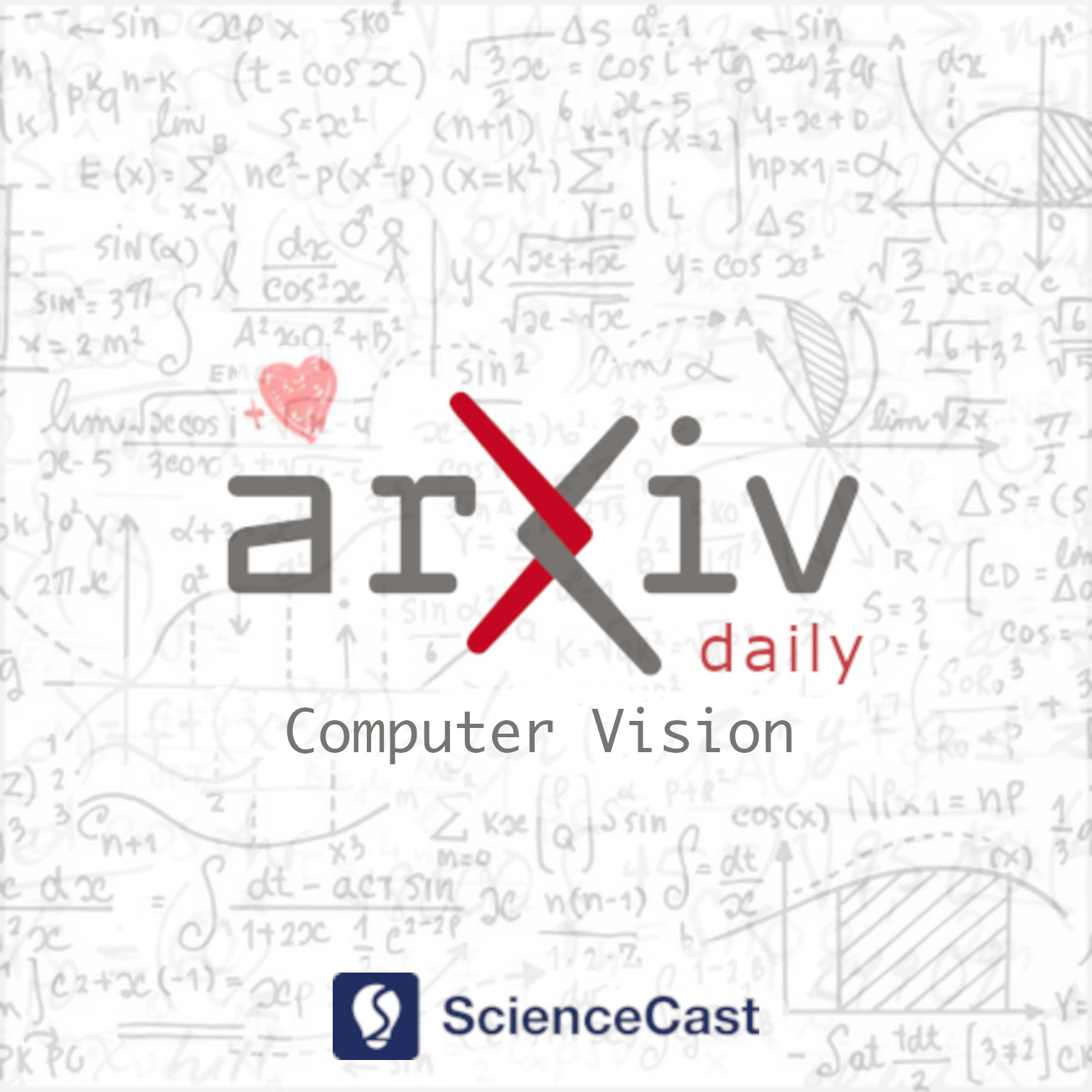
Computer Vision and Pattern Recognition (cs.CV)
Tue, 29 Aug 2023
1.Is it an i or an l: Test-time Adaptation of Text Line Recognition Models
Authors:Debapriya Tula, Sujoy Paul, Gagan Madan, Peter Garst, Reeve Ingle, Gaurav Aggarwal
Abstract: Recognizing text lines from images is a challenging problem, especially for handwritten documents due to large variations in writing styles. While text line recognition models are generally trained on large corpora of real and synthetic data, such models can still make frequent mistakes if the handwriting is inscrutable or the image acquisition process adds corruptions, such as noise, blur, compression, etc. Writing style is generally quite consistent for an individual, which can be leveraged to correct mistakes made by such models. Motivated by this, we introduce the problem of adapting text line recognition models during test time. We focus on a challenging and realistic setting where, given only a single test image consisting of multiple text lines, the task is to adapt the model such that it performs better on the image, without any labels. We propose an iterative self-training approach that uses feedback from the language model to update the optical model, with confident self-labels in each iteration. The confidence measure is based on an augmentation mechanism that evaluates the divergence of the prediction of the model in a local region. We perform rigorous evaluation of our method on several benchmark datasets as well as their corrupted versions. Experimental results on multiple datasets spanning multiple scripts show that the proposed adaptation method offers an absolute improvement of up to 8% in character error rate with just a few iterations of self-training at test time.
2.Pose-Free Neural Radiance Fields via Implicit Pose Regularization
Authors:Jiahui Zhang, Fangneng Zhan, Yingchen Yu, Kunhao Liu, Rongliang Wu, Xiaoqin Zhang, Ling Shao, Shijian Lu
Abstract: Pose-free neural radiance fields (NeRF) aim to train NeRF with unposed multi-view images and it has achieved very impressive success in recent years. Most existing works share the pipeline of training a coarse pose estimator with rendered images at first, followed by a joint optimization of estimated poses and neural radiance field. However, as the pose estimator is trained with only rendered images, the pose estimation is usually biased or inaccurate for real images due to the domain gap between real images and rendered images, leading to poor robustness for the pose estimation of real images and further local minima in joint optimization. We design IR-NeRF, an innovative pose-free NeRF that introduces implicit pose regularization to refine pose estimator with unposed real images and improve the robustness of the pose estimation for real images. With a collection of 2D images of a specific scene, IR-NeRF constructs a scene codebook that stores scene features and captures the scene-specific pose distribution implicitly as priors. Thus, the robustness of pose estimation can be promoted with the scene priors according to the rationale that a 2D real image can be well reconstructed from the scene codebook only when its estimated pose lies within the pose distribution. Extensive experiments show that IR-NeRF achieves superior novel view synthesis and outperforms the state-of-the-art consistently across multiple synthetic and real datasets.
3.iBARLE: imBalance-Aware Room Layout Estimation
Authors:Taotao Jing, Lichen Wang, Naji Khosravan, Zhiqiang Wan, Zachary Bessinger, Zhengming Ding, Sing Bing Kang
Abstract: Room layout estimation predicts layouts from a single panorama. It requires datasets with large-scale and diverse room shapes to train the models. However, there are significant imbalances in real-world datasets including the dimensions of layout complexity, camera locations, and variation in scene appearance. These issues considerably influence the model training performance. In this work, we propose the imBalance-Aware Room Layout Estimation (iBARLE) framework to address these issues. iBARLE consists of (1) Appearance Variation Generation (AVG) module, which promotes visual appearance domain generalization, (2) Complex Structure Mix-up (CSMix) module, which enhances generalizability w.r.t. room structure, and (3) a gradient-based layout objective function, which allows more effective accounting for occlusions in complex layouts. All modules are jointly trained and help each other to achieve the best performance. Experiments and ablation studies based on ZInD~\cite{cruz2021zillow} dataset illustrate that iBARLE has state-of-the-art performance compared with other layout estimation baselines.
