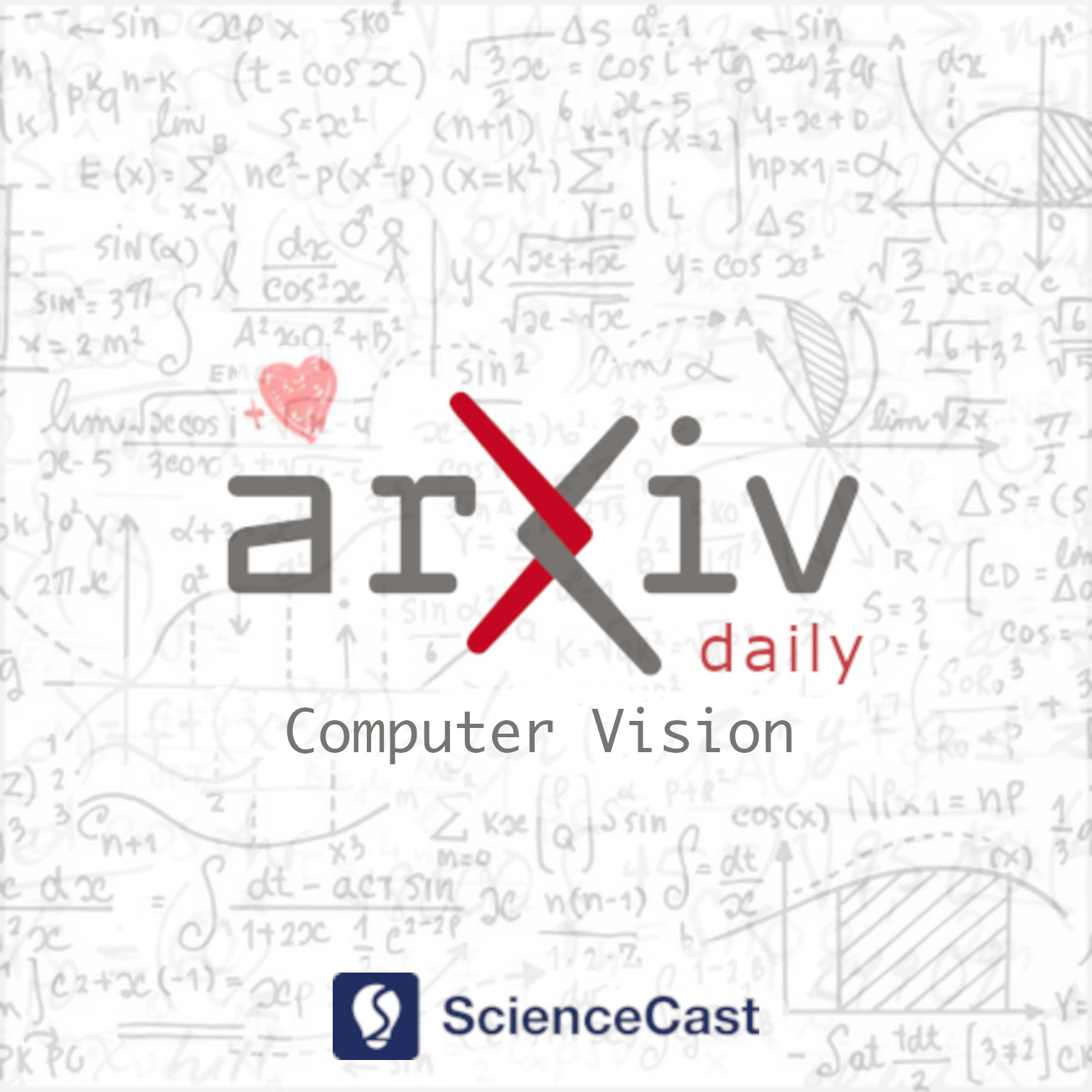
Computer Vision and Pattern Recognition (cs.CV)
Wed, 28 Jun 2023
1.Subclass-balancing Contrastive Learning for Long-tailed Recognition
Authors:Chengkai Hou, Jieyu Zhang, Haonan Wang, Tianyi Zhou
Abstract: Long-tailed recognition with imbalanced class distribution naturally emerges in practical machine learning applications. Existing methods such as data reweighing, resampling, and supervised contrastive learning enforce the class balance with a price of introducing imbalance between instances of head class and tail class, which may ignore the underlying rich semantic substructures of the former and exaggerate the biases in the latter. We overcome these drawbacks by a novel ``subclass-balancing contrastive learning (SBCL)'' approach that clusters each head class into multiple subclasses of similar sizes as the tail classes and enforce representations to capture the two-layer class hierarchy between the original classes and their subclasses. Since the clustering is conducted in the representation space and updated during the course of training, the subclass labels preserve the semantic substructures of head classes. Meanwhile, it does not overemphasize tail class samples, so each individual instance contribute to the representation learning equally. Hence, our method achieves both the instance- and subclass-balance, while the original class labels are also learned through contrastive learning among subclasses from different classes. We evaluate SBCL over a list of long-tailed benchmark datasets and it achieves the state-of-the-art performance. In addition, we present extensive analyses and ablation studies of SBCL to verify its advantages.
2.Multi-network Contrastive Learning Based on Global and Local Representations
Authors:Weiquan Li, Xianzhong Long, Yun Li
Abstract: The popularity of self-supervised learning has made it possible to train models without relying on labeled data, which saves expensive annotation costs. However, most existing self-supervised contrastive learning methods often overlook the combination of global and local feature information. This paper proposes a multi-network contrastive learning framework based on global and local representations. We introduce global and local feature information for self-supervised contrastive learning through multiple networks. The model learns feature information at different scales of an image by contrasting the embedding pairs generated by multiple networks. The framework also expands the number of samples used for contrast and improves the training efficiency of the model. Linear evaluation results on three benchmark datasets show that our method outperforms several existing classical self-supervised learning methods.
