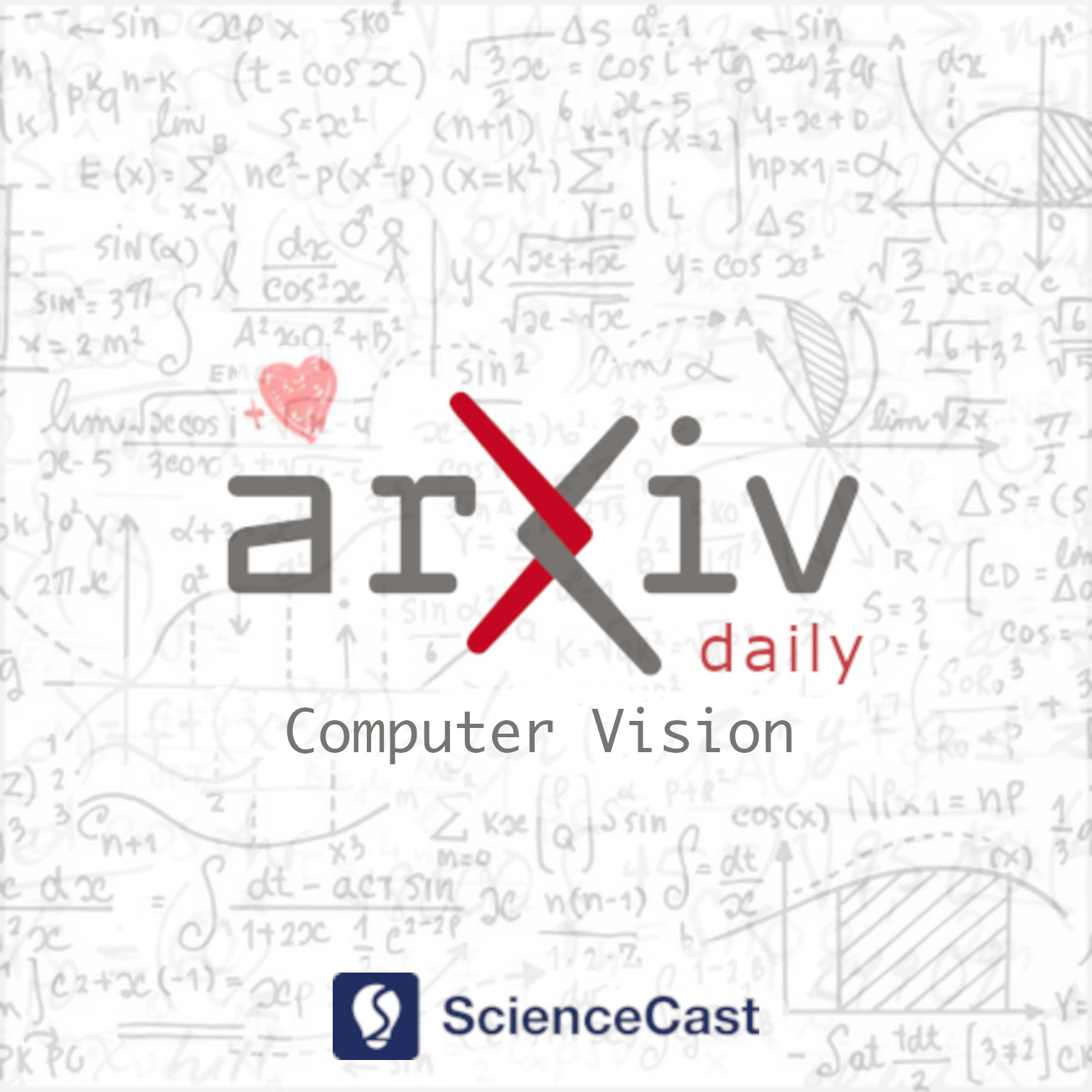
Computer Vision and Pattern Recognition (cs.CV)
Mon, 11 Sep 2023
1.HAT: Hybrid Attention Transformer for Image Restoration
Authors:Xiangyu Chen, Xintao Wang, Wenlong Zhang, Xiangtao Kong, Yu Qiao, Jiantao Zhou, Chao Dong
Abstract: Transformer-based methods have shown impressive performance in image restoration tasks, such as image super-resolution and denoising. However, we find that these networks can only utilize a limited spatial range of input information through attribution analysis. This implies that the potential of Transformer is still not fully exploited in existing networks. In order to activate more input pixels for better restoration, we propose a new Hybrid Attention Transformer (HAT). It combines both channel attention and window-based self-attention schemes, thus making use of their complementary advantages. Moreover, to better aggregate the cross-window information, we introduce an overlapping cross-attention module to enhance the interaction between neighboring window features. In the training stage, we additionally adopt a same-task pre-training strategy to further exploit the potential of the model for further improvement. Extensive experiments have demonstrated the effectiveness of the proposed modules. We further scale up the model to show that the performance of the SR task can be greatly improved. Besides, we extend HAT to more image restoration applications, including real-world image super-resolution, Gaussian image denoising and image compression artifacts reduction. Experiments on benchmark and real-world datasets demonstrate that our HAT achieves state-of-the-art performance both quantitatively and qualitatively. Codes and models are publicly available at https://github.com/XPixelGroup/HAT.