4.A Consumer-tier based Visual-Brain Machine Interface for Augmented Reality Glasses Interactions
Authors:Yuying Jiang, Fan Bai, Zicheng Zhang, Xiaochen Ye, Zheng Liu, Zhiping Shi, Jianwei Yao, Xiaojun Liu, Fangkun Zhu, Junling Li Qian Guo, Xiaoan Wang, Junwen Luo
Abstract: Objective.Visual-Brain Machine Interface(V-BMI) has provide a novel interaction technique for Augmented Reality (AR) industries. Several state-of-arts work has demonstates its high accuracy and real-time interaction capbilities. However, most of the studies employ EEGs devices that are rigid and difficult to apply in real-life AR glasseses application sceniraros. Here we develop a consumer-tier Visual-Brain Machine Inteface(V-BMI) system specialized for Augmented Reality(AR) glasses interactions. Approach. The developed system consists of a wearable hardware which takes advantages of fast set-up, reliable recording and comfortable wearable experience that specificized for AR glasses applications. Complementing this hardware, we have devised a software framework that facilitates real-time interactions within the system while accommodating a modular configuration to enhance scalability. Main results. The developed hardware is only 110g and 120x85x23 mm, which with 1 Tohm and peak to peak voltage is less than 1.5 uV, and a V-BMI based angry bird game and an Internet of Thing (IoT) AR applications are deisgned, we demonstrated such technology merits of intuitive experience and efficiency interaction. The real-time interaction accuracy is between 85 and 96 percentages in a commercial AR glasses (DTI is 2.24s and ITR 65 bits-min ). Significance. Our study indicates the developed system can provide an essential hardware-software framework for consumer based V-BMI AR glasses. Also, we derive several pivotal design factors for a consumer-grade V-BMI-based AR system: 1) Dynamic adaptation of stimulation patterns-classification methods via computer vision algorithms is necessary for AR glasses applications; and 2) Algorithmic localization to foster system stability and latency reduction.
5.Learning Cross-modality Information Bottleneck Representation for Heterogeneous Person Re-Identification
Authors:Haichao Shi, Mandi Luo, Xiao-Yu Zhang, Ran He
Abstract: Visible-Infrared person re-identification (VI-ReID) is an important and challenging task in intelligent video surveillance. Existing methods mainly focus on learning a shared feature space to reduce the modality discrepancy between visible and infrared modalities, which still leave two problems underexplored: information redundancy and modality complementarity. To this end, properly eliminating the identity-irrelevant information as well as making up for the modality-specific information are critical and remains a challenging endeavor. To tackle the above problems, we present a novel mutual information and modality consensus network, namely CMInfoNet, to extract modality-invariant identity features with the most representative information and reduce the redundancies. The key insight of our method is to find an optimal representation to capture more identity-relevant information and compress the irrelevant parts by optimizing a mutual information bottleneck trade-off. Besides, we propose an automatically search strategy to find the most prominent parts that identify the pedestrians. To eliminate the cross- and intra-modality variations, we also devise a modality consensus module to align the visible and infrared modalities for task-specific guidance. Moreover, the global-local feature representations can also be acquired for key parts discrimination. Experimental results on four benchmarks, i.e., SYSU-MM01, RegDB, Occluded-DukeMTMC, Occluded-REID, Partial-REID and Partial\_iLIDS dataset, have demonstrated the effectiveness of CMInfoNet.
6.DiffBIR: Towards Blind Image Restoration with Generative Diffusion Prior
Authors:Xinqi Lin, Jingwen He, Ziyan Chen, Zhaoyang Lyu, Ben Fei, Bo Dai, Wanli Ouyang, Yu Qiao, Chao Dong
Abstract: We present DiffBIR, which leverages pretrained text-to-image diffusion models for blind image restoration problem. Our framework adopts a two-stage pipeline. In the first stage, we pretrain a restoration module across diversified degradations to improve generalization capability in real-world scenarios. The second stage leverages the generative ability of latent diffusion models, to achieve realistic image restoration. Specifically, we introduce an injective modulation sub-network -- LAControlNet for finetuning, while the pre-trained Stable Diffusion is to maintain its generative ability. Finally, we introduce a controllable module that allows users to balance quality and fidelity by introducing the latent image guidance in the denoising process during inference. Extensive experiments have demonstrated its superiority over state-of-the-art approaches for both blind image super-resolution and blind face restoration tasks on synthetic and real-world datasets. The code is available at https://github.com/XPixelGroup/DiffBIR.
7.Exploring Model Transferability through the Lens of Potential Energy
Authors:Xiaotong Li, Zixuan Hu, Yixiao Ge, Ying Shan, Ling-Yu Duan
Abstract: Transfer learning has become crucial in computer vision tasks due to the vast availability of pre-trained deep learning models. However, selecting the optimal pre-trained model from a diverse pool for a specific downstream task remains a challenge. Existing methods for measuring the transferability of pre-trained models rely on statistical correlations between encoded static features and task labels, but they overlook the impact of underlying representation dynamics during fine-tuning, leading to unreliable results, especially for self-supervised models. In this paper, we present an insightful physics-inspired approach named PED to address these challenges. We reframe the challenge of model selection through the lens of potential energy and directly model the interaction forces that influence fine-tuning dynamics. By capturing the motion of dynamic representations to decline the potential energy within a force-driven physical model, we can acquire an enhanced and more stable observation for estimating transferability. The experimental results on 10 downstream tasks and 12 self-supervised models demonstrate that our approach can seamlessly integrate into existing ranking techniques and enhance their performances, revealing its effectiveness for the model selection task and its potential for understanding the mechanism in transfer learning. Code will be available at https://github.com/lixiaotong97/PED.
8.Class Prior-Free Positive-Unlabeled Learning with Taylor Variational Loss for Hyperspectral Remote Sensing Imagery
Authors:Hengwei Zhao, Xinyu Wang, Jingtao Li, Yanfei Zhong
Abstract: Positive-unlabeled learning (PU learning) in hyperspectral remote sensing imagery (HSI) is aimed at learning a binary classifier from positive and unlabeled data, which has broad prospects in various earth vision applications. However, when PU learning meets limited labeled HSI, the unlabeled data may dominate the optimization process, which makes the neural networks overfit the unlabeled data. In this paper, a Taylor variational loss is proposed for HSI PU learning, which reduces the weight of the gradient of the unlabeled data by Taylor series expansion to enable the network to find a balance between overfitting and underfitting. In addition, the self-calibrated optimization strategy is designed to stabilize the training process. Experiments on 7 benchmark datasets (21 tasks in total) validate the effectiveness of the proposed method. Code is at: https://github.com/Hengwei-Zhao96/T-HOneCls.
