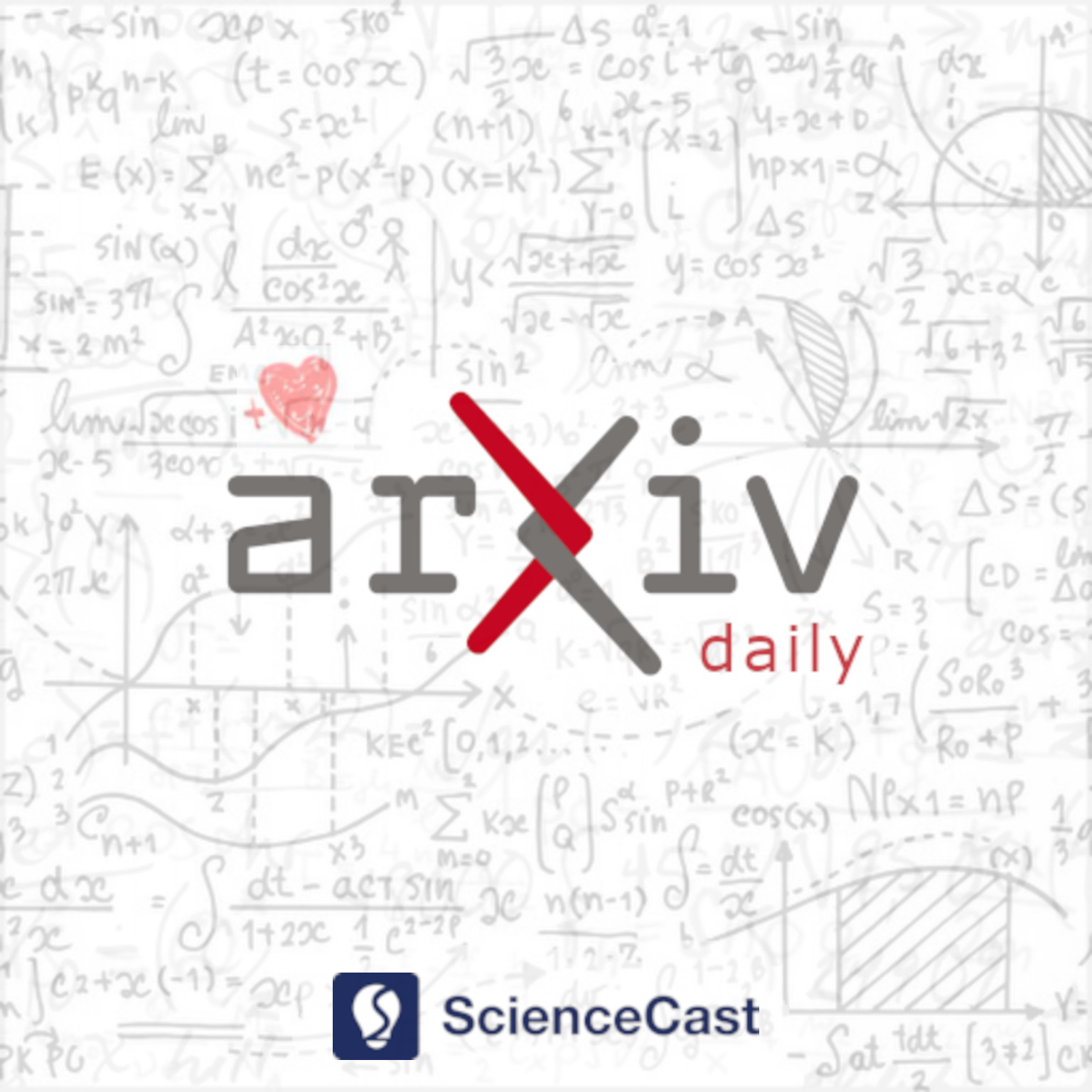
Methodology (stat.ME)
Tue, 09 May 2023
1.High-dimensional Feature Screening for Nonlinear Associations With Survival Outcome Using Restricted Mean Survival Time
Authors:Yaxian Chen, KF Lam, Zhonghua Liu
Abstract: Feature screening is an important tool in analyzing ultrahigh-dimensional data, particularly in the field of Omics and oncology studies. However, most attention has been focused on identifying features that have a linear or monotonic impact on the response variable. Detecting a sparse set of variables that have a nonlinear or non-monotonic relationship with the response variable is still a challenging task. To fill the gap, this paper proposed a robust model-free screening approach for right-censored survival data by providing a new perspective of quantifying the covariate effect on the restricted mean survival time, rather than the routinely used hazard function. The proposed measure, based on the difference between the restricted mean survival time of covariate-stratified and overall data, is able to identify comprehensive types of associations including linear, nonlinear, non-monotone, and even local dependencies like change points. This approach is highly interpretable and flexible without any distribution assumption. The sure screening property is established and an iterative screening procedure is developed to address multicollinearity between high-dimensional covariates. Simulation studies are carried out to demonstrate the superiority of the proposed method in selecting important features with a complex association with the response variable. The potential of applying the proposed method to handle interval-censored failure time data has also been explored in simulations, and the results have been promising. The method is applied to a breast cancer dataset to identify potential prognostic factors, which reveals potential associations between breast cancer and lymphoma.