3.Boosting Adversarial Transferability with Learnable Patch-wise Masks
Authors:Xingxing Wei, Shiji Zhao
Abstract: Adversarial examples have raised widespread attention in security-critical applications because of their transferability across different models. Although many methods have been proposed to boost adversarial transferability, a gap still exists in the practical demand. In this paper, we argue that the model-specific discriminative regions are a key factor to cause the over-fitting to the source model, and thus reduce the transferability to the target model. For that, a patch-wise mask is utilized to prune the model-specific regions when calculating adversarial perturbations. To accurately localize these regions, we present a learnable approach to optimize the mask automatically. Specifically, we simulate the target models in our framework, and adjust the patch-wise mask according to the feedback of simulated models. To improve the efficiency, Differential Evolutionary (DE) algorithm is utilized to search for patch-wise masks for a specific image. During iterative attacks, the learned masks are applied to the image to drop out the patches related to model-specific regions, thus making the gradients more generic and improving the adversarial transferability. The proposed approach is a pre-processing method and can be integrated with existing gradient-based methods to further boost the transfer attack success rate. Extensive experiments on the ImageNet dataset demonstrate the effectiveness of our method. We incorporate the proposed approach with existing methods in the ensemble attacks and achieve an average success rate of 93.01% against seven advanced defense methods, which can effectively enhance the state-of-the-art transfer-based attack performance.
4.NIPD: A Federated Learning Person Detection Benchmark Based on Real-World Non-IID Data
Authors:Kangning Yin, Zhen Ding, Zhihua Dong, Dongsheng Chen, Jie Fu, Xinhui Ji, Guangqiang Yin, Zhiguo Wang
Abstract: Federated learning (FL), a privacy-preserving distributed machine learning, has been rapidly applied in wireless communication networks. FL enables Internet of Things (IoT) clients to obtain well-trained models while preventing privacy leakage. Person detection can be deployed on edge devices with limited computing power if combined with FL to process the video data directly at the edge. However, due to the different hardware and deployment scenarios of different cameras, the data collected by the camera present non-independent and identically distributed (non-IID), and the global model derived from FL aggregation is less effective. Meanwhile, existing research lacks public data set for real-world FL object detection, which is not conducive to studying the non-IID problem on IoT cameras. Therefore, we open source a non-IID IoT person detection (NIPD) data set, which is collected from five different cameras. To our knowledge, this is the first true device-based non-IID person detection data set. Based on this data set, we explain how to establish a FL experimental platform and provide a benchmark for non-IID person detection. NIPD is expected to promote the application of FL and the security of smart city.
5.Knowledge-Enhanced Hierarchical Information Correlation Learning for Multi-Modal Rumor Detection
Authors:Jiawei Liu, Jingyi Xie, Fanrui Zhang, Qiang Zhang, Zheng-jun Zha
Abstract: The explosive growth of rumors with text and images on social media platforms has drawn great attention. Existing studies have made significant contributions to cross-modal information interaction and fusion, but they fail to fully explore hierarchical and complex semantic correlation across different modality content, severely limiting their performance on detecting multi-modal rumor. In this work, we propose a novel knowledge-enhanced hierarchical information correlation learning approach (KhiCL) for multi-modal rumor detection by jointly modeling the basic semantic correlation and high-order knowledge-enhanced entity correlation. Specifically, KhiCL exploits cross-modal joint dictionary to transfer the heterogeneous unimodality features into the common feature space and captures the basic cross-modal semantic consistency and inconsistency by a cross-modal fusion layer. Moreover, considering the description of multi-modal content is narrated around entities, KhiCL extracts visual and textual entities from images and text, and designs a knowledge relevance reasoning strategy to find the shortest semantic relevant path between each pair of entities in external knowledge graph, and absorbs all complementary contextual knowledge of other connected entities in this path for learning knowledge-enhanced entity representations. Furthermore, KhiCL utilizes a signed attention mechanism to model the knowledge-enhanced entity consistency and inconsistency of intra-modality and inter-modality entity pairs by measuring their corresponding semantic relevant distance. Extensive experiments have demonstrated the effectiveness of the proposed method.
6.Angle Sensitive Pixels for Lensless Imaging on Spherical Sensors
Authors:Yi Hua, Yongyi Zhao, Aswin C. Sankaranarayanan
Abstract: We propose OrbCam, a lensless architecture for imaging with spherical sensors. Prior work in lensless imager techniques have focused largely on using planar sensors; for such designs, it is important to use a modulation element, e.g. amplitude or phase masks, to construct a invertible imaging system. In contrast, we show that the diversity of pixel orientations on a curved surface is sufficient to improve the conditioning of the mapping between the scene and the sensor. Hence, when imaging on a spherical sensor, all pixels can have the same angular response function such that the lensless imager is comprised of pixels that are identical to each other and differ only in their orientations. We provide the computational tools for the design of the angular response of the pixels in a spherical sensor that leads to well-conditioned and noise-robust measurements. We validate our design in both simulation and a lab prototype. The implications of our design is that the lensless imaging can be enabled easily for curved and flexible surfaces thereby opening up a new set of application domains.
7.Bridging the Gap: Neural Collapse Inspired Prompt Tuning for Generalization under Class Imbalance
Authors:Didi Zhu, Yinchuan Li, Min Zhang, Junkun Yuan, Jiashuo Liu, Kun Kuang, Chao Wu
Abstract: Large-scale vision-language (V-L) models have demonstrated remarkable generalization capabilities for downstream tasks through prompt tuning. However, their performance suffers significantly in the presence of class imbalance, a common issue in real-world scenarios. In this paper, we investigate the effects of class imbalance on the generalization performance of V-L models and extend Neural Collapse phenomenon to these models, revealing the geometric reasons behind the impact of class imbalance on their generalization ability. To address this problem, we propose Neural Collapse based Prompt Tuning (NPT), a novel method that optimizes prompts so that both text and image features satisfy the same simplex ETF structure. NPT incorporates two regularization terms, geometric de-biasing and multi-modal isomorphism, to enhance the robustness of V-L models under class imbalance conditions while maintaining their generalization capabilities. Our comprehensive experiments show that NPT outperforms existing prompt learning techniques across 11 diverse image recognition datasets, achieving an absolute average gain of 2.63\% for novel classes and 2.47\% for harmonic mean when facing imbalanced data.
