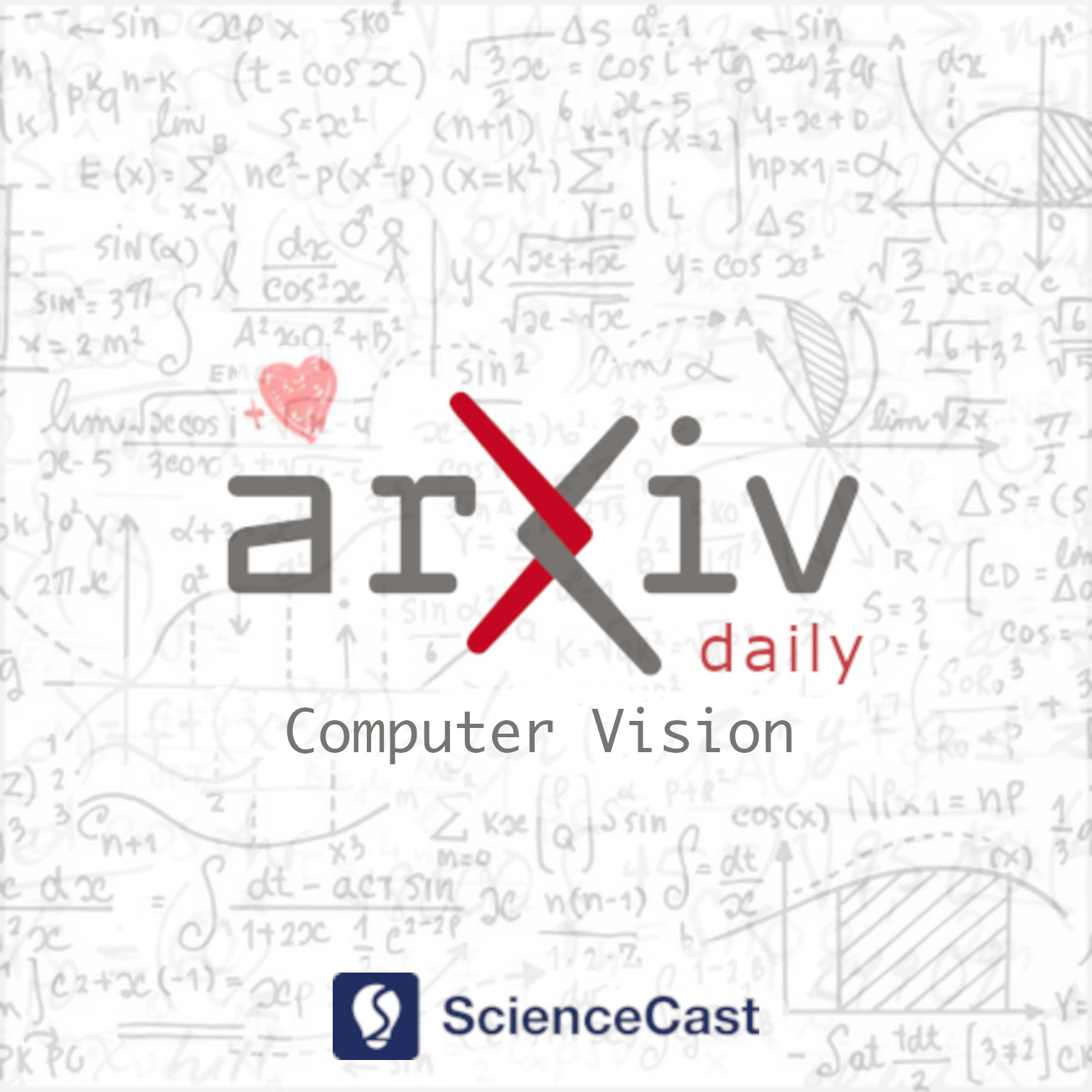
Computer Vision and Pattern Recognition (cs.CV)
Fri, 12 May 2023
1.A Central Asian Food Dataset for Personalized Dietary Interventions, Extended Abstract
Authors:Aknur Karabay, Arman Bolatov, Huseyin Atakan Varol, Mei-Yen Chan
Abstract: Nowadays, it is common for people to take photographs of every beverage, snack, or meal they eat and then post these photographs on social media platforms. Leveraging these social trends, real-time food recognition and reliable classification of these captured food images can potentially help replace some of the tedious recording and coding of food diaries to enable personalized dietary interventions. Although Central Asian cuisine is culturally and historically distinct, there has been little published data on the food and dietary habits of people in this region. To fill this gap, we aim to create a reliable dataset of regional foods that is easily accessible to both public consumers and researchers. To the best of our knowledge, this is the first work on creating a Central Asian Food Dataset (CAFD). The final dataset contains 42 food categories and over 16,000 images of national dishes unique to this region. We achieved a classification accuracy of 88.70\% (42 classes) on the CAFD using the ResNet152 neural network model. The food recognition models trained on the CAFD demonstrate computer vision's effectiveness and high accuracy for dietary assessment.
