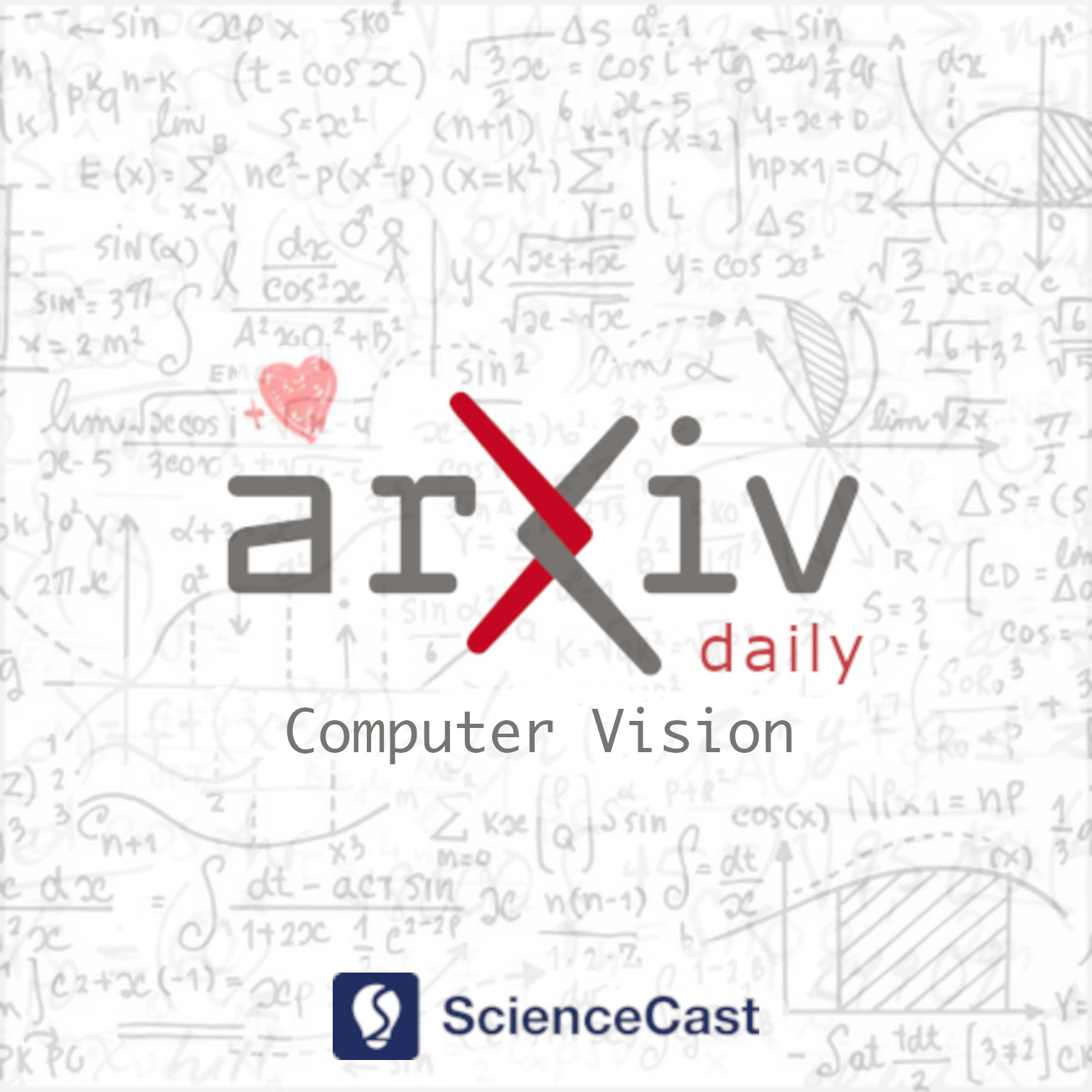
Computer Vision and Pattern Recognition (cs.CV)
Thu, 27 Apr 2023
1.Automatic Localization and Detection Applicable to Robust Image Watermarking Resisting against Camera Shooting
Authors:Ming Liu
Abstract: Robust image watermarking that can resist camera shooting has become an active research topic in recent years due to the increasing demand for preventing sensitive information displayed on computer screens from being captured. However, many mainstream schemes require human assistance during the watermark detection process and cannot adapt to scenarios that require processing a large number of images. Although deep learning-based schemes enable end-to-end watermark embedding and detection, their limited generalization ability makes them vulnerable to failure in complex scenarios. In this paper, we propose a carefully crafted watermarking system that can resist camera shooting. The proposed scheme deals with two important problems: automatic watermark localization (AWL) and automatic watermark detection (AWD). AWL automatically identifies the region of interest (RoI), which contains watermark information, in the camera-shooting image by analyzing the local statistical characteristics. Meanwhile, AWD extracts the hidden watermark from the identified RoI after applying perspective correction. Compared with previous works, the proposed scheme is fully automatic, making it ideal for application scenarios. Furthermore, the proposed scheme is not limited to any specific watermark embedding strategy, allowing for improvements in the watermark embedding and extraction procedure. Extensive experimental results and analysis show that the embedded watermark can be automatically and reliably extracted from the camera-shooting image in different scenarios, demonstrating the superiority and applicability of the proposed approach.
2.Human-machine knowledge hybrid augmentation method for surface defect detection based few-data learning
Authors:Yu Gonga, Xiaoqiao Wanga, Chichun Zhou
Abstract: Visual-based defect detection is a crucial but challenging task in industrial quality control. Most mainstream methods rely on large amounts of existing or related domain data as auxiliary information. However, in actual industrial production, there are often multi-batch, low-volume manufacturing scenarios with rapidly changing task demands, making it difficult to obtain sufficient and diverse defect data. This paper proposes a parallel solution that uses a human-machine knowledge hybrid augmentation method to help the model extract unknown important features. Specifically, by incorporating experts' knowledge of abnormality to create data with rich features, positions, sizes, and backgrounds, we can quickly accumulate an amount of data from scratch and provide it to the model as prior knowledge for few-data learning. The proposed method was evaluated on the magnetic tile dataset and achieved F1-scores of 60.73%, 70.82%, 77.09%, and 82.81% when using 2, 5, 10, and 15 training images, respectively. Compared to the traditional augmentation method's F1-score of 64.59%, the proposed method achieved an 18.22% increase in the best result, demonstrating its feasibility and effectiveness in few-data industrial defect detection.
3.SkinSAM: Empowering Skin Cancer Segmentation with Segment Anything Model
Authors:Mingzhe Hu, Yuheng Li, Xiaofeng Yang
Abstract: Skin cancer is a prevalent and potentially fatal disease that requires accurate and efficient diagnosis and treatment. Although manual tracing is the current standard in clinics, automated tools are desired to reduce human labor and improve accuracy. However, developing such tools is challenging due to the highly variable appearance of skin cancers and complex objects in the background. In this paper, we present SkinSAM, a fine-tuned model based on the Segment Anything Model that showed outstanding segmentation performance. The models are validated on HAM10000 dataset which includes 10015 dermatoscopic images. While larger models (ViT_L, ViT_H) performed better than the smaller one (ViT_b), the finetuned model (ViT_b_finetuned) exhibited the greatest improvement, with a Mean pixel accuracy of 0.945, Mean dice score of 0.8879, and Mean IoU score of 0.7843. Among the lesion types, vascular lesions showed the best segmentation results. Our research demonstrates the great potential of adapting SAM to medical image segmentation tasks.