2.Multi3DRefer: Grounding Text Description to Multiple 3D Objects
Authors:Yiming Zhang, ZeMing Gong, Angel X. Chang
Abstract: We introduce the task of localizing a flexible number of objects in real-world 3D scenes using natural language descriptions. Existing 3D visual grounding tasks focus on localizing a unique object given a text description. However, such a strict setting is unnatural as localizing potentially multiple objects is a common need in real-world scenarios and robotic tasks (e.g., visual navigation and object rearrangement). To address this setting we propose Multi3DRefer, generalizing the ScanRefer dataset and task. Our dataset contains 61926 descriptions of 11609 objects, where zero, single or multiple target objects are referenced by each description. We also introduce a new evaluation metric and benchmark methods from prior work to enable further investigation of multi-modal 3D scene understanding. Furthermore, we develop a better baseline leveraging 2D features from CLIP by rendering object proposals online with contrastive learning, which outperforms the state of the art on the ScanRefer benchmark.
3.Towards Better Data Exploitation In Self-Supervised Monocular Depth Estimation
Authors:Jinfeng Liu, Lingtong Kong, Jie Yang, Wei Liu
Abstract: Depth estimation plays an important role in the robotic perception system. Self-supervised monocular paradigm has gained significant attention since it can free training from the reliance on depth annotations. Despite recent advancements, existing self-supervised methods still underutilize the available training data, limiting their generalization ability. In this paper, we take two data augmentation techniques, namely Resizing-Cropping and Splitting-Permuting, to fully exploit the potential of training datasets. Specifically, the original image and the generated two augmented images are fed into the training pipeline simultaneously and we leverage them to conduct self-distillation. Additionally, we introduce the detail-enhanced DepthNet with an extra full-scale branch in the encoder and a grid decoder to enhance the restoration of fine details in depth maps. Experimental results demonstrate our method can achieve state-of-the-art performance on the KITTI benchmark, with both raw ground truth and improved ground truth. Moreover, our models also show superior generalization performance when transferring to Make3D and NYUv2 datasets. Our codes are available at https://github.com/Sauf4896/BDEdepth.
4.FusionFormer: A Multi-sensory Fusion in Bird's-Eye-View and Temporal Consistent Transformer for 3D Objection
Authors:Chunyong Hu, Hang Zheng, Kun Li, Jianyun Xu, Weibo Mao, Maochun Luo, Lingxuan Wang, Mingxia Chen, Kaixuan Liu, Yiru Zhao, Peihan Hao, Minzhe Liu, Kaicheng Yu
Abstract: Multi-sensor modal fusion has demonstrated strong advantages in 3D object detection tasks. However, existing methods that fuse multi-modal features through a simple channel concatenation require transformation features into bird's eye view space and may lose the information on Z-axis thus leads to inferior performance. To this end, we propose FusionFormer, an end-to-end multi-modal fusion framework that leverages transformers to fuse multi-modal features and obtain fused BEV features. And based on the flexible adaptability of FusionFormer to the input modality representation, we propose a depth prediction branch that can be added to the framework to improve detection performance in camera-based detection tasks. In addition, we propose a plug-and-play temporal fusion module based on transformers that can fuse historical frame BEV features for more stable and reliable detection results. We evaluate our method on the nuScenes dataset and achieve 72.6% mAP and 75.1% NDS for 3D object detection tasks, outperforming state-of-the-art methods.