2.Meta-Optimization for Higher Model Generalizability in Single-Image Depth Prediction
Authors:Cho-Ying Wu, Yiqi Zhong, Junying Wang, Ulrich Neumann
Abstract: Model generalizability to unseen datasets, concerned with in-the-wild robustness, is less studied for indoor single-image depth prediction. We leverage gradient-based meta-learning for higher generalizability on zero-shot cross-dataset inference. Unlike the most-studied image classification in meta-learning, depth is pixel-level continuous range values, and mappings from each image to depth vary widely across environments. Thus no explicit task boundaries exist. We instead propose fine-grained task that treats each RGB-D pair as a task in our meta-optimization. We first show meta-learning on limited data induces much better prior (max +29.4\%). Using meta-learned weights as initialization for following supervised learning, without involving extra data or information, it consistently outperforms baselines without the method. Compared to most indoor-depth methods that only train/ test on a single dataset, we propose zero-shot cross-dataset protocols, closely evaluate robustness, and show consistently higher generalizability and accuracy by our meta-initialization. The work at the intersection of depth and meta-learning potentially drives both research streams to step closer to practical use.
3.SSD-MonoDTR: Supervised Scale-constrained Deformable Transformer for Monocular 3D Object Detection
Authors:Xuan He, Fan Yang, Jiacheng Lin, Haolong Fu, Jin Yuan, Kailun Yang, Zhiyong Li
Abstract: Transformer-based methods have demonstrated superior performance for monocular 3D object detection recently, which predicts 3D attributes from a single 2D image. Most existing transformer-based methods leverage visual and depth representations to explore valuable query points on objects, and the quality of the learned queries has a great impact on detection accuracy. Unfortunately, existing unsupervised attention mechanisms in transformer are prone to generate low-quality query features due to inaccurate receptive fields, especially on hard objects. To tackle this problem, this paper proposes a novel ``Supervised Scale-constrained Deformable Attention'' (SSDA) for monocular 3D object detection. Specifically, SSDA presets several masks with different scales and utilizes depth and visual features to predict the local feature for each query. Imposing the scale constraint, SSDA could well predict the accurate receptive field of a query to support robust query feature generation. What is more, SSDA is assigned with a Weighted Scale Matching (WSM) loss to supervise scale prediction, which presents more confident results as compared to the unsupervised attention mechanisms. Extensive experiments on ``KITTI'' demonstrate that SSDA significantly improves the detection accuracy especially on moderate and hard objects, yielding SOTA performance as compared to the existing approaches. Code will be publicly available at https://github.com/mikasa3lili/SSD-MonoDETR.
4.Quaternion-valued Correlation Learning for Few-Shot Semantic Segmentation
Authors:Zewen Zheng, Guoheng Huang, Xiaochen Yuan, Chi-Man Pun, Hongrui Liu, Wing-Kuen Ling
Abstract: Few-shot segmentation (FSS) aims to segment unseen classes given only a few annotated samples. Encouraging progress has been made for FSS by leveraging semantic features learned from base classes with sufficient training samples to represent novel classes. The correlation-based methods lack the ability to consider interaction of the two subspace matching scores due to the inherent nature of the real-valued 2D convolutions. In this paper, we introduce a quaternion perspective on correlation learning and propose a novel Quaternion-valued Correlation Learning Network (QCLNet), with the aim to alleviate the computational burden of high-dimensional correlation tensor and explore internal latent interaction between query and support images by leveraging operations defined by the established quaternion algebra. Specifically, our QCLNet is formulated as a hyper-complex valued network and represents correlation tensors in the quaternion domain, which uses quaternion-valued convolution to explore the external relations of query subspace when considering the hidden relationship of the support sub-dimension in the quaternion space. Extensive experiments on the PASCAL-5i and COCO-20i datasets demonstrate that our method outperforms the existing state-of-the-art methods effectively. Our code is available at https://github.com/zwzheng98/QCLNet
5.The 3rd Anti-UAV Workshop & Challenge: Methods and Results
Authors:Jian Zhao, Jianan Li, Lei Jin, Jiaming Chu, Zhihao Zhang, Jun Wang, Jiangqiang Xia, Kai Wang, Yang Liu, Sadaf Gulshad, Jiaojiao Zhao, Tianyang Xu, Xuefeng Zhu, Shihan Liu, Zheng Zhu, Guibo Zhu, Zechao Li, Zheng Wang, Baigui Sun, Yandong Guo, Shin ichi Satoh, Junliang Xing, Jane Shen Shengmei
Abstract: The 3rd Anti-UAV Workshop & Challenge aims to encourage research in developing novel and accurate methods for multi-scale object tracking. The Anti-UAV dataset used for the Anti-UAV Challenge has been publicly released. There are two main differences between this year's competition and the previous two. First, we have expanded the existing dataset, and for the first time, released a training set so that participants can focus on improving their models. Second, we set up two tracks for the first time, i.e., Anti-UAV Tracking and Anti-UAV Detection & Tracking. Around 76 participating teams from the globe competed in the 3rd Anti-UAV Challenge. In this paper, we provide a brief summary of the 3rd Anti-UAV Workshop & Challenge including brief introductions to the top three methods in each track. The submission leaderboard will be reopened for researchers that are interested in the Anti-UAV challenge. The benchmark dataset and other information can be found at: https://anti-uav.github.io/.
