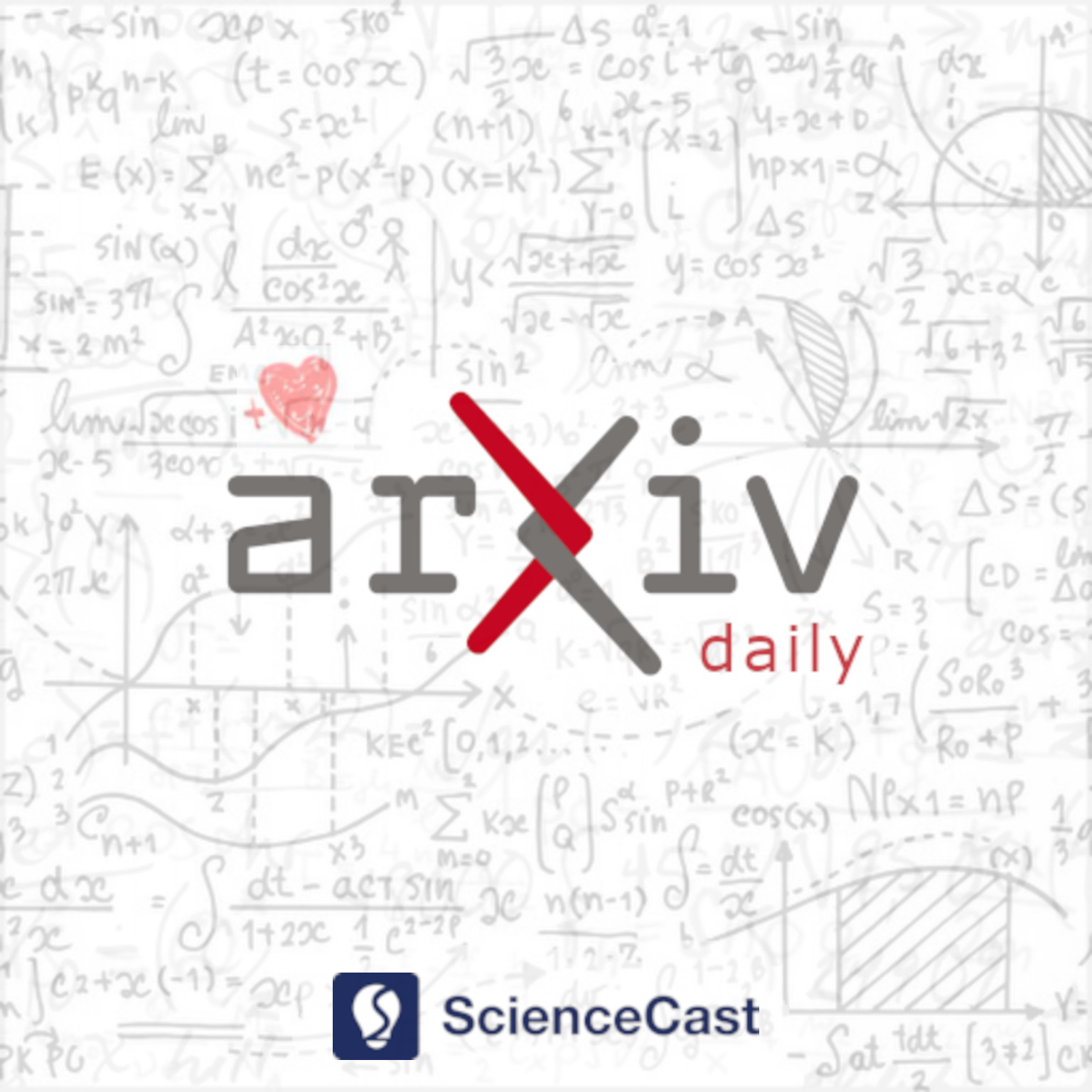
Methodology (stat.ME)
Wed, 05 Jul 2023
1.Replicability of Simulation Studies for the Investigation of Statistical Methods: The RepliSims Project
Authors:K. Luijken, A. Lohmann, U. Alter, J. Claramunt Gonzalez, F. J. Clouth, J. L. Fossum, L. Hesen, A. H. J. Huizing, J. Ketelaar, A. K. Montoya, L. Nab, R. C. C. Nijman, B. B. L. Penning de Vries, T. D. Tibbe, Y. A. Wang, R. H. H. Groenwold
Abstract: Results of simulation studies evaluating the performance of statistical methods are often considered actionable and thus can have a major impact on the way empirical research is implemented. However, so far there is limited evidence about the reproducibility and replicability of statistical simulation studies. Therefore, eight highly cited statistical simulation studies were selected, and their replicability was assessed by teams of replicators with formal training in quantitative methodology. The teams found relevant information in the original publications and used it to write simulation code with the aim of replicating the results. The primary outcome was the feasibility of replicability based on reported information in the original publications. Replicability varied greatly: Some original studies provided detailed information leading to almost perfect replication of results, whereas other studies did not provide enough information to implement any of the reported simulations. Replicators had to make choices regarding missing or ambiguous information in the original studies, error handling, and software environment. Factors facilitating replication included public availability of code, and descriptions of the data-generating procedure and methods in graphs, formulas, structured text, and publicly accessible additional resources such as technical reports. Replicability of statistical simulation studies was mainly impeded by lack of information and sustainability of information sources. Reproducibility could be achieved for simulation studies by providing open code and data as a supplement to the publication. Additionally, simulation studies should be transparently reported with all relevant information either in the research paper itself or in easily accessible supplementary material to allow for replicability.