2.Causal Discovery with Unobserved Variables: A Proxy Variable Approach
Authors:Mingzhou Liu, Xinwei Sun, Yu Qiao, Yizhou Wang
Abstract: Discovering causal relations from observational data is important. The existence of unobserved variables (e.g. latent confounding or mediation) can mislead the causal identification. To overcome this problem, proximal causal discovery methods attempted to adjust for the bias via the proxy of the unobserved variable. Particularly, hypothesis test-based methods proposed to identify the causal edge by testing the induced violation of linearity. However, these methods only apply to discrete data with strict level constraints, which limits their practice in the real world. In this paper, we fix this problem by extending the proximal hypothesis test to cases where the system consists of continuous variables. Our strategy is to present regularity conditions on the conditional distributions of the observed variables given the hidden factor, such that if we discretize its observed proxy with sufficiently fine, finite bins, the involved discretization error can be effectively controlled. Based on this, we can convert the problem of testing continuous causal relations to that of testing discrete causal relations in each bin, which can be effectively solved with existing methods. These non-parametric regularities we present are mild and can be satisfied by a wide range of structural causal models. Using both simulated and real-world data, we show the effectiveness of our method in recovering causal relations when unobserved variables exist.
3.Testing exchangeability in the batch mode with e-values and Markov alternatives
Authors:Vladimir Vovk
Abstract: The topic of this paper is testing exchangeability using e-values in the batch mode, with the Markov model as alternative. The null hypothesis of exchangeability is formalized as a Kolmogorov-type compression model, and the Bayes mixture of the Markov model w.r. to the uniform prior is taken as simple alternative hypothesis. Using e-values instead of p-values leads to a computationally efficient testing procedure. In the appendixes I explain connections with the algorithmic theory of randomness and with the traditional theory of testing statistical hypotheses. In the standard statistical terminology, this paper proposes a new permutation test. This test can also be interpreted as a poor man's version of Kolmogorov's deficiency of randomness.
4.Bayes Linear Analysis for Statistical Modelling with Uncertain Inputs
Authors:Samuel E. Jackson, David C. Woods
Abstract: Statistical models typically capture uncertainties in our knowledge of the corresponding real-world processes, however, it is less common for this uncertainty specification to capture uncertainty surrounding the values of the inputs to the model, which are often assumed known. We develop general modelling methodology with uncertain inputs in the context of the Bayes linear paradigm, which involves adjustment of second-order belief specifications over all quantities of interest only, without the requirement for probabilistic specifications. In particular, we propose an extension of commonly-employed second-order modelling assumptions to the case of uncertain inputs, with explicit implementation in the context of regression analysis, stochastic process modelling, and statistical emulation. We apply the methodology to a regression model for extracting aluminium by electrolysis, and emulation of the motivating epidemiological simulator chain to model the impact of an airborne infectious disease.
5.Point and probabilistic forecast reconciliation for general linearly constrained multiple time series
Authors:Daniele Girolimetto, Tommaso Di Fonzo
Abstract: Forecast reconciliation is the post-forecasting process aimed to revise a set of incoherent base forecasts into coherent forecasts in line with given data structures. Most of the point and probabilistic regression-based forecast reconciliation results ground on the so called "structural representation" and on the related unconstrained generalized least squares reconciliation formula. However, the structural representation naturally applies to genuine hierarchical/grouped time series, where the top- and bottom-level variables are uniquely identified. When a general linearly constrained multiple time series is considered, the forecast reconciliation is naturally expressed according to a projection approach. While it is well known that the classic structural reconciliation formula is equivalent to its projection approach counterpart, so far it is not completely understood if and how a structural-like reconciliation formula may be derived for a general linearly constrained multiple time series. Such an expression would permit to extend reconciliation definitions, theorems and results in a straightforward manner. In this paper, we show that for general linearly constrained multiple time series it is possible to express the reconciliation formula according to a "structural-like" approach that keeps distinct free and constrained, instead of bottom and upper (aggregated), variables, establish the probabilistic forecast reconciliation framework, and apply these findings to obtain fully reconciled point and probabilistic forecasts for the aggregates of the Australian GDP from income and expenditure sides, and for the European Area GDP disaggregated by income, expenditure and output sides and by 19 countries.