4.Adaptive-Mask Fusion Network for Segmentation of Drivable Road and Negative Obstacle With Untrustworthy Features
Authors:Zhen Feng, Yuchao Feng, Yanning Guo, Yuxiang Sun
Abstract: Segmentation of drivable roads and negative obstacles is critical to the safe driving of autonomous vehicles. Currently, many multi-modal fusion methods have been proposed to improve segmentation accuracy, such as fusing RGB and depth images. However, we find that when fusing two modals of data with untrustworthy features, the performance of multi-modal networks could be degraded, even lower than those using a single modality. In this paper, the untrustworthy features refer to those extracted from regions (e.g., far objects that are beyond the depth measurement range) with invalid depth data (i.e., 0 pixel value) in depth images. The untrustworthy features can confuse the segmentation results, and hence lead to inferior results. To provide a solution to this issue, we propose the Adaptive-Mask Fusion Network (AMFNet) by introducing adaptive-weight masks in the fusion module to fuse features from RGB and depth images with inconsistency. In addition, we release a large-scale RGB-depth dataset with manually-labeled ground truth based on the NPO dataset for drivable roads and negative obstacles segmentation. Extensive experimental results demonstrate that our network achieves state-of-the-art performance compared with other networks. Our code and dataset are available at: https://github.com/lab-sun/AMFNet.
5.A Review of Panoptic Segmentation for Mobile Mapping Point Clouds
Authors:Binbin Xiang, Yuanwen Yue, Torben Peters, Konrad Schindler
Abstract: 3D point cloud panoptic segmentation is the combined task to (i) assign each point to a semantic class and (ii) separate the points in each class into object instances. Recently there has been an increased interest in such comprehensive 3D scene understanding, building on the rapid advances of semantic segmentation due to the advent of deep 3D neural networks. Yet, to date there is very little work about panoptic segmentation of outdoor mobile-mapping data, and no systematic comparisons. The present paper tries to close that gap. It reviews the building blocks needed to assemble a panoptic segmentation pipeline and the related literature. Moreover, a modular pipeline is set up to perform comprehensive, systematic experiments to assess the state of panoptic segmentation in the context of street mapping. As a byproduct, we also provide the first public dataset for that task, by extending the NPM3D dataset to include instance labels.
6.Optimization-Inspired Cross-Attention Transformer for Compressive Sensing
Authors:Jiechong Song, Chong Mou, Shiqi Wang, Siwei Ma, Jian Zhang
Abstract: By integrating certain optimization solvers with deep neural networks, deep unfolding network (DUN) with good interpretability and high performance has attracted growing attention in compressive sensing (CS). However, existing DUNs often improve the visual quality at the price of a large number of parameters and have the problem of feature information loss during iteration. In this paper, we propose an Optimization-inspired Cross-attention Transformer (OCT) module as an iterative process, leading to a lightweight OCT-based Unfolding Framework (OCTUF) for image CS. Specifically, we design a novel Dual Cross Attention (Dual-CA) sub-module, which consists of an Inertia-Supplied Cross Attention (ISCA) block and a Projection-Guided Cross Attention (PGCA) block. ISCA block introduces multi-channel inertia forces and increases the memory effect by a cross attention mechanism between adjacent iterations. And, PGCA block achieves an enhanced information interaction, which introduces the inertia force into the gradient descent step through a cross attention block. Extensive CS experiments manifest that our OCTUF achieves superior performance compared to state-of-the-art methods while training lower complexity. Codes are available at https://github.com/songjiechong/OCTUF.
7.Vision Conformer: Incorporating Convolutions into Vision Transformer Layers
Authors:Brian Kenji Iwana, Akihiro Kusuda
Abstract: Transformers are popular neural network models that use layers of self-attention and fully-connected nodes with embedded tokens. Vision Transformers (ViT) adapt transformers for image recognition tasks. In order to do this, the images are split into patches and used as tokens. One issue with ViT is the lack of inductive bias toward image structures. Because ViT was adapted for image data from language modeling, the network does not explicitly handle issues such as local translations, pixel information, and information loss in the structures and features shared by multiple patches. Conversely, Convolutional Neural Networks (CNN) incorporate this information. Thus, in this paper, we propose the use of convolutional layers within ViT. Specifically, we propose a model called a Vision Conformer (ViC) which replaces the Multi-Layer Perceptron (MLP) in a ViT layer with a CNN. In addition, to use the CNN, we proposed to reconstruct the image data after the self-attention in a reverse embedding layer. Through the evaluation, we demonstrate that the proposed convolutions help improve the classification ability of ViT.
8.Rotation and Translation Invariant Representation Learning with Implicit Neural Representations
Authors:Sehyun Kwon, Joo Young Choi, Ernest K. Ryu
Abstract: In many computer vision applications, images are acquired with arbitrary or random rotations and translations, and in such setups, it is desirable to obtain semantic representations disentangled from the image orientation. Examples of such applications include semiconductor wafer defect inspection, plankton microscope images, and inference on single-particle cryo-electron microscopy (cryo-EM) micro-graphs. In this work, we propose Invariant Representation Learning with Implicit Neural Representation (IRL-INR), which uses an implicit neural representation (INR) with a hypernetwork to obtain semantic representations disentangled from the orientation of the image. We show that IRL-INR can effectively learn disentangled semantic representations on more complex images compared to those considered in prior works and show that these semantic representations synergize well with SCAN to produce state-of-the-art unsupervised clustering results.
9.ContraNeRF: 3D-Aware Generative Model via Contrastive Learning with Unsupervised Implicit Pose Embedding
Authors:Mijeoong Kim, Hyunjoon Lee, Bohyung Han
Abstract: Although 3D-aware GANs based on neural radiance fields have achieved competitive performance, their applicability is still limited to objects or scenes with the ground-truths or prediction models for clearly defined canonical camera poses. To extend the scope of applicable datasets, we propose a novel 3D-aware GAN optimization technique through contrastive learning with implicit pose embeddings. To this end, we first revise the discriminator design and remove dependency on ground-truth camera poses. Then, to capture complex and challenging 3D scene structures more effectively, we make the discriminator estimate a high-dimensional implicit pose embedding from a given image and perform contrastive learning on the pose embedding. The proposed approach can be employed for the dataset, where the canonical camera pose is ill-defined because it does not look up or estimate camera poses. Experimental results show that our algorithm outperforms existing methods by large margins on the datasets with multiple object categories and inconsistent canonical camera poses.
10.Edit Everything: A Text-Guided Generative System for Images Editing
Authors:Defeng Xie, Ruichen Wang, Jian Ma, Chen Chen, Haonan Lu, Dong Yang, Fobo Shi, Xiaodong Lin
Abstract: We introduce a new generative system called Edit Everything, which can take image and text inputs and produce image outputs. Edit Everything allows users to edit images using simple text instructions. Our system designs prompts to guide the visual module in generating requested images. Experiments demonstrate that Edit Everything facilitates the implementation of the visual aspects of Stable Diffusion with the use of Segment Anything model and CLIP. Our system is publicly available at https://github.com/DefengXie/Edit_Everything.
11.COSST: Multi-organ Segmentation with Partially Labeled Datasets Using Comprehensive Supervisions and Self-training
Authors:Han Liu, Zhoubing Xu, Riqiang Gao, Hao Li, Jianing Wang, Guillaume Chabin, Ipek Oguz, Sasa Grbic
Abstract: Deep learning models have demonstrated remarkable success in multi-organ segmentation but typically require large-scale datasets with all organs of interest annotated. However, medical image datasets are often low in sample size and only partially labeled, i.e., only a subset of organs are annotated. Therefore, it is crucial to investigate how to learn a unified model on the available partially labeled datasets to leverage their synergistic potential. In this paper, we empirically and systematically study the partial-label segmentation with in-depth analyses on the existing approaches and identify three distinct types of supervision signals, including two signals derived from ground truth and one from pseudo label. We propose a novel training framework termed COSST, which effectively and efficiently integrates comprehensive supervision signals with self-training. Concretely, we first train an initial unified model using two ground truth-based signals and then iteratively incorporate the pseudo label signal to the initial model using self-training. To mitigate performance degradation caused by unreliable pseudo labels, we assess the reliability of pseudo labels via outlier detection in latent space and exclude the most unreliable pseudo labels from each self-training iteration. Extensive experiments are conducted on six CT datasets for three partial-label segmentation tasks. Experimental results show that our proposed COSST achieves significant improvement over the baseline method, i.e., individual networks trained on each partially labeled dataset. Compared to the state-of-the-art partial-label segmentation methods, COSST demonstrates consistent superior performance on various segmentation tasks and with different training data size.