8.A Dimensional Structure based Knowledge Distillation Method for Cross-Modal Learning
Authors:Lingyu Si, Hongwei Dong, Wenwen Qiang, Junzhi Yu, Wenlong Zhai, Changwen Zheng, Fanjiang Xu, Fuchun Sun
Abstract: Due to limitations in data quality, some essential visual tasks are difficult to perform independently. Introducing previously unavailable information to transfer informative dark knowledge has been a common way to solve such hard tasks. However, research on why transferred knowledge works has not been extensively explored. To address this issue, in this paper, we discover the correlation between feature discriminability and dimensional structure (DS) by analyzing and observing features extracted from simple and hard tasks. On this basis, we express DS using deep channel-wise correlation and intermediate spatial distribution, and propose a novel cross-modal knowledge distillation (CMKD) method for better supervised cross-modal learning (CML) performance. The proposed method enforces output features to be channel-wise independent and intermediate ones to be uniformly distributed, thereby learning semantically irrelevant features from the hard task to boost its accuracy. This is especially useful in specific applications where the performance gap between dual modalities is relatively large. Furthermore, we collect a real-world CML dataset to promote community development. The dataset contains more than 10,000 paired optical and radar images and is continuously being updated. Experimental results on real-world and benchmark datasets validate the effectiveness of the proposed method.
9.AFPN: Asymptotic Feature Pyramid Network for Object Detection
Authors:Guoyu Yang, Jie Lei, Zhikuan Zhu, Siyu Cheng, Zunlei Feng, Ronghua Liang
Abstract: Multi-scale features are of great importance in encoding objects with scale variance in object detection tasks. A common strategy for multi-scale feature extraction is adopting the classic top-down and bottom-up feature pyramid networks. However, these approaches suffer from the loss or degradation of feature information, impairing the fusion effect of non-adjacent levels. This paper proposes an asymptotic feature pyramid network (AFPN) to support direct interaction at non-adjacent levels. AFPN is initiated by fusing two adjacent low-level features and asymptotically incorporates higher-level features into the fusion process. In this way, the larger semantic gap between non-adjacent levels can be avoided. Given the potential for multi-object information conflicts to arise during feature fusion at each spatial location, adaptive spatial fusion operation is further utilized to mitigate these inconsistencies. We incorporate the proposed AFPN into both two-stage and one-stage object detection frameworks and evaluate with the MS-COCO 2017 validation and test datasets. Experimental evaluation shows that our method achieves more competitive results than other state-of-the-art feature pyramid networks. The code is available at \href{https://github.com/gyyang23/AFPN}{https://github.com/gyyang23/AFPN}.
10.Positive Label Is All You Need for Multi-Label Classification
Authors:Zhixiang Yuan, Kaixin Zhang, Tao Huang
Abstract: Multi-label classification (MLC) suffers from the inevitable label noise in training data due to the difficulty in annotating various semantic labels in each image. To mitigate the influence of noisy labels, existing methods mainly devote to identifying and correcting the label mistakes via a trained MLC model. However, these methods still involve annoying noisy labels in training, which can result in imprecise recognition of noisy labels and weaken the performance. In this paper, considering that the negative labels are substantially more than positive labels, and most noisy labels are from the negative labels, we directly discard all the negative labels in the dataset, and propose a new method dubbed positive and unlabeled multi-label classification (PU-MLC). By extending positive-unlabeled learning into MLC task, our method trains model with only positive labels and unlabeled data, and introduces adaptive re-balance factor and adaptive temperature coefficient in the loss function to alleviate the catastrophic imbalance in label distribution and over-smoothing of probabilities in training. Our PU-MLC is simple and effective, and it is applicable to both MLC and MLC with partial labels (MLC-PL) tasks. Extensive experiments on MS-COCO and PASCAL VOC datasets demonstrate that our PU-MLC achieves significantly improvements on both MLC and MLC-PL settings with even fewer annotations. Code will be released.
11.Fast Recognition of birds in offshore wind farms based on an improved deep learning model
Authors:Yantong Liu, Xingke Li, Jong-Chan Lee
Abstract: The safety of wind turbines is a prerequisite for the stable operation of offshore wind farms. However, bird damage poses a direct threat to the safe operation of wind turbines and wind turbine blades. In addition, millions of birds are killed by wind turbines every year. In order to protect the ecological environment and maintain the safe operation of offshore wind turbines, and to address the problem of the low detection capability of current target detection algorithms in low-light environments such as at night, this paper proposes a method to improve the network performance by integrating the CBAM attention mechanism and the RetinexNet network into YOLOv5. First, the training set images are fed into the YOLOv5 network with integrated CBAM attention module for training, and the optimal weight model is stored. Then, low-light images are enhanced and denoised using Decom-Net and Enhance-Net, and the accuracy is tested on the optimal weight model. In addition, the k-means++ clustering algorithm is used to optimise the anchor box selection method, which solves the problem of unstable initial centroids and achieves better clustering results. Experimental results show that the accuracy of this model in bird detection tasks can reach 87.40%, an increase of 21.25%. The model can detect birds near wind turbines in real time and shows strong stability in night, rainy and shaky conditions, proving that the model can ensure the safe and stable operation of wind turbines.
