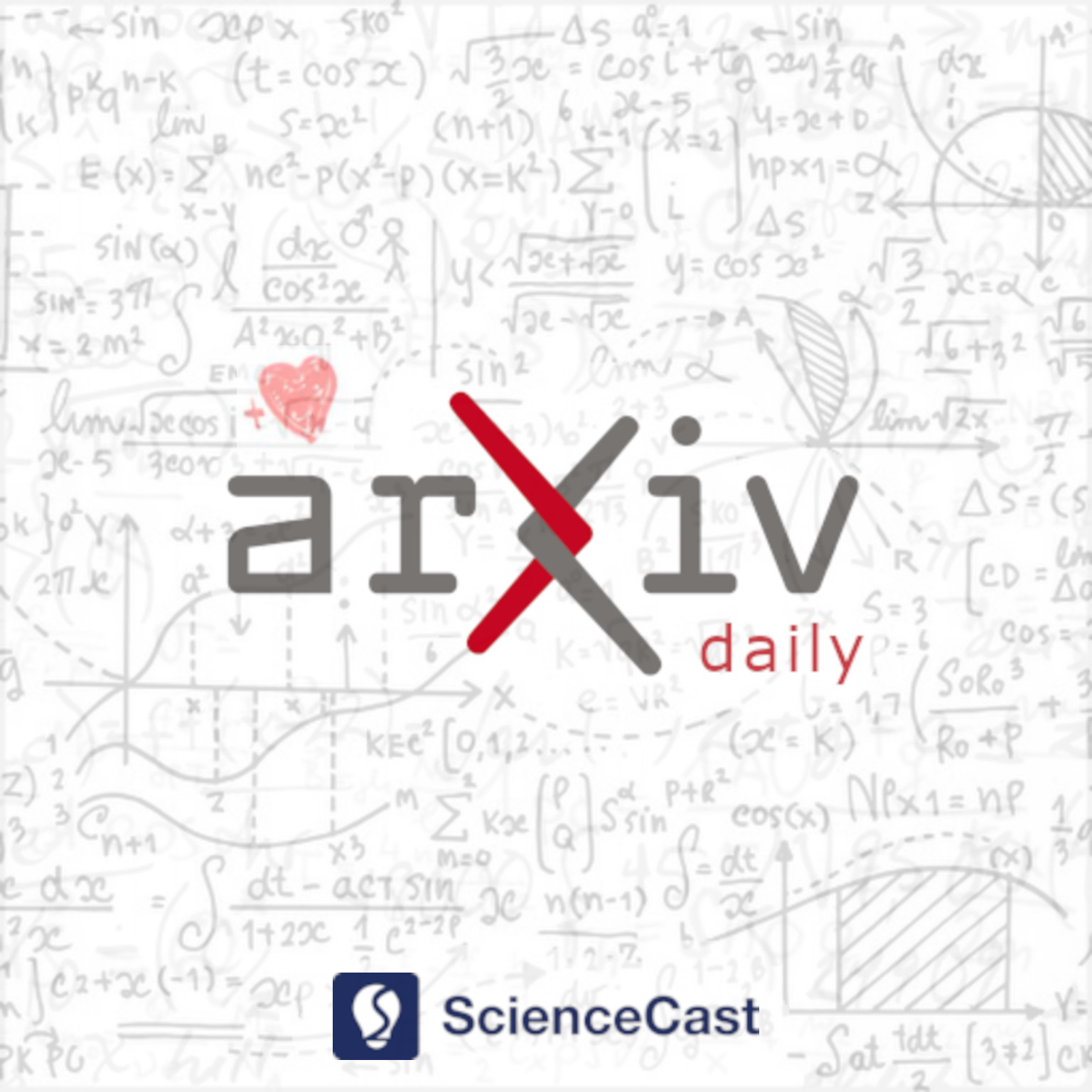
Methodology (stat.ME)
Wed, 03 May 2023
1.A Bayesian approach to identify changepoints in spatio-temporal ordered categorical data: An application to COVID-19 data
Authors:Siddharth Rawat, Abe Durrant, Adam Simpson, Grant Nielson, Candace Berrett, Soudeep Deb
Abstract: Although there is substantial literature on identifying structural changes for continuous spatio-temporal processes, the same is not true for categorical spatio-temporal data. This work bridges that gap and proposes a novel spatio-temporal model to identify changepoints in ordered categorical data. The model leverages an additive mean structure with separable Gaussian space-time processes for the latent variable. Our proposed methodology can detect significant changes in the mean structure as well as in the spatio-temporal covariance structures. We implement the model through a Bayesian framework that gives a computational edge over conventional approaches. From an application perspective, our approach's capability to handle ordinal categorical data provides an added advantage in real applications. This is illustrated using county-wise COVID-19 data (converted to categories according to CDC guidelines) from the state of New York in the USA. Our model identifies three changepoints in the transmission levels of COVID-19, which are indeed aligned with the ``waves'' due to specific variants encountered during the pandemic. The findings also provide interesting insights into the effects of vaccination and the extent of spatial and temporal dependence in different phases of the pandemic.
2.Elastic regression for irregularly sampled curves in $\mathbb{R}^d$
Authors:Lisa Steyer, Almond Stöcker, Sonja Greven
Abstract: We propose regression models for curve-valued responses in two or more dimensions, where only the image but not the parametrization of the curves is of interest. Examples of such data are handwritten letters, movement paths or outlines of objects. In the square-root-velocity framework, a parametrization invariant distance for curves is obtained as the quotient space metric with respect to the action of re-parametrization, which is by isometries. With this special case in mind, we discuss the generalization of 'linear' regression to quotient metric spaces more generally, before illustrating the usefulness of our approach for curves modulo re-parametrization. We address the issue of sparsely or irregularly sampled curves by using splines for modeling smooth conditional mean curves. We test this model in simulations and apply it to human hippocampal outlines, obtained from Magnetic Resonance Imaging scans. Here we model how the shape of the irregularly sampled hippocampus is related to age, Alzheimer's disease and sex.