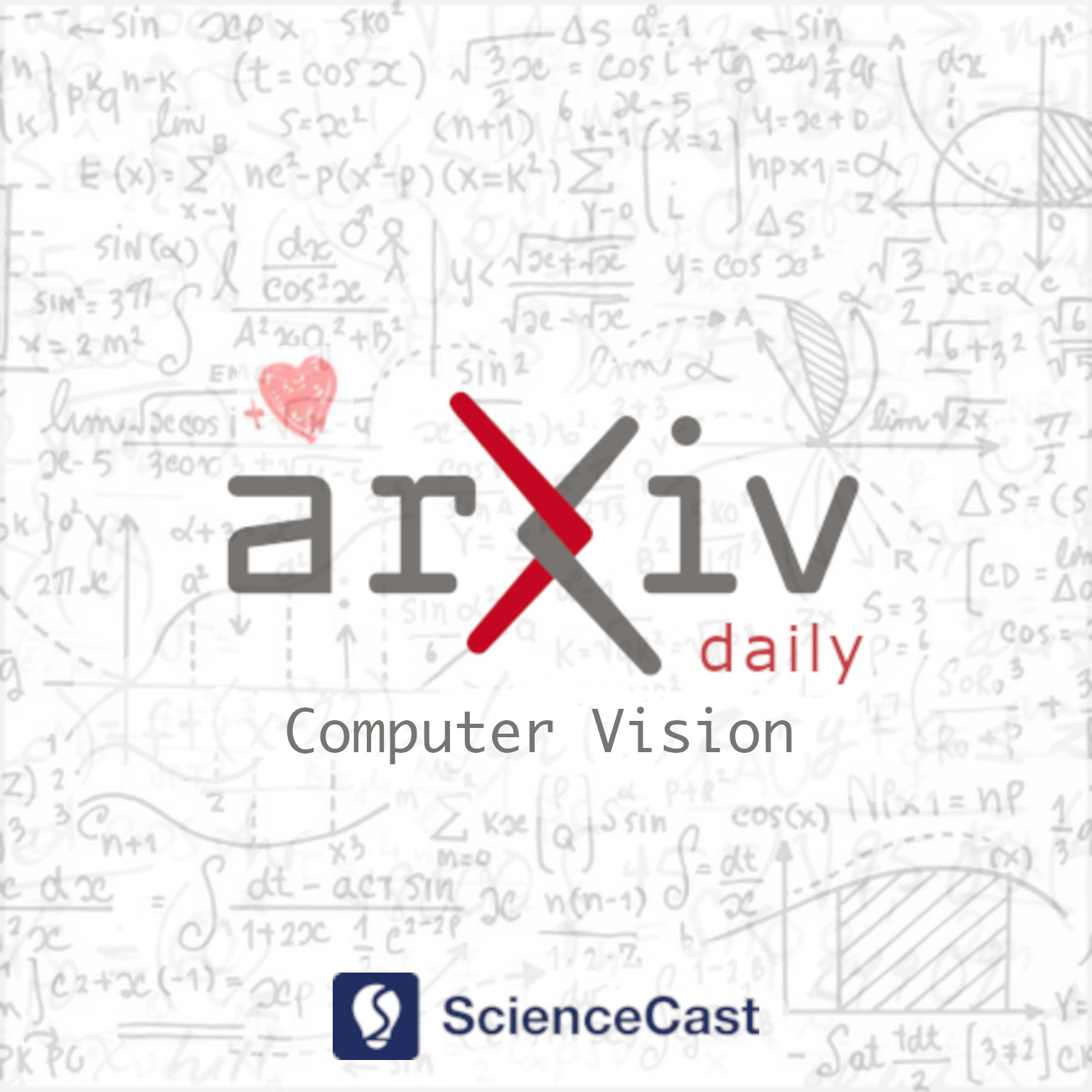
Computer Vision and Pattern Recognition (cs.CV)
Mon, 22 May 2023
1.Bridging the Gap Between End-to-end and Non-End-to-end Multi-Object Tracking
Authors:Feng Yan, Weixin Luo, Yujie Zhong, Yiyang Gan, Lin Ma
Abstract: Existing end-to-end Multi-Object Tracking (e2e-MOT) methods have not surpassed non-end-to-end tracking-by-detection methods. One potential reason is its label assignment strategy during training that consistently binds the tracked objects with tracking queries and then assigns the few newborns to detection queries. With one-to-one bipartite matching, such an assignment will yield unbalanced training, i.e., scarce positive samples for detection queries, especially for an enclosed scene, as the majority of the newborns come on stage at the beginning of videos. Thus, e2e-MOT will be easier to yield a tracking terminal without renewal or re-initialization, compared to other tracking-by-detection methods. To alleviate this problem, we present Co-MOT, a simple and effective method to facilitate e2e-MOT by a novel coopetition label assignment with a shadow concept. Specifically, we add tracked objects to the matching targets for detection queries when performing the label assignment for training the intermediate decoders. For query initialization, we expand each query by a set of shadow counterparts with limited disturbance to itself. With extensive ablations, Co-MOT achieves superior performance without extra costs, e.g., 69.4% HOTA on DanceTrack and 52.8% TETA on BDD100K. Impressively, Co-MOT only requires 38\% FLOPs of MOTRv2 to attain a similar performance, resulting in the 1.4$\times$ faster inference speed.
2.Towards Explainable In-the-Wild Video Quality Assessment: a Database and a Language-Prompted Approach
Authors:Haoning Wu, Erli Zhang, Liang Liao, Chaofeng Chen, Jingwen Hou, Annan Wang, Wenxiu Sun, Qiong Yan, Weisi Lin
Abstract: The proliferation of in-the-wild videos has greatly expanded the Video Quality Assessment (VQA) problem. Unlike early definitions that usually focus on limited distortion types, VQA on in-the-wild videos is especially challenging as it could be affected by complicated factors, including various distortions and diverse contents. Though subjective studies have collected overall quality scores for these videos, how the abstract quality scores relate with specific factors is still obscure, hindering VQA methods from more concrete quality evaluations (e.g. sharpness of a video). To solve this problem, we collect over two million opinions on 4,543 in-the-wild videos on 13 dimensions of quality-related factors, including in-capture authentic distortions (e.g. motion blur, noise, flicker), errors introduced by compression and transmission, and higher-level experiences on semantic contents and aesthetic issues (e.g. composition, camera trajectory), to establish the multi-dimensional Maxwell database. Specifically, we ask the subjects to label among a positive, a negative, and a neural choice for each dimension. These explanation-level opinions allow us to measure the relationships between specific quality factors and abstract subjective quality ratings, and to benchmark different categories of VQA algorithms on each dimension, so as to more comprehensively analyze their strengths and weaknesses. Furthermore, we propose the MaxVQA, a language-prompted VQA approach that modifies vision-language foundation model CLIP to better capture important quality issues as observed in our analyses. The MaxVQA can jointly evaluate various specific quality factors and final quality scores with state-of-the-art accuracy on all dimensions, and superb generalization ability on existing datasets. Code and data available at \url{https://github.com/VQAssessment/MaxVQA}.
3.EMEF: Ensemble Multi-Exposure Image Fusion
Authors:Renshuai Liu, Chengyang Li, Haitao Cao, Yinglin Zheng, Ming Zeng, Xuan Cheng
Abstract: Although remarkable progress has been made in recent years, current multi-exposure image fusion (MEF) research is still bounded by the lack of real ground truth, objective evaluation function, and robust fusion strategy. In this paper, we study the MEF problem from a new perspective. We don't utilize any synthesized ground truth, design any loss function, or develop any fusion strategy. Our proposed method EMEF takes advantage of the wisdom of multiple imperfect MEF contributors including both conventional and deep learning-based methods. Specifically, EMEF consists of two main stages: pre-train an imitator network and tune the imitator in the runtime. In the first stage, we make a unified network imitate different MEF targets in a style modulation way. In the second stage, we tune the imitator network by optimizing the style code, in order to find an optimal fusion result for each input pair. In the experiment, we construct EMEF from four state-of-the-art MEF methods and then make comparisons with the individuals and several other competitive methods on the latest released MEF benchmark dataset. The promising experimental results demonstrate that our ensemble framework can "get the best of all worlds". The code is available at https://github.com/medalwill/EMEF.