12.Points for Energy Renovation (PointER): A LiDAR-Derived Point Cloud Dataset of One Million English Buildings Linked to Energy Characteristics
Authors:Sebastian Krapf, Kevin Mayer, Martin Fischer
Abstract: Rapid renovation of Europe's inefficient buildings is required to reduce climate change. However, analyzing and evaluating buildings at scale is challenging because every building is unique. In current practice, the energy performance of buildings is assessed during on-site visits, which are slow, costly, and local. This paper presents a building point cloud dataset that promotes a data-driven, large-scale understanding of the 3D representation of buildings and their energy characteristics. We generate building point clouds by intersecting building footprints with geo-referenced LiDAR data and link them with attributes from UK's energy performance database via the Unique Property Reference Number (UPRN). To achieve a representative sample, we select one million buildings from a range of rural and urban regions across England, of which half a million are linked to energy characteristics. Building point clouds in new regions can be generated with the open-source code published alongside the paper. The dataset enables novel research in building energy modeling and can be easily expanded to other research fields by adding building features via the UPRN or geo-location.
13.OpenNDD: Open Set Recognition for Neurodevelopmental Disorders Detection
Authors:Jiaming Yu, Zihao Guan, Xinyue Chang, Xiumei Liu, Zhenshan Shi, Changcai Yang, Riqing Chen, Lanyan Xue, Lifang Wei
Abstract: Neurodevelopmental disorders (NDDs) are a highly prevalent group of disorders and represent strong clinical behavioral similarities, and that make it very challenging for accurate identification of different NDDs such as autism spectrum disorder (ASD) and attention-deficit hyperactivity disorder (ADHD). Moreover, there is no reliable physiological markers for NDDs diagnosis and it solely relies on psychological evaluation criteria. However, it is crucial to prevent misdiagnosis and underdiagnosis by intelligent assisted diagnosis, which is closely related to the follow-up corresponding treatment. In order to relieve these issues, we propose a novel open set recognition framework for NDDs screening and detection, which is the first application of open set recognition in this field. It combines auto encoder and adversarial reciprocal points open set recognition to accurately identify known classes as well as recognize classes never encountered. And considering the strong similarities between different subjects, we present a joint scaling method called MMS to distinguish unknown disorders. To validate the feasibility of our presented method, we design a reciprocal opposition experiment protocol on the hybrid datasets from Autism Brain Imaging Data Exchange I (ABIDE I) and THE ADHD-200 SAMPLE (ADHD-200) with 791 samples from four sites and the results demonstrate the superiority on various metrics. Our OpenNDD has achieved promising performance, where the accuracy is 77.38%, AUROC is 75.53% and the open set classification rate is as high as 59.43%.
14.Challenges of Zero-Shot Recognition with Vision-Language Models: Granularity and Correctness
Authors:Zhenlin Xu, Yi Zhu, Tiffany Deng, Abhay Mittal, Yanbei Chen, Manchen Wang, Paolo Favaro, Joseph Tighe, Davide Modolo
Abstract: This paper investigates the challenges of applying vision-language models (VLMs) to zero-shot visual recognition tasks in an open-world setting, with a focus on contrastive vision-language models such as CLIP. We first examine the performance of VLMs on concepts of different granularity levels. We propose a way to fairly evaluate the performance discrepancy under two experimental setups and find that VLMs are better at recognizing fine-grained concepts. Furthermore, we find that the similarity scores from VLMs do not strictly reflect the correctness of the textual inputs given visual input. We propose an evaluation protocol to test our hypothesis that the scores can be biased towards more informative descriptions, and the nature of the similarity score between embedding makes it challenging for VLMs to recognize the correctness between similar but wrong descriptions. Our study highlights the challenges of using VLMs in open-world settings and suggests directions for future research to improve their zero-shot capabilities.
15.Evaluating Similitude and Robustness of Deep Image Denoising Models via Adversarial Attack
Authors:Jie Ning, Yao Li, Zhichang Guo
Abstract: Deep neural networks (DNNs) have a wide range of applications in the field of image denoising, and they are superior to traditional image denoising. However, DNNs inevitably show vulnerability, which is the weak robustness in the face of adversarial attacks. In this paper, we find some similitudes between existing deep image denoising methods, as they are consistently fooled by adversarial attacks. First, denoising-PGD is proposed which is a denoising model full adversarial method. The current mainstream non-blind denoising models (DnCNN, FFDNet, ECNDNet, BRDNet), blind denoising models (DnCNN-B, Noise2Noise, RDDCNN-B, FAN), and plug-and-play (DPIR, CurvPnP) and unfolding denoising models (DeamNet) applied to grayscale and color images can be attacked by the same set of methods. Second, since the transferability of denoising-PGD is prominent in the image denoising task, we design experiments to explore the characteristic of the latent under the transferability. We correlate transferability with similitude and conclude that the deep image denoising models have high similitude. Third, we investigate the characteristic of the adversarial space and use adversarial training to complement the vulnerability of deep image denoising to adversarial attacks on image denoising. Finally, we constrain this adversarial attack method and propose the L2-denoising-PGD image denoising adversarial attack method that maintains the Gaussian distribution. Moreover, the model-driven image denoising BM3D shows some resistance in the face of adversarial attacks.
16.SVNR: Spatially-variant Noise Removal with Denoising Diffusion
Authors:Naama Pearl, Yaron Brodsky, Dana Berman, Assaf Zomet, Alex Rav Acha, Daniel Cohen-Or, Dani Lischinski
Abstract: Denoising diffusion models have recently shown impressive results in generative tasks. By learning powerful priors from huge collections of training images, such models are able to gradually modify complete noise to a clean natural image via a sequence of small denoising steps, seemingly making them well-suited for single image denoising. However, effectively applying denoising diffusion models to removal of realistic noise is more challenging than it may seem, since their formulation is based on additive white Gaussian noise, unlike noise in real-world images. In this work, we present SVNR, a novel formulation of denoising diffusion that assumes a more realistic, spatially-variant noise model. SVNR enables using the noisy input image as the starting point for the denoising diffusion process, in addition to conditioning the process on it. To this end, we adapt the diffusion process to allow each pixel to have its own time embedding, and propose training and inference schemes that support spatially-varying time maps. Our formulation also accounts for the correlation that exists between the condition image and the samples along the modified diffusion process. In our experiments we demonstrate the advantages of our approach over a strong diffusion model baseline, as well as over a state-of-the-art single image denoising method.
