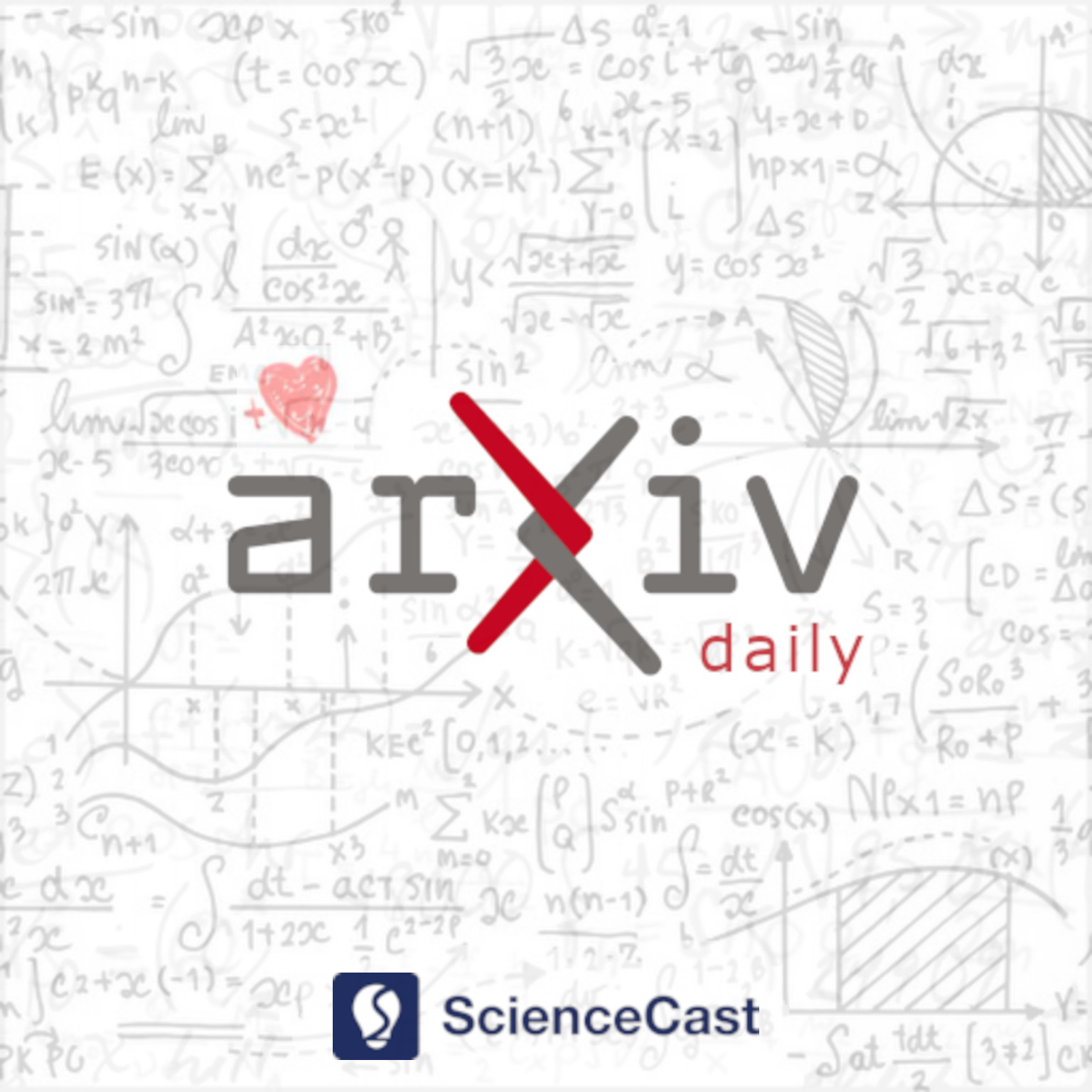
Methodology (stat.ME)
Fri, 14 Jul 2023
1.Two-Sample Test with Copula Entropy
Authors:Jian Ma
Abstract: In this paper we propose a two-sample test based on copula entropy (CE). The proposed test statistic is defined as the difference between the CEs of the null hypothesis and the alternative. The estimator of the test statistic is proposed with the non-parametric estimator of CE, which is non-parametric and hyperparameter-free. Simulation experiments demonstrate the effectiveness of the proposed test with the simulated bi-variate normal data.
2.Bounded-memory adjusted scores estimation in generalized linear models with large data sets
Authors:Patrick Zietkiewicz, Ioannis Kosmidis
Abstract: The widespread use of maximum Jeffreys'-prior penalized likelihood in binomial-response generalized linear models, and in logistic regression, in particular, are supported by the results of Kosmidis and Firth (2021, Biometrika), who show that the resulting estimates are also always finite-valued, even in cases where the maximum likelihood estimates are not, which is a practical issue regardless of the size of the data set. In logistic regression, the implied adjusted score equations are formally bias-reducing in asymptotic frameworks with a fixed number of parameters and appear to deliver a substantial reduction in the persistent bias of the maximum likelihood estimator in high-dimensional settings where the number of parameters grows asymptotically linearly and slower than the number of observations. In this work, we develop and present two new variants of iteratively reweighted least squares for estimating generalized linear models with adjusted score equations for mean bias reduction and maximization of the likelihood penalized by a positive power of the Jeffreys-prior penalty, which eliminate the requirement of storing $O(n)$ quantities in memory, and can operate with data sets that exceed computer memory or even hard drive capacity. We achieve that through incremental QR decompositions, which enable IWLS iterations to have access only to data chunks of predetermined size. We assess the procedures through a real-data application with millions of observations, and in high-dimensional logistic regression, where a large-scale simulation experiment produces concrete evidence for the existence of a simple adjustment to the maximum Jeffreys'-penalized likelihood estimates that delivers high accuracy in terms of signal recovery even in cases where estimates from ML and other recently-proposed corrective methods do not exist.
3.Sensitivity Analysis for Unmeasured Confounding in Medical Product Development and Evaluation Using Real World Evidence
Authors:Peng Ding, Yixin Fang, Doug Faries, Susan Gruber, Hana Lee, Joo-Yeon Lee, Pallavi Mishra-Kalyani, Mingyang Shan, Mark van der Laan, Shu Yang, Xiang Zhang
Abstract: The American Statistical Association Biopharmaceutical Section (ASA BIOP) working group on real-world evidence (RWE) has been making continuous, extended effort towards a goal of supporting and advancing regulatory science with respect to non-interventional, clinical studies intended to use real-world data for evidence generation for the purpose of medical product development and evaluation (i.e., RWE studies). In 2023, the working group published a manuscript delineating challenges and opportunities in constructing estimands for RWE studies following a framework in ICH E9(R1) guidance on estimand and sensitivity analysis. As a follow-up task, we describe the other issue in RWE studies, sensitivity analysis. Focusing on the issue of unmeasured confounding, we review availability and applicability of sensitivity analysis methods for different types unmeasured confounding. We discuss consideration on the choice and use of sensitivity analysis for RWE studies. Updated version of this article will present how findings from sensitivity analysis could support regulatory decision-making using a real example.