12.Large Scale Genealogical Information Extraction From Handwritten Quebec Parish Records
Authors:Solène Tarride, Martin Maarand, Mélodie Boillet, James McGrath, Eugénie Capel, Hélène Vézina, Christopher Kermorvant
Abstract: This paper presents a complete workflow designed for extracting information from Quebec handwritten parish registers. The acts in these documents contain individual and family information highly valuable for genetic, demographic and social studies of the Quebec population. From an image of parish records, our workflow is able to identify the acts and extract personal information. The workflow is divided into successive steps: page classification, text line detection, handwritten text recognition, named entity recognition and act detection and classification. For all these steps, different machine learning models are compared. Once the information is extracted, validation rules designed by experts are then applied to standardize the extracted information and ensure its consistency with the type of act (birth, marriage, and death). This validation step is able to reject records that are considered invalid or merged. The full workflow has been used to process over two million pages of Quebec parish registers from the 19-20th centuries. On a sample comprising 65% of registers, 3.2 million acts were recognized. Verification of the birth and death acts from this sample shows that 74% of them are considered complete and valid. These records will be integrated into the BALSAC database and linked together to recreate family and genealogical relations at large scale.
13.Interweaved Graph and Attention Network for 3D Human Pose Estimation
Authors:Ti Wang, Hong Liu, Runwei Ding, Wenhao Li, Yingxuan You, Xia Li
Abstract: Despite substantial progress in 3D human pose estimation from a single-view image, prior works rarely explore global and local correlations, leading to insufficient learning of human skeleton representations. To address this issue, we propose a novel Interweaved Graph and Attention Network (IGANet) that allows bidirectional communications between graph convolutional networks (GCNs) and attentions. Specifically, we introduce an IGA module, where attentions are provided with local information from GCNs and GCNs are injected with global information from attentions. Additionally, we design a simple yet effective U-shaped multi-layer perceptron (uMLP), which can capture multi-granularity information for body joints. Extensive experiments on two popular benchmark datasets (i.e. Human3.6M and MPI-INF-3DHP) are conducted to evaluate our proposed method.The results show that IGANet achieves state-of-the-art performance on both datasets. Code is available at https://github.com/xiu-cs/IGANet.
14.Lightweight, Pre-trained Transformers for Remote Sensing Timeseries
Authors:Gabriel Tseng, Ivan Zvonkov, Mirali Purohit, David Rolnick, Hannah Kerner
Abstract: Machine learning algorithms for parsing remote sensing data have a wide range of societally relevant applications, but labels used to train these algorithms can be difficult or impossible to acquire. This challenge has spurred research into self-supervised learning for remote sensing data aiming to unlock the use of machine learning in geographies or application domains where labelled datasets are small. Current self-supervised learning approaches for remote sensing data draw significant inspiration from techniques applied to natural images. However, remote sensing data has important differences from natural images -- for example, the temporal dimension is critical for many tasks and data is collected from many complementary sensors. We show that designing models and self-supervised training techniques specifically for remote sensing data results in both smaller and more performant models. We introduce the Pretrained Remote Sensing Transformer (Presto), a transformer-based model pre-trained on remote sensing pixel-timeseries data. Presto excels at a wide variety of globally distributed remote sensing tasks and outperforms much larger models. Presto can be used for transfer learning or as a feature extractor for simple models, enabling efficient deployment at scale.
15.Compositional 3D Human-Object Neural Animation
Authors:Zhi Hou, Baosheng Yu, Dacheng Tao
Abstract: Human-object interactions (HOIs) are crucial for human-centric scene understanding applications such as human-centric visual generation, AR/VR, and robotics. Since existing methods mainly explore capturing HOIs, rendering HOI remains less investigated. In this paper, we address this challenge in HOI animation from a compositional perspective, i.e., animating novel HOIs including novel interaction, novel human and/or novel object driven by a novel pose sequence. Specifically, we adopt neural human-object deformation to model and render HOI dynamics based on implicit neural representations. To enable the interaction pose transferring among different persons and objects, we then devise a new compositional conditional neural radiance field (or CC-NeRF), which decomposes the interdependence between human and object using latent codes to enable compositionally animation control of novel HOIs. Experiments show that the proposed method can generalize well to various novel HOI animation settings. Our project page is https://zhihou7.github.io/CHONA/
16.RegHEC: Hand-Eye Calibration via Simultaneous Multi-view Point Clouds Registration of Arbitrary Object
Authors:Shiyu Xing, Fengshui Jing, Min Tan
Abstract: RegHEC is a registration-based hand-eye calibration technique with no need for accurate calibration rig but arbitrary available objects, applicable for both eye-in-hand and eye-to-hand cases. It tries to find the hand-eye relation which brings multi-view point clouds of arbitrary scene into simultaneous registration under a common reference frame. RegHEC first achieves initial alignment of multi-view point clouds via Bayesian optimization, where registration problem is modeled as a Gaussian process over hand-eye relation and the covariance function is modified to be compatible with distance metric in 3-D motion space SE(3), then passes the initial guess of hand-eye relation to an Anderson Accelerated ICP variant for later fine registration and accurate calibration. RegHEC has little requirement on calibration object, it is applicable with sphere, cone, cylinder and even simple plane, which can be quite challenging for correct point cloud registration and sensor motion estimation using existing methods. While suitable for most 3-D vision guided tasks, RegHEC is especially favorable for robotic 3-D reconstruction, as calibration and multi-view point clouds registration of reconstruction target are unified into a single process. Our technique is verified with extensive experiments using varieties of arbitrary objects and real hand-eye system. We release an open-source C++ implementation of RegHEC.
17.Learning Human-Human Interactions in Images from Weak Textual Supervision
Authors:Morris Alper, Hadar Averbuch-Elor
Abstract: Interactions between humans are diverse and context-dependent, but previous works have treated them as categorical, disregarding the heavy tail of possible interactions. We propose a new paradigm of learning human-human interactions as free text from a single still image, allowing for flexibility in modeling the unlimited space of situations and relationships between people. To overcome the absence of data labelled specifically for this task, we use knowledge distillation applied to synthetic caption data produced by a large language model without explicit supervision. We show that the pseudo-labels produced by this procedure can be used to train a captioning model to effectively understand human-human interactions in images, as measured by a variety of metrics that measure textual and semantic faithfulness and factual groundedness of our predictions. We further show that our approach outperforms SOTA image captioning and situation recognition models on this task. We will release our code and pseudo-labels along with Waldo and Wenda, a manually-curated test set for still image human-human interaction understanding.