4.Semantic Invariant Multi-view Clustering with Fully Incomplete Information
Authors:Pengxin Zeng, Mouxing Yang, Yiding Lu, Changqing Zhang, Peng Hu, Xi Peng
Abstract: Robust multi-view learning with incomplete information has received significant attention due to issues such as incomplete correspondences and incomplete instances that commonly affect real-world multi-view applications. Existing approaches heavily rely on paired samples to realign or impute defective ones, but such preconditions cannot always be satisfied in practice due to the complexity of data collection and transmission. To address this problem, we present a novel framework called SeMantic Invariance LEarning (SMILE) for multi-view clustering with incomplete information that does not require any paired samples. To be specific, we discover the existence of invariant semantic distribution across different views, which enables SMILE to alleviate the cross-view discrepancy to learn consensus semantics without requiring any paired samples. The resulting consensus semantics remains unaffected by cross-view distribution shifts, making them useful for realigning/imputing defective instances and forming clusters. We demonstrate the effectiveness of SMILE through extensive comparison experiments with 13 state-of-the-art baselines on five benchmarks. Our approach improves the clustering accuracy of NoisyMNIST from 19.3\%/23.2\% to 82.7\%/69.0\% when the correspondences/instances are fully incomplete. We will release the code after acceptance.
5.D$^2$TV: Dual Knowledge Distillation and Target-oriented Vision Modeling for Many-to-Many Multimodal Summarization
Authors:Yunlong Liang, Fandong Meng, Jiaan Wang, Jinan Xu, Yufeng Chen, Jie Zhou
Abstract: Many-to-many multimodal summarization (M$^3$S) task aims to generate summaries in any language with document inputs in any language and the corresponding image sequence, which essentially comprises multimodal monolingual summarization (MMS) and multimodal cross-lingual summarization (MXLS) tasks. Although much work has been devoted to either MMS or MXLS and has obtained increasing attention in recent years, little research pays attention to the M$^3$S task. Besides, existing studies mainly focus on 1) utilizing MMS to enhance MXLS via knowledge distillation without considering the performance of MMS or 2) improving MMS models by filtering summary-unrelated visual features with implicit learning or explicitly complex training objectives. In this paper, we first introduce a general and practical task, i.e., M$^3$S. Further, we propose a dual knowledge distillation and target-oriented vision modeling framework for the M$^3$S task. Specifically, the dual knowledge distillation method guarantees that the knowledge of MMS and MXLS can be transferred to each other and thus mutually prompt both of them. To offer target-oriented visual features, a simple yet effective target-oriented contrastive objective is designed and responsible for discarding needless visual information. Extensive experiments on the many-to-many setting show the effectiveness of the proposed approach. Additionally, we will contribute a many-to-many multimodal summarization (M$^3$Sum) dataset.
6.Semantic Segmentation of Radar Detections using Convolutions on Point Clouds
Authors:Marco Braun, Alessandro Cennamo, Markus Schoeler, Kevin Kollek, Anton Kummert
Abstract: For autonomous driving, radar sensors provide superior reliability regardless of weather conditions as well as a significantly high detection range. State-of-the-art algorithms for environment perception based on radar scans build up on deep neural network architectures that can be costly in terms of memory and computation. By processing radar scans as point clouds, however, an increase in efficiency can be achieved in this respect. While Convolutional Neural Networks show superior performance on pattern recognition of regular data formats like images, the concept of convolutions is not yet fully established in the domain of radar detections represented as point clouds. The main challenge in convolving point clouds lies in their irregular and unordered data format and the associated permutation variance. Therefore, we apply a deep-learning based method introduced by PointCNN that weights and permutes grouped radar detections allowing the resulting permutation invariant cluster to be convolved. In addition, we further adapt this algorithm to radar-specific properties through distance-dependent clustering and pre-processing of input point clouds. Finally, we show that our network outperforms state-of-the-art approaches that are based on PointNet++ on the task of semantic segmentation of radar point clouds.
7.Spatiotemporal Attention-based Semantic Compression for Real-time Video Recognition
Authors:Nan Li, Mehdi Bennis, Alexandros Iosifidis, Qi Zhang
Abstract: This paper studies the computational offloading of video action recognition in edge computing. To achieve effective semantic information extraction and compression, following semantic communication we propose a novel spatiotemporal attention-based autoencoder (STAE) architecture, including a frame attention module and a spatial attention module, to evaluate the importance of frames and pixels in each frame. Additionally, we use entropy encoding to remove statistical redundancy in the compressed data to further reduce communication overhead. At the receiver, we develop a lightweight decoder that leverages a 3D-2D CNN combined architecture to reconstruct missing information by simultaneously learning temporal and spatial information from the received data to improve accuracy. To fasten convergence, we use a step-by-step approach to train the resulting STAE-based vision transformer (ViT_STAE) models. Experimental results show that ViT_STAE can compress the video dataset HMDB51 by 104x with only 5% accuracy loss, outperforming the state-of-the-art baseline DeepISC. The proposed ViT_STAE achieves faster inference and higher accuracy than the DeepISC-based ViT model under time-varying wireless channel, which highlights the effectiveness of STAE in guaranteeing higher accuracy under time constraints.
8.Interactive Data Synthesis for Systematic Vision Adaptation via LLMs-AIGCs Collaboration
Authors:Qifan Yu, Juncheng Li, Wentao Ye, Siliang Tang, Yueting Zhuang
Abstract: Recent text-to-image generation models have shown promising results in generating high-fidelity photo-realistic images. In parallel, the problem of data scarcity has brought a growing interest in employing AIGC technology for high-quality data expansion. However, this paradigm requires well-designed prompt engineering that cost-less data expansion and labeling remain under-explored. Inspired by LLM's powerful capability in task guidance, we propose a new paradigm of annotated data expansion named as ChatGenImage. The core idea behind it is to leverage the complementary strengths of diverse models to establish a highly effective and user-friendly pipeline for interactive data augmentation. In this work, we extensively study how LLMs communicate with AIGC model to achieve more controllable image generation and make the first attempt to collaborate them for automatic data augmentation for a variety of downstream tasks. Finally, we present fascinating results obtained from our ChatGenImage framework and demonstrate the powerful potential of our synthetic data for systematic vision adaptation. Our codes are available at https://github.com/Yuqifan1117/Labal-Anything-Pipeline.
9.Single Domain Dynamic Generalization for Iris Presentation Attack Detection
Authors:Yachun Li, Jingjing Wang, Yuhui Chen, Di Xie, Shiliang Pu
Abstract: Iris presentation attack detection (PAD) has achieved great success under intra-domain settings but easily degrades on unseen domains. Conventional domain generalization methods mitigate the gap by learning domain-invariant features. However, they ignore the discriminative information in the domain-specific features. Moreover, we usually face a more realistic scenario with only one single domain available for training. To tackle the above issues, we propose a Single Domain Dynamic Generalization (SDDG) framework, which simultaneously exploits domain-invariant and domain-specific features on a per-sample basis and learns to generalize to various unseen domains with numerous natural images. Specifically, a dynamic block is designed to adaptively adjust the network with a dynamic adaptor. And an information maximization loss is further combined to increase diversity. The whole network is integrated into the meta-learning paradigm. We generate amplitude perturbed images and cover diverse domains with natural images. Therefore, the network can learn to generalize to the perturbed domains in the meta-test phase. Extensive experiments show the proposed method is effective and outperforms the state-of-the-art on LivDet-Iris 2017 dataset.
10.Label Smarter, Not Harder: CleverLabel for Faster Annotation of Ambiguous Image Classification with Higher Quality
Authors:Lars Schmarje, Vasco Grossmann, Tim Michels, Jakob Nazarenus, Monty Santarossa, Claudius Zelenka, Reinhard Koch
Abstract: High-quality data is crucial for the success of machine learning, but labeling large datasets is often a time-consuming and costly process. While semi-supervised learning can help mitigate the need for labeled data, label quality remains an open issue due to ambiguity and disagreement among annotators. Thus, we use proposal-guided annotations as one option which leads to more consistency between annotators. However, proposing a label increases the probability of the annotators deciding in favor of this specific label. This introduces a bias which we can simulate and remove. We propose a new method CleverLabel for Cost-effective LabEling using Validated proposal-guidEd annotations and Repaired LABELs. CleverLabel can reduce labeling costs by up to 30.0%, while achieving a relative improvement in Kullback-Leibler divergence of up to 29.8% compared to the previous state-of-the-art on a multi-domain real-world image classification benchmark. CleverLabel offers a novel solution to the challenge of efficiently labeling large datasets while also improving the label quality.
11.READMem: Robust Embedding Association for a Diverse Memory in Unconstrained Video Object Segmentation
Authors:Stéphane Vujasinović, Sebastian Bullinger, Stefan Becker, Norbert Scherer-Negenborn, Michael Arens, Rainer Stiefelhagen
Abstract: We present READMem (Robust Embedding Association for a Diverse Memory), a modular framework for semi-automatic video object segmentation (sVOS) methods designed to handle unconstrained videos. Contemporary sVOS works typically aggregate video frames in an ever-expanding memory, demanding high hardware resources for long-term applications. To mitigate memory requirements and prevent near object duplicates (caused by information of adjacent frames), previous methods introduce a hyper-parameter that controls the frequency of frames eligible to be stored. This parameter has to be adjusted according to concrete video properties (such as rapidity of appearance changes and video length) and does not generalize well. Instead, we integrate the embedding of a new frame into the memory only if it increases the diversity of the memory content. Furthermore, we propose a robust association of the embeddings stored in the memory with query embeddings during the update process. Our approach avoids the accumulation of redundant data, allowing us in return, to restrict the memory size and prevent extreme memory demands in long videos. We extend popular sVOS baselines with READMem, which previously showed limited performance on long videos. Our approach achieves competitive results on the Long-time Video dataset (LV1) while not hindering performance on short sequences. Our code is publicly available.