9.Learning to Upsample by Learning to Sample
Authors:Wenze Liu, Hao Lu, Hongtao Fu, Zhiguo Cao
Abstract: We present DySample, an ultra-lightweight and effective dynamic upsampler. While impressive performance gains have been witnessed from recent kernel-based dynamic upsamplers such as CARAFE, FADE, and SAPA, they introduce much workload, mostly due to the time-consuming dynamic convolution and the additional sub-network used to generate dynamic kernels. Further, the need for high-res feature guidance of FADE and SAPA somehow limits their application scenarios. To address these concerns, we bypass dynamic convolution and formulate upsampling from the perspective of point sampling, which is more resource-efficient and can be easily implemented with the standard built-in function in PyTorch. We first showcase a naive design, and then demonstrate how to strengthen its upsampling behavior step by step towards our new upsampler, DySample. Compared with former kernel-based dynamic upsamplers, DySample requires no customized CUDA package and has much fewer parameters, FLOPs, GPU memory, and latency. Besides the light-weight characteristics, DySample outperforms other upsamplers across five dense prediction tasks, including semantic segmentation, object detection, instance segmentation, panoptic segmentation, and monocular depth estimation. Code is available at https://github.com/tiny-smart/dysample.
10.Group-Conditional Conformal Prediction via Quantile Regression Calibration for Crop and Weed Classification
Authors:Paul Melki IMS, Lionel Bombrun IMS, Boubacar Diallo IMS, Jérôme Dias IMS, Jean-Pierre da Costa IMS
Abstract: As deep learning predictive models become an integral part of a large spectrum of precision agricultural systems, a barrier to the adoption of such automated solutions is the lack of user trust in these highly complex, opaque and uncertain models. Indeed, deep neural networks are not equipped with any explicit guarantees that can be used to certify the system's performance, especially in highly varying uncontrolled environments such as the ones typically faced in computer vision for agriculture.Fortunately, certain methods developed in other communities can prove to be important for agricultural applications. This article presents the conformal prediction framework that provides valid statistical guarantees on the predictive performance of any black box prediction machine, with almost no assumptions, applied to the problem of deep visual classification of weeds and crops in real-world conditions. The framework is exposed with a focus on its practical aspects and special attention accorded to the Adaptive Prediction Sets (APS) approach that delivers marginal guarantees on the model's coverage. Marginal results are then shown to be insufficient to guarantee performance on all groups of individuals in the population as characterized by their environmental and pedo-climatic auxiliary data gathered during image acquisition.To tackle this shortcoming, group-conditional conformal approaches are presented: the ''classical'' method that consists of iteratively applying the APS procedure on all groups, and a proposed elegant reformulation and implementation of the procedure using quantile regression on group membership indicators. Empirical results showing the validity of the proposed approach are presented and compared to the marginal APS then discussed.
11.DiffusionVMR: Diffusion Model for Video Moment Retrieval
Authors:Henghao Zhao, Kevin Qinghong Lin, Rui Yan, Zechao Li
Abstract: Video moment retrieval is a fundamental visual-language task that aims to retrieve target moments from an untrimmed video based on a language query. Existing methods typically generate numerous proposals manually or via generative networks in advance as the support set for retrieval, which is not only inflexible but also time-consuming. Inspired by the success of diffusion models on object detection, this work aims at reformulating video moment retrieval as a denoising generation process to get rid of the inflexible and time-consuming proposal generation. To this end, we propose a novel proposal-free framework, namely DiffusionVMR, which directly samples random spans from noise as candidates and introduces denoising learning to ground target moments. During training, Gaussian noise is added to the real moments, and the model is trained to learn how to reverse this process. In inference, a set of time spans is progressively refined from the initial noise to the final output. Notably, the training and inference of DiffusionVMR are decoupled, and an arbitrary number of random spans can be used in inference without being consistent with the training phase. Extensive experiments conducted on three widely-used benchmarks (i.e., QVHighlight, Charades-STA, and TACoS) demonstrate the effectiveness of the proposed DiffusionVMR by comparing it with state-of-the-art methods.
12.Abdominal Multi-Organ Segmentation Based on Feature Pyramid Network and Spatial Recurrent Neural Network
Authors:Yuhan Song, Armagan Elibol, Nak Young Chong
Abstract: As recent advances in AI are causing the decline of conventional diagnostic methods, the realization of end-to-end diagnosis is fast approaching. Ultrasound image segmentation is an important step in the diagnostic process. An accurate and robust segmentation model accelerates the process and reduces the burden of sonographers. In contrast to previous research, we take two inherent features of ultrasound images into consideration: (1) different organs and tissues vary in spatial sizes, (2) the anatomical structures inside human body form a relatively constant spatial relationship. Based on those two ideas, we propose a new image segmentation model combining Feature Pyramid Network (FPN) and Spatial Recurrent Neural Network (SRNN). We discuss why we use FPN to extract anatomical structures of different scales and how SRNN is implemented to extract the spatial context features in abdominal ultrasound images.
13.A Multimodal Visual Encoding Model Aided by Introducing Verbal Semantic Information
Authors:Shuxiao Ma, Linyuan Wang, Bin Yan
Abstract: Biological research has revealed that the verbal semantic information in the brain cortex, as an additional source, participates in nonverbal semantic tasks, such as visual encoding. However, previous visual encoding models did not incorporate verbal semantic information, contradicting this biological finding. This paper proposes a multimodal visual information encoding network model based on stimulus images and associated textual information in response to this issue. Our visual information encoding network model takes stimulus images as input and leverages textual information generated by a text-image generation model as verbal semantic information. This approach injects new information into the visual encoding model. Subsequently, a Transformer network aligns image and text feature information, creating a multimodal feature space. A convolutional network then maps from this multimodal feature space to voxel space, constructing the multimodal visual information encoding network model. Experimental results demonstrate that the proposed multimodal visual information encoding network model outperforms previous models under the exact training cost. In voxel prediction of the left hemisphere of subject 1's brain, the performance improves by approximately 15.87%, while in the right hemisphere, the performance improves by about 4.6%. The multimodal visual encoding network model exhibits superior encoding performance. Additionally, ablation experiments indicate that our proposed model better simulates the brain's visual information processing.
14.Uncovering the Unseen: Discover Hidden Intentions by Micro-Behavior Graph Reasoning
Authors:Zhuo Zhou, Wenxuan Liu, Danni Xu, Zheng Wang, Jian Zhao
Abstract: This paper introduces a new and challenging Hidden Intention Discovery (HID) task. Unlike existing intention recognition tasks, which are based on obvious visual representations to identify common intentions for normal behavior, HID focuses on discovering hidden intentions when humans try to hide their intentions for abnormal behavior. HID presents a unique challenge in that hidden intentions lack the obvious visual representations to distinguish them from normal intentions. Fortunately, from a sociological and psychological perspective, we find that the difference between hidden and normal intentions can be reasoned from multiple micro-behaviors, such as gaze, attention, and facial expressions. Therefore, we first discover the relationship between micro-behavior and hidden intentions and use graph structure to reason about hidden intentions. To facilitate research in the field of HID, we also constructed a seminal dataset containing a hidden intention annotation of a typical theft scenario for HID. Extensive experiments show that the proposed network improves performance on the HID task by 9.9\% over the state-of-the-art method SBP.