6.CLIP-Count: Towards Text-Guided Zero-Shot Object Counting
Authors:Ruixiang Jiang, Lingbo Liu, Changwen Chen
Abstract: Recent advances in visual-language models have shown remarkable zero-shot text-image matching ability that is transferable to down-stream tasks such as object detection and segmentation. However, adapting these models for object counting, which involves estimating the number of objects in an image, remains a formidable challenge. In this study, we conduct the first exploration of transferring visual-language models for class-agnostic object counting. Specifically, we propose CLIP-Count, a novel pipeline that estimates density maps for open-vocabulary objects with text guidance in a zero-shot manner, without requiring any finetuning on specific object classes. To align the text embedding with dense image features, we introduce a patch-text contrastive loss that guides the model to learn informative patch-level image representations for dense prediction. Moreover, we design a hierarchical patch-text interaction module that propagates semantic information across different resolution levels of image features. Benefiting from the full exploitation of the rich image-text alignment knowledge of pretrained visual-language models, our method effectively generates high-quality density maps for objects-of-interest. Extensive experiments on FSC-147, CARPK, and ShanghaiTech crowd counting datasets demonstrate that our proposed method achieves state-of-the-art accuracy and generalizability for zero-shot object counting. Project page at https://github.com/songrise/CLIP-Count
7.Self-Learning Symmetric Multi-view Probabilistic Clustering
Authors:Junjie Liu, Junlong Liu, Rongxin Jiang, Xuesong Liu, Boxuan Gu, Yaowu Chen, Chen Shen, Jieping Ye
Abstract: Multi-view Clustering (MVC) has achieved significant progress, with many efforts dedicated to learn knowledge from multiple views. However, most existing methods are either not applicable or require additional steps for incomplete multi-view clustering. Such a limitation results in poor-quality clustering performance and poor missing view adaptation. Besides, noise or outliers might significantly degrade the overall clustering performance, which are not handled well by most existing methods. Moreover, category information is required in most existing methods, which severely affects the clustering performance. In this paper, we propose a novel unified framework for incomplete and complete MVC named self-learning symmetric multi-view probabilistic clustering (SLS-MPC). SLS-MPC proposes a novel symmetric multi-view probability estimation and equivalently transforms multi-view pairwise posterior matching probability into composition of each view's individual distribution, which tolerates data missing and might extend to any number of views. Then, SLS-MPC proposes a novel self-learning probability function without any prior knowledge and hyper-parameters to learn each view's individual distribution from the aspect of consistency in single-view, cross-view and multi-view. Next, graph-context-aware refinement with path propagation and co-neighbor propagation is used to refine pairwise probability, which alleviates the impact of noise and outliers. Finally, SLS-MPC proposes a probabilistic clustering algorithm to adjust clustering assignments by maximizing the joint probability iteratively, in which category information is not required. Extensive experiments on multiple benchmarks for incomplete and complete MVC show that SLS-MPC significantly outperforms previous state-of-the-art methods.
8.Efficient Search of Comprehensively Robust Neural Architectures via Multi-fidelity Evaluation
Authors:Jialiang Sun, Wen Yao, Tingsong Jiang, Xiaoqian Chen
Abstract: Neural architecture search (NAS) has emerged as one successful technique to find robust deep neural network (DNN) architectures. However, most existing robustness evaluations in NAS only consider $l_{\infty}$ norm-based adversarial noises. In order to improve the robustness of DNN models against multiple types of noises, it is necessary to consider a comprehensive evaluation in NAS for robust architectures. But with the increasing number of types of robustness evaluations, it also becomes more time-consuming to find comprehensively robust architectures. To alleviate this problem, we propose a novel efficient search of comprehensively robust neural architectures via multi-fidelity evaluation (ES-CRNA-ME). Specifically, we first search for comprehensively robust architectures under multiple types of evaluations using the weight-sharing-based NAS method, including different $l_{p}$ norm attacks, semantic adversarial attacks, and composite adversarial attacks. In addition, we reduce the number of robustness evaluations by the correlation analysis, which can incorporate similar evaluations and decrease the evaluation cost. Finally, we propose a multi-fidelity online surrogate during optimization to further decrease the search cost. On the basis of the surrogate constructed by low-fidelity data, the online high-fidelity data is utilized to finetune the surrogate. Experiments on CIFAR10 and CIFAR100 datasets show the effectiveness of our proposed method.
9.Configurable Spatial-Temporal Hierarchical Analysis for Flexible Video Anomaly Detection
Authors:Kai Cheng, Xinhua Zeng, Yang Liu, Tian Wang, Chengxin Pang, Jing Teng, Zhaoyang Xia, Jing Liu
Abstract: Video anomaly detection (VAD) is a vital task with great practical applications in industrial surveillance, security system, and traffic control. Unlike previous unsupervised VAD methods that adopt a fixed structure to learn normality without considering different detection demands, we design a spatial-temporal hierarchical architecture (STHA) as a configurable architecture to flexibly detect different degrees of anomaly. The comprehensive structure of the STHA is delineated into a tripartite hierarchy, encompassing the following tiers: the stream level, the stack level, and the block level. Specifically, we design several auto-encoder-based blocks that possess varying capacities for extracting normal patterns. Then, we stack blocks according to the complexity degrees with both intra-stack and inter-stack residual links to learn hierarchical normality gradually. Considering the multisource knowledge of videos, we also model the spatial normality of video frames and temporal normality of RGB difference by designing two parallel streams consisting of stacks. Thus, STHA can provide various representation learning abilities by expanding or contracting hierarchically to detect anomalies of different degrees. Since the anomaly set is complicated and unbounded, our STHA can adjust its detection ability to adapt to the human detection demands and the complexity degree of anomaly that happened in the history of a scene. We conduct experiments on three benchmarks and perform extensive analysis, and the results demonstrate that our method performs comparablely to the state-of-the-art methods. In addition, we design a toy dataset to prove that our model can better balance the learning ability to adapt to different detection demands.