12.Uncertainty-based Detection of Adversarial Attacks in Semantic Segmentation
Authors:Kira Maag, Asja Fischer
Abstract: State-of-the-art deep neural networks have proven to be highly powerful in a broad range of tasks, including semantic image segmentation. However, these networks are vulnerable against adversarial attacks, i.e., non-perceptible perturbations added to the input image causing incorrect predictions, which is hazardous in safety-critical applications like automated driving. Adversarial examples and defense strategies are well studied for the image classification task, while there has been limited research in the context of semantic segmentation. First works however show that the segmentation outcome can be severely distorted by adversarial attacks. In this work, we introduce an uncertainty-based method for the detection of adversarial attacks in semantic segmentation. We observe that uncertainty as for example captured by the entropy of the output distribution behaves differently on clean and perturbed images using this property to distinguish between the two cases. Our method works in a light-weight and post-processing manner, i.e., we do not modify the model or need knowledge of the process used for generating adversarial examples. In a thorough empirical analysis, we demonstrate the ability of our approach to detect perturbed images across multiple types of adversarial attacks.
13.Boosting Long-tailed Object Detection via Step-wise Learning on Smooth-tail Data
Authors:Na Dong, Yongqiang Zhang, Mingli Ding, Gim Hee Lee
Abstract: Real-world data tends to follow a long-tailed distribution, where the class imbalance results in dominance of the head classes during training. In this paper, we propose a frustratingly simple but effective step-wise learning framework to gradually enhance the capability of the model in detecting all categories of long-tailed datasets. Specifically, we build smooth-tail data where the long-tailed distribution of categories decays smoothly to correct the bias towards head classes. We pre-train a model on the whole long-tailed data to preserve discriminability between all categories. We then fine-tune the class-agnostic modules of the pre-trained model on the head class dominant replay data to get a head class expert model with improved decision boundaries from all categories. Finally, we train a unified model on the tail class dominant replay data while transferring knowledge from the head class expert model to ensure accurate detection of all categories. Extensive experiments on long-tailed datasets LVIS v0.5 and LVIS v1.0 demonstrate the superior performance of our method, where we can improve the AP with ResNet-50 backbone from 27.0% to 30.3% AP, and especially for the rare categories from 15.5% to 24.9% AP. Our best model using ResNet-101 backbone can achieve 30.7% AP, which suppresses all existing detectors using the same backbone.
14.Registering Neural Radiance Fields as 3D Density Images
Authors:Han Jiang, Ruoxuan Li, Haosen Sun, Yu-Wing Tai, Chi-Keung Tang
Abstract: No significant work has been done to directly merge two partially overlapping scenes using NeRF representations. Given pre-trained NeRF models of a 3D scene with partial overlapping, this paper aligns them with a rigid transform, by generalizing the traditional registration pipeline, that is, key point detection and point set registration, to operate on 3D density fields. To describe corner points as key points in 3D, we propose to use universal pre-trained descriptor-generating neural networks that can be trained and tested on different scenes. We perform experiments to demonstrate that the descriptor networks can be conveniently trained using a contrastive learning strategy. We demonstrate that our method, as a global approach, can effectively register NeRF models, thus making possible future large-scale NeRF construction by registering its smaller and overlapping NeRFs captured individually.
15.Bright Channel Prior Attention for Multispectral Pedestrian Detection
Authors:Chenhang Cui, Jinyu Xie, Yechenhao Yang
Abstract: Multispectral methods have gained considerable attention due to their promising performance across various fields. However, most existing methods cannot effectively utilize information from two modalities while optimizing time efficiency. These methods often prioritize accuracy or time efficiency, leaving room for improvement in their performance. To this end, we propose a new method bright channel prior attention for enhancing pedestrian detection in low-light conditions by integrating image enhancement and detection within a unified framework. The method uses the V-channel of the HSV image of the thermal image as an attention map to trigger the unsupervised auto-encoder for visible light images, which gradually emphasizes pedestrian features across layers. Moreover, we utilize unsupervised bright channel prior algorithms to address light compensation in low light images. The proposed method includes a self-attention enhancement module and a detection module, which work together to improve object detection. An initial illumination map is estimated using the BCP, guiding the learning of the self-attention map from the enhancement network to obtain more informative representation focused on pedestrians. The extensive experiments show effectiveness of the proposed method is demonstrated through.
16.Cycle Consistency-based Uncertainty Quantification of Neural Networks in Inverse Imaging Problems
Authors:Luzhe Huang, Jianing Li, Xiaofu Ding, Yijie Zhang, Hanlong Chen, Aydogan Ozcan
Abstract: Uncertainty estimation is critical for numerous applications of deep neural networks and draws growing attention from researchers. Here, we demonstrate an uncertainty quantification approach for deep neural networks used in inverse problems based on cycle consistency. We build forward-backward cycles using the physical forward model available and a trained deep neural network solving the inverse problem at hand, and accordingly derive uncertainty estimators through regression analysis on the consistency of these forward-backward cycles. We theoretically analyze cycle consistency metrics and derive their relationship with respect to uncertainty, bias, and robustness of the neural network inference. To demonstrate the effectiveness of these cycle consistency-based uncertainty estimators, we classified corrupted and out-of-distribution input image data using some of the widely used image deblurring and super-resolution neural networks as testbeds. The blind testing of our method outperformed other models in identifying unseen input data corruption and distribution shifts. This work provides a simple-to-implement and rapid uncertainty quantification method that can be universally applied to various neural networks used for solving inverse problems.
17.Real-Aug: Realistic Scene Synthesis for LiDAR Augmentation in 3D Object Detection
Authors:Jinglin Zhan, Tiejun Liu, Rengang Li, Jingwei Zhang, Zhaoxiang Zhang, Yuntao Chen
Abstract: Data and model are the undoubtable two supporting pillars for LiDAR object detection. However, data-centric works have fallen far behind compared with the ever-growing list of fancy new models. In this work, we systematically study the synthesis-based LiDAR data augmentation approach (so-called GT-Aug) which offers maxium controllability over generated data samples. We pinpoint the main shortcoming of existing works is introducing unrealistic LiDAR scan patterns during GT-Aug. In light of this finding, we propose Real-Aug, a synthesis-based augmentation method which prioritizes on generating realistic LiDAR scans. Our method consists a reality-conforming scene composition module which handles the details of the composition and a real-synthesis mixing up training strategy which gradually adapts the data distribution from synthetic data to the real one. To verify the effectiveness of our methods, we conduct extensive ablation studies and validate the proposed Real-Aug on a wide combination of detectors and datasets. We achieve a state-of-the-art 0.744 NDS and 0.702 mAP on nuScenes test set. The code shall be released soon.
18.Towards Benchmarking and Assessing Visual Naturalness of Physical World Adversarial Attacks
Authors:Simin Li, Shuing Zhang, Gujun Chen, Dong Wang, Pu Feng, Jiakai Wang, Aishan Liu, Xin Yi, Xianglong Liu
Abstract: Physical world adversarial attack is a highly practical and threatening attack, which fools real world deep learning systems by generating conspicuous and maliciously crafted real world artifacts. In physical world attacks, evaluating naturalness is highly emphasized since human can easily detect and remove unnatural attacks. However, current studies evaluate naturalness in a case-by-case fashion, which suffers from errors, bias and inconsistencies. In this paper, we take the first step to benchmark and assess visual naturalness of physical world attacks, taking autonomous driving scenario as the first attempt. First, to benchmark attack naturalness, we contribute the first Physical Attack Naturalness (PAN) dataset with human rating and gaze. PAN verifies several insights for the first time: naturalness is (disparately) affected by contextual features (i.e., environmental and semantic variations) and correlates with behavioral feature (i.e., gaze signal). Second, to automatically assess attack naturalness that aligns with human ratings, we further introduce Dual Prior Alignment (DPA) network, which aims to embed human knowledge into model reasoning process. Specifically, DPA imitates human reasoning in naturalness assessment by rating prior alignment and mimics human gaze behavior by attentive prior alignment. We hope our work fosters researches to improve and automatically assess naturalness of physical world attacks. Our code and dataset can be found at https://github.com/zhangsn-19/PAN.