15.A lightweight 3D dense facial landmark estimation model from position map data
Authors:Shubhajit Basak, Sathish Mangapuram, Gabriel Costache, Rachel McDonnell, Michael Schukat
Abstract: The incorporation of 3D data in facial analysis tasks has gained popularity in recent years. Though it provides a more accurate and detailed representation of the human face, accruing 3D face data is more complex and expensive than 2D face images. Either one has to rely on expensive 3D scanners or depth sensors which are prone to noise. An alternative option is the reconstruction of 3D faces from uncalibrated 2D images in an unsupervised way without any ground truth 3D data. However, such approaches are computationally expensive and the learned model size is not suitable for mobile or other edge device applications. Predicting dense 3D landmarks over the whole face can overcome this issue. As there is no public dataset available containing dense landmarks, we propose a pipeline to create a dense keypoint training dataset containing 520 key points across the whole face from an existing facial position map data. We train a lightweight MobileNet-based regressor model with the generated data. As we do not have access to any evaluation dataset with dense landmarks in it we evaluate our model against the 68 keypoint detection task. Experimental results show that our trained model outperforms many of the existing methods in spite of its lower model size and minimal computational cost. Also, the qualitative evaluation shows the efficiency of our trained models in extreme head pose angles as well as other facial variations and occlusions.
16.Optron: Better Medical Image Registration via Training in the Loop
Authors:Yicheng Chen, Shengxiang Ji, Yuelin Xin, Kun Han, Xiaohui Xie
Abstract: Previously, in the field of medical image registration, there are primarily two paradigms, the traditional optimization-based methods, and the deep-learning-based methods. Each of these paradigms has its advantages, and in this work, we aim to take the best of both worlds. Instead of developing a new deep learning model, we designed a robust training architecture that is simple and generalizable. We present Optron, a general training architecture incorporating the idea of training-in-the-loop. By iteratively optimizing the prediction result of a deep learning model through a plug-and-play optimizer module in the training loop, Optron introduces pseudo ground truth to an unsupervised training process. And by bringing the training process closer to that of supervised training, Optron can consistently improve the models' performance and convergence speed. We evaluated our method on various combinations of models and datasets, and we have achieved state-of-the-art performance on the IXI dataset, improving the previous state-of-the-art method TransMorph by a significant margin of +1.6% DSC. Moreover, Optron also consistently achieved positive results with other models and datasets. It increases the validation DSC for VoxelMorph and ViT-V-Net by +2.3% and +2.2% respectively on IXI, demonstrating our method's generalizability. Our implementation is publicly available at https://github.com/miraclefactory/optron
17.CLIPTrans: Transferring Visual Knowledge with Pre-trained Models for Multimodal Machine Translation
Authors:Devaansh Gupta, Siddhant Kharbanda, Jiawei Zhou, Wanhua Li, Hanspeter Pfister, Donglai Wei
Abstract: There has been a growing interest in developing multimodal machine translation (MMT) systems that enhance neural machine translation (NMT) with visual knowledge. This problem setup involves using images as auxiliary information during training, and more recently, eliminating their use during inference. Towards this end, previous works face a challenge in training powerful MMT models from scratch due to the scarcity of annotated multilingual vision-language data, especially for low-resource languages. Simultaneously, there has been an influx of multilingual pre-trained models for NMT and multimodal pre-trained models for vision-language tasks, primarily in English, which have shown exceptional generalisation ability. However, these are not directly applicable to MMT since they do not provide aligned multimodal multilingual features for generative tasks. To alleviate this issue, instead of designing complex modules for MMT, we propose CLIPTrans, which simply adapts the independently pre-trained multimodal M-CLIP and the multilingual mBART. In order to align their embedding spaces, mBART is conditioned on the M-CLIP features by a prefix sequence generated through a lightweight mapping network. We train this in a two-stage pipeline which warms up the model with image captioning before the actual translation task. Through experiments, we demonstrate the merits of this framework and consequently push forward the state-of-the-art across standard benchmarks by an average of +2.67 BLEU. The code can be found at www.github.com/devaansh100/CLIPTrans.
18.Rotation Augmented Distillation for Exemplar-Free Class Incremental Learning with Detailed Analysis
Authors:Xiuwei Chen, Xiaobin Chang
Abstract: Class incremental learning (CIL) aims to recognize both the old and new classes along the increment tasks. Deep neural networks in CIL suffer from catastrophic forgetting and some approaches rely on saving exemplars from previous tasks, known as the exemplar-based setting, to alleviate this problem. On the contrary, this paper focuses on the Exemplar-Free setting with no old class sample preserved. Balancing the plasticity and stability in deep feature learning with only supervision from new classes is more challenging. Most existing Exemplar-Free CIL methods report the overall performance only and lack further analysis. In this work, different methods are examined with complementary metrics in greater detail. Moreover, we propose a simple CIL method, Rotation Augmented Distillation (RAD), which achieves one of the top-tier performances under the Exemplar-Free setting. Detailed analysis shows our RAD benefits from the superior balance between plasticity and stability. Finally, more challenging exemplar-free settings with fewer initial classes are undertaken for further demonstrations and comparisons among the state-of-the-art methods.
19.Enhancing OCR Performance through Post-OCR Models: Adopting Glyph Embedding for Improved Correction
Authors:Yung-Hsin Chen, Yuli Zhou
Abstract: The study investigates the potential of post-OCR models to overcome limitations in OCR models and explores the impact of incorporating glyph embedding on post-OCR correction performance. In this study, we have developed our own post-OCR correction model. The novelty of our approach lies in embedding the OCR output using CharBERT and our unique embedding technique, capturing the visual characteristics of characters. Our findings show that post-OCR correction effectively addresses deficiencies in inferior OCR models, and glyph embedding enables the model to achieve superior results, including the ability to correct individual words.
20.NOVIS: A Case for End-to-End Near-Online Video Instance Segmentation
Authors:Tim Meinhardt, Matt Feiszli, Yuchen Fan, Laura Leal-Taixe, Rakesh Ranjan
Abstract: Until recently, the Video Instance Segmentation (VIS) community operated under the common belief that offline methods are generally superior to a frame by frame online processing. However, the recent success of online methods questions this belief, in particular, for challenging and long video sequences. We understand this work as a rebuttal of those recent observations and an appeal to the community to focus on dedicated near-online VIS approaches. To support our argument, we present a detailed analysis on different processing paradigms and the new end-to-end trainable NOVIS (Near-Online Video Instance Segmentation) method. Our transformer-based model directly predicts spatio-temporal mask volumes for clips of frames and performs instance tracking between clips via overlap embeddings. NOVIS represents the first near-online VIS approach which avoids any handcrafted tracking heuristics. We outperform all existing VIS methods by large margins and provide new state-of-the-art results on both YouTube-VIS (2019/2021) and the OVIS benchmarks.