18.DataComp: In search of the next generation of multimodal datasets
Authors:Samir Yitzhak Gadre, Gabriel Ilharco, Alex Fang, Jonathan Hayase, Georgios Smyrnis, Thao Nguyen, Ryan Marten, Mitchell Wortsman, Dhruba Ghosh, Jieyu Zhang, Eyal Orgad, Rahim Entezari, Giannis Daras, Sarah Pratt, Vivek Ramanujan, Yonatan Bitton, Kalyani Marathe, Stephen Mussmann, Richard Vencu, Mehdi Cherti, Ranjay Krishna, Pang Wei Koh, Olga Saukh, Alexander Ratner, Shuran Song, Hannaneh Hajishirzi, Ali Farhadi, Romain Beaumont, Sewoong Oh, Alex Dimakis, Jenia Jitsev, Yair Carmon, Vaishaal Shankar, Ludwig Schmidt
Abstract: Large multimodal datasets have been instrumental in recent breakthroughs such as CLIP, Stable Diffusion, and GPT-4. At the same time, datasets rarely receive the same research attention as model architectures or training algorithms. To address this shortcoming in the machine learning ecosystem, we introduce DataComp, a benchmark where the training code is fixed and researchers innovate by proposing new training sets. We provide a testbed for dataset experiments centered around a new candidate pool of 12.8B image-text pairs from Common Crawl. Participants in our benchmark design new filtering techniques or curate new data sources and then evaluate their new dataset by running our standardized CLIP training code and testing on 38 downstream test sets. Our benchmark consists of multiple scales, with four candidate pool sizes and associated compute budgets ranging from 12.8M to 12.8B samples seen during training. This multi-scale design facilitates the study of scaling trends and makes the benchmark accessible to researchers with varying resources. Our baseline experiments show that the DataComp workflow is a promising way of improving multimodal datasets. We introduce DataComp-1B, a dataset created by applying a simple filtering algorithm to the 12.8B candidate pool. The resulting 1.4B subset enables training a CLIP ViT-L/14 from scratch to 79.2% zero-shot accuracy on ImageNet. Our new ViT-L/14 model outperforms a larger ViT-g/14 trained on LAION-2B by 0.7 percentage points while requiring 9x less training compute. We also outperform OpenAI's CLIP ViT-L/14 by 3.7 percentage points, which is trained with the same compute budget as our model. These gains highlight the potential for improving model performance by carefully curating training sets. We view DataComp-1B as only the first step and hope that DataComp paves the way toward the next generation of multimodal datasets.
19.Towards Precise Weakly Supervised Object Detection via Interactive Contrastive Learning of Context Information
Authors:Lai Qi
Abstract: Weakly supervised object detection (WSOD) aims at learning precise object detectors with only image-level tags. In spite of intensive research on deep learning (DL) approaches over the past few years, there is still a significant performance gap between WSOD and fully supervised object detection. In fact, most existing WSOD methods only consider the visual appearance of each region proposal but ignore employing the useful context information in the image. To this end, this paper proposes an interactive end-to-end WSDO framework called JLWSOD with two innovations: i) two types of WSOD-specific context information (i.e., instance-wise correlation andsemantic-wise correlation) are proposed and introduced into WSOD framework; ii) an interactive graph contrastive learning (iGCL) mechanism is designed to jointly optimize the visual appearance and context information for better WSOD performance. Specifically, the iGCL mechanism takes full advantage of the complementary interpretations of the WSOD, namely instance-wise detection and semantic-wise prediction tasks, forming a more comprehensive solution. Extensive experiments on the widely used PASCAL VOC and MS COCO benchmarks verify the superiority of JLWSOD over alternative state-of-the-art approaches and baseline models (improvement of 3.6%~23.3% on mAP and 3.4%~19.7% on CorLoc, respectively).
20.Deeply-Coupled Convolution-Transformer with Spatial-temporal Complementary Learning for Video-based Person Re-identification
Authors:Xuehu Liu, Chenyang Yu, Pingping Zhang, Huchuan Lu
Abstract: Advanced deep Convolutional Neural Networks (CNNs) have shown great success in video-based person Re-Identification (Re-ID). However, they usually focus on the most obvious regions of persons with a limited global representation ability. Recently, it witnesses that Transformers explore the inter-patch relations with global observations for performance improvements. In this work, we take both sides and propose a novel spatial-temporal complementary learning framework named Deeply-Coupled Convolution-Transformer (DCCT) for high-performance video-based person Re-ID. Firstly, we couple CNNs and Transformers to extract two kinds of visual features and experimentally verify their complementarity. Further, in spatial, we propose a Complementary Content Attention (CCA) to take advantages of the coupled structure and guide independent features for spatial complementary learning. In temporal, a Hierarchical Temporal Aggregation (HTA) is proposed to progressively capture the inter-frame dependencies and encode temporal information. Besides, a gated attention is utilized to deliver aggregated temporal information into the CNN and Transformer branches for temporal complementary learning. Finally, we introduce a self-distillation training strategy to transfer the superior spatial-temporal knowledge to backbone networks for higher accuracy and more efficiency. In this way, two kinds of typical features from same videos are integrated mechanically for more informative representations. Extensive experiments on four public Re-ID benchmarks demonstrate that our framework could attain better performances than most state-of-the-art methods.
21.MCLFIQ: Mobile Contactless Fingerprint Image Quality
Authors:Jannis Priesnitz, Axel Weißenfeld, Christian Rathgeb, Bernhard Strobl, Ralph Lessmann, Christoph Busch1
Abstract: We propose MCLFIQ: Mobile Contactless Fingerprint Image Quality, the first quality assessment algorithm for mobile contactless fingerprint samples. To this end, we retrained the NIST Fingerprint Image Quality (NFIQ) 2 method, which was originally designed for contact-based fingerprints, with a synthetic contactless fingerprint database. We evaluate the predictive performance of the resulting MCLFIQ model in terms of Error-vs.-Discard Characteristic (EDC) curves on three real-world contactless fingerprint databases using two recognition algorithms. In experiments, the MCLFIQ method is compared against the original NFIQ 2 method and a sharpness-based quality assessment algorithm developed for contactless fingerprint images. Obtained results show that the re-training of NFIQ 2 on synthetic data is a viable alternative to training on real databases. Moreover, the evaluation shows that our MCLFIQ method works more accurate and robust compared to NFIQ 2 and the sharpness-based quality assessment. We suggest considering the proposed MCLFIQ method as a candidate for a new standard algorithm for contactless fingerprint quality assessment.
22.Exploiting Inductive Bias in Transformer for Point Cloud Classification and Segmentation
Authors:Zihao Li, Pan Gao, Hui Yuan, Ran Wei, Manoranjan Paul
Abstract: Discovering inter-point connection for efficient high-dimensional feature extraction from point coordinate is a key challenge in processing point cloud. Most existing methods focus on designing efficient local feature extractors while ignoring global connection, or vice versa. In this paper, we design a new Inductive Bias-aided Transformer (IBT) method to learn 3D inter-point relations, which considers both local and global attentions. Specifically, considering local spatial coherence, local feature learning is performed through Relative Position Encoding and Attentive Feature Pooling. We incorporate the learned locality into the Transformer module. The local feature affects value component in Transformer to modulate the relationship between channels of each point, which can enhance self-attention mechanism with locality based channel interaction. We demonstrate its superiority experimentally on classification and segmentation tasks. The code is available at: https://github.com/jiamang/IBT
23.Density Invariant Contrast Maximization for Neuromorphic Earth Observations
Authors:Sami Arja, Alexandre Marcireau, Richard L. Balthazor, Matthew G. McHarg, Saeed Afshar, Gregory Cohen
Abstract: Contrast maximization (CMax) techniques are widely used in event-based vision systems to estimate the motion parameters of the camera and generate high-contrast images. However, these techniques are noise-intolerance and suffer from the multiple extrema problem which arises when the scene contains more noisy events than structure, causing the contrast to be higher at multiple locations. This makes the task of estimating the camera motion extremely challenging, which is a problem for neuromorphic earth observation, because, without a proper estimation of the motion parameters, it is not possible to generate a map with high contrast, causing important details to be lost. Similar methods that use CMax addressed this problem by changing or augmenting the objective function to enable it to converge to the correct motion parameters. Our proposed solution overcomes the multiple extrema and noise-intolerance problems by correcting the warped event before calculating the contrast and offers the following advantages: it does not depend on the event data, it does not require a prior about the camera motion, and keeps the rest of the CMax pipeline unchanged. This is to ensure that the contrast is only high around the correct motion parameters. Our approach enables the creation of better motion-compensated maps through an analytical compensation technique using a novel dataset from the International Space Station (ISS). Code is available at \url{https://github.com/neuromorphicsystems/event_warping}
24.Human Semantic Segmentation using Millimeter-Wave Radar Sparse Point Clouds
Authors:Pengfei Song, Luoyu MEI, Han Cheng
Abstract: This paper presents a framework for semantic segmentation on sparse sequential point clouds of millimeter-wave radar. Compared with cameras and lidars, millimeter-wave radars have the advantage of not revealing privacy, having a strong anti-interference ability, and having long detection distance. The sparsity and capturing temporal-topological features of mmWave data is still a problem. However, the issue of capturing the temporal-topological coupling features under the human semantic segmentation task prevents previous advanced segmentation methods (e.g PointNet, PointCNN, Point Transformer) from being well utilized in practical scenarios. To address the challenge caused by the sparsity and temporal-topological feature of the data, we (i) introduce graph structure and topological features to the point cloud, (ii) propose a semantic segmentation framework including a global feature-extracting module and a sequential feature-extracting module. In addition, we design an efficient and more fitting loss function for a better training process and segmentation results based on graph clustering. Experimentally, we deploy representative semantic segmentation algorithms (Transformer, GCNN, etc.) on a custom dataset. Experimental results indicate that our model achieves mean accuracy on the custom dataset by $\mathbf{82.31}\%$ and outperforms the state-of-the-art algorithms. Moreover, to validate the model's robustness, we deploy our model on the well-known S3DIS dataset. On the S3DIS dataset, our model achieves mean accuracy by $\mathbf{92.6}\%$, outperforming baseline algorithms.