17.Dynamic Path-Controllable Deep Unfolding Network for Compressive Sensing
Authors:Jiechong Song, Bin Chen, Jian Zhang
Abstract: Deep unfolding network (DUN) that unfolds the optimization algorithm into a deep neural network has achieved great success in compressive sensing (CS) due to its good interpretability and high performance. Each stage in DUN corresponds to one iteration in optimization. At the test time, all the sampling images generally need to be processed by all stages, which comes at a price of computation burden and is also unnecessary for the images whose contents are easier to restore. In this paper, we focus on CS reconstruction and propose a novel Dynamic Path-Controllable Deep Unfolding Network (DPC-DUN). DPC-DUN with our designed path-controllable selector can dynamically select a rapid and appropriate route for each image and is slimmable by regulating different performance-complexity tradeoffs. Extensive experiments show that our DPC-DUN is highly flexible and can provide excellent performance and dynamic adjustment to get a suitable tradeoff, thus addressing the main requirements to become appealing in practice. Codes are available at https://github.com/songjiechong/DPC-DUN.
18.A serial dual-channel library occupancy detection system based on Faster RCNN
Authors:Guoqiang Yang, Xiaowen Chang, Zitong Wang, Min Yang, Xin Chen
Abstract: The phenomenon of seat occupancy in university libraries is a prevalent issue. However, existing solutions, such as software-based seat reservations and sensors-based occupancy detection, have proven to be inadequate in effectively addressing this problem. In this study, we propose a novel approach: a serial dual-channel object detection model based on Faster RCNN. Furthermore, we develop a user-friendly Web interface and mobile APP to create a computer vision-based platform for library seat occupancy detection. To construct our dataset, we combine real-world data collec-tion with UE5 virtual reality. The results of our tests also demonstrate that the utilization of per-sonalized virtual dataset significantly enhances the performance of the convolutional neural net-work (CNN) in dedicated scenarios. The serial dual-channel detection model comprises three es-sential steps. Firstly, we employ Faster RCNN algorithm to determine whether a seat is occupied by an individual. Subsequently, we utilize an object classification algorithm based on transfer learning, to classify and identify images of unoccupied seats. This eliminates the need for manual judgment regarding whether a person is suspected of occupying a seat. Lastly, the Web interface and APP provide seat information to librarians and students respectively, enabling comprehensive services. By leveraging deep learning methodologies, this research effectively addresses the issue of seat occupancy in library systems. It significantly enhances the accuracy of seat occupancy recognition, reduces the computational resources required for training CNNs, and greatly improves the effi-ciency of library seat management.
19.Lifelong Change Detection: Continuous Domain Adaptation for Small Object Change Detection in Every Robot Navigation
Authors:Koji Takeda, Kanji Tanaka, Yoshimasa Nakamura
Abstract: The recently emerging research area in robotics, ground view change detection, suffers from its ill-posed-ness because of visual uncertainty combined with complex nonlinear perspective projection. To regularize the ill-posed-ness, the commonly applied supervised learning methods (e.g., CSCD-Net) rely on manually annotated high-quality object-class-specific priors. In this work, we consider general application domains where no manual annotation is available and present a fully self-supervised approach. The present approach adopts the powerful and versatile idea that object changes detected during everyday robot navigation can be reused as additional priors to improve future change detection tasks. Furthermore, a robustified framework is implemented and verified experimentally in a new challenging practical application scenario: ground-view small object change detection.
20.Fast Marching Energy CNN
Authors:Nicolas Makaroff, Théo Bertrand, Laurent D. Cohen
Abstract: Leveraging geodesic distances and the geometrical information they convey is key for many data-oriented applications in imaging. Geodesic distance computation has been used for long for image segmentation using Image based metrics. We introduce a new method by generating isotropic Riemannian metrics adapted to a problem using CNN and give as illustrations an example of application. We then apply this idea to the segmentation of brain tumours as unit balls for the geodesic distance computed with the metric potential output by a CNN, thus imposing geometrical and topological constraints on the output mask. We show that geodesic distance modules work well in machine learning frameworks and can be used to achieve state-of-the-art performances while ensuring geometrical and/or topological properties.
21.Real-World Performance of Autonomously Reporting Normal Chest Radiographs in NHS Trusts Using a Deep-Learning Algorithm on the GP Pathway
Authors:Jordan Smith, Tom Naunton Morgan, Paul Williams, Qaiser Malik, Simon Rasalingham
Abstract: AIM To analyse the performance of a deep-learning (DL) algorithm currently deployed as diagnostic decision support software in two NHS Trusts used to identify normal chest x-rays in active clinical pathways. MATERIALS AND METHODS A DL algorithm has been deployed in Somerset NHS Foundation Trust (SFT) since December 2022, and at Calderdale & Huddersfield NHS Foundation Trust (CHFT) since March 2023. The algorithm was developed and trained prior to deployment, and is used to assign abnormality scores to each GP-requested chest x-ray (CXR). The algorithm classifies a subset of examinations with the lowest abnormality scores as High Confidence Normal (HCN), and displays this result to the Trust. This two-site study includes 4,654 CXR continuous examinations processed by the algorithm over a six-week period. RESULTS When classifying 20.0% of assessed examinations (930) as HCN, the model classified exams with a negative predictive value (NPV) of 0.96. There were 0.77% of examinations (36) classified incorrectly as HCN, with none of the abnormalities considered clinically significant by auditing radiologists. The DL software maintained fast levels of service to clinicians, with results returned to Trusts in a mean time of 7.1 seconds. CONCLUSION The DL algorithm performs with a low rate of error and is highly effective as an automated diagnostic decision support tool, used to autonomously report a subset of CXRs as normal with high confidence. Removing 20% of all CXRs reduces workload for reporters and allows radiology departments to focus resources elsewhere.