5.Gall Bladder Cancer Detection from US Images with Only Image Level Labels
Authors:Soumen Basu, Ashish Papanai, Mayank Gupta, Pankaj Gupta, Chetan Arora
Abstract: Automated detection of Gallbladder Cancer (GBC) from Ultrasound (US) images is an important problem, which has drawn increased interest from researchers. However, most of these works use difficult-to-acquire information such as bounding box annotations or additional US videos. In this paper, we focus on GBC detection using only image-level labels. Such annotation is usually available based on the diagnostic report of a patient, and do not require additional annotation effort from the physicians. However, our analysis reveals that it is difficult to train a standard image classification model for GBC detection. This is due to the low inter-class variance (a malignant region usually occupies only a small portion of a US image), high intra-class variance (due to the US sensor capturing a 2D slice of a 3D object leading to large viewpoint variations), and low training data availability. We posit that even when we have only the image level label, still formulating the problem as object detection (with bounding box output) helps a deep neural network (DNN) model focus on the relevant region of interest. Since no bounding box annotations is available for training, we pose the problem as weakly supervised object detection (WSOD). Motivated by the recent success of transformer models in object detection, we train one such model, DETR, using multi-instance-learning (MIL) with self-supervised instance selection to suit the WSOD task. Our proposed method demonstrates an improvement of AP and detection sensitivity over the SOTA transformer-based and CNN-based WSOD methods. Project page is at https://gbc-iitd.github.io/wsod-gbc
6.A horizon line annotation tool for streamlining autonomous sea navigation experiments
Authors:Yassir Zardoua, Abdelhamid El Wahabi, Mohammed Boulaala, Abdelali Astito
Abstract: Horizon line (or sea line) detection (HLD) is a critical component in multiple marine autonomous navigation tasks, such as identifying the navigation area (i.e., the sea), obstacle detection and geo-localization, and digital video stabilization. A recent survey highlighted several weaknesses of such detectors, particularly on sea conditions lacking from the most extensive dataset currently used by HLD researchers. Experimental validation of more robust HLDs involves collecting an extensive set of these lacking sea conditions and annotating each collected image with the correct position and orientation of the horizon line. The annotation task is daunting without a proper tool. Therefore, we present the first public annotation software with tailored features to make the sea line annotation process fast and easy. The software is available at: https://drive.google.com/drive/folders/1c0ZmvYDckuQCPIWfh_70P7E1A_DWlIvF?usp=sharing
7.Diving into Darkness: A Dual-Modulated Framework for High-Fidelity Super-Resolution in Ultra-Dark Environments
Authors:Jiaxin Gao, Ziyu Yue, Yaohua Liu, Sihan Xie, Xin Fan, Risheng Liu
Abstract: Super-resolution tasks oriented to images captured in ultra-dark environments is a practical yet challenging problem that has received little attention. Due to uneven illumination and low signal-to-noise ratio in dark environments, a multitude of problems such as lack of detail and color distortion may be magnified in the super-resolution process compared to normal-lighting environments. Consequently, conventional low-light enhancement or super-resolution methods, whether applied individually or in a cascaded manner for such problem, often encounter limitations in recovering luminance, color fidelity, and intricate details. To conquer these issues, this paper proposes a specialized dual-modulated learning framework that, for the first time, attempts to deeply dissect the nature of the low-light super-resolution task. Leveraging natural image color characteristics, we introduce a self-regularized luminance constraint as a prior for addressing uneven lighting. Expanding on this, we develop Illuminance-Semantic Dual Modulation (ISDM) components to enhance feature-level preservation of illumination and color details. Besides, instead of deploying naive up-sampling strategies, we design the Resolution-Sensitive Merging Up-sampler (RSMU) module that brings together different sampling modalities as substrates, effectively mitigating the presence of artifacts and halos. Comprehensive experiments showcases the applicability and generalizability of our approach to diverse and challenging ultra-low-light conditions, outperforming state-of-the-art methods with a notable improvement (i.e., $\uparrow$5\% in PSNR, and $\uparrow$43\% in LPIPS). Especially noteworthy is the 19-fold increase in the RMSE score, underscoring our method's exceptional generalization across different darkness levels. The code will be available online upon publication of the paper.