21.Cross-Modal Retrieval Meets Inference:Improving Zero-Shot Classification with Cross-Modal Retrieval
Authors:Seongha Eom, Namgyu Ho, Jaehoon Oh, Se-Young Yun
Abstract: Contrastive language-image pre-training (CLIP) has demonstrated remarkable zero-shot classification ability, namely image classification using novel text labels. Existing works have attempted to enhance CLIP by fine-tuning on downstream tasks, but these have inadvertently led to performance degradation on unseen classes, thus harming zero-shot generalization. This paper aims to address this challenge by leveraging readily available image-text pairs from an external dataset for cross-modal guidance during inference. To this end, we propose X-MoRe, a novel inference method comprising two key steps: (1) cross-modal retrieval and (2) modal-confidence-based ensemble. Given a query image, we harness the power of CLIP's cross-modal representations to retrieve relevant textual information from an external image-text pair dataset. Then, we assign higher weights to the more reliable modality between the original query image and retrieved text, contributing to the final prediction. X-MoRe demonstrates robust performance across a diverse set of tasks without the need for additional training, showcasing the effectiveness of utilizing cross-modal features to maximize CLIP's zero-shot ability.
22.ADFA: Attention-augmented Differentiable top-k Feature Adaptation for Unsupervised Medical Anomaly Detection
Authors:Yiming Huang, Guole Liu, Yaoru Luo, Ge Yang
Abstract: The scarcity of annotated data, particularly for rare diseases, limits the variability of training data and the range of detectable lesions, presenting a significant challenge for supervised anomaly detection in medical imaging. To solve this problem, we propose a novel unsupervised method for medical image anomaly detection: Attention-Augmented Differentiable top-k Feature Adaptation (ADFA). The method utilizes Wide-ResNet50-2 (WR50) network pre-trained on ImageNet to extract initial feature representations. To reduce the channel dimensionality while preserving relevant channel information, we employ an attention-augmented patch descriptor on the extracted features. We then apply differentiable top-k feature adaptation to train the patch descriptor, mapping the extracted feature representations to a new vector space, enabling effective detection of anomalies. Experiments show that ADFA outperforms state-of-the-art (SOTA) methods on multiple challenging medical image datasets, confirming its effectiveness in medical anomaly detection.
23.ARTxAI: Explainable Artificial Intelligence Curates Deep Representation Learning for Artistic Images using Fuzzy Techniques
Authors:Javier Fumanal-Idocin, Javier Andreu-Perez, Oscar Cordón, Hani Hagras, Humberto Bustince
Abstract: Automatic art analysis employs different image processing techniques to classify and categorize works of art. When working with artistic images, we need to take into account further considerations compared to classical image processing. This is because such artistic paintings change drastically depending on the author, the scene depicted, and their artistic style. This can result in features that perform very well in a given task but do not grasp the whole of the visual and symbolic information contained in a painting. In this paper, we show how the features obtained from different tasks in artistic image classification are suitable to solve other ones of similar nature. We present different methods to improve the generalization capabilities and performance of artistic classification systems. Furthermore, we propose an explainable artificial intelligence method to map known visual traits of an image with the features used by the deep learning model considering fuzzy rules. These rules show the patterns and variables that are relevant to solve each task and how effective is each of the patterns found. Our results show that our proposed context-aware features can achieve up to $6\%$ and $26\%$ more accurate results than other context- and non-context-aware solutions, respectively, depending on the specific task. We also show that some of the features used by these models can be more clearly correlated to visual traits in the original image than others.
24.MSFlow: Multi-Scale Flow-based Framework for Unsupervised Anomaly Detection
Authors:Yixuan Zhou, Xing Xu, Jingkuan Song, Fumin Shen, Heng Tao Shen
Abstract: Unsupervised anomaly detection (UAD) attracts a lot of research interest and drives widespread applications, where only anomaly-free samples are available for training. Some UAD applications intend to further locate the anomalous regions without any anomaly information. Although the absence of anomalous samples and annotations deteriorates the UAD performance, an inconspicuous yet powerful statistics model, the normalizing flows, is appropriate for anomaly detection and localization in an unsupervised fashion. The flow-based probabilistic models, only trained on anomaly-free data, can efficiently distinguish unpredictable anomalies by assigning them much lower likelihoods than normal data. Nevertheless, the size variation of unpredictable anomalies introduces another inconvenience to the flow-based methods for high-precision anomaly detection and localization. To generalize the anomaly size variation, we propose a novel Multi-Scale Flow-based framework dubbed MSFlow composed of asymmetrical parallel flows followed by a fusion flow to exchange multi-scale perceptions. Moreover, different multi-scale aggregation strategies are adopted for image-wise anomaly detection and pixel-wise anomaly localization according to the discrepancy between them. The proposed MSFlow is evaluated on three anomaly detection datasets, significantly outperforming existing methods. Notably, on the challenging MVTec AD benchmark, our MSFlow achieves a new state-of-the-art with a detection AUORC score of up to 99.7%, localization AUCROC score of 98.8%, and PRO score of 97.1%. The reproducible code is available at https://github.com/cool-xuan/msflow.
25.Spatio-temporal MLP-graph network for 3D human pose estimation
Authors:Tanvir Hassan, A. Ben Hamza
Abstract: Graph convolutional networks and their variants have shown significant promise in 3D human pose estimation. Despite their success, most of these methods only consider spatial correlations between body joints and do not take into account temporal correlations, thereby limiting their ability to capture relationships in the presence of occlusions and inherent ambiguity. To address this potential weakness, we propose a spatio-temporal network architecture composed of a joint-mixing multi-layer perceptron block that facilitates communication among different joints and a graph weighted Jacobi network block that enables communication among various feature channels. The major novelty of our approach lies in a new weighted Jacobi feature propagation rule obtained through graph filtering with implicit fairing. We leverage temporal information from the 2D pose sequences, and integrate weight modulation into the model to enable untangling of the feature transformations of distinct nodes. We also employ adjacency modulation with the aim of learning meaningful correlations beyond defined linkages between body joints by altering the graph topology through a learnable modulation matrix. Extensive experiments on two benchmark datasets demonstrate the effectiveness of our model, outperforming recent state-of-the-art methods for 3D human pose estimation.
26.3D-MuPPET: 3D Multi-Pigeon Pose Estimation and Tracking
Authors:Urs Waldmann, Alex Hoi Hang Chan, Hemal Naik, Máté Nagy, Iain D. Couzin, Oliver Deussen, Bastian Goldluecke, Fumihiro Kano
Abstract: Markerless methods for animal posture tracking have been developing recently, but frameworks and benchmarks for tracking large animal groups in 3D are still lacking. To overcome this gap in the literature, we present 3D-MuPPET, a framework to estimate and track 3D poses of up to 10 pigeons at interactive speed using multiple-views. We train a pose estimator to infer 2D keypoints and bounding boxes of multiple pigeons, then triangulate the keypoints to 3D. For correspondence matching, we first dynamically match 2D detections to global identities in the first frame, then use a 2D tracker to maintain correspondences accross views in subsequent frames. We achieve comparable accuracy to a state of the art 3D pose estimator for Root Mean Square Error (RMSE) and Percentage of Correct Keypoints (PCK). We also showcase a novel use case where our model trained with data of single pigeons provides comparable results on data containing multiple pigeons. This can simplify the domain shift to new species because annotating single animal data is less labour intensive than multi-animal data. Additionally, we benchmark the inference speed of 3D-MuPPET, with up to 10 fps in 2D and 1.5 fps in 3D, and perform quantitative tracking evaluation, which yields encouraging results. Finally, we show that 3D-MuPPET also works in natural environments without model fine-tuning on additional annotations. To the best of our knowledge we are the first to present a framework for 2D/3D posture and trajectory tracking that works in both indoor and outdoor environments.
