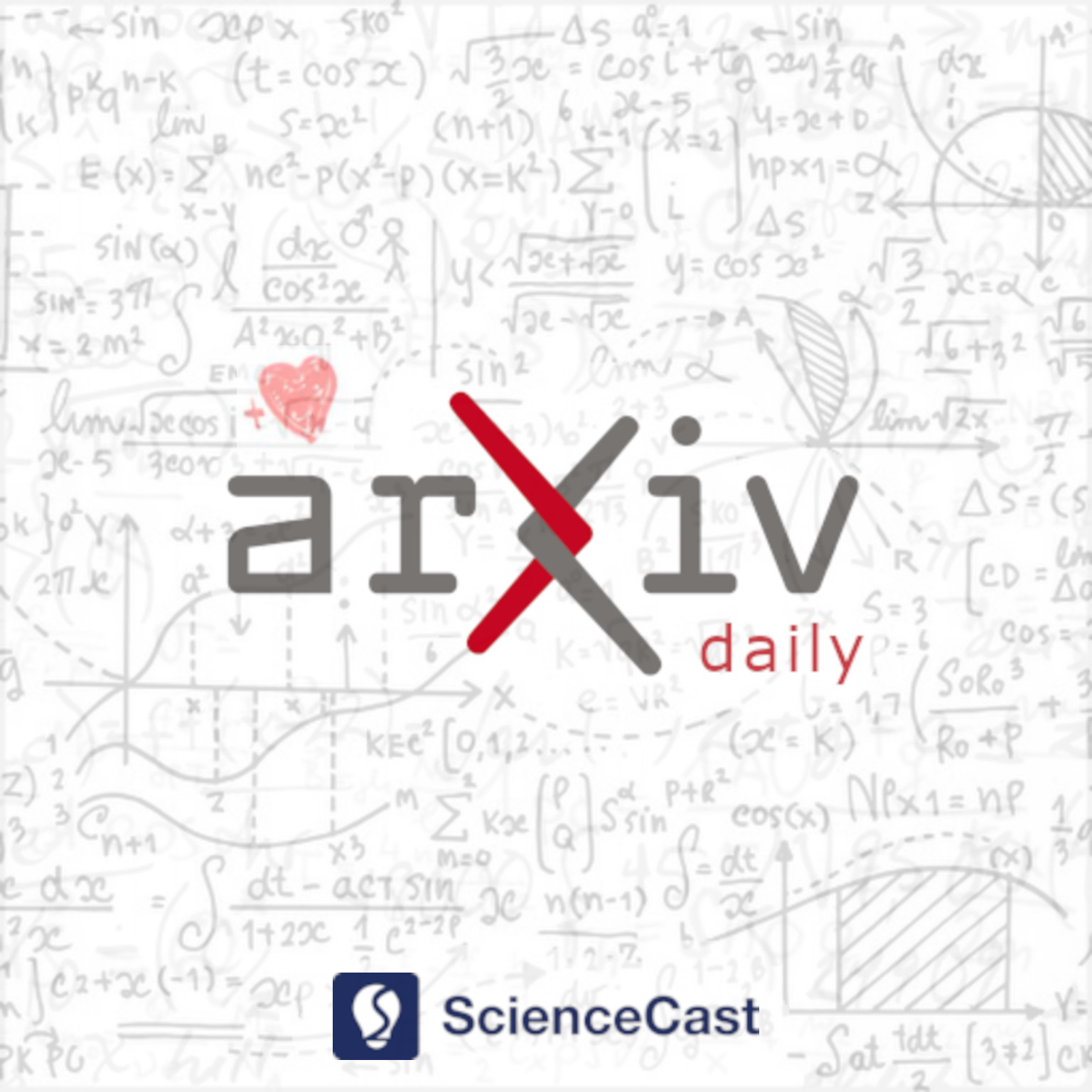
Methodology (stat.ME)
Tue, 29 Aug 2023
1.Adjusting inverse regression for predictors with clustered distribution
Authors:Wei Luo, Yan Guo
Abstract: A major family of sufficient dimension reduction (SDR) methods, called inverse regression, commonly require the distribution of the predictor $X$ to have a linear $E(X|\beta^\mathsf{T}X)$ and a degenerate $\mathrm{var}(X|\beta^\mathsf{T}X)$ for the desired reduced predictor $\beta^\mathsf{T}X$. In this paper, we adjust the first and second-order inverse regression methods by modeling $E(X|\beta^\mathsf{T}X)$ and $\mathrm{var}(X|\beta^\mathsf{T}X)$ under the mixture model assumption on $X$, which allows these terms to convey more complex patterns and is most suitable when $X$ has a clustered sample distribution. The proposed SDR methods build a natural path between inverse regression and the localized SDR methods, and in particular inherit the advantages of both; that is, they are $\sqrt{n}$-consistent, efficiently implementable, directly adjustable under the high-dimensional settings, and fully recovering the desired reduced predictor. These findings are illustrated by simulation studies and a real data example at the end, which also suggest the effectiveness of the proposed methods for nonclustered data.