2.On multivariate orderings of some general ordered random vectors
Authors:Tanmay sahoo, Nil Kamal Hazra, Narayanaswamy Balakrishnan
Abstract: Ordered random vectors are frequently encountered in many problems. The generalized order statistics (GOS) and sequential order statistics (SOS) are two general models for ordered random vectors. However, these two models do not capture the dependency structures that are present in the underlying random variables. In this paper, we study the developed sequential order statistics (DSOS) and developed generalized order statistics (DGOS) models that describe the dependency structures of ordered random vectors. We then study various univariate and multivariate ordering properties of DSOS and DGOS models under Archimedean copula. We consider both one-sample and two-sample scenarios and develop corresponding results.
3.Extending the Dixon and Coles model: an application to women's football data
Authors:Rouven Michels, Marius Ötting, Dimitris Karlis
Abstract: The prevalent model by Dixon and Coles (1997) extends the double Poisson model where two independent Poisson distributions model the number of goals scored by each team by moving probabilities between the scores 0-0, 0-1, 1-0, and 1-1. We show that this is a special case of a multiplicative model known as the Sarmanov family. Based on this family, we create more suitable models by moving probabilities between scores and employing other discrete distributions. We apply the new models to women's football scores, which exhibit some characteristics different than that of men's football.
4.Noise reduction for functional time series
Authors:Cees Diks, Bram Wouters
Abstract: A novel method for noise reduction in the setting of curve time series with error contamination is proposed, based on extending the framework of functional principal component analysis (FPCA). We employ the underlying, finite-dimensional dynamics of the functional time series to separate the serially dependent dynamical part of the observed curves from the noise. Upon identifying the subspaces of the signal and idiosyncratic components, we construct a projection of the observed curve time series along the noise subspace, resulting in an estimate of the underlying denoised curves. This projection is optimal in the sense that it minimizes the mean integrated squared error. By applying our method to similated and real data, we show the denoising estimator is consistent and outperforms existing denoising techniques. Furthermore, we show it can be used as a pre-processing step to improve forecasting.
5.Table inference for combinatorial origin-destination choices in agent-based population synthesis
Authors:Ioannis Zachos, Theodoros Damoulas, Mark Girolami
Abstract: A key challenge in agent-based mobility simulations is the synthesis of individual agent socioeconomic profiles. Such profiles include locations of agent activities, which dictate the quality of the simulated travel patterns. These locations are typically represented in origin-destination matrices that are sampled using coarse travel surveys. This is because fine-grained trip profiles are scarce and fragmented due to privacy and cost reasons. The discrepancy between data and sampling resolutions renders agent traits non-identifiable due to the combinatorial space of data-consistent individual attributes. This problem is pertinent to any agent-based inference setting where the latent state is discrete. Existing approaches have used continuous relaxations of the underlying location assignments and subsequent ad-hoc discretisation thereof. We propose a framework to efficiently navigate this space offering improved reconstruction and coverage as well as linear-time sampling of the ground truth origin-destination table. This allows us to avoid factorially growing rejection rates and poor summary statistic consistency inherent in discrete choice modelling. We achieve this by introducing joint sampling schemes for the continuous intensity and discrete table of agent trips, as well as Markov bases that can efficiently traverse this combinatorial space subject to summary statistic constraints. Our framework's benefits are demonstrated in multiple controlled experiments and a large-scale application to agent work trip reconstruction in Cambridge, UK.