8.Interactive Class-Agnostic Object Counting
Authors:Yifeng Huang, Viresh Ranjan, Minh Hoai
Abstract: We propose a novel framework for interactive class-agnostic object counting, where a human user can interactively provide feedback to improve the accuracy of a counter. Our framework consists of two main components: a user-friendly visualizer to gather feedback and an efficient mechanism to incorporate it. In each iteration, we produce a density map to show the current prediction result, and we segment it into non-overlapping regions with an easily verifiable number of objects. The user can provide feedback by selecting a region with obvious counting errors and specifying the range for the estimated number of objects within it. To improve the counting result, we develop a novel adaptation loss to force the visual counter to output the predicted count within the user-specified range. For effective and efficient adaptation, we propose a refinement module that can be used with any density-based visual counter, and only the parameters in the refinement module will be updated during adaptation. Our experiments on two challenging class-agnostic object counting benchmarks, FSCD-LVIS and FSC-147, show that our method can reduce the mean absolute error of multiple state-of-the-art visual counters by roughly 30% to 40% with minimal user input. Our project can be found at https://yifehuang97.github.io/ICACountProjectPage/.
9.Class-Incremental Grouping Network for Continual Audio-Visual Learning
Authors:Shentong Mo, Weiguo Pian, Yapeng Tian
Abstract: Continual learning is a challenging problem in which models need to be trained on non-stationary data across sequential tasks for class-incremental learning. While previous methods have focused on using either regularization or rehearsal-based frameworks to alleviate catastrophic forgetting in image classification, they are limited to a single modality and cannot learn compact class-aware cross-modal representations for continual audio-visual learning. To address this gap, we propose a novel class-incremental grouping network (CIGN) that can learn category-wise semantic features to achieve continual audio-visual learning. Our CIGN leverages learnable audio-visual class tokens and audio-visual grouping to continually aggregate class-aware features. Additionally, it utilizes class tokens distillation and continual grouping to prevent forgetting parameters learned from previous tasks, thereby improving the model's ability to capture discriminative audio-visual categories. We conduct extensive experiments on VGGSound-Instruments, VGGSound-100, and VGG-Sound Sources benchmarks. Our experimental results demonstrate that the CIGN achieves state-of-the-art audio-visual class-incremental learning performance. Code is available at https://github.com/stoneMo/CIGN.
10.Can you text what is happening? Integrating pre-trained language encoders into trajectory prediction models for autonomous driving
Authors:Ali Keysan, Andreas Look, Eitan Kosman, Gonca Gürsun, Jörg Wagner, Yao Yu, Barbara Rakitsch
Abstract: In autonomous driving tasks, scene understanding is the first step towards predicting the future behavior of the surrounding traffic participants. Yet, how to represent a given scene and extract its features are still open research questions. In this study, we propose a novel text-based representation of traffic scenes and process it with a pre-trained language encoder. First, we show that text-based representations, combined with classical rasterized image representations, lead to descriptive scene embeddings. Second, we benchmark our predictions on the nuScenes dataset and show significant improvements compared to baselines. Third, we show in an ablation study that a joint encoder of text and rasterized images outperforms the individual encoders confirming that both representations have their complementary strengths.
11.Task-driven Compression for Collision Encoding based on Depth Images
Authors:Mihir Kulkarni, Kostas Alexis
Abstract: This paper contributes a novel learning-based method for aggressive task-driven compression of depth images and their encoding as images tailored to collision prediction for robotic systems. A novel 3D image processing methodology is proposed that accounts for the robot's size in order to appropriately "inflate" the obstacles represented in the depth image and thus obtain the distance that can be traversed by the robot in a collision-free manner along any given ray within the camera frustum. Such depth-and-collision image pairs are used to train a neural network that follows the architecture of Variational Autoencoders to compress-and-transform the information in the original depth image to derive a latent representation that encodes the collision information for the given depth image. We compare our proposed task-driven encoding method with classical task-agnostic methods and demonstrate superior performance for the task of collision image prediction from extremely low-dimensional latent spaces. A set of comparative studies show that the proposed approach is capable of encoding depth image-and-collision image tuples from complex scenes with thin obstacles at long distances better than the classical methods at compression ratios as high as 4050:1.