2.Small Area Estimation with Random Forests and the LASSO
Authors:Victoire Michal, Jon Wakefield, Alexandra M. Schmidt, Alicia Cavanaugh, Brian Robinson, Jill Baumgartner
Abstract: We consider random forests and LASSO methods for model-based small area estimation when the number of areas with sampled data is a small fraction of the total areas for which estimates are required. Abundant auxiliary information is available for the sampled areas, from the survey, and for all areas, from an exterior source, and the goal is to use auxiliary variables to predict the outcome of interest. We compare areal-level random forests and LASSO approaches to a frequentist forward variable selection approach and a Bayesian shrinkage method. Further, to measure the uncertainty of estimates obtained from random forests and the LASSO, we propose a modification of the split conformal procedure that relaxes the assumption of identically distributed data. This work is motivated by Ghanaian data available from the sixth Living Standard Survey (GLSS) and the 2010 Population and Housing Census. We estimate the areal mean household log consumption using both datasets. The outcome variable is measured only in the GLSS for 3\% of all the areas (136 out of 5019) and more than 170 potential covariates are available from both datasets. Among the four modelling methods considered, the Bayesian shrinkage performed the best in terms of bias, MSE and prediction interval coverages and scores, as assessed through a cross-validation study. We find substantial between-area variation, the log consumption areal point estimates showing a 1.3-fold variation across the GAMA region. The western areas are the poorest while the Accra Metropolitan Area district gathers the richest areas.
3.Flexible parametrization of graph-theoretical features from individual-specific networks for prediction
Authors:Mariella Gregorich, Sean L. Simpson, Georg Heinze
Abstract: Statistical techniques are needed to analyse data structures with complex dependencies such that clinically useful information can be extracted. Individual-specific networks, which capture dependencies in complex biological systems, are often summarized by graph-theoretical features. These features, which lend themselves to outcome modelling, can be subject to high variability due to arbitrary decisions in network inference and noise. Correlation-based adjacency matrices often need to be sparsified before meaningful graph-theoretical features can be extracted, requiring the data analysts to determine an optimal threshold.. To address this issue, we propose to incorporate a flexible weighting function over the full range of possible thresholds to capture the variability of graph-theoretical features over the threshold domain. The potential of this approach, which extends concepts from functional data analysis to a graph-theoretical setting, is explored in a plasmode simulation study using real functional magnetic resonance imaging (fMRI) data from the Autism Brain Imaging Data Exchange (ABIDE) Preprocessed initiative. The simulations show that our modelling approach yields accurate estimates of the functional form of the weight function, improves inference efficiency, and achieves a comparable or reduced root mean square prediction error compared to competitor modelling approaches. This assertion holds true in settings where both complex functional forms underlie the outcome-generating process and a universal threshold value is employed. We demonstrate the practical utility of our approach by using resting-state fMRI data to predict biological age in children. Our study establishes the flexible modelling approach as a statistically principled, serious competitor to ad-hoc methods with superior performance.
4.Hedging Forecast Combinations With an Application to the Random Forest
Authors:Elliot Beck, Damian Kozbur, Michael Wolf
Abstract: This papers proposes a generic, high-level methodology for generating forecast combinations that would deliver the optimal linearly combined forecast in terms of the mean-squared forecast error if one had access to two population quantities: the mean vector and the covariance matrix of the vector of individual forecast errors. We point out that this problem is identical to a mean-variance portfolio construction problem, in which portfolio weights correspond to forecast combination weights. We allow negative forecast weights and interpret such weights as hedging over and under estimation risks across estimators. This interpretation follows directly as an implication of the portfolio analogy. We demonstrate our method's improved out-of-sample performance relative to standard methods in combining tree forecasts to form weighted random forests in 14 data sets.