22.Semantic Positive Pairs for Enhancing Contrastive Instance Discrimination
Authors:Mohammad Alkhalefi, Georgios Leontidis, Mingjun Zhong
Abstract: Self-supervised learning algorithms based on instance discrimination effectively prevent representation collapse and produce promising results in representation learning. However, the process of attracting positive pairs (i.e., two views of the same instance) in the embedding space and repelling all other instances (i.e., negative pairs) irrespective of their categories could result in discarding important features. To address this issue, we propose an approach to identifying those images with similar semantic content and treating them as positive instances, named semantic positive pairs set (SPPS), thereby reducing the risk of discarding important features during representation learning. Our approach could work with any contrastive instance discrimination framework such as SimCLR or MOCO. We conduct experiments on three datasets: ImageNet, STL-10 and CIFAR-10 to evaluate our approach. The experimental results show that our approach consistently outperforms the baseline method vanilla SimCLR across all three datasets; for example, our approach improves upon vanilla SimCLR under linear evaluation protocol by 4.18% on ImageNet with a batch size 1024 and 800 epochs.
23.Distributional Modeling for Location-Aware Adversarial Patches
Authors:Xingxing Wei, Shouwei Ruan, Yinpeng Dong, Hang Su
Abstract: Adversarial patch is one of the important forms of performing adversarial attacks in the physical world. To improve the naturalness and aggressiveness of existing adversarial patches, location-aware patches are proposed, where the patch's location on the target object is integrated into the optimization process to perform attacks. Although it is effective, efficiently finding the optimal location for placing the patches is challenging, especially under the black-box attack settings. In this paper, we propose the Distribution-Optimized Adversarial Patch (DOPatch), a novel method that optimizes a multimodal distribution of adversarial locations instead of individual ones. DOPatch has several benefits: Firstly, we find that the locations' distributions across different models are pretty similar, and thus we can achieve efficient query-based attacks to unseen models using a distributional prior optimized on a surrogate model. Secondly, DOPatch can generate diverse adversarial samples by characterizing the distribution of adversarial locations. Thus we can improve the model's robustness to location-aware patches via carefully designed Distributional-Modeling Adversarial Training (DOP-DMAT). We evaluate DOPatch on various face recognition and image recognition tasks and demonstrate its superiority and efficiency over existing methods. We also conduct extensive ablation studies and analyses to validate the effectiveness of our method and provide insights into the distribution of adversarial locations.
24.INSTA-BEEER: Explicit Error Estimation and Refinement for Fast and Accurate Unseen Object Instance Segmentation
Authors:Seunghyeok Back, Sangbeom Lee, Kangmin Kim, Joosoon Lee, Sungho Shin, Jaemo Maeng, Kyoobin Lee
Abstract: Efficient and accurate segmentation of unseen objects is crucial for robotic manipulation. However, it remains challenging due to over- or under-segmentation. Although existing refinement methods can enhance the segmentation quality, they fix only minor boundary errors or are not sufficiently fast. In this work, we propose INSTAnce Boundary Explicit Error Estimation and Refinement (INSTA-BEEER), a novel refinement model that allows for adding and deleting instances and sharpening boundaries. Leveraging an error-estimation-then-refinement scheme, the model first estimates the pixel-wise boundary explicit errors: true positive, true negative, false positive, and false negative pixels of the instance boundary in the initial segmentation. It then refines the initial segmentation using these error estimates as guidance. Experiments show that the proposed model significantly enhances segmentation, achieving state-of-the-art performance. Furthermore, with a fast runtime (less than 0.1 s), the model consistently improves performance across various initial segmentation methods, making it highly suitable for practical robotic applications.
25.$\mathbf{C}^2$Former: Calibrated and Complementary Transformer for RGB-Infrared Object Detection
Authors:Maoxun Yuan, Xingxing Wei
Abstract: Object detection on visible (RGB) and infrared (IR) images, as an emerging solution to facilitate robust detection for around-the-clock applications, has received extensive attention in recent years. With the help of IR images, object detectors have been more reliable and robust in practical applications by using RGB-IR combined information. However, existing methods still suffer from modality miscalibration and fusion imprecision problems. Since transformer has the powerful capability to model the pairwise correlations between different features, in this paper, we propose a novel Calibrated and Complementary Transformer called $\mathrm{C}^2$Former to address these two problems simultaneously. In $\mathrm{C}^2$Former, we design an Inter-modality Cross-Attention (ICA) module to obtain the calibrated and complementary features by learning the cross-attention relationship between the RGB and IR modality. To reduce the computational cost caused by computing the global attention in ICA, an Adaptive Feature Sampling (AFS) module is introduced to decrease the dimension of feature maps. Because $\mathrm{C}^2$Former performs in the feature domain, it can be embedded into existed RGB-IR object detectors via the backbone network. Thus, one single-stage and one two-stage object detector both incorporating our $\mathrm{C}^2$Former are constructed to evaluate its effectiveness and versatility. With extensive experiments on the DroneVehicle and KAIST RGB-IR datasets, we verify that our method can fully utilize the RGB-IR complementary information and achieve robust detection results. The code is available at https://github.com/yuanmaoxun/Calibrated-and-Complementary-Transformer-for-RGB-Infrared-Object-Detection.git.