25.Figments and Misalignments: A Framework for Fine-grained Crossmodal Misinformation Detection
Authors:Stefanos-Iordanis Papadopoulos, Christos Koutlis, Symeon Papadopoulos, Panagiotis C. Petrantonakis
Abstract: Multimedia content has become ubiquitous on social media platforms, leading to the rise of multimodal misinformation and the urgent need for effective strategies to detect and prevent its spread. This study focuses on CrossModal Misinformation (CMM) where image-caption pairs work together to spread falsehoods. We contrast CMM with Asymmetric Multimodal Misinformation (AMM), where one dominant modality propagates falsehoods while other modalities have little or no influence. We show that AMM adds noise to the training and evaluation process while exacerbating the unimodal bias, where text-only or image-only detectors can seemingly outperform their multimodal counterparts on an inherently multimodal task. To address this issue, we collect and curate FIGMENTS, a robust evaluation benchmark for CMM, which consists of real world cases of misinformation, excludes AMM and utilizes modality balancing to successfully alleviate unimodal bias. FIGMENTS also provides a first step towards fine-grained CMM detection by including three classes: truthful, out-of-context, and miscaptioned image-caption pairs. Furthermore, we introduce a method for generating realistic synthetic training data that maintains crossmodal relations between legitimate images and false human-written captions that we term Crossmodal HArd Synthetic MisAlignment (CHASMA). We conduct extensive comparative study using a Transformer-based architecture. Our results show that incorporating CHASMA in conjunction with other generated datasets consistently improved the overall performance on FIGMENTS in both binary (+6.26%) and multiclass settings (+15.8%).We release our code at: https://github.com/stevejpapad/figments-and-misalignments
26.Quadric Representations for LiDAR Odometry, Mapping and Localization
Authors:Chao Xia, Chenfeng Xu, Patrick Rim, Mingyu Ding, Nanning Zheng, Kurt Keutzer, Masayoshi Tomizuka, Wei Zhan
Abstract: Current LiDAR odometry, mapping and localization methods leverage point-wise representations of 3D scenes and achieve high accuracy in autonomous driving tasks. However, the space-inefficiency of methods that use point-wise representations limits their development and usage in practical applications. In particular, scan-submap matching and global map representation methods are restricted by the inefficiency of nearest neighbor searching (NNS) for large-volume point clouds. To improve space-time efficiency, we propose a novel method of describing scenes using quadric surfaces, which are far more compact representations of 3D objects than conventional point clouds. In contrast to point cloud-based methods, our quadric representation-based method decomposes a 3D scene into a collection of sparse quadric patches, which improves storage efficiency and avoids the slow point-wise NNS process. Our method first segments a given point cloud into patches and fits each of them to a quadric implicit function. Each function is then coupled with other geometric descriptors of the patch, such as its center position and covariance matrix. Collectively, these patch representations fully describe a 3D scene, which can be used in place of the original point cloud and employed in LiDAR odometry, mapping and localization algorithms. We further design a novel incremental growing method for quadric representations, which eliminates the need to repeatedly re-fit quadric surfaces from the original point cloud. Extensive odometry, mapping and localization experiments on large-volume point clouds in the KITTI and UrbanLoco datasets demonstrate that our method maintains low latency and memory utility while achieving competitive, and even superior, accuracy.
27.FLAC: Fairness-Aware Representation Learning by Suppressing Attribute-Class Associations
Authors:Ioannis Sarridis, Christos Koutlis, Symeon Papadopoulos, Christos Diou
Abstract: Bias in computer vision systems can perpetuate or even amplify discrimination against certain populations. Considering that bias is often introduced by biased visual datasets, many recent research efforts focus on training fair models using such data. However, most of them heavily rely on the availability of protected attribute labels in the dataset, which limits their applicability, while label-unaware approaches, i.e., approaches operating without such labels, exhibit considerably lower performance. To overcome these limitations, this work introduces FLAC, a methodology that minimizes mutual information between the features extracted by the model and a protected attribute, without the use of attribute labels. To do that, FLAC proposes a sampling strategy that highlights underrepresented samples in the dataset, and casts the problem of learning fair representations as a probability matching problem that leverages representations extracted by a bias-capturing classifier. It is theoretically shown that FLAC can indeed lead to fair representations, that are independent of the protected attributes. FLAC surpasses the current state-of-the-art on Biased MNIST, CelebA, and UTKFace, by 29.1%, 18.1%, and 21.9%, respectively. Additionally, FLAC exhibits 2.2% increased accuracy on ImageNet-A consisting of the most challenging samples of ImageNet. Finally, in most experiments, FLAC even outperforms the bias label-aware state-of-the-art methods.
28.Adaptive manifold for imbalanced transductive few-shot learning
Authors:Michalis Lazarou, Yannis Avrithis, Tania Stathaki
Abstract: Transductive few-shot learning algorithms have showed substantially superior performance over their inductive counterparts by leveraging the unlabeled queries. However, the vast majority of such methods are evaluated on perfectly class-balanced benchmarks. It has been shown that they undergo remarkable drop in performance under a more realistic, imbalanced setting. To this end, we propose a novel algorithm to address imbalanced transductive few-shot learning, named Adaptive Manifold. Our method exploits the underlying manifold of the labeled support examples and unlabeled queries by using manifold similarity to predict the class probability distribution per query. It is parameterized by one centroid per class as well as a set of graph-specific parameters that determine the manifold. All parameters are optimized through a loss function that can be tuned towards class-balanced or imbalanced distributions. The manifold similarity shows substantial improvement over Euclidean distance, especially in the 1-shot setting. Our algorithm outperforms or is on par with other state of the art methods in three benchmark datasets, namely miniImageNet, tieredImageNet and CUB, and three different backbones, namely ResNet-18, WideResNet-28-10 and DenseNet-121. In certain cases, our algorithm outperforms the previous state of the art by as much as 4.2%.
29.EDAPS: Enhanced Domain-Adaptive Panoptic Segmentation
Authors:Suman Saha, Lukas Hoyer, Anton Obukhov, Dengxin Dai, Luc Van Gool
Abstract: With autonomous industries on the rise, domain adaptation of the visual perception stack is an important research direction due to the cost savings promise. Much prior art was dedicated to domain-adaptive semantic segmentation in the synthetic-to-real context. Despite being a crucial output of the perception stack, panoptic segmentation has been largely overlooked by the domain adaptation community. Therefore, we revisit well-performing domain adaptation strategies from other fields, adapt them to panoptic segmentation, and show that they can effectively enhance panoptic domain adaptation. Further, we study the panoptic network design and propose a novel architecture (EDAPS) designed explicitly for domain-adaptive panoptic segmentation. It uses a shared, domain-robust transformer encoder to facilitate the joint adaptation of semantic and instance features, but task-specific decoders tailored for the specific requirements of both domain-adaptive semantic and instance segmentation. As a result, the performance gap seen in challenging panoptic benchmarks is substantially narrowed. EDAPS significantly improves the state-of-the-art performance for panoptic segmentation UDA by a large margin of 25% on SYNTHIA-to-Cityscapes and even 72% on the more challenging SYNTHIA-to-Mapillary Vistas. The implementation is available at https://github.com/susaha/edaps.