10.MotionBEV: Attention-Aware Online LiDAR Moving Object Segmentation with Bird's Eye View based Appearance and Motion Features
Authors:Bo Zhou, Jiapeng Xie, Yan Pan, Jiajie Wu, Chuanzhao Lu
Abstract: Identifying moving objects is an essential capability for autonomous systems, as it provides critical information for pose estimation, navigation, collision avoidance and static map construction. In this paper, we present MotionBEV, a fast and accurate framework for LiDAR moving object segmentation, which segments moving objects with appearance and motion features in bird's eye view (BEV) domain. Our approach converts 3D LiDAR scans into 2D polar BEV representation to achieve real-time performance. Specifically, we learn appearance features with a simplified PointNet, and compute motion features through the height differences of consecutive frames of point clouds projected onto vertical columns in the polar BEV coordinate system. We employ a dual-branch network bridged by the Appearance-Motion Co-attention Module (AMCM) to adaptively fuse the spatio-temporal information from appearance and motion features. Our approach achieves state-of-the-art performance on the SemanticKITTI-MOS benchmark, with an average inference time of 23ms on an RTX 3090 GPU. Furthermore, to demonstrate the practical effectiveness of our method, we provide a LiDAR-MOS dataset recorded by a solid-state LiDAR, which features non-repetitive scanning patterns and small field of view.
11.BundleRecon: Ray Bundle-Based 3D Neural Reconstruction
Authors:Weikun Zhang, Jianke Zhu
Abstract: With the growing popularity of neural rendering, there has been an increasing number of neural implicit multi-view reconstruction methods. While many models have been enhanced in terms of positional encoding, sampling, rendering, and other aspects to improve the reconstruction quality, current methods do not fully leverage the information among neighboring pixels during the reconstruction process. To address this issue, we propose an enhanced model called BundleRecon. In the existing approaches, sampling is performed by a single ray that corresponds to a single pixel. In contrast, our model samples a patch of pixels using a bundle of rays, which incorporates information from neighboring pixels. Furthermore, we design bundle-based constraints to further improve the reconstruction quality. Experimental results demonstrate that BundleRecon is compatible with the existing neural implicit multi-view reconstruction methods and can improve their reconstruction quality.
12.A Survey on Deep Learning-Based Monocular Spacecraft Pose Estimation: Current State, Limitations and Prospects
Authors:Leo Pauly, Wassim Rharbaoui, Carl Shneider, Arunkumar Rathinam, Vincent Gaudilliere, Djamila Aouada
Abstract: Estimating the pose of an uncooperative spacecraft is an important computer vision problem for enabling the deployment of automatic vision-based systems in orbit, with applications ranging from on-orbit servicing to space debris removal. Following the general trend in computer vision, more and more works have been focusing on leveraging Deep Learning (DL) methods to address this problem. However and despite promising research-stage results, major challenges preventing the use of such methods in real-life missions still stand in the way. In particular, the deployment of such computation-intensive algorithms is still under-investigated, while the performance drop when training on synthetic and testing on real images remains to mitigate. The primary goal of this survey is to describe the current DL-based methods for spacecraft pose estimation in a comprehensive manner. The secondary goal is to help define the limitations towards the effective deployment of DL-based spacecraft pose estimation solutions for reliable autonomous vision-based applications. To this end, the survey first summarises the existing algorithms according to two approaches: hybrid modular pipelines and direct end-to-end regression methods. A comparison of algorithms is presented not only in terms of pose accuracy but also with a focus on network architectures and models' sizes keeping potential deployment in mind. Then, current monocular spacecraft pose estimation datasets used to train and test these methods are discussed. The data generation methods: simulators and testbeds, the domain gap and the performance drop between synthetically generated and lab/space collected images and the potential solutions are also discussed. Finally, the paper presents open research questions and future directions in the field, drawing parallels with other computer vision applications.
13.Learning Monocular Depth in Dynamic Environment via Context-aware Temporal Attention
Authors:Zizhang Wu, Zhuozheng Li, Zhi-Gang Fan, Yunzhe Wu, Yuanzhu Gan, Jian Pu, Xianzhi Li
Abstract: The monocular depth estimation task has recently revealed encouraging prospects, especially for the autonomous driving task. To tackle the ill-posed problem of 3D geometric reasoning from 2D monocular images, multi-frame monocular methods are developed to leverage the perspective correlation information from sequential temporal frames. However, moving objects such as cars and trains usually violate the static scene assumption, leading to feature inconsistency deviation and misaligned cost values, which would mislead the optimization algorithm. In this work, we present CTA-Depth, a Context-aware Temporal Attention guided network for multi-frame monocular Depth estimation. Specifically, we first apply a multi-level attention enhancement module to integrate multi-level image features to obtain an initial depth and pose estimation. Then the proposed CTA-Refiner is adopted to alternatively optimize the depth and pose. During the refinement process, context-aware temporal attention (CTA) is developed to capture the global temporal-context correlations to maintain the feature consistency and estimation integrity of moving objects. In particular, we propose a long-range geometry embedding (LGE) module to produce a long-range temporal geometry prior. Our approach achieves significant improvements over state-of-the-art approaches on three benchmark datasets.