19.Gloss-Free End-to-End Sign Language Translation
Authors:Kezhou Lin, Xiaohan Wang, Linchao Zhu, Ke Sun, Bang Zhang, Yi Yang
Abstract: In this paper, we tackle the problem of sign language translation (SLT) without gloss annotations. Although intermediate representation like gloss has been proven effective, gloss annotations are hard to acquire, especially in large quantities. This limits the domain coverage of translation datasets, thus handicapping real-world applications. To mitigate this problem, we design the Gloss-Free End-to-end sign language translation framework (GloFE). Our method improves the performance of SLT in the gloss-free setting by exploiting the shared underlying semantics of signs and the corresponding spoken translation. Common concepts are extracted from the text and used as a weak form of intermediate representation. The global embedding of these concepts is used as a query for cross-attention to find the corresponding information within the learned visual features. In a contrastive manner, we encourage the similarity of query results between samples containing such concepts and decrease those that do not. We obtained state-of-the-art results on large-scale datasets, including OpenASL and How2Sign. The code and model will be available at https://github.com/HenryLittle/GloFE.
20.Yes, this Way! Learning to Ground Referring Expressions into Actions with Intra-episodic Feedback from Supportive Teachers
Authors:Philipp Sadler, Sherzod Hakimov, David Schlangen
Abstract: The ability to pick up on language signals in an ongoing interaction is crucial for future machine learning models to collaborate and interact with humans naturally. In this paper, we present an initial study that evaluates intra-episodic feedback given in a collaborative setting. We use a referential language game as a controllable example of a task-oriented collaborative joint activity. A teacher utters a referring expression generated by a well-known symbolic algorithm (the "Incremental Algorithm") as an initial instruction and then monitors the follower's actions to possibly intervene with intra-episodic feedback (which does not explicitly have to be requested). We frame this task as a reinforcement learning problem with sparse rewards and learn a follower policy for a heuristic teacher. Our results show that intra-episodic feedback allows the follower to generalize on aspects of scene complexity and performs better than providing only the initial statement.
21.Building an Invisible Shield for Your Portrait against Deepfakes
Authors:Jiazhi Guan, Tianshu Hu, Hang Zhou, Zhizhi Guo, Lirui Deng, Chengbin Quan, Errui Ding, Youjian Zhao
Abstract: The issue of detecting deepfakes has garnered significant attention in the research community, with the goal of identifying facial manipulations for abuse prevention. Although recent studies have focused on developing generalized models that can detect various types of deepfakes, their performance is not always be reliable and stable, which poses limitations in real-world applications. Instead of learning a forgery detector, in this paper, we propose a novel framework - Integrity Encryptor, aiming to protect portraits in a proactive strategy. Our methodology involves covertly encoding messages that are closely associated with key facial attributes into authentic images prior to their public release. Unlike authentic images, where the hidden messages can be extracted with precision, manipulating the facial attributes through deepfake techniques can disrupt the decoding process. Consequently, the modified facial attributes serve as a mean of detecting manipulated images through a comparison of the decoded messages. Our encryption approach is characterized by its brevity and efficiency, and the resulting method exhibits a good robustness against typical image processing traces, such as image degradation and noise. When compared to baselines that struggle to detect deepfakes in a black-box setting, our method utilizing conditional encryption showcases superior performance when presented with a range of different types of forgeries. In experiments conducted on our protected data, our approach outperforms existing state-of-the-art methods by a significant margin.
22.DiffAVA: Personalized Text-to-Audio Generation with Visual Alignment
Authors:Shentong Mo, Jing Shi, Yapeng Tian
Abstract: Text-to-audio (TTA) generation is a recent popular problem that aims to synthesize general audio given text descriptions. Previous methods utilized latent diffusion models to learn audio embedding in a latent space with text embedding as the condition. However, they ignored the synchronization between audio and visual content in the video, and tended to generate audio mismatching from video frames. In this work, we propose a novel and personalized text-to-sound generation approach with visual alignment based on latent diffusion models, namely DiffAVA, that can simply fine-tune lightweight visual-text alignment modules with frozen modality-specific encoders to update visual-aligned text embeddings as the condition. Specifically, our DiffAVA leverages a multi-head attention transformer to aggregate temporal information from video features, and a dual multi-modal residual network to fuse temporal visual representations with text embeddings. Then, a contrastive learning objective is applied to match visual-aligned text embeddings with audio features. Experimental results on the AudioCaps dataset demonstrate that the proposed DiffAVA can achieve competitive performance on visual-aligned text-to-audio generation.
23.BMB: Balanced Memory Bank for Imbalanced Semi-supervised Learning
Authors:Wujian Peng, Zejia Weng, Hengduo Li, Zuxuan Wu
Abstract: Exploring a substantial amount of unlabeled data, semi-supervised learning (SSL) boosts the recognition performance when only a limited number of labels are provided. However, traditional methods assume that the data distribution is class-balanced, which is difficult to achieve in reality due to the long-tailed nature of real-world data. While the data imbalance problem has been extensively studied in supervised learning (SL) paradigms, directly transferring existing approaches to SSL is nontrivial, as prior knowledge about data distribution remains unknown in SSL. In light of this, we propose Balanced Memory Bank (BMB), a semi-supervised framework for long-tailed recognition. The core of BMB is an online-updated memory bank that caches historical features with their corresponding pseudo labels, and the memory is also carefully maintained to ensure the data therein are class-rebalanced. Additionally, an adaptive weighting module is introduced to work jointly with the memory bank so as to further re-calibrate the biased training process. We conduct experiments on multiple datasets and demonstrate, among other things, that BMB surpasses state-of-the-art approaches by clear margins, for example 8.2$\%$ on the 1$\%$ labeled subset of ImageNet127 (with a resolution of 64$\times$64) and 4.3$\%$ on the 50$\%$ labeled subset of ImageNet-LT.
24.Album Storytelling with Iterative Story-aware Captioning and Large Language Models
Authors:Munan Ning, Yujia Xie, Dongdong Chen, Zeyin Song, Lu Yuan, Yonghong Tian, Qixiang Ye, Li Yuan
Abstract: This work studies how to transform an album to vivid and coherent stories, a task we refer to as "album storytelling''. While this task can help preserve memories and facilitate experience sharing, it remains an underexplored area in current literature. With recent advances in Large Language Models (LLMs), it is now possible to generate lengthy, coherent text, opening up the opportunity to develop an AI assistant for album storytelling. One natural approach is to use caption models to describe each photo in the album, and then use LLMs to summarize and rewrite the generated captions into an engaging story. However, we find this often results in stories containing hallucinated information that contradicts the images, as each generated caption ("story-agnostic") is not always about the description related to the whole story or miss some necessary information. To address these limitations, we propose a new iterative album storytelling pipeline. Specifically, we start with an initial story and build a story-aware caption model to refine the captions using the whole story as guidance. The polished captions are then fed into the LLMs to generate a new refined story. This process is repeated iteratively until the story contains minimal factual errors while maintaining coherence. To evaluate our proposed pipeline, we introduce a new dataset of image collections from vlogs and a set of systematic evaluation metrics. Our results demonstrate that our method effectively generates more accurate and engaging stories for albums, with enhanced coherence and vividness.
25.Enhancing Next Active Object-based Egocentric Action Anticipation with Guided Attention
Authors:Sanket Thakur, Cigdem Beyan, Pietro Morerio, Vittorio Murino, Alessio Del Bue
Abstract: Short-term action anticipation (STA) in first-person videos is a challenging task that involves understanding the next active object interactions and predicting future actions. Existing action anticipation methods have primarily focused on utilizing features extracted from video clips, but often overlooked the importance of objects and their interactions. To this end, we propose a novel approach that applies a guided attention mechanism between the objects, and the spatiotemporal features extracted from video clips, enhancing the motion and contextual information, and further decoding the object-centric and motion-centric information to address the problem of STA in egocentric videos. Our method, GANO (Guided Attention for Next active Objects) is a multi-modal, end-to-end, single transformer-based network. The experimental results performed on the largest egocentric dataset demonstrate that GANO outperforms the existing state-of-the-art methods for the prediction of the next active object label, its bounding box location, the corresponding future action, and the time to contact the object. The ablation study shows the positive contribution of the guided attention mechanism compared to other fusion methods. Moreover, it is possible to improve the next active object location and class label prediction results of GANO by just appending the learnable object tokens with the region of interest embeddings.
26.Is Synthetic Data From Diffusion Models Ready for Knowledge Distillation?