6.Improving Algorithms for Fantasy Basketball
Authors:Zach Rosenof
Abstract: Fantasy basketball has a rich underlying mathematical structure which makes optimal drafting strategy unclear. The most commonly used heuristic, "Z-score" ranking, is appropriate only with the unrealistic condition that weekly player performance is known exactly beforehand. An alternative heuristic is derived by adjusting the assumptions that justify Z-scores to incorporate uncertainty in weekly performance. It is dubbed the "G-score", and it outperforms the traditional Z-score approach in simulations. A more sophisticated algorithm is also derived, which dynamically adapts to previously chosen players to create a cohesive team. It is dubbed "H-scoring" and outperforms both Z-score and G-score.
7.D-optimal Subsampling Design for Massive Data Linear Regression
Authors:Torsten Reuter, Rainer Schwabe
Abstract: Data reduction is a fundamental challenge of modern technology, where classical statistical methods are not applicable because of computational limitations. We consider linear regression for an extraordinarily large number of observations, but only a few covariates. Subsampling aims at the selection of a given percentage of the existing original data. Under distributional assumptions on the covariates, we derive D-optimal subsampling designs and study their theoretical properties. We make use of fundamental concepts of optimal design theory and an equivalence theorem from constrained convex optimization. The thus obtained subsampling designs provide simple rules for whether to accept or reject a data point, allowing for an easy algorithmic implementation. In addition, we propose a simplified subsampling method that differs from the D-optimal design but requires lower computing time. We present a simulation study, comparing both subsampling schemes with the IBOSS method.
8.Differential recall bias in estimating treatment effects in observational studies
Authors:Suhwan Bong, Kwonsang Lee, Francesca Dominici
Abstract: Observational studies are frequently used to estimate the effect of an exposure or treatment on an outcome. To obtain an unbiased estimate of the treatment effect, it is crucial to measure the exposure accurately. A common type of exposure misclassification is recall bias, which occurs in retrospective cohort studies when study subjects may inaccurately recall their past exposure. Specifically, differential recall bias can be problematic when examining the effect of a self-reported binary exposure since the magnitude of recall bias can differ between groups. In this paper, we provide the following contributions: 1) we derive bounds for the average treatment effect (ATE) in the presence of recall bias; 2) we develop several estimation approaches under different identification strategies; 3) we conduct simulation studies to evaluate their performance under several scenarios of model misspecification; 4) we propose a sensitivity analysis method that can examine the robustness of our results with respect to different assumptions; and 5) we apply the proposed framework to an observational study, estimating the effect of childhood physical abuse on adulthood mental health.
9.Multi-Task Learning with Summary Statistics
Authors:Parker Knight, Rui Duan
Abstract: Multi-task learning has emerged as a powerful machine learning paradigm for integrating data from multiple sources, leveraging similarities between tasks to improve overall model performance. However, the application of multi-task learning to real-world settings is hindered by data-sharing constraints, especially in healthcare settings. To address this challenge, we propose a flexible multi-task learning framework utilizing summary statistics from various sources. Additionally, we present an adaptive parameter selection approach based on a variant of Lepski's method, allowing for data-driven tuning parameter selection when only summary statistics are available. Our systematic non-asymptotic analysis characterizes the performance of the proposed methods under various regimes of the sample complexity and overlap. We demonstrate our theoretical findings and the performance of the method through extensive simulations. This work offers a more flexible tool for training related models across various domains, with practical implications in genetic risk prediction and many other fields.
10.Bayesian Structure Learning in Undirected Gaussian Graphical Models: Literature Review with Empirical Comparison
Authors:Lucas Vogels, Reza Mohammadi, Marit Schoonhoven, Ş. İlker Birbil
Abstract: Gaussian graphical models are graphs that represent the conditional relationships among multivariate normal variables. The process of uncovering the structure of these graphs is known as structure learning. Despite the fact that Bayesian methods in structure learning offer intuitive and well-founded ways to measure model uncertainty and integrate prior information, frequentist methods are often preferred due to the computational burden of the Bayesian approach. Over the last decade, Bayesian methods have seen substantial improvements, with some now capable of generating accurate estimates of graphs up to a thousand variables in mere minutes. Despite these advancements, a comprehensive review or empirical comparison of all cutting-edge methods has not been conducted. This paper delves into a wide spectrum of Bayesian approaches used in structure learning, evaluates their efficacy through a simulation study, and provides directions for future research. This study gives an exhaustive overview of this dynamic field for both newcomers and experts.
