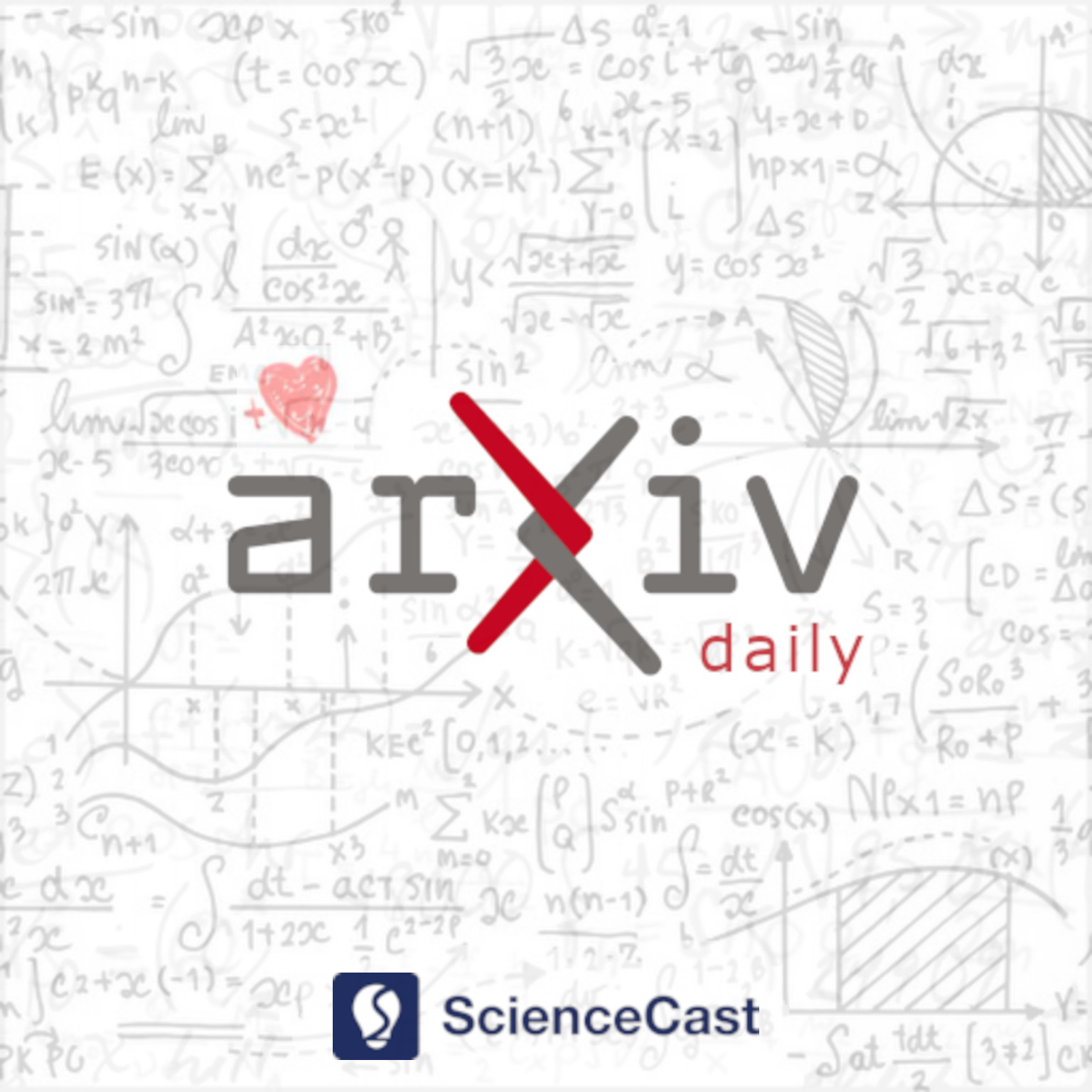
Methodology (stat.ME)
Mon, 10 Apr 2023
1.On new omnibus tests of uniformity on the hypersphere
Authors:Alberto Fernández-de-Marcos, Eduardo García-Portugués
Abstract: Two new omnibus tests of uniformity for data on the hypersphere are proposed. The new test statistics leverage closed-form expressions for orthogonal polynomials, feature tuning parameters, and are related to a "smooth maximum" function and the Poisson kernel. We obtain exact moments of the test statistics under uniformity and rotationally symmetric alternatives, and give their null asymptotic distributions. We consider approximate oracle tuning parameters that maximize the power of the tests against generic alternatives and provide tests that estimate oracle parameters through cross-validated procedures while maintaining the significance level. Numerical experiments explore the effectiveness of null asymptotic distributions and the accuracy of inexpensive approximations of exact null distributions. A simulation study compares the powers of the new tests with other tests of the Sobolev class, showing the benefits of the former. The proposed tests are applied to the study of the (seemingly uniform) nursing times of wild polar bears.