30.Instance Segmentation in the Dark
Authors:Linwei Chen, Ying Fu, Kaixuan Wei, Dezhi Zheng, Felix Heide
Abstract: Existing instance segmentation techniques are primarily tailored for high-visibility inputs, but their performance significantly deteriorates in extremely low-light environments. In this work, we take a deep look at instance segmentation in the dark and introduce several techniques that substantially boost the low-light inference accuracy. The proposed method is motivated by the observation that noise in low-light images introduces high-frequency disturbances to the feature maps of neural networks, thereby significantly degrading performance. To suppress this ``feature noise", we propose a novel learning method that relies on an adaptive weighted downsampling layer, a smooth-oriented convolutional block, and disturbance suppression learning. These components effectively reduce feature noise during downsampling and convolution operations, enabling the model to learn disturbance-invariant features. Furthermore, we discover that high-bit-depth RAW images can better preserve richer scene information in low-light conditions compared to typical camera sRGB outputs, thus supporting the use of RAW-input algorithms. Our analysis indicates that high bit-depth can be critical for low-light instance segmentation. To mitigate the scarcity of annotated RAW datasets, we leverage a low-light RAW synthetic pipeline to generate realistic low-light data. In addition, to facilitate further research in this direction, we capture a real-world low-light instance segmentation dataset comprising over two thousand paired low/normal-light images with instance-level pixel-wise annotations. Remarkably, without any image preprocessing, we achieve satisfactory performance on instance segmentation in very low light (4~\% AP higher than state-of-the-art competitors), meanwhile opening new opportunities for future research.
31.A Probabilistic Attention Model with Occlusion-aware Texture Regression for 3D Hand Reconstruction from a Single RGB Image
Authors:Zheheng Jiang, Hossein Rahmani, Sue Black, Bryan M. Williams
Abstract: Recently, deep learning based approaches have shown promising results in 3D hand reconstruction from a single RGB image. These approaches can be roughly divided into model-based approaches, which are heavily dependent on the model's parameter space, and model-free approaches, which require large numbers of 3D ground truths to reduce depth ambiguity and struggle in weakly-supervised scenarios. To overcome these issues, we propose a novel probabilistic model to achieve the robustness of model-based approaches and reduced dependence on the model's parameter space of model-free approaches. The proposed probabilistic model incorporates a model-based network as a prior-net to estimate the prior probability distribution of joints and vertices. An Attention-based Mesh Vertices Uncertainty Regression (AMVUR) model is proposed to capture dependencies among vertices and the correlation between joints and mesh vertices to improve their feature representation. We further propose a learning based occlusion-aware Hand Texture Regression model to achieve high-fidelity texture reconstruction. We demonstrate the flexibility of the proposed probabilistic model to be trained in both supervised and weakly-supervised scenarios. The experimental results demonstrate our probabilistic model's state-of-the-art accuracy in 3D hand and texture reconstruction from a single image in both training schemes, including in the presence of severe occlusions.
32.Combining HoloLens with Instant-NeRFs: Advanced Real-Time 3D Mobile Mapping
Authors:Dennis Haitz, Boris Jutzi, Markus Ulrich, Miriam Jaeger, Patrick Huebner
Abstract: This work represents a large step into modern ways of fast 3D reconstruction based on RGB camera images. Utilizing a Microsoft HoloLens 2 as a multisensor platform that includes an RGB camera and an inertial measurement unit for SLAM-based camera-pose determination, we train a Neural Radiance Field (NeRF) as a neural scene representation in real-time with the acquired data from the HoloLens. The HoloLens is connected via Wifi to a high-performance PC that is responsible for the training and 3D reconstruction. After the data stream ends, the training is stopped and the 3D reconstruction is initiated, which extracts a point cloud of the scene. With our specialized inference algorithm, five million scene points can be extracted within 1 second. In addition, the point cloud also includes radiometry per point. Our method of 3D reconstruction outperforms grid point sampling with NeRFs by multiple orders of magnitude and can be regarded as a complete real-time 3D reconstruction method in a mobile mapping setup.
33.Incremental Generalized Category Discovery
Authors:Bingchen Zhao, Oisin Mac Aodha
Abstract: We explore the problem of Incremental Generalized Category Discovery (IGCD). This is a challenging category incremental learning setting where the goal is to develop models that can correctly categorize images from previously seen categories, in addition to discovering novel ones. Learning is performed over a series of time steps where the model obtains new labeled and unlabeled data, and discards old data, at each iteration. The difficulty of the problem is compounded in our generalized setting as the unlabeled data can contain images from categories that may or may not have been observed before. We present a new method for IGCD which combines non-parametric categorization with efficient image sampling to mitigate catastrophic forgetting. To quantify performance, we propose a new benchmark dataset named iNatIGCD that is motivated by a real-world fine-grained visual categorization task. In our experiments we outperform existing related methods
34.Person Re-ID through Unsupervised Hypergraph Rank Selection and Fusion
Authors:Lucas Pascotti Valem, Daniel Carlos Guimarães Pedronette
Abstract: Person Re-ID has been gaining a lot of attention and nowadays is of fundamental importance in many camera surveillance applications. The task consists of identifying individuals across multiple cameras that have no overlapping views. Most of the approaches require labeled data, which is not always available, given the huge amount of demanded data and the difficulty of manually assigning a class for each individual. Recently, studies have shown that re-ranking methods are capable of achieving significant gains, especially in the absence of labeled data. Besides that, the fusion of feature extractors and multiple-source training is another promising research direction not extensively exploited. We aim to fill this gap through a manifold rank aggregation approach capable of exploiting the complementarity of different person Re-ID rankers. In this work, we perform a completely unsupervised selection and fusion of diverse ranked lists obtained from multiple and diverse feature extractors. Among the contributions, this work proposes a query performance prediction measure that models the relationship among images considering a hypergraph structure and does not require the use of any labeled data. Expressive gains were obtained in four datasets commonly used for person Re-ID. We achieved results competitive to the state-of-the-art in most of the scenarios.
35.SparseFusion: Fusing Multi-Modal Sparse Representations for Multi-Sensor 3D Object Detection
Authors:Yichen Xie, Chenfeng Xu, Marie-Julie Rakotosaona, Patrick Rim, Federico Tombari, Kurt Keutzer, Masayoshi Tomizuka, Wei Zhan
Abstract: By identifying four important components of existing LiDAR-camera 3D object detection methods (LiDAR and camera candidates, transformation, and fusion outputs), we observe that all existing methods either find dense candidates or yield dense representations of scenes. However, given that objects occupy only a small part of a scene, finding dense candidates and generating dense representations is noisy and inefficient. We propose SparseFusion, a novel multi-sensor 3D detection method that exclusively uses sparse candidates and sparse representations. Specifically, SparseFusion utilizes the outputs of parallel detectors in the LiDAR and camera modalities as sparse candidates for fusion. We transform the camera candidates into the LiDAR coordinate space by disentangling the object representations. Then, we can fuse the multi-modality candidates in a unified 3D space by a lightweight self-attention module. To mitigate negative transfer between modalities, we propose novel semantic and geometric cross-modality transfer modules that are applied prior to the modality-specific detectors. SparseFusion achieves state-of-the-art performance on the nuScenes benchmark while also running at the fastest speed, even outperforming methods with stronger backbones. We perform extensive experiments to demonstrate the effectiveness and efficiency of our modules and overall method pipeline. Our code will be made publicly available at https://github.com/yichen928/SparseFusion.