12.DeCUR: decoupling common & unique representations for multimodal self-supervision
Authors:Yi Wang, Conrad M Albrecht, Nassim Ait Ali Braham, Chenying Liu, Zhitong Xiong, Xiao Xiang Zhu
Abstract: The increasing availability of multi-sensor data sparks interest in multimodal self-supervised learning. However, most existing approaches learn only common representations across modalities while ignoring intra-modal training and modality-unique representations. We propose Decoupling Common and Unique Representations (DeCUR), a simple yet effective method for multimodal self-supervised learning. By distinguishing inter- and intra-modal embeddings, DeCUR is trained to integrate complementary information across different modalities. We evaluate DeCUR in three common multimodal scenarios (radar-optical, RGB-elevation, and RGB-depth), and demonstrate its consistent benefits on scene classification and semantic segmentation downstream tasks. Notably, we get straightforward improvements by transferring our pretrained backbones to state-of-the-art supervised multimodal methods without any hyperparameter tuning. Furthermore, we conduct a comprehensive explainability analysis to shed light on the interpretation of common and unique features in our multimodal approach. Codes are available at \url{https://github.com/zhu-xlab/DeCUR}.
13.Semantic Latent Decomposition with Normalizing Flows for Face Editing
Authors:Binglei Li, Zhizhong Huang, Hongming Shan, Junping Zhang
Abstract: Navigating in the latent space of StyleGAN has shown effectiveness for face editing. However, the resulting methods usually encounter challenges in complicated navigation due to the entanglement among different attributes in the latent space. To address this issue, this paper proposes a novel framework, termed SDFlow, with a semantic decomposition in original latent space using continuous conditional normalizing flows. Specifically, SDFlow decomposes the original latent code into different irrelevant variables by jointly optimizing two components: (i) a semantic encoder to estimate semantic variables from input faces and (ii) a flow-based transformation module to map the latent code into a semantic-irrelevant variable in Gaussian distribution, conditioned on the learned semantic variables. To eliminate the entanglement between variables, we employ a disentangled learning strategy under a mutual information framework, thereby providing precise manipulation controls. Experimental results demonstrate that SDFlow outperforms existing state-of-the-art face editing methods both qualitatively and quantitatively. The source code is made available at https://github.com/phil329/SDFlow.
14.Diff-Privacy: Diffusion-based Face Privacy Protection
Authors:Xiao He, Mingrui Zhu, Dongxin Chen, Nannan Wang, Xinbo Gao
Abstract: Privacy protection has become a top priority as the proliferation of AI techniques has led to widespread collection and misuse of personal data. Anonymization and visual identity information hiding are two important facial privacy protection tasks that aim to remove identification characteristics from facial images at the human perception level. However, they have a significant difference in that the former aims to prevent the machine from recognizing correctly, while the latter needs to ensure the accuracy of machine recognition. Therefore, it is difficult to train a model to complete these two tasks simultaneously. In this paper, we unify the task of anonymization and visual identity information hiding and propose a novel face privacy protection method based on diffusion models, dubbed Diff-Privacy. Specifically, we train our proposed multi-scale image inversion module (MSI) to obtain a set of SDM format conditional embeddings of the original image. Based on the conditional embeddings, we design corresponding embedding scheduling strategies and construct different energy functions during the denoising process to achieve anonymization and visual identity information hiding. Extensive experiments have been conducted to validate the effectiveness of our proposed framework in protecting facial privacy.