Authors:Zheng Li, Yuxuan Li, Penghai Zhao, Renjie Song, Xiang Li, Jian Yang
Abstract: Diffusion models have recently achieved astonishing performance in generating high-fidelity photo-realistic images. Given their huge success, it is still unclear whether synthetic images are applicable for knowledge distillation when real images are unavailable. In this paper, we extensively study whether and how synthetic images produced from state-of-the-art diffusion models can be used for knowledge distillation without access to real images, and obtain three key conclusions: (1) synthetic data from diffusion models can easily lead to state-of-the-art performance among existing synthesis-based distillation methods, (2) low-fidelity synthetic images are better teaching materials, and (3) relatively weak classifiers are better teachers. Code is available at https://github.com/zhengli97/DM-KD.
27.Gated Stereo: Joint Depth Estimation from Gated and Wide-Baseline Active Stereo Cues
Authors:Stefanie Walz, Mario Bijelic, Andrea Ramazzina, Amanpreet Walia, Fahim Mannan, Felix Heide
Abstract: We propose Gated Stereo, a high-resolution and long-range depth estimation technique that operates on active gated stereo images. Using active and high dynamic range passive captures, Gated Stereo exploits multi-view cues alongside time-of-flight intensity cues from active gating. To this end, we propose a depth estimation method with a monocular and stereo depth prediction branch which are combined in a final fusion stage. Each block is supervised through a combination of supervised and gated self-supervision losses. To facilitate training and validation, we acquire a long-range synchronized gated stereo dataset for automotive scenarios. We find that the method achieves an improvement of more than 50 % MAE compared to the next best RGB stereo method, and 74 % MAE to existing monocular gated methods for distances up to 160 m. Our code,models and datasets are available here.
28.Contrastive Predictive Autoencoders for Dynamic Point Cloud Self-Supervised Learning
Authors:Xiaoxiao Sheng, Zhiqiang Shen, Gang Xiao
Abstract: We present a new self-supervised paradigm on point cloud sequence understanding. Inspired by the discriminative and generative self-supervised methods, we design two tasks, namely point cloud sequence based Contrastive Prediction and Reconstruction (CPR), to collaboratively learn more comprehensive spatiotemporal representations. Specifically, dense point cloud segments are first input into an encoder to extract embeddings. All but the last ones are then aggregated by a context-aware autoregressor to make predictions for the last target segment. Towards the goal of modeling multi-granularity structures, local and global contrastive learning are performed between predictions and targets. To further improve the generalization of representations, the predictions are also utilized to reconstruct raw point cloud sequences by a decoder, where point cloud colorization is employed to discriminate against different frames. By combining classic contrast and reconstruction paradigms, it makes the learned representations with both global discrimination and local perception. We conduct experiments on four point cloud sequence benchmarks, and report the results on action recognition and gesture recognition under multiple experimental settings. The performances are comparable with supervised methods and show powerful transferability.
29.Enhanced Meta Label Correction for Coping with Label Corruption
Authors:Mitchell Keren Taraday, Chaim Baskin
Abstract: Traditional methods for learning with the presence of noisy labels have successfully handled datasets with artificially injected noise but still fall short of adequately handling real-world noise. With the increasing use of meta-learning in the diverse fields of machine learning, researchers leveraged auxiliary small clean datasets to meta-correct the training labels. Nonetheless, existing meta-label correction approaches are not fully exploiting their potential. In this study, we propose an Enhanced Meta Label Correction approach abbreviated as EMLC for the learning with noisy labels (LNL) problem. We re-examine the meta-learning process and introduce faster and more accurate meta-gradient derivations. We propose a novel teacher architecture tailored explicitly to the LNL problem, equipped with novel training objectives. EMLC outperforms prior approaches and achieves state-of-the-art results in all standard benchmarks. Notably, EMLC enhances the previous art on the noisy real-world dataset Clothing1M by $1.52\%$ while requiring $\times 0.5$ the time per epoch and with much faster convergence of the meta-objective when compared to the baseline approach.
30.Text-based Person Search without Parallel Image-Text Data
Authors:Yang Bai, Jingyao Wang, Min Cao, Chen Chen, Ziqiang Cao, Liqiang Nie, Min Zhang
Abstract: Text-based person search (TBPS) aims to retrieve the images of the target person from a large image gallery based on a given natural language description. Existing methods are dominated by training models with parallel image-text pairs, which are very costly to collect. In this paper, we make the first attempt to explore TBPS without parallel image-text data ($\mu$-TBPS), in which only non-parallel images and texts, or even image-only data, can be adopted. Towards this end, we propose a two-stage framework, generation-then-retrieval (GTR), to first generate the corresponding pseudo text for each image and then perform the retrieval in a supervised manner. In the generation stage, we propose a fine-grained image captioning strategy to obtain an enriched description of the person image, which firstly utilizes a set of instruction prompts to activate the off-the-shelf pretrained vision-language model to capture and generate fine-grained person attributes, and then converts the extracted attributes into a textual description via the finetuned large language model or the hand-crafted template. In the retrieval stage, considering the noise interference of the generated texts for training model, we develop a confidence score-based training scheme by enabling more reliable texts to contribute more during the training. Experimental results on multiple TBPS benchmarks (i.e., CUHK-PEDES, ICFG-PEDES and RSTPReid) show that the proposed GTR can achieve a promising performance without relying on parallel image-text data.
31.Hierarchical Integration Diffusion Model for Realistic Image Deblurring
Authors:Zheng Chen, Yulun Zhang, Ding Liu, Bin Xia, Jinjin Gu, Linghe Kong, Xin Yuan
Abstract: Diffusion models (DMs) have recently been introduced in image deblurring and exhibited promising performance, particularly in terms of details reconstruction. However, the diffusion model requires a large number of inference iterations to recover the clean image from pure Gaussian noise, which consumes massive computational resources. Moreover, the distribution synthesized by the diffusion model is often misaligned with the target results, leading to restrictions in distortion-based metrics. To address the above issues, we propose the Hierarchical Integration Diffusion Model (HI-Diff), for realistic image deblurring. Specifically, we perform the DM in a highly compacted latent space to generate the prior feature for the deblurring process. The deblurring process is implemented by a regression-based method to obtain better distortion accuracy. Meanwhile, the highly compact latent space ensures the efficiency of the DM. Furthermore, we design the hierarchical integration module to fuse the prior into the regression-based model from multiple scales, enabling better generalization in complex blurry scenarios. Comprehensive experiments on synthetic and real-world blur datasets demonstrate that our HI-Diff outperforms state-of-the-art methods. Code and trained models are available at https://github.com/zhengchen1999/HI-Diff.
32.VanillaNet: the Power of Minimalism in Deep Learning
Authors:Hanting Chen, Yunhe Wang, Jianyuan Guo, Dacheng Tao
Abstract: At the heart of foundation models is the philosophy of "more is different", exemplified by the astonishing success in computer vision and natural language processing. However, the challenges of optimization and inherent complexity of transformer models call for a paradigm shift towards simplicity. In this study, we introduce VanillaNet, a neural network architecture that embraces elegance in design. By avoiding high depth, shortcuts, and intricate operations like self-attention, VanillaNet is refreshingly concise yet remarkably powerful. Each layer is carefully crafted to be compact and straightforward, with nonlinear activation functions pruned after training to restore the original architecture. VanillaNet overcomes the challenges of inherent complexity, making it ideal for resource-constrained environments. Its easy-to-understand and highly simplified architecture opens new possibilities for efficient deployment. Extensive experimentation demonstrates that VanillaNet delivers performance on par with renowned deep neural networks and vision transformers, showcasing the power of minimalism in deep learning. This visionary journey of VanillaNet has significant potential to redefine the landscape and challenge the status quo of foundation model, setting a new path for elegant and effective model design. Pre-trained models and codes are available at https://github.com/huawei-noah/VanillaNet and https://gitee.com/mindspore/models/tree/master/research/cv/VanillaNet.
33.Why current rain denoising models fail on CycleGAN created rain images in autonomous driving
Authors:Michael Kranl, Hubert Ramsauer, Bernhard Knapp
Abstract: One of the main tasks of an autonomous agent in a vehicle is to correctly perceive its environment. Much of the data that needs to be processed is collected by optical sensors such as cameras. Unfortunately, the data collected in this way can be affected by a variety of factors, including environmental influences such as inclement weather conditions (e.g., rain). Such noisy data can cause autonomous agents to take wrong decisions with potentially fatal outcomes. This paper addresses the rain image challenge by two steps: First, rain is artificially added to a set of clear-weather condition images using a Generative Adversarial Network (GAN). This yields good/bad weather image pairs for training de-raining models. This artificial generation of rain images is sufficiently realistic as in 7 out of 10 cases, human test subjects believed the generated rain images to be real. In a second step, this paired good/bad weather image data is used to train two rain denoising models, one based primarily on a Convolutional Neural Network (CNN) and the other using a Vision Transformer. This rain de-noising step showed limited performance as the quality gain was only about 15%. This lack of performance on realistic rain images as used in our study is likely due to current rain de-noising models being developed for simplistic rain overlay data. Our study shows that there is ample space for improvement of de-raining models in autonomous driving.