6.Variational Bayesian Inference for Bipartite Mixed-membership Stochastic Block Model with Applications to Collaborative Filtering
Authors:Jie Liu, Zifeng Ye, Kun Chen, Panpan Zhang
Abstract: Motivated by the connections between collaborative filtering and network clustering, we consider a network-based approach to improving rating prediction in recommender systems. We propose a novel Bipartite Mixed-Membership Stochastic Block Model ($\mathrm{BM}^2$) with a conjugate prior from the exponential family. We derive the analytical expression of the model and introduce a variational Bayesian expectation-maximization algorithm, which is computationally feasible for approximating the untractable posterior distribution. We carry out extensive simulations to show that $\mathrm{BM}^2$ provides more accurate inference than standard SBM with the emergence of outliers. Finally, we apply the proposed model to a MovieLens dataset and find that it outperforms other competing methods for collaborative filtering.
7.Spatially smoothed robust covariance estimation for local outlier detection
Authors:Patricia Puchhammer, Peter Filzmoser
Abstract: Most multivariate outlier detection procedures ignore the spatial dependency of observations, which is present in many real data sets from various application areas. This paper introduces a new outlier detection method that accounts for a (continuously) varying covariance structure, depending on the spatial neighborhood of the observations. The underlying estimator thus constitutes a compromise between a unified global covariance estimation, and local covariances estimated for individual neighborhoods. Theoretical properties of the estimator are presented, in particular related to robustness properties, and an efficient algorithm for its computation is introduced. The performance of the method is evaluated and compared based on simulated data and for a data set recorded from Austrian weather stations.
8.Inference for heavy-tailed data with Gaussian dependence
Authors:Bikramjit Das
Abstract: We consider a model for multivariate data with heavy-tailed marginals and a Gaussian dependence structure. The marginal distributions are allowed to have non-homogeneous tail behavior which is in contrast to most popular modeling paradigms for multivariate heavy-tails. Estimation and analysis in such models have been limited due to the so-called asymptotic tail independence property of Gaussian copula rendering probabilities of many relevant extreme sets negligible. In this paper we obtain precise asymptotic expressions for these erstwhile negligible probabilities. We also provide consistent estimates of the marginal tail indices and the Gaussian correlation parameters, and, establish their joint asymptotic normality. The efficacy of our estimation methods are exhibited using extensive simulations as well as real data sets from online networks, insurance claims, and internet traffic.
9.On near-redundancy and identifiability of parametric hazard regression models under censoring
Authors:F. J. Rubio, J. A. Espindola, J. A. Montoya
Abstract: We study parametric inference on a rich class of hazard regression models in the presence of right-censoring. Previous literature has reported some inferential challenges, such as multimodal or flat likelihood surfaces, in this class of models for some particular data sets. We formalize the study of these inferential problems by linking them to the concepts of near-redundancy and practical non-identifiability of parameters. We show that the maximum likelihood estimators of the parameters in this class of models are consistent and asymptotically normal. Thus, the inferential problems in this class of models are related to the finite-sample scenario, where it is difficult to distinguish between the fitted model and a nested non-identifiable (i.e., parameter-redundant) model. We propose a method for detecting near-redundancy, based on distances between probability distributions. We also employ methods used in other areas for detecting practical non-identifiability and near-redundancy, including the inspection of the profile likelihood function and the Hessian method. For cases where inferential problems are detected, we discuss alternatives such as using model selection tools to identify simpler models that do not exhibit these inferential problems, increasing the sample size, or extending the follow-up time. We illustrate the performance of the proposed methods through a simulation study. Our simulation study reveals a link between the presence of near-redundancy and practical non-identifiability. Two illustrative applications using real data, with and without inferential problems, are presented.