26.Pseudo-Bag Mixup Augmentation for Multiple Instance Learning Based Whole Slide Image Classification
Authors:Pei Liu, Luping Ji, Xinyu Zhang, Feng Ye
Abstract: Given the special situation of modeling gigapixel images, multiple instance learning (MIL) has become one of the most important frameworks for Whole Slide Image (WSI) classification. In current practice, most MIL networks often face two unavoidable problems in training: i) insufficient WSI data, and ii) the data memorization nature inherent in neural networks. These problems may hinder MIL models from adequate and efficient training, suppressing the continuous performance promotion of classification models on WSIs. Inspired by the basic idea of Mixup, this paper proposes a Pseudo-bag Mixup (PseMix) data augmentation scheme to improve the training of MIL models. This scheme generalizes the Mixup strategy for general images to special WSIs via pseudo-bags so as to be applied in MIL-based WSI classification. Cooperated by pseudo-bags, our PseMix fulfills the critical size alignment and semantic alignment in Mixup strategy. Moreover, it is designed as an efficient and decoupled method adaptive to MIL, neither involving time-consuming operations nor relying on MIL model predictions. Comparative experiments and ablation studies are specially designed to evaluate the effectiveness and advantages of our PseMix. Test results show that PseMix could often improve the performance of MIL networks in WSI classification. Besides, it could also boost the generalization capacity of MIL models, and promote their robustness to patch occlusion and noisy labels. Our source code is available at https://github.com/liupei101/PseMix.
27.Learning to Pan-sharpening with Memories of Spatial Details
Authors:Maoxun Yuan, Tianyi Zhao, Bo Li, Xingxing Wei
Abstract: Pan-sharpening, as one of the most commonly used techniques in remote sensing systems, aims to inject spatial details from panchromatic images into multi-spectral images to obtain high-resolution MS images. Since deep learning has received widespread attention because of its powerful fitting ability and efficient feature extraction, a variety of pan-sharpening methods have been proposed to achieve remarkable performance. However, current pan-sharpening methods usually require the paired PAN and MS images as the input, which limits their usage in some scenarios. To address this issue, in this paper, we observe that the spatial details from PAN images are mainly high-frequency cues, i.e., the edges reflect the contour of input PAN images. This motivates us to develop a PAN-agnostic representation to store some base edges, so as to compose the contour for the corresponding PAN image via them. As a result, we can perform the pan-sharpening task with only the MS image when inference. To this end, a memory-based network is adapted to extract and memorize the spatial details during the training phase and is used to replace the process of obtaining spatial information from PAN images when inference, which is called Memory-based Spatial Details Network (MSDN). We finally integrate the proposed MSDN module into the existing DL-based pan-sharpening methods to achieve an end-to-end pan-sharpening network. With extensive experiments on the Gaofen1 and WorldView-4 satellites, we verify that our method constructs good spatial details without PAN images and achieves the best performance. The code is available at https://github.com/Zhao-Tian-yi/Learning-to-Pan-sharpening-with-Memories-of-Spatial-Details.git.
28.Effective Transfer of Pretrained Large Visual Model for Fabric Defect Segmentation via Specifc Knowledge Injection
Authors:Zhewei Chen, Wai Keung Wong, Zuofeng Zhong, Jinpiao Liao, Ying Qu
Abstract: Fabric defect segmentation is integral to textile quality control. Despite this, the scarcity of high-quality annotated data and the diversity of fabric defects present significant challenges to the application of deep learning in this field. These factors limit the generalization and segmentation performance of existing models, impeding their ability to handle the complexity of diverse fabric types and defects. To overcome these obstacles, this study introduces an innovative method to infuse specialized knowledge of fabric defects into the Segment Anything Model (SAM), a large-scale visual model. By introducing and training a unique set of fabric defect-related parameters, this approach seamlessly integrates domain-specific knowledge into SAM without the need for extensive modifications to the pre-existing model parameters. The revamped SAM model leverages generalized image understanding learned from large-scale natural image datasets while incorporating fabric defect-specific knowledge, ensuring its proficiency in fabric defect segmentation tasks. The experimental results reveal a significant improvement in the model's segmentation performance, attributable to this novel amalgamation of generic and fabric-specific knowledge. When benchmarking against popular existing segmentation models across three datasets, our proposed model demonstrates a substantial leap in performance. Its impressive results in cross-dataset comparisons and few-shot learning experiments further demonstrate its potential for practical applications in textile quality control.
29.Multi-IMU with Online Self-Consistency for Freehand 3D Ultrasound Reconstruction
Authors:Mingyuan Luo, Xin Yang, Zhongnuo Yan, Yuanji Zhang, Junyu Li, Jiongquan Chen, Xindi Hu, Jikuan Qian, Jun Cheng, Dong Ni
Abstract: Ultrasound (US) imaging is a popular tool in clinical diagnosis, offering safety, repeatability, and real-time capabilities. Freehand 3D US is a technique that provides a deeper understanding of scanned regions without increasing complexity. However, estimating elevation displacement and accumulation error remains challenging, making it difficult to infer the relative position using images alone. The addition of external lightweight sensors has been proposed to enhance reconstruction performance without adding complexity, which has been shown to be beneficial. We propose a novel online self-consistency network (OSCNet) using multiple inertial measurement units (IMUs) to improve reconstruction performance. OSCNet utilizes a modal-level self-supervised strategy to fuse multiple IMU information and reduce differences between reconstruction results obtained from each IMU data. Additionally, a sequence-level self-consistency strategy is proposed to improve the hierarchical consistency of prediction results among the scanning sequence and its sub-sequences. Experiments on large-scale arm and carotid datasets with multiple scanning tactics demonstrate that our OSCNet outperforms previous methods, achieving state-of-the-art reconstruction performance.
30.Low-Confidence Samples Mining for Semi-supervised Object Detection
Authors:Guandu Liu, Fangyuan Zhang, Tianxiang Pan, Bin Wang
Abstract: Reliable pseudo-labels from unlabeled data play a key role in semi-supervised object detection (SSOD). However, the state-of-the-art SSOD methods all rely on pseudo-labels with high confidence, which ignore valuable pseudo-labels with lower confidence. Additionally, the insufficient excavation for unlabeled data results in an excessively low recall rate thus hurting the network training. In this paper, we propose a novel Low-confidence Samples Mining (LSM) method to utilize low-confidence pseudo-labels efficiently. Specifically, we develop an additional pseudo information mining (PIM) branch on account of low-resolution feature maps to extract reliable large-area instances, the IoUs of which are higher than small-area ones. Owing to the complementary predictions between PIM and the main branch, we further design self-distillation (SD) to compensate for both in a mutually-learning manner. Meanwhile, the extensibility of the above approaches enables our LSM to apply to Faster-RCNN and Deformable-DETR respectively. On the MS-COCO benchmark, our method achieves 3.54% mAP improvement over state-of-the-art methods under 5% labeling ratios.
