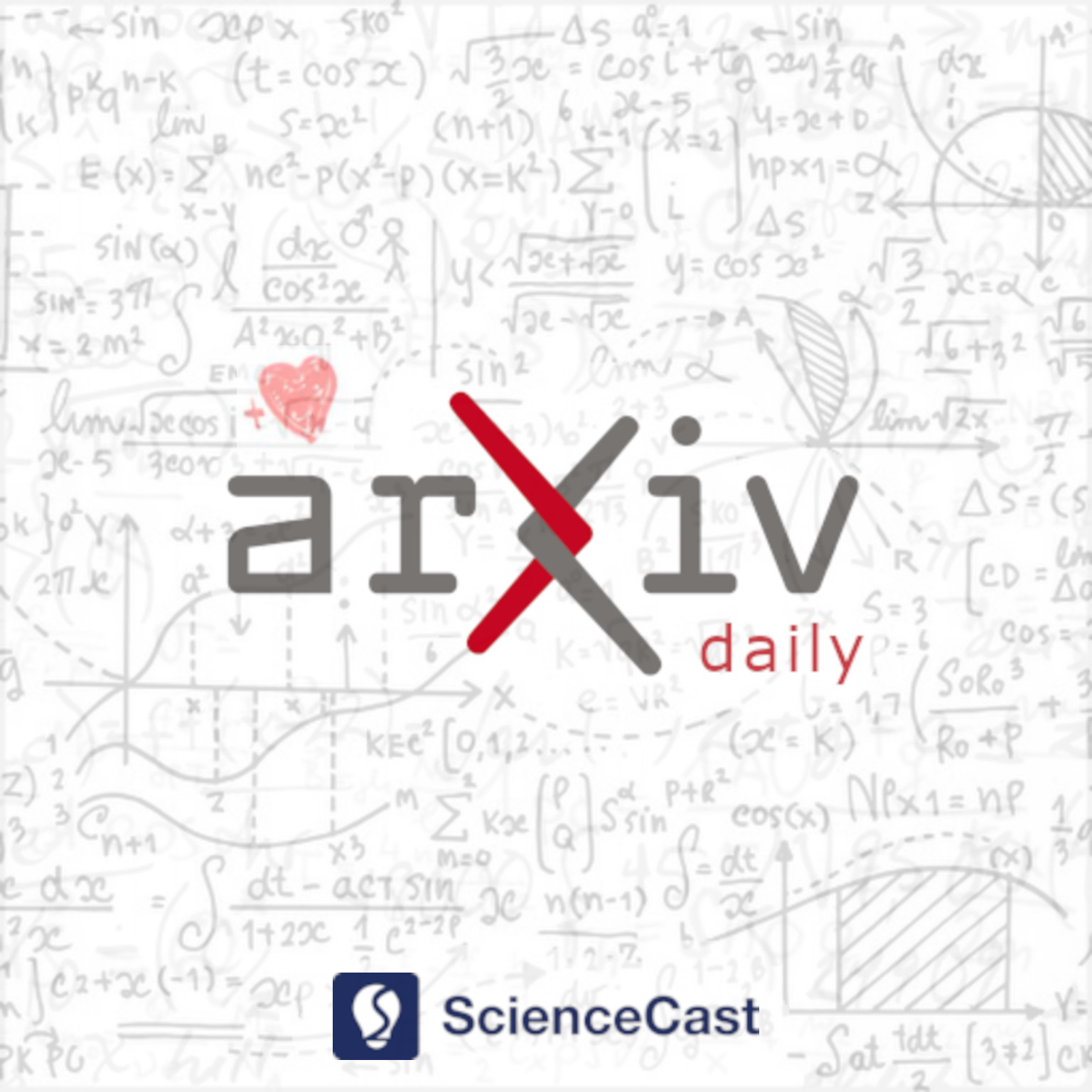
Methodology (stat.ME)
Thu, 06 Jul 2023
1.Decomposing Triple-Differences Regression under Staggered Adoption
Authors:Anton Strezhnev
Abstract: The triple-differences (TD) design is a popular identification strategy for causal effects in settings where researchers do not believe the parallel trends assumption of conventional difference-in-differences (DiD) is satisfied. TD designs augment the conventional 2x2 DiD with a "placebo" stratum -- observations that are nested in the same units and time periods but are known to be entirely unaffected by the treatment. However, many TD applications go beyond this simple 2x2x2 and use observations on many units in many "placebo" strata across multiple time periods. A popular estimator for this setting is the triple-differences regression (TDR) fixed-effects estimator -- an extension of the common "two-way fixed effects" estimator for DiD. This paper decomposes the TDR estimator into its component two-group/two-period/two-strata triple-differences and illustrates how interpreting this parameter causally in settings with arbitrary staggered adoption requires strong effect homogeneity assumptions as many placebo DiDs incorporate observations under treatment. The decomposition clarifies the implied identifying variation behind the triple-differences regression estimator and suggests researchers should be cautious when implementing these estimators in settings more complex than the 2x2x2 case. Alternative approaches that only incorporate "clean placebos" such as direct imputation of the counterfactual may be more appropriate. The paper concludes by demonstrating the utility of this imputation estimator in an application of the "gravity model" to the estimation of the effect of the WTO/GATT on international trade.
2.Geometric Mean Type of Proportional Reduction in Variation Measure for Two-Way Contingency Tables
Authors:Wataru Urasaki, Yuki Wada, Tomoyuki Nakagawa, Kouji Tahata, Sadao Tomizawa
Abstract: In a two-way contingency table analysis with explanatory and response variables, the analyst is interested in the independence of the two variables. However, if the test of independence does not show independence or clearly shows a relationship, the analyst is interested in the degree of their association. Various measures have been proposed to calculate the degree of their association, one of which is the proportional reduction in variation (PRV) measure which describes the PRV from the marginal distribution to the conditional distribution of the response. The conventional PRV measures can assess the association of the entire contingency table, but they can not accurately assess the association for each explanatory variable. In this paper, we propose a geometric mean type of PRV (geoPRV) measure that aims to sensitively capture the association of each explanatory variable to the response variable by using a geometric mean, and it enables analysis without underestimation when there is partial bias in cells of the contingency table. Furthermore, the geoPRV measure is constructed by using any functions that satisfy specific conditions, which has application advantages and makes it possible to express conventional PRV measures as geometric mean types in special cases.