27.Occlusion-Aware Deep Convolutional Neural Network via Homogeneous Tanh-transforms for Face Parsing
Authors:Weihua Liu, Chaochao Lin, Haoping Yu, Said Boumaraf, Zhaoqiong Pi
Abstract: Face parsing infers a pixel-wise label map for each semantic facial component. Previous methods generally work well for uncovered faces, however overlook the facial occlusion and ignore some contextual area outside a single face, especially when facial occlusion has become a common situation during the COVID-19 epidemic. Inspired by the illumination theory of image, we propose a novel homogeneous tanh-transforms for image preprocessing, which made up of four tanh-transforms, that fuse the central vision and the peripheral vision together. Our proposed method addresses the dilemma of face parsing under occlusion and compresses more information of surrounding context. Based on homogeneous tanh-transforms, we propose an occlusion-aware convolutional neural network for occluded face parsing. It combines the information both in Tanh-polar space and Tanh-Cartesian space, capable of enhancing receptive fields. Furthermore, we introduce an occlusion-aware loss to focus on the boundaries of occluded regions. The network is simple and flexible, and can be trained end-to-end. To facilitate future research of occluded face parsing, we also contribute a new cleaned face parsing dataset, which is manually purified from several academic or industrial datasets, including CelebAMask-HQ, Short-video Face Parsing as well as Helen dataset and will make it public. Experiments demonstrate that our method surpasses state-of-art methods of face parsing under occlusion.
28.IndGIC: Supervised Action Recognition under Low Illumination
Authors:Jingbo Zeng
Abstract: Technologies of human action recognition in the dark are gaining more and more attention as huge demand in surveillance, motion control and human-computer interaction. However, because of limitation in image enhancement method and low-lighting video datasets, e.g. labeling cost, existing methods meet some problems. Some video-based approached are effect and efficient in specific datasets but cannot generalize to most cases while others methods using multiple sensors rely heavily to prior knowledge to deal with noisy nature from video stream. In this paper, we proposes action recognition method using deep multi-input network. Furthermore, we proposed a Independent Gamma Intensity Corretion (Ind-GIC) to enhance poor-illumination video, generating one gamma for one frame to increase enhancement performance. To prove our method is effective, there is some evaluation and comparison between our method and existing methods. Experimental results show that our model achieves high accuracy in on ARID dataset.
29.Enhancing Mobile Face Anti-Spoofing: A Robust Framework for Diverse Attack Types under Screen Flash
Authors:Weihua Liu, Chaochao Lin, Yu Yan
Abstract: Face anti-spoofing (FAS) is crucial for securing face recognition systems. However, existing FAS methods with handcrafted binary or pixel-wise labels have limitations due to diverse presentation attacks (PAs). In this paper, we propose an attack type robust face anti-spoofing framework under light flash, called ATR-FAS. Due to imaging differences caused by various attack types, traditional FAS methods based on single binary classification network may result in excessive intra-class distance of spoof faces, leading to a challenge of decision boundary learning. Therefore, we employed multiple networks to reconstruct multi-frame depth maps as auxiliary supervision, and each network experts in one type of attack. A dual gate module (DGM) consisting of a type gate and a frame-attention gate is introduced, which perform attack type recognition and multi-frame attention generation, respectively. The outputs of DGM are utilized as weight to mix the result of multiple expert networks. The multi-experts mixture enables ATR-FAS to generate spoof-differentiated depth maps, and stably detects spoof faces without being affected by different types of PAs. Moreover, we design a differential normalization procedure to convert original flash frames into differential frames. This simple but effective processing enhances the details in flash frames, aiding in the generation of depth maps. To verify the effectiveness of our framework, we collected a large-scale dataset containing 12,660 live and spoof videos with diverse PAs under dynamic flash from the smartphone screen. Extensive experiments illustrate that the proposed ATR-FAS significantly outperforms existing state-of-the-art methods. The code and dataset will be available at https://github.com/Chaochao-Lin/ATR-FAS.
30.Detect, Augment, Compose, and Adapt: Four Steps for Unsupervised Domain Adaptation in Object Detection
Authors:Mohamed L. Mekhalfi, Davide Boscaini, Fabio Poiesi
Abstract: Unsupervised domain adaptation (UDA) plays a crucial role in object detection when adapting a source-trained detector to a target domain without annotated data. In this paper, we propose a novel and effective four-step UDA approach that leverages self-supervision and trains source and target data concurrently. We harness self-supervised learning to mitigate the lack of ground truth in the target domain. Our method consists of the following steps: (1) identify the region with the highest-confidence set of detections in each target image, which serve as our pseudo-labels; (2) crop the identified region and generate a collection of its augmented versions; (3) combine these latter into a composite image; (4) adapt the network to the target domain using the composed image. Through extensive experiments under cross-camera, cross-weather, and synthetic-to-real scenarios, our approach achieves state-of-the-art performance, improving upon the nearest competitor by more than 2% in terms of mean Average Precision (mAP). The code is available at https://github.com/MohamedTEV/DACA.
31.AnomalyGPT: Detecting Industrial Anomalies using Large Vision-Language Models
Authors:Zhaopeng Gu, Bingke Zhu, Guibo Zhu, Yingying Chen, Ming Tang, Jinqiao Wang
Abstract: Large Vision-Language Models (LVLMs) such as MiniGPT-4 and LLaVA have demonstrated the capability of understanding images and achieved remarkable performance in various visual tasks. Despite their strong abilities in recognizing common objects due to extensive training datasets, they lack specific domain knowledge and have a weaker understanding of localized details within objects, which hinders their effectiveness in the Industrial Anomaly Detection (IAD) task. On the other hand, most existing IAD methods only provide anomaly scores and necessitate the manual setting of thresholds to distinguish between normal and abnormal samples, which restricts their practical implementation. In this paper, we explore the utilization of LVLM to address the IAD problem and propose AnomalyGPT, a novel IAD approach based on LVLM. We generate training data by simulating anomalous images and producing corresponding textual descriptions for each image. We also employ an image decoder to provide fine-grained semantic and design a prompt learner to fine-tune the LVLM using prompt embeddings. Our AnomalyGPT eliminates the need for manual threshold adjustments, thus directly assesses the presence and locations of anomalies. Additionally, AnomalyGPT supports multi-turn dialogues and exhibits impressive few-shot in-context learning capabilities. With only one normal shot, AnomalyGPT achieves the state-of-the-art performance with an accuracy of 86.1%, an image-level AUC of 94.1%, and a pixel-level AUC of 95.3% on the MVTec-AD dataset. Code is available at https://github.com/CASIA-IVA-Lab/AnomalyGPT.