31.Land Cover Segmentation with Sparse Annotations from Sentinel-2 Imagery
Authors:Marco Galatola, Edoardo Arnaudo, Luca Barco, Claudio Rossi, Fabrizio Dominici
Abstract: Land cover (LC) segmentation plays a critical role in various applications, including environmental analysis and natural disaster management. However, generating accurate LC maps is a complex and time-consuming task that requires the expertise of multiple annotators and regular updates to account for environmental changes. In this work, we introduce SPADA, a framework for fuel map delineation that addresses the challenges associated with LC segmentation using sparse annotations and domain adaptation techniques for semantic segmentation. Performance evaluations using reliable ground truths, such as LUCAS and Urban Atlas, demonstrate the technique's effectiveness. SPADA outperforms state-of-the-art semantic segmentation approaches as well as third-party products, achieving a mean Intersection over Union (IoU) score of 42.86 and an F1 score of 67.93 on Urban Atlas and LUCAS, respectively.
32.RSPrompter: Learning to Prompt for Remote Sensing Instance Segmentation based on Visual Foundation Model
Authors:Keyan Chen, Chenyang Liu, Hao Chen, Haotian Zhang, Wenyuan Li, Zhengxia Zou, Zhenwei Shi
Abstract: Leveraging vast training data (SA-1B), the foundation Segment Anything Model (SAM) proposed by Meta AI Research exhibits remarkable generalization and zero-shot capabilities. Nonetheless, as a category-agnostic instance segmentation method, SAM heavily depends on prior manual guidance involving points, boxes, and coarse-grained masks. Additionally, its performance on remote sensing image segmentation tasks has yet to be fully explored and demonstrated. In this paper, we consider designing an automated instance segmentation approach for remote sensing images based on the SAM foundation model, incorporating semantic category information. Inspired by prompt learning, we propose a method to learn the generation of appropriate prompts for SAM input. This enables SAM to produce semantically discernible segmentation results for remote sensing images, which we refer to as RSPrompter. We also suggest several ongoing derivatives for instance segmentation tasks, based on recent developments in the SAM community, and compare their performance with RSPrompter. Extensive experimental results on the WHU building, NWPU VHR-10, and SSDD datasets validate the efficacy of our proposed method. Our code is accessible at \url{https://kyanchen.github.io/RSPrompter}.
33.Point2Point : A Framework for Efficient Deep Learning on Hilbert sorted Point Clouds with applications in Spatio-Temporal Occupancy Prediction
Authors:Athrva Atul Pandhare
Abstract: The irregularity and permutation invariance of point cloud data pose challenges for effective learning. Conventional methods for addressing this issue involve converting raw point clouds to intermediate representations such as 3D voxel grids or range images. While such intermediate representations solve the problem of permutation invariance, they can result in significant loss of information. Approaches that do learn on raw point clouds either have trouble in resolving neighborhood relationships between points or are too complicated in their formulation. In this paper, we propose a novel approach to representing point clouds as a locality preserving 1D ordering induced by the Hilbert space-filling curve. We also introduce Point2Point, a neural architecture that can effectively learn on Hilbert-sorted point clouds. We show that Point2Point shows competitive performance on point cloud segmentation and generation tasks. Finally, we show the performance of Point2Point on Spatio-temporal Occupancy prediction from Point clouds.
34.DiffComplete: Diffusion-based Generative 3D Shape Completion
Authors:Ruihang Chu, Enze Xie, Shentong Mo, Zhenguo Li, Matthias Nießner, Chi-Wing Fu, Jiaya Jia
Abstract: We introduce a new diffusion-based approach for shape completion on 3D range scans. Compared with prior deterministic and probabilistic methods, we strike a balance between realism, multi-modality, and high fidelity. We propose DiffComplete by casting shape completion as a generative task conditioned on the incomplete shape. Our key designs are two-fold. First, we devise a hierarchical feature aggregation mechanism to inject conditional features in a spatially-consistent manner. So, we can capture both local details and broader contexts of the conditional inputs to control the shape completion. Second, we propose an occupancy-aware fusion strategy in our model to enable the completion of multiple partial shapes and introduce higher flexibility on the input conditions. DiffComplete sets a new SOTA performance (e.g., 40% decrease on l_1 error) on two large-scale 3D shape completion benchmarks. Our completed shapes not only have a realistic outlook compared with the deterministic methods but also exhibit high similarity to the ground truths compared with the probabilistic alternatives. Further, DiffComplete has strong generalizability on objects of entirely unseen classes for both synthetic and real data, eliminating the need for model re-training in various applications.
35.Theater Aid System for the Visually Impaired Through Transfer Learning of Spatio-Temporal Graph Convolution Networks
Authors:Leyla Benhamida, Slimane Larabi
Abstract: The aim of this research is to recognize human actions performed on stage to aid visually impaired and blind individuals. To achieve this, we have created a theatre human action recognition system that uses skeleton data captured by depth image as input. We collected new samples of human actions in a theatre environment, and then tested the transfer learning technique with three pre-trained Spatio-Temporal Graph Convolution Networks for skeleton-based human action recognition: the spatio-temporal graph convolution network, the two-stream adaptive graph convolution network, and the multi-scale disentangled unified graph convolution network. We selected the NTU-RGBD human action benchmark as the source domain and used our collected dataset as the target domain. We analyzed the transferability of the pre-trained models and proposed two configurations to apply and adapt the transfer learning technique to the diversity between the source and target domains. The use of transfer learning helped to improve the performance of the human action system within the context of theatre. The results indicate that Spatio-Temporal Graph Convolution Networks is positively transferred, and there was an improvement in performance compared to the baseline without transfer learning.