36.Structure Analysis of the FRP Rebar Using Computer Vision Techniques
Authors:Juraj Lagin, Simon Bilik
Abstract: In this paper we present a method to analyze the inner structure of the composite FRP rebar, namely the shift of the real center of gravity with a respect to the geometrical center of rebar and changes of cross-sectional characteristics. We propose an automated pipeline based on classical computer vision techniques and on the ratio between the glass fibers and epoxy filament in the analyzed cross-section to compute the shift vector of the real center of gravity in respect to the geometrical center together with the cross-section area and its principal moments. We discuss the achieved results over two cross sections in a different portion of the rebar and in the end, we suggest possible direction and improvements for our future work. We also made our code publicly available.
37.Occ3D: A Large-Scale 3D Occupancy Prediction Benchmark for Autonomous Driving
Authors:Xiaoyu Tian, Tao Jiang, Longfei Yun, Yue Wang, Yilun Wang, Hang Zhao
Abstract: Robotic perception requires the modeling of both 3D geometry and semantics. Existing methods typically focus on estimating 3D bounding boxes, neglecting finer geometric details and struggling to handle general, out-of-vocabulary objects. To overcome these limitations, we introduce a novel task for 3D occupancy prediction, which aims to estimate the detailed occupancy and semantics of objects from multi-view images. To facilitate this task, we develop a label generation pipeline that produces dense, visibility-aware labels for a given scene. This pipeline includes point cloud aggregation, point labeling, and occlusion handling. We construct two benchmarks based on the Waymo Open Dataset and the nuScenes Dataset, resulting in the Occ3D-Waymo and Occ3D-nuScenes benchmarks. Lastly, we propose a model, dubbed Coarse-to-Fine Occupancy (CTF-Occ) network, which demonstrates superior performance in the 3D occupancy prediction task. This approach addresses the need for finer geometric understanding in a coarse-to-fine fashion. The code, data, and benchmarks are released at https://tsinghua-mars-lab.github.io/Occ3D/.
38.Zero-shot Unsupervised Transfer Instance Segmentation
Authors:Gyungin Shin, Samuel Albanie, Weidi Xie
Abstract: Segmentation is a core computer vision competency, with applications spanning a broad range of scientifically and economically valuable domains. To date, however, the prohibitive cost of annotation has limited the deployment of flexible segmentation models. In this work, we propose Zero-shot Unsupervised Transfer Instance Segmentation (ZUTIS), a framework that aims to meet this challenge. The key strengths of ZUTIS are: (i) no requirement for instance-level or pixel-level annotations; (ii) an ability of zero-shot transfer, i.e., no assumption on access to a target data distribution; (iii) a unified framework for semantic and instance segmentations with solid performance on both tasks compared to state-of-the-art unsupervised methods. While comparing to previous work, we show ZUTIS achieves a gain of 2.2 mask AP on COCO-20K and 14.5 mIoU on ImageNet-S with 919 categories for instance and semantic segmentations, respectively. The code is made publicly available.
39.Co-SLAM: Joint Coordinate and Sparse Parametric Encodings for Neural Real-Time SLAM
Authors:Hengyi Wang, Jingwen Wang, Lourdes Agapito
Abstract: We present Co-SLAM, a neural RGB-D SLAM system based on a hybrid representation, that performs robust camera tracking and high-fidelity surface reconstruction in real time. Co-SLAM represents the scene as a multi-resolution hash-grid to exploit its high convergence speed and ability to represent high-frequency local features. In addition, Co-SLAM incorporates one-blob encoding, to encourage surface coherence and completion in unobserved areas. This joint parametric-coordinate encoding enables real-time and robust performance by bringing the best of both worlds: fast convergence and surface hole filling. Moreover, our ray sampling strategy allows Co-SLAM to perform global bundle adjustment over all keyframes instead of requiring keyframe selection to maintain a small number of active keyframes as competing neural SLAM approaches do. Experimental results show that Co-SLAM runs at 10-17Hz and achieves state-of-the-art scene reconstruction results, and competitive tracking performance in various datasets and benchmarks (ScanNet, TUM, Replica, Synthetic RGBD). Project page: https://hengyiwang.github.io/projects/CoSLAM
40.$π$-Tuning: Transferring Multimodal Foundation Models with Optimal Multi-task Interpolation
Authors:Chengyue Wu, Teng Wang, Yixiao Ge, Zeyu Lu, Ruisong Zhou, Ping Luo, Ying Shan
Abstract: Foundation models have achieved great advances in multi-task learning with a unified interface of unimodal and multimodal tasks. However, the potential of such multi-task learners has not been exploited during transfer learning. In this work, we present a universal parameter-efficient transfer learning method, termed Predict-Interpolate Tuning ($\pi$-Tuning), for vision, language, and vision-language tasks. It aggregates the parameters of lightweight task-specific experts learned from similar tasks to aid the target downstream task. The task similarities are predicted in a unified modality-independent space, yielding a scalable graph to demonstrate task relationships. $\pi$-Tuning has several appealing benefits. First, it flexibly explores both intra- and inter-modal transferability between similar tasks to improve the accuracy and robustness of transfer learning, especially in data-scarce scenarios. Second, it offers a systematical solution for transfer learning with multi-task prediction-and-then-interpolation, compatible with diverse types of parameter-efficient experts, such as prompt and adapter. Third, an extensive study of task-level mutual benefits on 14 unimodal and 6 multimodal datasets shows that $\pi$-Tuning surpasses fine-tuning and other parameter-efficient transfer learning methods both in full-shot and low-shot regimes. The task graph also enables an in-depth interpretable analysis of task transferability across modalities.
41.Analogy-Forming Transformers for Few-Shot 3D Parsing
Authors:Nikolaos Gkanatsios, Mayank Singh, Zhaoyuan Fang, Shubham Tulsiani, Katerina Fragkiadaki
Abstract: We present Analogical Networks, a model that encodes domain knowledge explicitly, in a collection of structured labelled 3D scenes, in addition to implicitly, as model parameters, and segments 3D object scenes with analogical reasoning: instead of mapping a scene to part segments directly, our model first retrieves related scenes from memory and their corresponding part structures, and then predicts analogous part structures for the input scene, via an end-to-end learnable modulation mechanism. By conditioning on more than one retrieved memories, compositions of structures are predicted, that mix and match parts across the retrieved memories. One-shot, few-shot or many-shot learning are treated uniformly in Analogical Networks, by conditioning on the appropriate set of memories, whether taken from a single, few or many memory exemplars, and inferring analogous parses. We show Analogical Networks are competitive with state-of-the-art 3D segmentation transformers in many-shot settings, and outperform them, as well as existing paradigms of meta-learning and few-shot learning, in few-shot settings. Analogical Networks successfully segment instances of novel object categories simply by expanding their memory, without any weight updates. Our code and models are publicly available in the project webpage: http://analogicalnets.github.io/.
42.SeqTrack: Sequence to Sequence Learning for Visual Object Tracking
Authors:Xin Chen, Houwen Peng, Dong Wang, Huchuan Lu, Han Hu
Abstract: In this paper, we present a new sequence-to-sequence learning framework for visual tracking, dubbed SeqTrack. It casts visual tracking as a sequence generation problem, which predicts object bounding boxes in an autoregressive fashion. This is different from prior Siamese trackers and transformer trackers, which rely on designing complicated head networks, such as classification and regression heads. SeqTrack only adopts a simple encoder-decoder transformer architecture. The encoder extracts visual features with a bidirectional transformer, while the decoder generates a sequence of bounding box values autoregressively with a causal transformer. The loss function is a plain cross-entropy. Such a sequence learning paradigm not only simplifies tracking framework, but also achieves competitive performance on benchmarks. For instance, SeqTrack gets 72.5% AUC on LaSOT, establishing a new state-of-the-art performance. Code and models are available at here.