11.Bayesian D- and I-optimal designs for choice experiments involving mixtures and process variables
Authors:Mario Becerra, Peter Goos
Abstract: Many food products involve mixtures of ingredients, where the mixtures can be expressed as combinations of ingredient proportions. In many cases, the quality and the consumer preference may also depend on the way in which the mixtures are processed. The processing is generally defined by the settings of one or more process variables. Experimental designs studying the joint impact of the mixture ingredient proportions and the settings of the process variables are called mixture-process variable experiments. In this article, we show how to combine mixture-process variable experiments and discrete choice experiments, to quantify and model consumer preferences for food products that can be viewed as processed mixtures. First, we describe the modeling of data from such combined experiments. Next, we describe how to generate D- and I-optimal designs for choice experiments involving mixtures and process variables, and we compare the two kinds of designs using two examples.
12.A Complete Characterisation of Structured Missingness
Authors:James Jackson, Robin Mitra, Niels Hagenbuch, Sarah McGough, Chris Harbron
Abstract: Our capacity to process large complex data sources is ever-increasing, providing us with new, important applied research questions to address, such as how to handle missing values in large-scale databases. Mitra et al. (2023) noted the phenomenon of Structured Missingness (SM), which is where missingness has an underlying structure. Existing taxonomies for defining missingness mechanisms typically assume that variables' missingness indicator vectors $M_1$, $M_2$, ..., $M_p$ are independent after conditioning on the relevant portion of the data matrix $\mathbf{X}$. As this is often unsuitable for characterising SM in multivariate settings, we introduce a taxonomy for SM, where each ${M}_j$ can depend on $\mathbf{M}_{-j}$ (i.e., all missingness indicator vectors except ${M}_j$), in addition to $\mathbf{X}$. We embed this new framework within the well-established decomposition of mechanisms into MCAR, MAR, and MNAR (Rubin, 1976), allowing us to recast mechanisms into a broader setting, where we can consider the combined effect of $\mathbf{X}$ and $\mathbf{M}_{-j}$ on ${M}_j$. We also demonstrate, via simulations, the impact of SM on inference and prediction, and consider contextual instances of SM arising in a de-identified nationwide (US-based) clinico-genomic database (CGDB). We hope to stimulate interest in SM, and encourage timely research into this phenomenon.
13.Unveiling Causal Mediation Pathways in High-Dimensional Mixed Exposures: A Data-Adaptive Target Parameter Strategy
Authors:David B. McCoy, Alan E. Hubbard, Mark van der Laan, Alejandro Schuler
Abstract: Mediation analysis in causal inference typically concentrates on one binary exposure, using deterministic interventions to split the average treatment effect into direct and indirect effects through a single mediator. Yet, real-world exposure scenarios often involve multiple continuous exposures impacting health outcomes through varied mediation pathways, which remain unknown a priori. Addressing this complexity, we introduce NOVAPathways, a methodological framework that identifies exposure-mediation pathways and yields unbiased estimates of direct and indirect effects when intervening on these pathways. By pairing data-adaptive target parameters with stochastic interventions, we offer a semi-parametric approach for estimating causal effects in the context of high-dimensional, continuous, binary, and categorical exposures and mediators. In our proposed cross-validation procedure, we apply sequential semi-parametric regressions to a parameter-generating fold of the data, discovering exposure-mediation pathways. We then use stochastic interventions on these pathways in an estimation fold of the data to construct efficient estimators of natural direct and indirect effects using flexible machine learning techniques. Our estimator proves to be asymptotically linear under conditions necessitating n to the negative quarter consistency of nuisance function estimation. Simulation studies demonstrate the square root n consistency of our estimator when the exposure is quantized, whereas for truly continuous data, approximations in numerical integration prevent square root n consistency. Our NOVAPathways framework, part of the open-source SuperNOVA package in R, makes our proposed methodology for high-dimensional mediation analysis available to researchers, paving the way for the application of modified exposure policies which can delivery more informative statistical results for public policy.
14.High-Dimensional Expected Shortfall Regression
Authors:Shushu Zhang, Xuming He, Kean Ming Tan, Wen-Xin Zhou
Abstract: The expected shortfall is defined as the average over the tail below (or above) a certain quantile of a probability distribution. The expected shortfall regression provides powerful tools for learning the relationship between a response variable and a set of covariates while exploring the heterogeneous effects of the covariates. In the health disparity research, for example, the lower/upper tail of the conditional distribution of a health-related outcome, given high-dimensional covariates, is often of importance. Under sparse models, we propose the lasso-penalized expected shortfall regression and establish non-asymptotic error bounds, depending explicitly on the sample size, dimension, and sparsity, for the proposed estimator. To perform statistical inference on a covariate of interest, we propose a debiased estimator and establish its asymptotic normality, from which asymptotically valid tests can be constructed. We illustrate the finite sample performance of the proposed method through numerical studies and a data application on health disparity.