14.Gallery Sampling for Robust and Fast Face Identification
Authors:Myung-cheol Roh, Pyoung-gang Lim, Jongju Shin
Abstract: Deep learning methods have been achieved brilliant results in face recognition. One of the important tasks to improve the performance is to collect and label images as many as possible. However, labeling identities and checking qualities of large image data are difficult task and mistakes cannot be avoided in processing large data. Previous works have been trying to deal with the problem only in training domain, however it can cause much serious problem if the mistakes are in gallery data of face identification. We proposed gallery data sampling methods which are robust to outliers including wrong labeled, low quality, and less-informative images and reduce searching time. The proposed sampling-by-pruning and sampling-by-generating methods significantly improved face identification performance on our 5.4M web image dataset of celebrities. The proposed method achieved 0.0975 in terms of FNIR at FPIR=0.01, while conventional method showed 0.3891. The average number of feature vectors for each individual gallery was reduced to 17.1 from 115.9 and it can provide much faster search. We also made experiments on public datasets and our method achieved 0.1314 and 0.0668 FNIRs at FPIR=0.01 on the CASIA-WebFace and MS1MV2, while the convectional method did 0.5446, and 0.1327, respectively.
15.Visual Information Extraction in the Wild: Practical Dataset and End-to-end Solution
Authors:Jianfeng Kuang, Wei Hua, Dingkang Liang, Mingkun Yang, Deqiang Jiang, Bo Ren, Yu Zhou, Xiang Bai
Abstract: Visual information extraction (VIE), which aims to simultaneously perform OCR and information extraction in a unified framework, has drawn increasing attention due to its essential role in various applications like understanding receipts, goods, and traffic signs. However, as existing benchmark datasets for VIE mainly consist of document images without the adequate diversity of layout structures, background disturbs, and entity categories, they cannot fully reveal the challenges of real-world applications. In this paper, we propose a large-scale dataset consisting of camera images for VIE, which contains not only the larger variance of layout, backgrounds, and fonts but also much more types of entities. Besides, we propose a novel framework for end-to-end VIE that combines the stages of OCR and information extraction in an end-to-end learning fashion. Different from the previous end-to-end approaches that directly adopt OCR features as the input of an information extraction module, we propose to use contrastive learning to narrow the semantic gap caused by the difference between the tasks of OCR and information extraction. We evaluate the existing end-to-end methods for VIE on the proposed dataset and observe that the performance of these methods has a distinguishable drop from SROIE (a widely used English dataset) to our proposed dataset due to the larger variance of layout and entities. These results demonstrate our dataset is more practical for promoting advanced VIE algorithms. In addition, experiments demonstrate that the proposed VIE method consistently achieves the obvious performance gains on the proposed and SROIE datasets.
16.BlendFields: Few-Shot Example-Driven Facial Modeling
Authors:Kacper Kania, Stephan J. Garbin, Andrea Tagliasacchi, Virginia Estellers, Kwang Moo Yi, Julien Valentin, Tomasz Trzciński, Marek Kowalski
Abstract: Generating faithful visualizations of human faces requires capturing both coarse and fine-level details of the face geometry and appearance. Existing methods are either data-driven, requiring an extensive corpus of data not publicly accessible to the research community, or fail to capture fine details because they rely on geometric face models that cannot represent fine-grained details in texture with a mesh discretization and linear deformation designed to model only a coarse face geometry. We introduce a method that bridges this gap by drawing inspiration from traditional computer graphics techniques. Unseen expressions are modeled by blending appearance from a sparse set of extreme poses. This blending is performed by measuring local volumetric changes in those expressions and locally reproducing their appearance whenever a similar expression is performed at test time. We show that our method generalizes to unseen expressions, adding fine-grained effects on top of smooth volumetric deformations of a face, and demonstrate how it generalizes beyond faces.
17.HFLIC: Human Friendly Perceptual Learned Image Compression with Reinforced Transform
Authors:Peirong Ning, Wei Jiang, Ronggang Wang
Abstract: In recent years, there has been rapid development in learned image compression techniques that prioritize ratedistortion-perceptual compression, preserving fine details even at lower bit-rates. However, current learning-based image compression methods often sacrifice human-friendly compression and require long decoding times. In this paper, we propose enhancements to the backbone network and loss function of existing image compression model, focusing on improving human perception and efficiency. Our proposed approach achieves competitive subjective results compared to state-of-the-art end-to-end learned image compression methods and classic methods, while requiring less decoding time and offering human-friendly compression. Through empirical evaluation, we demonstrate the effectiveness of our proposed method in achieving outstanding performance, with more than 25% bit-rate saving at the same subjective quality.
18.WEDGE: A multi-weather autonomous driving dataset built from generative vision-language models
Authors:Aboli Marathe, Deva Ramanan, Rahee Walambe, Ketan Kotecha
Abstract: The open road poses many challenges to autonomous perception, including poor visibility from extreme weather conditions. Models trained on good-weather datasets frequently fail at detection in these out-of-distribution settings. To aid adversarial robustness in perception, we introduce WEDGE (WEather images by DALL-E GEneration): a synthetic dataset generated with a vision-language generative model via prompting. WEDGE consists of 3360 images in 16 extreme weather conditions manually annotated with 16513 bounding boxes, supporting research in the tasks of weather classification and 2D object detection. We have analyzed WEDGE from research standpoints, verifying its effectiveness for extreme-weather autonomous perception. We establish baseline performance for classification and detection with 53.87% test accuracy and 45.41 mAP. Most importantly, WEDGE can be used to fine-tune state-of-the-art detectors, improving SOTA performance on real-world weather benchmarks (such as DAWN) by 4.48 AP for well-generated classes like trucks. WEDGE has been collected under OpenAI's terms of use and is released for public use under the CC BY-NC-SA 4.0 license. The repository for this work and dataset is available at https://infernolia.github.io/WEDGE.
