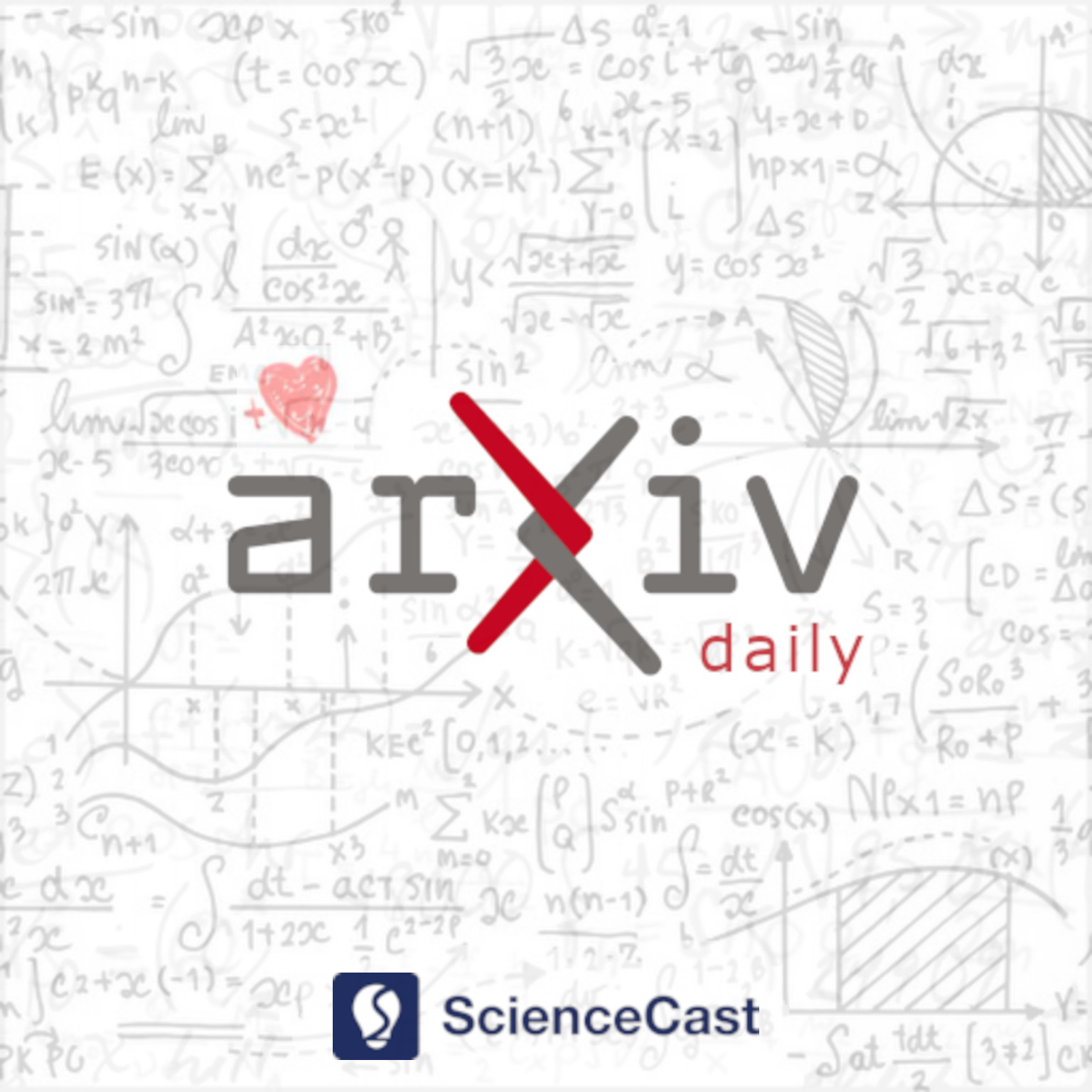
Methodology (stat.ME)
Tue, 11 Jul 2023
1.Summary characteristics for multivariate function-valued spatial point process attributes
Authors:Matthias Eckardt, Carles Comas, Jorge Mateu
Abstract: Prompted by modern technologies in data acquisition, the statistical analysis of spatially distributed function-valued quantities has attracted a lot of attention in recent years. In particular, combinations of functional variables and spatial point processes yield a highly challenging instance of such modern spatial data applications. Indeed, the analysis of spatial random point configurations, where the point attributes themselves are functions rather than scalar-valued quantities, is just in its infancy, and extensions to function-valued quantities still remain limited. In this view, we extend current existing first- and second-order summary characteristics for real-valued point attributes to the case where in addition to every spatial point location a set of distinct function-valued quantities are available. Providing a flexible treatment of more complex point process scenarios, we build a framework to consider points with multivariate function-valued marks, and develop sets of different cross-function (cross-type and also multi-function cross-type) versions of summary characteristics that allow for the analysis of highly demanding modern spatial point process scenarios. We consider estimators of the theoretical tools and analyse their behaviour through a simulation study and two real data applications.
2.CR-Lasso: Robust cellwise regularized sparse regression
Authors:Peng Su, Garth Tarr, Samuel Muller, Suojin Wang
Abstract: Cellwise contamination remains a challenging problem for data scientists, particularly in research fields that require the selection of sparse features. Traditional robust methods may not be feasible nor efficient in dealing with such contaminated datasets. We propose CR-Lasso, a robust Lasso-type cellwise regularization procedure that performs feature selection in the presence of cellwise outliers by minimising a regression loss and cell deviation measure simultaneously. To evaluate the approach, we conduct empirical studies comparing its selection and prediction performance with several sparse regression methods. We show that CR-Lasso is competitive under the settings considered. We illustrate the effectiveness of the proposed method on real data through an analysis of a bone mineral density dataset.
3.A stochastic optimization approach to minimize robust density power-based divergences for general parametric density models
Authors:Akifumi Okuno
Abstract: Density power divergence (DPD) [Basu et al. (1998), Biometrika], designed to estimate the underlying distribution of the observations robustly, comprises an integral term of the power of the parametric density models to be estimated. While the explicit form of the integral term can be obtained for some specific densities (such as normal density and exponential density), its computational intractability has prohibited the application of DPD-based estimation to more general parametric densities, over a quarter of a century since the proposal of DPD. This study proposes a stochastic optimization approach to minimize DPD for general parametric density models and explains its adequacy by referring to conventional theories on stochastic optimization. The proposed approach also can be applied to the minimization of another density power-based $\gamma$-divergence with the aid of unnormalized models [Kanamori and Fujisawa (2015), Biometrika].