32.Efficient Model Personalization in Federated Learning via Client-Specific Prompt Generation
Authors:Fu-En Yang, Chien-Yi Wang, Yu-Chiang Frank Wang
Abstract: Federated learning (FL) emerges as a decentralized learning framework which trains models from multiple distributed clients without sharing their data to preserve privacy. Recently, large-scale pre-trained models (e.g., Vision Transformer) have shown a strong capability of deriving robust representations. However, the data heterogeneity among clients, the limited computation resources, and the communication bandwidth restrict the deployment of large-scale models in FL frameworks. To leverage robust representations from large-scale models while enabling efficient model personalization for heterogeneous clients, we propose a novel personalized FL framework of client-specific Prompt Generation (pFedPG), which learns to deploy a personalized prompt generator at the server for producing client-specific visual prompts that efficiently adapts frozen backbones to local data distributions. Our proposed framework jointly optimizes the stages of personalized prompt adaptation locally and personalized prompt generation globally. The former aims to train visual prompts that adapt foundation models to each client, while the latter observes local optimization directions to generate personalized prompts for all clients. Through extensive experiments on benchmark datasets, we show that our pFedPG is favorable against state-of-the-art personalized FL methods under various types of data heterogeneity, allowing computation and communication efficient model personalization.
33.On the Robustness of Object Detection Models in Aerial Images
Authors:Haodong He, Jian Ding, Gui-Song Xia
Abstract: The robustness of object detection models is a major concern when applied to real-world scenarios. However, the performance of most object detection models degrades when applied to images subjected to corruptions, since they are usually trained and evaluated on clean datasets. Enhancing the robustness of object detection models is of utmost importance, especially for those designed for aerial images, which feature complex backgrounds, substantial variations in scales and orientations of objects. This paper addresses the challenge of assessing the robustness of object detection models in aerial images, with a specific emphasis on scenarios where images are affected by clouds. In this study, we introduce two novel benchmarks based on DOTA-v1.0. The first benchmark encompasses 19 prevalent corruptions, while the second focuses on cloud-corrupted images-a phenomenon uncommon in natural pictures yet frequent in aerial photography. We systematically evaluate the robustness of mainstream object detection models and perform numerous ablation experiments. Through our investigations, we find that enhanced model architectures, larger networks, well-crafted modules, and judicious data augmentation strategies collectively enhance the robustness of aerial object detection models. The benchmarks we propose and our comprehensive experimental analyses can facilitate research on robust object detection in aerial images. Codes and datasets are available at: (https://github.com/hehaodong530/DOTA-C)
34.Color Aesthetics: Fuzzy based User-driven Method for Harmony and Preference Prediction
Authors:Pakizar Shamoi, Atsushi Inoue, Hiroharu Kawanaka
Abstract: Color is the most important intrinsic sensory feature that has a powerful impact on product sales. Color is even responsible for raising the aesthetic senses in our brains. Account for individual differences is crucial in color aesthetics. It requires user-driven mechanisms for various e-commerce applications. We propose a method for quantitative evaluation of all types of perceptual responses to color(s): distinct color preference, color harmony, and color combination preference. Preference for color schemes can be predicted by combining preferences for the basic colors and ratings of color harmony. Harmonious pallets are extracted from big data set using comparison algorithms based on fuzzy similarity and grouping. The proposed model results in useful predictions of harmony and preference of multicolored images. For example, in the context of apparel coordination, it allows predicting a preference for a look based on clothing colors. Our approach differs from standard aesthetic models, since in accounts for a personal variation. In addition, it can process not only lower-order color pairs, but also groups of several colors.
35.WrappingNet: Mesh Autoencoder via Deep Sphere Deformation
Authors:Eric Lei, Muhammad Asad Lodhi, Jiahao Pang, Junghyun Ahn, Dong Tian
Abstract: There have been recent efforts to learn more meaningful representations via fixed length codewords from mesh data, since a mesh serves as a complete model of underlying 3D shape compared to a point cloud. However, the mesh connectivity presents new difficulties when constructing a deep learning pipeline for meshes. Previous mesh unsupervised learning approaches typically assume category-specific templates, e.g., human face/body templates. It restricts the learned latent codes to only be meaningful for objects in a specific category, so the learned latent spaces are unable to be used across different types of objects. In this work, we present WrappingNet, the first mesh autoencoder enabling general mesh unsupervised learning over heterogeneous objects. It introduces a novel base graph in the bottleneck dedicated to representing mesh connectivity, which is shown to facilitate learning a shared latent space representing object shape. The superiority of WrappingNet mesh learning is further demonstrated via improved reconstruction quality and competitive classification compared to point cloud learning, as well as latent interpolation between meshes of different categories.
36.Complementing Onboard Sensors with Satellite Map: A New Perspective for HD Map Construction
Authors:Wenjie Gao, Jiawei Fu, Haodong Jing, Nanning Zheng
Abstract: High-Definition (HD) maps play a crucial role in autonomous driving systems. Recent methods have attempted to construct HD maps in real-time based on information obtained from vehicle onboard sensors. However, the performance of these methods is significantly susceptible to the environment surrounding the vehicle due to the inherent limitation of onboard sensors, such as weak capacity for long-range detection. In this study, we demonstrate that supplementing onboard sensors with satellite maps can enhance the performance of HD map construction methods, leveraging the broad coverage capability of satellite maps. For the purpose of further research, we release the satellite map tiles as a complementary dataset of nuScenes dataset. Meanwhile, we propose a hierarchical fusion module that enables better fusion of satellite maps information with existing methods. Specifically, we design an attention mask based on segmentation and distance, applying the cross-attention mechanism to fuse onboard Bird's Eye View (BEV) features and satellite features in feature-level fusion. An alignment module is introduced before concatenation in BEV-level fusion to mitigate the impact of misalignment between the two features. The experimental results on the augmented nuScenes dataset showcase the seamless integration of our module into three existing HD map construction methods. It notably enhances their performance in both HD map semantic segmentation and instance detection tasks.
37.Pseudo-Boolean Polynomials Approach To Edge Detection And Image Segmentation
Authors:Tendai Mapungwana Chikake, Boris Goldengorin, Alexey Samosyuk
Abstract: We introduce a deterministic approach to edge detection and image segmentation by formulating pseudo-Boolean polynomials on image patches. The approach works by applying a binary classification of blob and edge regions in an image based on the degrees of pseudo-Boolean polynomials calculated on patches extracted from the provided image. We test our method on simple images containing primitive shapes of constant and contrasting colour and establish the feasibility before applying it to complex instances like aerial landscape images. The proposed method is based on the exploitation of the reduction, polynomial degree, and equivalence properties of penalty-based pseudo-Boolean polynomials.