19.Content-based jewellery item retrieval using the local region-based histograms
Authors:Amin Muhammad Shoib, Summaira Jabeen, Changbo Wang, Tassawar Ali
Abstract: Jewellery item retrieval is regularly used to find what people want on online marketplaces using a sample query reference image. Considering recent developments, due to the simultaneous nature of various jewelry items, various jewelry goods' occlusion in images or visual streams, as well as shape deformation, content-based jewellery item retrieval (CBJIR) still has limitations whenever it pertains to visual searching in the actual world. This article proposed a content-based jewellery item retrieval method using the local region-based histograms in HSV color space. Using five local regions, our novel jewellery classification module extracts the specific feature vectors from the query image. The jewellery classification module is also applied to the jewellery database to extract feature vectors. Finally, the similarity score is matched between the database and query features vectors to retrieve the jewellery items from the database. The proposed method performance is tested on publicly available jewellery item retrieval datasets, i.e. ringFIR and Fashion Product Images dataset. The experimental results demonstrate the dominance of the proposed method over the baseline methods for retrieving desired jewellery products.
20.Dish detection in food platters: A framework for automated diet logging and nutrition management
Authors:Mansi Goel, Shashank Dargar, Shounak Ghatak, Nidhi Verma, Pratik Chauhan, Anushka Gupta, Nikhila Vishnumolakala, Hareesh Amuru, Ekta Gambhir, Ronak Chhajed, Meenal Jain, Astha Jain, Samiksha Garg, Nitesh Narwade, Nikhilesh Verhwani, Abhuday Tiwari, Kirti Vashishtha, Ganesh Bagler
Abstract: Diet is central to the epidemic of lifestyle disorders. Accurate and effortless diet logging is one of the significant bottlenecks for effective diet management and calorie restriction. Dish detection from food platters is a challenging problem due to a visually complex food layout. We present an end-to-end computational framework for diet management, from data compilation, annotation, and state-of-the-art model identification to its mobile app implementation. As a case study, we implement the framework in the context of Indian food platters known for their complex presentation that poses a challenge for the automated detection of dishes. Starting with the 61 most popular Indian dishes, we identify the state-of-the-art model through a comparative analysis of deep-learning-based object detection architectures. Rooted in a meticulous compilation of 68,005 platter images with 134,814 manual dish annotations, we first compare ten architectures for multi-label classification to identify ResNet152 (mAP=84.51%) as the best model. YOLOv8x (mAP=87.70%) emerged as the best model architecture for dish detection among the eight deep-learning models implemented after a thorough performance evaluation. By comparing with the state-of-the-art model for the IndianFood10 dataset, we demonstrate the superior object detection performance of YOLOv8x for this subset and establish Resnet152 as the best architecture for multi-label classification. The models thus trained on richly annotated data can be extended to include dishes from across global cuisines. The proposed framework is demonstrated through a proof-of-concept mobile application with diverse applications for diet logging, food recommendation systems, nutritional interventions, and mitigation of lifestyle disorders.
21.Knowledge distillation with Segment Anything (SAM) model for Planetary Geological Mapping
Authors:Sahib Julka, Michael Granitzer
Abstract: Planetary science research involves analysing vast amounts of remote sensing data, which are often costly and time-consuming to annotate and process. One of the essential tasks in this field is geological mapping, which requires identifying and outlining regions of interest in planetary images, including geological features and landforms. However, manually labelling these images is a complex and challenging task that requires significant domain expertise and effort. To expedite this endeavour, we propose the use of knowledge distillation using the recently introduced cutting-edge Segment Anything (SAM) model. We demonstrate the effectiveness of this prompt-based foundation model for rapid annotation and quick adaptability to a prime use case of mapping planetary skylights. Our work reveals that with a small set of annotations obtained with the right prompts from the model and subsequently training a specialised domain decoder, we can achieve satisfactory semantic segmentation on this task. Key results indicate that the use of knowledge distillation can significantly reduce the effort required by domain experts for manual annotation and improve the efficiency of image segmentation tasks. This approach has the potential to accelerate extra-terrestrial discovery by automatically detecting and segmenting Martian landforms.
22.RHINO: Rotated DETR with Dynamic Denoising via Hungarian Matching for Oriented Object Detection
Authors:Hakjin Lee, Minki Song, Jamyoung Koo, Junghoon Seo
Abstract: With the publication of DINO, a variant of the Detection Transformer (DETR), Detection Transformers are breaking the record in the object detection benchmark with the merits of their end-to-end design and scalability. However, the extension of DETR to oriented object detection has not been thoroughly studied although more benefits from its end-to-end architecture are expected such as removing NMS and anchor-related costs. In this paper, we propose a first strong DINO-based baseline for oriented object detection. We found that straightforward employment of DETRs for oriented object detection does not guarantee non-duplicate prediction, and propose a simple cost to mitigate this. Furthermore, we introduce a novel denoising strategy that uses Hungarian matching to filter redundant noised queries and query alignment to preserve matching consistency between Transformer decoder layers. Our proposed model outperforms previous rotated DETRs and other counterparts, achieving state-of-the-art performance in DOTA-v1.0/v1.5/v2.0, and DIOR-R benchmarks.