34.Sparsity and Coefficient Permutation Based Two-Domain AMP for Image Block Compressed Sensing
Authors:Junhui Li, Xingsong Hou, Huake Wang, Shuhao Bi
Abstract: The learned denoising-based approximate message passing (LDAMP) algorithm has attracted great attention for image compressed sensing (CS) tasks. However, it has two issues: first, its global measurement model severely restricts its applicability to high-dimensional images, and its block-based measurement method exhibits obvious block artifacts; second, the denoiser in the LDAMP is too simple, and existing denoisers have limited ability in detail recovery. In this paper, to overcome the issues and develop a high-performance LDAMP method for image block compressed sensing (BCS), we propose a novel sparsity and coefficient permutation-based AMP (SCP-AMP) method consisting of the block-based sampling and the two-domain reconstruction modules. In the sampling module, SCP-AMP adopts a discrete cosine transform (DCT) based sparsity strategy to reduce the impact of the high-frequency coefficient on the reconstruction, followed by a coefficient permutation strategy to avoid block artifacts. In the reconstruction module, a two-domain AMP method with DCT domain noise correction and pixel domain denoising is proposed for iterative reconstruction. Regarding the denoiser, we proposed a multi-level deep attention network (MDANet) to enhance the texture details by employing multi-level features and multiple attention mechanisms. Extensive experiments demonstrated that the proposed SCP-AMP method achieved better reconstruction accuracy than other state-of-the-art BCS algorithms in terms of both visual perception and objective metrics.
35.MFT: Long-Term Tracking of Every Pixel
Authors:Michal Neoral, Jonáš Šerých, Jiří Matas
Abstract: We propose MFT -- Multi-Flow dense Tracker -- a novel method for dense, pixel-level, long-term tracking. The approach exploits optical flows estimated not only between consecutive frames, but also for pairs of frames at logarithmically spaced intervals. It then selects the most reliable sequence of flows on the basis of estimates of its geometric accuracy and the probability of occlusion, both provided by a pre-trained CNN. We show that MFT achieves state-of-the-art results on the TAP-Vid-DAVIS benchmark, outperforming the baselines, their combination, and published methods by a significant margin, achieving an average position accuracy of 70.8%, average Jaccard of 56.1% and average occlusion accuracy of 86.9%. The method is insensitive to medium-length occlusions and it is robustified by estimating flow with respect to the reference frame, which reduces drift.
36.HGFormer: Hierarchical Grouping Transformer for Domain Generalized Semantic Segmentation
Authors:Jian Ding, Nan Xue, Gui-Song Xia, Bernt Schiele, Dengxin Dai
Abstract: Current semantic segmentation models have achieved great success under the independent and identically distributed (i.i.d.) condition. However, in real-world applications, test data might come from a different domain than training data. Therefore, it is important to improve model robustness against domain differences. This work studies semantic segmentation under the domain generalization setting, where a model is trained only on the source domain and tested on the unseen target domain. Existing works show that Vision Transformers are more robust than CNNs and show that this is related to the visual grouping property of self-attention. In this work, we propose a novel hierarchical grouping transformer (HGFormer) to explicitly group pixels to form part-level masks and then whole-level masks. The masks at different scales aim to segment out both parts and a whole of classes. HGFormer combines mask classification results at both scales for class label prediction. We assemble multiple interesting cross-domain settings by using seven public semantic segmentation datasets. Experiments show that HGFormer yields more robust semantic segmentation results than per-pixel classification methods and flat grouping transformers, and outperforms previous methods significantly. Code will be available at https://github.com/dingjiansw101/HGFormer.
37.Getting ViT in Shape: Scaling Laws for Compute-Optimal Model Design
Authors:Ibrahim Alabdulmohsin, Xiaohua Zhai, Alexander Kolesnikov, Lucas Beyer
Abstract: Scaling laws have been recently employed to derive compute-optimal model size (number of parameters) for a given compute duration. We advance and refine such methods to infer compute-optimal model shapes, such as width and depth, and successfully implement this in vision transformers. Our shape-optimized vision transformer, SoViT, achieves results competitive with models that exceed twice its size, despite being pre-trained with an equivalent amount of compute. For example, SoViT-400m/14 achieves 90.3% fine-tuning accuracy on ILSRCV2012, surpassing the much larger ViT-g/14 and approaching ViT-G/14 under identical settings, with also less than half the inference cost. We conduct a thorough evaluation across multiple tasks, such as image classification, captioning, VQA and zero-shot transfer, demonstrating the effectiveness of our model across a broad range of domains and identifying limitations. Overall, our findings challenge the prevailing approach of blindly scaling up vision models and pave a path for a more informed scaling.
38.POEM: Polarization of Embeddings for Domain-Invariant Representations
Authors:Sang-Yeong Jo, Sung Whan Yoon
Abstract: Handling out-of-distribution samples is a long-lasting challenge for deep visual models. In particular, domain generalization (DG) is one of the most relevant tasks that aims to train a model with a generalization capability on novel domains. Most existing DG approaches share the same philosophy to minimize the discrepancy between domains by finding the domain-invariant representations. On the contrary, our proposed method called POEM acquires a strong DG capability by learning domain-invariant and domain-specific representations and polarizing them. Specifically, POEM cotrains category-classifying and domain-classifying embeddings while regularizing them to be orthogonal via minimizing the cosine-similarity between their features, i.e., the polarization of embeddings. The clear separation of embeddings suppresses domain-specific features in the domain-invariant embeddings. The concept of POEM shows a unique direction to enhance the domain robustness of representations that brings considerable and consistent performance gains when combined with existing DG methods. Extensive simulation results in popular DG benchmarks with the PACS, VLCS, OfficeHome, TerraIncognita, and DomainNet datasets show that POEM indeed facilitates the category-classifying embedding to be more domain-invariant.
39.Parallelizing Optical Flow Estimation on an Ultra-Low Power RISC-V Cluster for Nano-UAV Navigation
Authors:Jonas Kühne, Michele Magno, Luca Benini
Abstract: Optical flow estimation is crucial for autonomous navigation and localization of unmanned aerial vehicles (UAV). On micro and nano UAVs, real-time calculation of the optical flow is run on low power and resource-constrained microcontroller units (MCUs). Thus, lightweight algorithms for optical flow have been proposed targeting real-time execution on traditional single-core MCUs. This paper introduces an efficient parallelization strategy for optical flow computation targeting new-generation multicore low power RISC-V based microcontroller units. Our approach enables higher frame rates at lower clock speeds. It has been implemented and evaluated on the eight-core cluster of a commercial octa-core MCU (GAP8) reaching a parallelization speedup factor of 7.21 allowing for a frame rate of 500 frames per second when running on a 50 MHz clock frequency. The proposed parallel algorithm significantly boosts the camera frame rate on micro unmanned aerial vehicles, which enables higher flight speeds: the maximum flight speed can be doubled, while using less than a third of the clock frequency of previous single-core implementations.
40.ControlVideo: Training-free Controllable Text-to-Video Generation
Authors:Yabo Zhang, Yuxiang Wei, Dongsheng Jiang, Xiaopeng Zhang, Wangmeng Zuo, Qi Tian
Abstract: Text-driven diffusion models have unlocked unprecedented abilities in image generation, whereas their video counterpart still lags behind due to the excessive training cost of temporal modeling. Besides the training burden, the generated videos also suffer from appearance inconsistency and structural flickers, especially in long video synthesis. To address these challenges, we design a \emph{training-free} framework called \textbf{ControlVideo} to enable natural and efficient text-to-video generation. ControlVideo, adapted from ControlNet, leverages coarsely structural consistency from input motion sequences, and introduces three modules to improve video generation. Firstly, to ensure appearance coherence between frames, ControlVideo adds fully cross-frame interaction in self-attention modules. Secondly, to mitigate the flicker effect, it introduces an interleaved-frame smoother that employs frame interpolation on alternated frames. Finally, to produce long videos efficiently, it utilizes a hierarchical sampler that separately synthesizes each short clip with holistic coherency. Empowered with these modules, ControlVideo outperforms the state-of-the-arts on extensive motion-prompt pairs quantitatively and qualitatively. Notably, thanks to the efficient designs, it generates both short and long videos within several minutes using one NVIDIA 2080Ti. Code is available at https://github.com/YBYBZhang/ControlVideo.