43.Learning Articulated Shape with Keypoint Pseudo-labels from Web Images
Authors:Anastasis Stathopoulos, Georgios Pavlakos, Ligong Han, Dimitris Metaxas
Abstract: This paper shows that it is possible to learn models for monocular 3D reconstruction of articulated objects (e.g., horses, cows, sheep), using as few as 50-150 images labeled with 2D keypoints. Our proposed approach involves training category-specific keypoint estimators, generating 2D keypoint pseudo-labels on unlabeled web images, and using both the labeled and self-labeled sets to train 3D reconstruction models. It is based on two key insights: (1) 2D keypoint estimation networks trained on as few as 50-150 images of a given object category generalize well and generate reliable pseudo-labels; (2) a data selection mechanism can automatically create a "curated" subset of the unlabeled web images that can be used for training -- we evaluate four data selection methods. Coupling these two insights enables us to train models that effectively utilize web images, resulting in improved 3D reconstruction performance for several articulated object categories beyond the fully-supervised baseline. Our approach can quickly bootstrap a model and requires only a few images labeled with 2D keypoints. This requirement can be easily satisfied for any new object category. To showcase the practicality of our approach for predicting the 3D shape of arbitrary object categories, we annotate 2D keypoints on giraffe and bear images from COCO -- the annotation process takes less than 1 minute per image.
44.IconShop: Text-Based Vector Icon Synthesis with Autoregressive Transformers
Authors:Ronghuan Wu, Wanchao Su, Kede Ma, Jing Liao
Abstract: Scalable Vector Graphics (SVG) is a prevalent vector image format with good support for interactivity and animation. Despite such appealing characteristics, it is generally challenging for users to create their own SVG content because of the long learning curve to comprehend SVG grammars or acquaint themselves with professional editing software. Recent progress in text-to-image generation has inspired researchers to explore image-based icon synthesis (i.e., text -> raster image -> vector image) via differential rendering and language-based icon synthesis (i.e., text -> vector image script) via the "zero-shot" capabilities of large language models. However, these methods may suffer from several limitations regarding generation quality, diversity, flexibility, and speed. In this paper, we introduce IconShop, a text-guided vector icon synthesis method using an autoregressive transformer. The key to success of our approach is to sequentialize and tokenize the SVG paths (and textual descriptions) into a uniquely decodable command sequence. With such a single sequence as input, we are able to fully exploit the sequence learning power of autoregressive transformers, while enabling various icon synthesis and manipulation tasks. Through standard training to predict the next token on a large-scale icon dataset accompanied by textural descriptions, the proposed IconShop consistently exhibits better icon synthesis performance than existing image-based and language-based methods both quantitatively (using the FID and CLIP score) and qualitatively (through visual inspection). Meanwhile, we observe a dramatic improvement in generation diversity, which is supported by objective measures (Uniqueness and Novelty). More importantly, we demonstrate the flexibility of IconShop with two novel icon manipulation tasks - text-guided icon infilling, and text-combined icon synthesis.
45.ActorsNeRF: Animatable Few-shot Human Rendering with Generalizable NeRFs
Authors:Jiteng Mu, Shen Sang, Nuno Vasconcelos, Xiaolong Wang
Abstract: While NeRF-based human representations have shown impressive novel view synthesis results, most methods still rely on a large number of images / views for training. In this work, we propose a novel animatable NeRF called ActorsNeRF. It is first pre-trained on diverse human subjects, and then adapted with few-shot monocular video frames for a new actor with unseen poses. Building on previous generalizable NeRFs with parameter sharing using a ConvNet encoder, ActorsNeRF further adopts two human priors to capture the large human appearance, shape, and pose variations. Specifically, in the encoded feature space, we will first align different human subjects in a category-level canonical space, and then align the same human from different frames in an instance-level canonical space for rendering. We quantitatively and qualitatively demonstrate that ActorsNeRF significantly outperforms the existing state-of-the-art on few-shot generalization to new people and poses on multiple datasets. Project Page: https://jitengmu.github.io/ActorsNeRF/
46.Make It So: Steering StyleGAN for Any Image Inversion and Editing
Authors:Anand Bhattad, Viraj Shah, Derek Hoiem, D. A. Forsyth
Abstract: StyleGAN's disentangled style representation enables powerful image editing by manipulating the latent variables, but accurately mapping real-world images to their latent variables (GAN inversion) remains a challenge. Existing GAN inversion methods struggle to maintain editing directions and produce realistic results. To address these limitations, we propose Make It So, a novel GAN inversion method that operates in the $\mathcal{Z}$ (noise) space rather than the typical $\mathcal{W}$ (latent style) space. Make It So preserves editing capabilities, even for out-of-domain images. This is a crucial property that was overlooked in prior methods. Our quantitative evaluations demonstrate that Make It So outperforms the state-of-the-art method PTI~\cite{roich2021pivotal} by a factor of five in inversion accuracy and achieves ten times better edit quality for complex indoor scenes.
47.Motion-Conditioned Diffusion Model for Controllable Video Synthesis
Authors:Tsai-Shien Chen, Chieh Hubert Lin, Hung-Yu Tseng, Tsung-Yi Lin, Ming-Hsuan Yang
Abstract: Recent advancements in diffusion models have greatly improved the quality and diversity of synthesized content. To harness the expressive power of diffusion models, researchers have explored various controllable mechanisms that allow users to intuitively guide the content synthesis process. Although the latest efforts have primarily focused on video synthesis, there has been a lack of effective methods for controlling and describing desired content and motion. In response to this gap, we introduce MCDiff, a conditional diffusion model that generates a video from a starting image frame and a set of strokes, which allow users to specify the intended content and dynamics for synthesis. To tackle the ambiguity of sparse motion inputs and achieve better synthesis quality, MCDiff first utilizes a flow completion model to predict the dense video motion based on the semantic understanding of the video frame and the sparse motion control. Then, the diffusion model synthesizes high-quality future frames to form the output video. We qualitatively and quantitatively show that MCDiff achieves the state-the-of-art visual quality in stroke-guided controllable video synthesis. Additional experiments on MPII Human Pose further exhibit the capability of our model on diverse content and motion synthesis.
48.ChatVideo: A Tracklet-centric Multimodal and Versatile Video Understanding System
Authors:Junke Wang, Dongdong Chen, Chong Luo, Xiyang Dai, Lu Yuan, Zuxuan Wu, Yu-Gang Jiang
Abstract: Existing deep video models are limited by specific tasks, fixed input-output spaces, and poor generalization capabilities, making it difficult to deploy them in real-world scenarios. In this paper, we present our vision for multimodal and versatile video understanding and propose a prototype system, \system. Our system is built upon a tracklet-centric paradigm, which treats tracklets as the basic video unit and employs various Video Foundation Models (ViFMs) to annotate their properties e.g., appearance, motion, \etc. All the detected tracklets are stored in a database and interact with the user through a database manager. We have conducted extensive case studies on different types of in-the-wild videos, which demonstrates the effectiveness of our method in answering various video-related problems. Our project is available at https://www.wangjunke.info/ChatVideo/
49.Putting People in Their Place: Affordance-Aware Human Insertion into Scenes
Authors:Sumith Kulal, Tim Brooks, Alex Aiken, Jiajun Wu, Jimei Yang, Jingwan Lu, Alexei A. Efros, Krishna Kumar Singh
Abstract: We study the problem of inferring scene affordances by presenting a method for realistically inserting people into scenes. Given a scene image with a marked region and an image of a person, we insert the person into the scene while respecting the scene affordances. Our model can infer the set of realistic poses given the scene context, re-pose the reference person, and harmonize the composition. We set up the task in a self-supervised fashion by learning to re-pose humans in video clips. We train a large-scale diffusion model on a dataset of 2.4M video clips that produces diverse plausible poses while respecting the scene context. Given the learned human-scene composition, our model can also hallucinate realistic people and scenes when prompted without conditioning and also enables interactive editing. A quantitative evaluation shows that our method synthesizes more realistic human appearance and more natural human-scene interactions than prior work.