23.ViT Unified: Joint Fingerprint Recognition and Presentation Attack Detection
Authors:Steven A. Grosz, Kanishka P. Wijewardena, Anil K. Jain
Abstract: A secure fingerprint recognition system must contain both a presentation attack (i.e., spoof) detection and recognition module in order to protect users against unwanted access by malicious users. Traditionally, these tasks would be carried out by two independent systems; however, recent studies have demonstrated the potential to have one unified system architecture in order to reduce the computational burdens on the system, while maintaining high accuracy. In this work, we leverage a vision transformer architecture for joint spoof detection and matching and report competitive results with state-of-the-art (SOTA) models for both a sequential system (two ViT models operating independently) and a unified architecture (a single ViT model for both tasks). ViT models are particularly well suited for this task as the ViT's global embedding encodes features useful for recognition, whereas the individual, local embeddings are useful for spoof detection. We demonstrate the capability of our unified model to achieve an average integrated matching (IM) accuracy of 98.87% across LivDet 2013 and 2015 CrossMatch sensors. This is comparable to IM accuracy of 98.95% of our sequential dual-ViT system, but with ~50% of the parameters and ~58% of the latency.
24.Spider GAN: Leveraging Friendly Neighbors to Accelerate GAN Training
Authors:Siddarth Asokan, Chandra Sekhar Seelamantula
Abstract: Training Generative adversarial networks (GANs) stably is a challenging task. The generator in GANs transform noise vectors, typically Gaussian distributed, into realistic data such as images. In this paper, we propose a novel approach for training GANs with images as inputs, but without enforcing any pairwise constraints. The intuition is that images are more structured than noise, which the generator can leverage to learn a more robust transformation. The process can be made efficient by identifying closely related datasets, or a ``friendly neighborhood'' of the target distribution, inspiring the moniker, Spider GAN. To define friendly neighborhoods leveraging proximity between datasets, we propose a new measure called the signed inception distance (SID), inspired by the polyharmonic kernel. We show that the Spider GAN formulation results in faster convergence, as the generator can discover correspondence even between seemingly unrelated datasets, for instance, between Tiny-ImageNet and CelebA faces. Further, we demonstrate cascading Spider GAN, where the output distribution from a pre-trained GAN generator is used as the input to the subsequent network. Effectively, transporting one distribution to another in a cascaded fashion until the target is learnt -- a new flavor of transfer learning. We demonstrate the efficacy of the Spider approach on DCGAN, conditional GAN, PGGAN, StyleGAN2 and StyleGAN3. The proposed approach achieves state-of-the-art Frechet inception distance (FID) values, with one-fifth of the training iterations, in comparison to their baseline counterparts on high-resolution small datasets such as MetFaces, Ukiyo-E Faces and AFHQ-Cats.
25.Uncertainty Estimation for Deep Learning Image Reconstruction using a Local Lipschitz Metric
Authors:Danyal F. Bhutto, Bo Zhu, Jeremiah Z. Liu, Neha Koonjoo, Bruce R. Rosen, Matthew S. Rosen
Abstract: The use of deep learning approaches for image reconstruction is of contemporary interest in radiology, especially for approaches that solve inverse problems associated with imaging. In deployment, these models may be exposed to input distributions that are widely shifted from training data, due in part to data biases or drifts. We propose a metric based on local Lipschitz determined from a single trained model that can be used to estimate the model uncertainty for image reconstructions. We demonstrate a monotonic relationship between the local Lipschitz value and Mean Absolute Error and show that this method can be used to provide a threshold that determines whether a given DL reconstruction approach was well suited to the task. Our uncertainty estimation method can be used to identify out-of-distribution test samples, relate information regarding epistemic uncertainties, and guide proper data augmentation. Quantifying uncertainty of learned reconstruction approaches is especially pertinent to the medical domain where reconstructed images must remain diagnostically accurate.
26.Meta Omnium: A Benchmark for General-Purpose Learning-to-Learn
Authors:Ondrej Bohdal, Yinbing Tian, Yongshuo Zong, Ruchika Chavhan, Da Li, Henry Gouk, Li Guo, Timothy Hospedales
Abstract: Meta-learning and other approaches to few-shot learning are widely studied for image recognition, and are increasingly applied to other vision tasks such as pose estimation and dense prediction. This naturally raises the question of whether there is any few-shot meta-learning algorithm capable of generalizing across these diverse task types? To support the community in answering this question, we introduce Meta Omnium, a dataset-of-datasets spanning multiple vision tasks including recognition, keypoint localization, semantic segmentation and regression. We experiment with popular few-shot meta-learning baselines and analyze their ability to generalize across tasks and to transfer knowledge between them. Meta Omnium enables meta-learning researchers to evaluate model generalization to a much wider array of tasks than previously possible, and provides a single framework for evaluating meta-learners across a wide suite of vision applications in a consistent manner.