41.Restore Anything Pipeline: Segment Anything Meets Image Restoration
Authors:Jiaxi Jiang, Christian Holz
Abstract: Recent image restoration methods have produced significant advancements using deep learning. However, existing methods tend to treat the whole image as a single entity, failing to account for the distinct objects in the image that exhibit individual texture properties. Existing methods also typically generate a single result, which may not suit the preferences of different users. In this paper, we introduce the Restore Anything Pipeline (RAP), a novel interactive and per-object level image restoration approach that incorporates a controllable model to generate different results that users may choose from. RAP incorporates image segmentation through the recent Segment Anything Model (SAM) into a controllable image restoration model to create a user-friendly pipeline for several image restoration tasks. We demonstrate the versatility of RAP by applying it to three common image restoration tasks: image deblurring, image denoising, and JPEG artifact removal. Our experiments show that RAP produces superior visual results compared to state-of-the-art methods. RAP represents a promising direction for image restoration, providing users with greater control, and enabling image restoration at an object level.
42.Open-world Semi-supervised Novel Class Discovery
Authors:Jiaming Liu, Yangqiming Wang, Tongze Zhang, Yulu Fan, Qinli Yang, Junming Shao
Abstract: Traditional semi-supervised learning tasks assume that both labeled and unlabeled data follow the same class distribution, but the realistic open-world scenarios are of more complexity with unknown novel classes mixed in the unlabeled set. Therefore, it is of great challenge to not only recognize samples from known classes but also discover the unknown number of novel classes within the unlabeled data. In this paper, we introduce a new open-world semi-supervised novel class discovery approach named OpenNCD, a progressive bi-level contrastive learning method over multiple prototypes. The proposed method is composed of two reciprocally enhanced parts. First, a bi-level contrastive learning method is introduced, which maintains the pair-wise similarity of the prototypes and the prototype group levels for better representation learning. Then, a reliable prototype similarity metric is proposed based on the common representing instances. Prototypes with high similarities will be grouped progressively for known class recognition and novel class discovery. Extensive experiments on three image datasets are conducted and the results show the effectiveness of the proposed method in open-world scenarios, especially with scarce known classes and labels.
43.VLAB: Enhancing Video Language Pre-training by Feature Adapting and Blending
Authors:Xingjian He, Sihan Chen, Fan Ma, Zhicheng Huang, Xiaojie Jin, Zikang Liu, Dongmei Fu, Yi Yang, Jing Liu, Jiashi Feng
Abstract: Large-scale image-text contrastive pre-training models, such as CLIP, have been demonstrated to effectively learn high-quality multimodal representations. However, there is limited research on learning video-text representations for general video multimodal tasks based on these powerful features. Towards this goal, we propose a novel video-text pre-training method dubbed VLAB: Video Language pre-training by feature Adapting and Blending, which transfers CLIP representations to video pre-training tasks and develops unified video multimodal models for a wide range of video-text tasks. Specifically, VLAB is founded on two key strategies: feature adapting and feature blending. In the former, we introduce a new video adapter module to address CLIP's deficiency in modeling temporal information and extend the model's capability to encompass both contrastive and generative tasks. In the latter, we propose an end-to-end training method that further enhances the model's performance by exploiting the complementarity of image and video features. We validate the effectiveness and versatility of VLAB through extensive experiments on highly competitive video multimodal tasks, including video text retrieval, video captioning, and video question answering. Remarkably, VLAB outperforms competing methods significantly and sets new records in video question answering on MSRVTT, MSVD, and TGIF datasets. It achieves an accuracy of 49.6, 61.0, and 79.0, respectively. Codes and models will be released.
44.Semantic-Promoted Debiasing and Background Disambiguation for Zero-Shot Instance Segmentation
Authors:Shuting He, Henghui Ding, Wei Jiang
Abstract: Zero-shot instance segmentation aims to detect and precisely segment objects of unseen categories without any training samples. Since the model is trained on seen categories, there is a strong bias that the model tends to classify all the objects into seen categories. Besides, there is a natural confusion between background and novel objects that have never shown up in training. These two challenges make novel objects hard to be raised in the final instance segmentation results. It is desired to rescue novel objects from background and dominated seen categories. To this end, we propose D$^2$Zero with Semantic-Promoted Debiasing and Background Disambiguation to enhance the performance of Zero-shot instance segmentation. Semantic-promoted debiasing utilizes inter-class semantic relationships to involve unseen categories in visual feature training and learns an input-conditional classifier to conduct dynamical classification based on the input image. Background disambiguation produces image-adaptive background representation to avoid mistaking novel objects for background. Extensive experiments show that we significantly outperform previous state-of-the-art methods by a large margin, e.g., 16.86% improvement on COCO. Project page: https://henghuiding.github.io/D2Zero/
45.Fast Monocular Scene Reconstruction with Global-Sparse Local-Dense Grids
Authors:Wei Dong, Chris Choy, Charles Loop, Or Litany, Yuke Zhu, Anima Anandkumar
Abstract: Indoor scene reconstruction from monocular images has long been sought after by augmented reality and robotics developers. Recent advances in neural field representations and monocular priors have led to remarkable results in scene-level surface reconstructions. The reliance on Multilayer Perceptrons (MLP), however, significantly limits speed in training and rendering. In this work, we propose to directly use signed distance function (SDF) in sparse voxel block grids for fast and accurate scene reconstruction without MLPs. Our globally sparse and locally dense data structure exploits surfaces' spatial sparsity, enables cache-friendly queries, and allows direct extensions to multi-modal data such as color and semantic labels. To apply this representation to monocular scene reconstruction, we develop a scale calibration algorithm for fast geometric initialization from monocular depth priors. We apply differentiable volume rendering from this initialization to refine details with fast convergence. We also introduce efficient high-dimensional Continuous Random Fields (CRFs) to further exploit the semantic-geometry consistency between scene objects. Experiments show that our approach is 10x faster in training and 100x faster in rendering while achieving comparable accuracy to state-of-the-art neural implicit methods.
46.Revisiting Data Augmentation in Model Compression: An Empirical and Comprehensive Study
Authors:Muzhou Yu, Linfeng Zhang, Kaisheng Ma
Abstract: The excellent performance of deep neural networks is usually accompanied by a large number of parameters and computations, which have limited their usage on the resource-limited edge devices. To address this issue, abundant methods such as pruning, quantization and knowledge distillation have been proposed to compress neural networks and achieved significant breakthroughs. However, most of these compression methods focus on the architecture or the training method of neural networks but ignore the influence from data augmentation. In this paper, we revisit the usage of data augmentation in model compression and give a comprehensive study on the relation between model sizes and their optimal data augmentation policy. To sum up, we mainly have the following three observations: (A) Models in different sizes prefer data augmentation with different magnitudes. Hence, in iterative pruning, data augmentation with varying magnitudes leads to better performance than data augmentation with a consistent magnitude. (B) Data augmentation with a high magnitude may significantly improve the performance of large models but harm the performance of small models. Fortunately, small models can still benefit from strong data augmentations by firstly learning them with "additional parameters" and then discard these "additional parameters" during inference. (C) The prediction of a pre-trained large model can be utilized to measure the difficulty of data augmentation. Thus it can be utilized as a criterion to design better data augmentation policies. We hope this paper may promote more research on the usage of data augmentation in model compression.
47.U-TILISE: A Sequence-to-sequence Model for Cloud Removal in Optical Satellite Time Series
Authors:Corinne Stucker, Vivien Sainte Fare Garnot, Konrad Schindler
Abstract: Satellite image time series in the optical and infrared spectrum suffer from frequent data gaps due to cloud cover, cloud shadows, and temporary sensor outages. It has been a long-standing problem of remote sensing research how to best reconstruct the missing pixel values and obtain complete, cloud-free image sequences. We approach that problem from the perspective of representation learning and develop U-TILISE, an efficient neural model that is able to implicitly capture spatio-temporal patterns of the spectral intensities, and that can therefore be trained to map a cloud-masked input sequence to a cloud-free output sequence. The model consists of a convolutional spatial encoder that maps each individual frame of the input sequence to a latent encoding; an attention-based temporal encoder that captures dependencies between those per-frame encodings and lets them exchange information along the time dimension; and a convolutional spatial decoder that decodes the latent embeddings back into multi-spectral images. We experimentally evaluate the proposed model on EarthNet2021, a dataset of Sentinel-2 time series acquired all over Europe, and demonstrate its superior ability to reconstruct the missing pixels. Compared to a standard interpolation baseline, it increases the PSNR by 1.8 dB at previously seen locations and by 1.3 dB at unseen locations.