2.Non-asymptotic inference for multivariate change point detection
Authors:Ian Barnett
Abstract: Traditional methods for inference in change point detection often rely on a large number of observed data points and can be inaccurate in non-asymptotic settings. With the rise of mobile health and digital phenotyping studies, where patients are monitored through the use of smartphones or other digital devices, change point detection is needed in non-asymptotic settings where it may be important to identify behavioral changes that occur just days before an adverse event such as relapse or suicide. Furthermore, analytical and computationally efficient means of inference are necessary for the monitoring and online analysis of large-scale digital phenotyping cohorts. We extend the result for asymptotic tail probabilities of the likelihood ratio test to the multivariate change point detection setting, and demonstrate through simulation its inaccuracy when the number of observed data points is not large. We propose a non-asymptotic approach for inference on the likelihood ratio test, and compare the efficiency of this estimated p-value to the popular empirical p-value obtained through simulation of the null distribution. The accuracy and power of this approach relative to competing methods is demonstrated through simulation and through the detection of a change point in the behavior of a patient with schizophrenia in the week prior to relapse.
3.A Framework for Understanding Selection Bias in Real-World Healthcare Data
Authors:Ritoban Kundu, Xu Shi, Jean Morrison, Bhramar Mukherjee
Abstract: Using administrative patient-care data such as Electronic Health Records and medical/pharmaceutical claims for population-based scientific research has become increasingly common. With vast sample sizes leading to very small standard errors, researchers need to pay more attention to potential biases in the estimates of association parameters of interest, specifically to biases that do not diminish with increasing sample size. Of these multiple sources of biases, in this paper, we focus on understanding selection bias. We present an analytic framework using directed acyclic graphs for guiding applied researchers to dissect how different sources of selection bias may affect their parameter estimates of interest. We review four easy-to-implement weighting approaches to reduce selection bias and explain through a simulation study when they can rescue us in practice with analysis of real world data. We provide annotated R codes to implement these methods.
4.Scalable Randomized Kernel Methods for Multiview Data Integration and Prediction
Authors:Sandra E. Safo, Han Lu
Abstract: We develop scalable randomized kernel methods for jointly associating data from multiple sources and simultaneously predicting an outcome or classifying a unit into one of two or more classes. The proposed methods model nonlinear relationships in multiview data together with predicting a clinical outcome and are capable of identifying variables or groups of variables that best contribute to the relationships among the views. We use the idea that random Fourier bases can approximate shift-invariant kernel functions to construct nonlinear mappings of each view and we use these mappings and the outcome variable to learn view-independent low-dimensional representations. Through simulation studies, we show that the proposed methods outperform several other linear and nonlinear methods for multiview data integration. When the proposed methods were applied to gene expression, metabolomics, proteomics, and lipidomics data pertaining to COVID-19, we identified several molecular signatures forCOVID-19 status and severity. Results from our real data application and simulations with small sample sizes suggest that the proposed methods may be useful for small sample size problems. Availability: Our algorithms are implemented in Pytorch and interfaced in R and would be made available at: https://github.com/lasandrall/RandMVLearn.
5.Testing for linearity in scalar-on-function regression with responses missing at random
Authors:Manuel Febrero-Bande, Pedro Galeano, Eduardo García-Portugués, Wenceslao González-Manteiga
Abstract: We construct a goodness-of-fit test for the Functional Linear Model with Scalar Response (FLMSR) with responses Missing At Random (MAR). For that, we extend an existing testing procedure for the case where all responses have been observed to the case where the responses are MAR. The testing procedure gives rise to a statistic based on a marked empirical process indexed by the randomly projected functional covariate. The test statistic depends on a suitable estimator of the functional slope of the FLMSR when the sample has MAR responses, so several estimators are proposed and compared. With any of them, the test statistic is relatively easy to compute and its distribution under the null hypothesis is simple to calibrate based on a wild bootstrap procedure. The behavior of the resulting testing procedure as a function of the estimators of the functional slope of the FLMSR is illustrated by means of several Monte Carlo experiments. Additionally, the testing procedure is applied to a real data set to check whether the linear hypothesis holds.
6.Causal mediation analysis with a failure time outcome in the presence of exposure measurement error
Authors:Chao Cheng, Donna Spiegelman, Fan Li
Abstract: Causal mediation analysis is widely used in health science research to evaluate the extent to which an intermediate variable explains an observed exposure-outcome relationship. However, the validity of analysis can be compromised when the exposure is measured with error, which is common in health science studies. This article investigates the impact of exposure measurement error on assessing mediation with a failure time outcome, where a Cox proportional hazard model is considered for the outcome. When the outcome is rare with no exposure-mediator interaction, we show that the unadjusted estimators of the natural indirect and direct effects can be biased into either direction, but the unadjusted estimator of the mediation proportion is approximately unbiased as long as measurement error is not large or the mediator-exposure association is not strong. We propose ordinary regression calibration and risk set regression calibration approaches to correct the exposure measurement error-induced bias in estimating mediation effects and to allow for an exposure-mediator interaction in the Cox outcome model. The proposed approaches require a validation study to characterize the measurement error process between the true exposure and its error-prone counterpart. We apply the proposed approaches to the Health Professionals Follow-up study to evaluate extent to which body mass index mediates the effect of vigorous physical activity on the risk of cardiovascular diseases, and assess the finite-sample properties of the proposed estimators via simulations.