27.Efficient Neural Network based Classification and Outlier Detection for Image Moderation using Compressed Sensing and Group Testing
Authors:Sabyasachi Ghosh, Sanyam Saxena, Ajit Rajwade
Abstract: Popular social media platforms employ neural network based image moderation engines to classify images uploaded on them as having potentially objectionable content. Such moderation engines must answer a large number of queries with heavy computational cost, even though the actual number of images with objectionable content is usually a tiny fraction. Inspired by recent work on Neural Group Testing, we propose an approach which exploits this fact to reduce the overall computational cost of such engines using the technique of Compressed Sensing (CS). We present the quantitative matrix-pooled neural network (QMPNN), which takes as input $n$ images, and a $m \times n$ binary pooling matrix with $m < n$, whose rows indicate $m$ pools of images i.e. selections of $r$ images out of $n$. The QMPNN efficiently outputs the product of this matrix with the unknown sparse binary vector indicating whether each image is objectionable or not, i.e. it outputs the number of objectionable images in each pool. For suitable matrices, this is decoded using CS decoding algorithms to predict which images were objectionable. The computational cost of running the QMPNN and the CS algorithms is significantly lower than the cost of using a neural network with the same number of parameters separately on each image to classify the images, which we demonstrate via extensive experiments. Our technique is inherently resilient to moderate levels of errors in the prediction from the QMPNN. Furthermore, we present pooled deep outlier detection, which brings CS and group testing techniques to deep outlier detection, to provide for the case when the objectionable images do not belong to a set of pre-defined classes. This technique enables efficient automated moderation of off-topic images shared on topical forums dedicated to sharing images of a certain single class, many of which are currently human-moderated.
28.The ASNR-MICCAI Brain Tumor Segmentation (BraTS) Challenge 2023: Intracranial Meningioma
Authors:Dominic LaBella, Maruf Adewole, Michelle Alonso-Basanta, Talissa Altes, Syed Muhammad Anwar, Ujjwal Baid, Timothy Bergquist, Radhika Bhalerao, Sully Chen, Verena Chung, Gian-Marco Conte, Farouk Dako, James Eddy, Ivan Ezhov, Devon Godfrey, Fathi Hilal, Ariana Familiar, Keyvan Farahani, Juan Eugenio Iglesias, Zhifan Jiang, Elaine Johanson, Anahita Fathi Kazerooni, Collin Kent, John Kirkpatrick, Florian Kofler, Koen Van Leemput, Hongwei Bran Li, Xinyang Liu, Aria Mahtabfar, Shan McBurney-Lin, Ryan McLean, Zeke Meier, Ahmed W Moawad, John Mongan, Pierre Nedelec, Maxence Pajot, Marie Piraud, Arif Rashid, Zachary Reitman, Russell Takeshi Shinohara, Yury Velichko, Chunhao Wang, Pranav Warman, Walter Wiggins, Mariam Aboian, Jake Albrecht, Udunna Anazodo, Spyridon Bakas, Adam Flanders, Anastasia Janas, Goldey Khanna, Marius George Linguraru, Bjoern Menze, Ayman Nada, Andreas M Rauschecker, Jeff Rudie, Nourel Hoda Tahon, Javier Villanueva-Meyer, Benedikt Wiestler, Evan Calabrese
Abstract: Meningiomas are the most common primary intracranial tumor in adults and can be associated with significant morbidity and mortality. Radiologists, neurosurgeons, neuro-oncologists, and radiation oncologists rely on multiparametric MRI (mpMRI) for diagnosis, treatment planning, and longitudinal treatment monitoring; yet automated, objective, and quantitative tools for non-invasive assessment of meningiomas on mpMRI are lacking. The BraTS meningioma 2023 challenge will provide a community standard and benchmark for state-of-the-art automated intracranial meningioma segmentation models based on the largest expert annotated multilabel meningioma mpMRI dataset to date. Challenge competitors will develop automated segmentation models to predict three distinct meningioma sub-regions on MRI including enhancing tumor, non-enhancing tumor core, and surrounding nonenhancing T2/FLAIR hyperintensity. Models will be evaluated on separate validation and held-out test datasets using standardized metrics utilized across the BraTS 2023 series of challenges including the Dice similarity coefficient and Hausdorff distance. The models developed during the course of this challenge will aid in incorporation of automated meningioma MRI segmentation into clinical practice, which will ultimately improve care of patients with meningioma.
29.A Critical View Of Vision-Based Long-Term Dynamics Prediction Under Environment Misalignment
Authors:Hanchen Xie, Jiageng Zhu, Mahyar Khayatkhoei, Jiazhi Li, Mohamed E. Hussein, Wael AbdAlmgaeed
Abstract: Dynamics prediction, which is the problem of predicting future states of scene objects based on current and prior states, is drawing increasing attention as an instance of learning physics. To solve this problem, Region Proposal Convolutional Interaction Network (RPCIN), a vision-based model, was proposed and achieved state-of-the-art performance in long-term prediction. RPCIN only takes raw images and simple object descriptions, such as the bounding box and segmentation mask of each object, as input. However, despite its success, the model's capability can be compromised under conditions of environment misalignment. In this paper, we investigate two challenging conditions for environment misalignment: Cross-Domain and Cross-Context by proposing four datasets that are designed for these challenges: SimB-Border, SimB-Split, BlenB-Border, and BlenB-Split. The datasets cover two domains and two contexts. Using RPCIN as a probe, experiments conducted on the combinations of the proposed datasets reveal potential weaknesses of the vision-based long-term dynamics prediction model. Furthermore, we propose a promising direction to mitigate the Cross-Domain challenge and provide concrete evidence supporting such a direction, which provides dramatic alleviation of the challenge on the proposed datasets.