48.Target-Aware Generative Augmentations for Single-Shot Adaptation
Authors:Kowshik Thopalli, Rakshith Subramanyam, Pavan Turaga, Jayaraman J. Thiagarajan
Abstract: In this paper, we address the problem of adapting models from a source domain to a target domain, a task that has become increasingly important due to the brittle generalization of deep neural networks. While several test-time adaptation techniques have emerged, they typically rely on synthetic toolbox data augmentations in cases of limited target data availability. We consider the challenging setting of single-shot adaptation and explore the design of augmentation strategies. We argue that augmentations utilized by existing methods are insufficient to handle large distribution shifts, and hence propose a new approach SiSTA, which first fine-tunes a generative model from the source domain using a single-shot target, and then employs novel sampling strategies for curating synthetic target data. Using experiments on a variety of benchmarks, distribution shifts and image corruptions, we find that SiSTA produces significantly improved generalization over existing baselines in face attribute detection and multi-class object recognition. Furthermore, SiSTA performs competitively to models obtained by training on larger target datasets. Our codes can be accessed at https://github.com/Rakshith-2905/SiSTA.
49.Materialistic: Selecting Similar Materials in Images
Authors:Prafull Sharma, Julien Philip, Michaël Gharbi, William T. Freeman, Fredo Durand, Valentin Deschaintre
Abstract: Separating an image into meaningful underlying components is a crucial first step for both editing and understanding images. We present a method capable of selecting the regions of a photograph exhibiting the same material as an artist-chosen area. Our proposed approach is robust to shading, specular highlights, and cast shadows, enabling selection in real images. As we do not rely on semantic segmentation (different woods or metal should not be selected together), we formulate the problem as a similarity-based grouping problem based on a user-provided image location. In particular, we propose to leverage the unsupervised DINO features coupled with a proposed Cross-Similarity module and an MLP head to extract material similarities in an image. We train our model on a new synthetic image dataset, that we release. We show that our method generalizes well to real-world images. We carefully analyze our model's behavior on varying material properties and lighting. Additionally, we evaluate it against a hand-annotated benchmark of 50 real photographs. We further demonstrate our model on a set of applications, including material editing, in-video selection, and retrieval of object photographs with similar materials.
50.VideoLLM: Modeling Video Sequence with Large Language Models
Authors:Guo Chen, Yin-Dong Zheng, Jiahao Wang, Jilan Xu, Yifei Huang, Junting Pan, Yi Wang, Yali Wang, Yu Qiao, Tong Lu, Limin Wang
Abstract: With the exponential growth of video data, there is an urgent need for automated technology to analyze and comprehend video content. However, existing video understanding models are often task-specific and lack a comprehensive capability of handling diverse tasks. The success of large language models (LLMs) like GPT has demonstrated their impressive abilities in sequence causal reasoning. Building upon this insight, we propose a novel framework called VideoLLM that leverages the sequence reasoning capabilities of pre-trained LLMs from natural language processing (NLP) for video sequence understanding. VideoLLM incorporates a carefully designed Modality Encoder and Semantic Translator, which convert inputs from various modalities into a unified token sequence. This token sequence is then fed into a decoder-only LLM. Subsequently, with the aid of a simple task head, our VideoLLM yields an effective unified framework for different kinds of video understanding tasks. To evaluate the efficacy of VideoLLM, we conduct extensive experiments using multiple LLMs and fine-tuning methods. We evaluate our VideoLLM on eight tasks sourced from four different datasets. The experimental results demonstrate that the understanding and reasoning capabilities of LLMs can be effectively transferred to video understanding tasks.
51.NeRFuser: Large-Scale Scene Representation by NeRF Fusion
Authors:Jiading Fang, Shengjie Lin, Igor Vasiljevic, Vitor Guizilini, Rares Ambrus, Adrien Gaidon, Gregory Shakhnarovich, Matthew R. Walter
Abstract: A practical benefit of implicit visual representations like Neural Radiance Fields (NeRFs) is their memory efficiency: large scenes can be efficiently stored and shared as small neural nets instead of collections of images. However, operating on these implicit visual data structures requires extending classical image-based vision techniques (e.g., registration, blending) from image sets to neural fields. Towards this goal, we propose NeRFuser, a novel architecture for NeRF registration and blending that assumes only access to pre-generated NeRFs, and not the potentially large sets of images used to generate them. We propose registration from re-rendering, a technique to infer the transformation between NeRFs based on images synthesized from individual NeRFs. For blending, we propose sample-based inverse distance weighting to blend visual information at the ray-sample level. We evaluate NeRFuser on public benchmarks and a self-collected object-centric indoor dataset, showing the robustness of our method, including to views that are challenging to render from the individual source NeRFs.
52.If at First You Don't Succeed, Try, Try Again: Faithful Diffusion-based Text-to-Image Generation by Selection
Authors:Shyamgopal Karthik, Karsten Roth, Massimiliano Mancini, Zeynep Akata
Abstract: Despite their impressive capabilities, diffusion-based text-to-image (T2I) models can lack faithfulness to the text prompt, where generated images may not contain all the mentioned objects, attributes or relations. To alleviate these issues, recent works proposed post-hoc methods to improve model faithfulness without costly retraining, by modifying how the model utilizes the input prompt. In this work, we take a step back and show that large T2I diffusion models are more faithful than usually assumed, and can generate images faithful to even complex prompts without the need to manipulate the generative process. Based on that, we show how faithfulness can be simply treated as a candidate selection problem instead, and introduce a straightforward pipeline that generates candidate images for a text prompt and picks the best one according to an automatic scoring system that can leverage already existing T2I evaluation metrics. Quantitative comparisons alongside user studies on diverse benchmarks show consistently improved faithfulness over post-hoc enhancement methods, with comparable or lower computational cost. Code is available at \url{https://github.com/ExplainableML/ImageSelect}.
53.Matcher: Segment Anything with One Shot Using All-Purpose Feature Matching
Authors:Yang Liu, Muzhi Zhu, Hengtao Li, Hao Chen, Xinlong Wang, Chunhua Shen
Abstract: Powered by large-scale pre-training, vision foundation models exhibit significant potential in open-world image understanding. Even though individual models have limited capabilities, combining multiple such models properly can lead to positive synergies and unleash their full potential. In this work, we present Matcher, which segments anything with one shot by integrating an all-purpose feature extraction model and a class-agnostic segmentation model. Naively connecting the models results in unsatisfying performance, e.g., the models tend to generate matching outliers and false-positive mask fragments. To address these issues, we design a bidirectional matching strategy for accurate cross-image semantic dense matching and a robust prompt sampler for mask proposal generation. In addition, we propose a novel instance-level matching strategy for controllable mask merging. The proposed Matcher method delivers impressive generalization performance across various segmentation tasks, all without training. For example, it achieves 52.7% mIoU on COCO-20$^i$ for one-shot semantic segmentation, surpassing the state-of-the-art specialist model by 1.6%. In addition, our visualization results show open-world generality and flexibility on images in the wild. The code shall be released at https://github.com/aim-uofa/Matcher.
54.VDT: An Empirical Study on Video Diffusion with Transformers
Authors:Haoyu Lu, Guoxing Yang, Nanyi Fei, Yuqi Huo, Zhiwu Lu, Ping Luo, Mingyu Ding
Abstract: This work introduces Video Diffusion Transformer (VDT), which pioneers the use of transformers in diffusion-based video generation. It features transformer blocks with modularized temporal and spatial attention modules, allowing separate optimization of each component and leveraging the rich spatial-temporal representation inherited from transformers. VDT offers several appealing benefits. 1) It excels at capturing temporal dependencies to produce temporally consistent video frames and even simulate the dynamics of 3D objects over time. 2) It enables flexible conditioning information through simple concatenation in the token space, effectively unifying video generation and prediction tasks. 3) Its modularized design facilitates a spatial-temporal decoupled training strategy, leading to improved efficiency. Extensive experiments on video generation, prediction, and dynamics modeling (i.e., physics-based QA) tasks have been conducted to demonstrate the effectiveness of VDT in various scenarios, including autonomous driving, human action, and physics-based simulation. We hope our study on the capabilities of transformer-based video diffusion in capturing accurate temporal dependencies, handling conditioning information, and achieving efficient training will benefit future research and advance the field. Codes and models are available at https://github.com/RERV/VDT.
55.Contextualising Implicit Representations for Semantic Tasks
Authors:Theo W. Costain, Kejie Li, Victor A. Prisacariu
Abstract: Prior works have demonstrated that implicit representations trained only for reconstruction tasks typically generate encodings that are not useful for semantic tasks. In this work, we propose a method that contextualises the encodings of implicit representations, enabling their use in downstream tasks (e.g. semantic segmentation), without requiring access to the original training data or encoding network. Using an implicit representation trained for a reconstruction task alone, our contextualising module takes an encoding trained for reconstruction only and reveals meaningful semantic information that is hidden in the encodings, without compromising the reconstruction performance. With our proposed module, it becomes possible to pre-train implicit representations on larger datasets, improving their reconstruction performance compared to training on only a smaller labelled dataset, whilst maintaining their segmentation performance on the labelled dataset. Importantly, our method allows for future foundation implicit representation models to be fine-tuned on unseen tasks, regardless of encoder or dataset availability.