38.Canonical Factors for Hybrid Neural Fields
Authors:Brent Yi, Weijia Zeng, Sam Buchanan, Yi Ma
Abstract: Factored feature volumes offer a simple way to build more compact, efficient, and intepretable neural fields, but also introduce biases that are not necessarily beneficial for real-world data. In this work, we (1) characterize the undesirable biases that these architectures have for axis-aligned signals -- they can lead to radiance field reconstruction differences of as high as 2 PSNR -- and (2) explore how learning a set of canonicalizing transformations can improve representations by removing these biases. We prove in a two-dimensional model problem that simultaneously learning these transformations together with scene appearance succeeds with drastically improved efficiency. We validate the resulting architectures, which we call TILTED, using image, signed distance, and radiance field reconstruction tasks, where we observe improvements across quality, robustness, compactness, and runtime. Results demonstrate that TILTED can enable capabilities comparable to baselines that are 2x larger, while highlighting weaknesses of neural field evaluation procedures.
39.Online Overexposed Pixels Hallucination in Videos with Adaptive Reference Frame Selection
Authors:Yazhou Xing, Amrita Mazumdar, Anjul Patney, Chao Liu, Hongxu Yin, Qifeng Chen, Jan Kautz, Iuri Frosio
Abstract: Low dynamic range (LDR) cameras cannot deal with wide dynamic range inputs, frequently leading to local overexposure issues. We present a learning-based system to reduce these artifacts without resorting to complex acquisition mechanisms like alternating exposures or costly processing that are typical of high dynamic range (HDR) imaging. We propose a transformer-based deep neural network (DNN) to infer the missing HDR details. In an ablation study, we show the importance of using a multiscale DNN and train it with the proper cost function to achieve state-of-the-art quality. To aid the reconstruction of the overexposed areas, our DNN takes a reference frame from the past as an additional input. This leverages the commonly occurring temporal instabilities of autoexposure to our advantage: since well-exposed details in the current frame may be overexposed in the future, we use reinforcement learning to train a reference frame selection DNN that decides whether to adopt the current frame as a future reference. Without resorting to alternating exposures, we obtain therefore a causal, HDR hallucination algorithm with potential application in common video acquisition settings. Our demo video can be found at https://drive.google.com/file/d/1-r12BKImLOYCLUoPzdebnMyNjJ4Rk360/view
40.Multimodal Contrastive Learning and Tabular Attention for Automated Alzheimer's Disease Prediction
Authors:Weichen Huang
Abstract: Alongside neuroimaging such as MRI scans and PET, Alzheimer's disease (AD) datasets contain valuable tabular data including AD biomarkers and clinical assessments. Existing computer vision approaches struggle to utilize this additional information. To address these needs, we propose a generalizable framework for multimodal contrastive learning of image data and tabular data, a novel tabular attention module for amplifying and ranking salient features in tables, and the application of these techniques onto Alzheimer's disease prediction. Experimental evaulations demonstrate the strength of our framework by detecting Alzheimer's disease (AD) from over 882 MR image slices from the ADNI database. We take advantage of the high interpretability of tabular data and our novel tabular attention approach and through attribution of the attention scores for each row of the table, we note and rank the most predominant features. Results show that the model is capable of an accuracy of over 83.8%, almost a 10% increase from previous state of the art.
41.Learning Modulated Transformation in GANs
Authors:Ceyuan Yang, Qihang Zhang, Yinghao Xu, Jiapeng Zhu, Yujun Shen, Bo Dai
Abstract: The success of style-based generators largely benefits from style modulation, which helps take care of the cross-instance variation within data. However, the instance-wise stochasticity is typically introduced via regular convolution, where kernels interact with features at some fixed locations, limiting its capacity for modeling geometric variation. To alleviate this problem, we equip the generator in generative adversarial networks (GANs) with a plug-and-play module, termed as modulated transformation module (MTM). This module predicts spatial offsets under the control of latent codes, based on which the convolution operation can be applied at variable locations for different instances, and hence offers the model an additional degree of freedom to handle geometry deformation. Extensive experiments suggest that our approach can be faithfully generalized to various generative tasks, including image generation, 3D-aware image synthesis, and video generation, and get compatible with state-of-the-art frameworks without any hyper-parameter tuning. It is noteworthy that, towards human generation on the challenging TaiChi dataset, we improve the FID of StyleGAN3 from 21.36 to 13.60, demonstrating the efficacy of learning modulated geometry transformation.
42.A General-Purpose Self-Supervised Model for Computational Pathology
Authors:Richard J. Chen, Tong Ding, Ming Y. Lu, Drew F. K. Williamson, Guillaume Jaume, Bowen Chen, Andrew Zhang, Daniel Shao, Andrew H. Song, Muhammad Shaban, Mane Williams, Anurag Vaidya, Sharifa Sahai, Lukas Oldenburg, Luca L. Weishaupt, Judy J. Wang, Walt Williams, Long Phi Le, Georg Gerber, Faisal Mahmood
Abstract: Tissue phenotyping is a fundamental computational pathology (CPath) task in learning objective characterizations of histopathologic biomarkers in anatomic pathology. However, whole-slide imaging (WSI) poses a complex computer vision problem in which the large-scale image resolutions of WSIs and the enormous diversity of morphological phenotypes preclude large-scale data annotation. Current efforts have proposed using pretrained image encoders with either transfer learning from natural image datasets or self-supervised pretraining on publicly-available histopathology datasets, but have not been extensively developed and evaluated across diverse tissue types at scale. We introduce UNI, a general-purpose self-supervised model for pathology, pretrained using over 100 million tissue patches from over 100,000 diagnostic haematoxylin and eosin-stained WSIs across 20 major tissue types, and evaluated on 33 representative CPath clinical tasks in CPath of varying diagnostic difficulties. In addition to outperforming previous state-of-the-art models, we demonstrate new modeling capabilities in CPath such as resolution-agnostic tissue classification, slide classification using few-shot class prototypes, and disease subtyping generalization in classifying up to 108 cancer types in the OncoTree code classification system. UNI advances unsupervised representation learning at scale in CPath in terms of both pretraining data and downstream evaluation, enabling data-efficient AI models that can generalize and transfer to a gamut of diagnostically-challenging tasks and clinical workflows in anatomic pathology.
43.3D Adversarial Augmentations for Robust Out-of-Domain Predictions
Authors:Alexander Lehner, Stefano Gasperini, Alvaro Marcos-Ramiro, Michael Schmidt, Nassir Navab, Benjamin Busam, Federico Tombari
Abstract: Since real-world training datasets cannot properly sample the long tail of the underlying data distribution, corner cases and rare out-of-domain samples can severely hinder the performance of state-of-the-art models. This problem becomes even more severe for dense tasks, such as 3D semantic segmentation, where points of non-standard objects can be confidently associated to the wrong class. In this work, we focus on improving the generalization to out-of-domain data. We achieve this by augmenting the training set with adversarial examples. First, we learn a set of vectors that deform the objects in an adversarial fashion. To prevent the adversarial examples from being too far from the existing data distribution, we preserve their plausibility through a series of constraints, ensuring sensor-awareness and shapes smoothness. Then, we perform adversarial augmentation by applying the learned sample-independent vectors to the available objects when training a model. We conduct extensive experiments across a variety of scenarios on data from KITTI, Waymo, and CrashD for 3D object detection, and on data from SemanticKITTI, Waymo, and nuScenes for 3D semantic segmentation. Despite training on a standard single dataset, our approach substantially improves the robustness and generalization of both 3D object detection and 3D semantic segmentation methods to out-of-domain data.