15.MultIOD: Rehearsal-free Multihead Incremental Object Detector
Authors:Eden Belouadah, Arnaud Dapogny, Kevin Bailly
Abstract: Class-Incremental learning (CIL) is the ability of artificial agents to accommodate new classes as they appear in a stream. It is particularly interesting in evolving environments where agents have limited access to memory and computational resources. The main challenge of class-incremental learning is catastrophic forgetting, the inability of neural networks to retain past knowledge when learning a new one. Unfortunately, most existing class-incremental object detectors are applied to two-stage algorithms such as Faster-RCNN and rely on rehearsal memory to retain past knowledge. We believe that the current benchmarks are not realistic, and more effort should be dedicated to anchor-free and rehearsal-free object detection. In this context, we propose MultIOD, a class-incremental object detector based on CenterNet. Our main contributions are: (1) we propose a multihead feature pyramid and multihead detection architecture to efficiently separate class representations, (2) we employ transfer learning between classes learned initially and those learned incrementally to tackle catastrophic forgetting, and (3) we use a class-wise non-max-suppression as a post-processing technique to remove redundant boxes. Without bells and whistles, our method outperforms a range of state-of-the-art methods on two Pascal VOC datasets.
16.CNN or ViT? Revisiting Vision Transformers Through the Lens of Convolution
Authors:Chenghao Li, Chaoning Zhang
Abstract: The success of Vision Transformer (ViT) has been widely reported on a wide range of image recognition tasks. The merit of ViT over CNN has been largely attributed to large training datasets or auxiliary pre-training. Without pre-training, the performance of ViT on small datasets is limited because the global self-attention has limited capacity in local modeling. Towards boosting ViT on small datasets without pre-training, this work improves its local modeling by applying a weight mask on the original self-attention matrix. A straightforward way to locally adapt the self-attention matrix can be realized by an element-wise learnable weight mask (ELM), for which our preliminary results show promising results. However, the element-wise simple learnable weight mask not only induces a non-trivial additional parameter overhead but also increases the optimization complexity. To this end, this work proposes a novel Gaussian mixture mask (GMM) in which one mask only has two learnable parameters and it can be conveniently used in any ViT variants whose attention mechanism allows the use of masks. Experimental results on multiple small datasets demonstrate that the effectiveness of our proposed Gaussian mask for boosting ViTs for free (almost zero additional parameter or computation cost). Our code will be publicly available at \href{https://github.com/CatworldLee/Gaussian-Mixture-Mask-Attention}{https://github.com/CatworldLee/Gaussian-Mixture-Mask-Attention}.
17.Collective PV-RCNN: A Novel Fusion Technique using Collective Detections for Enhanced Local LiDAR-Based Perception
Authors:Sven Teufel, Jörg Gamerdinger, Georg Volk, Oliver Bringmann
Abstract: Comprehensive perception of the environment is crucial for the safe operation of autonomous vehicles. However, the perception capabilities of autonomous vehicles are limited due to occlusions, limited sensor ranges, or environmental influences. Collective Perception (CP) aims to mitigate these problems by enabling the exchange of information between vehicles. A major challenge in CP is the fusion of the exchanged information. Due to the enormous bandwidth requirement of early fusion approaches and the interchangeability issues of intermediate fusion approaches, only the late fusion of shared detections is practical. Current late fusion approaches neglect valuable information for local detection, this is why we propose a novel fusion method to fuse the detections of cooperative vehicles within the local LiDAR-based detection pipeline. Therefore, we present Collective PV-RCNN (CPV-RCNN), which extends the PV-RCNN++ framework to fuse collective detections. Code is available at https://github.com/ekut-es
18.Robust Single Rotation Averaging Revisited
Authors:Seong Hun Lee, Javier Civera
Abstract: In this work, we propose a novel method for robust single rotation averaging that can efficiently handle an extremely large fraction of outliers. Our approach is to minimize the total truncated least unsquared deviations (TLUD) cost of geodesic distances. The proposed algorithm consists of three steps: First, we consider each input rotation as a potential initial solution and choose the one that yields the least sum of truncated chordal deviations. Next, we obtain the inlier set using the initial solution and compute its chordal $L_2$-mean. Finally, starting from this estimate, we iteratively compute the geodesic $L_1$-mean of the inliers using the Weiszfeld algorithm on $SO(3)$. An extensive evaluation shows that our method is robust against up to 99% outliers given a sufficient number of accurate inliers, outperforming the current state of the art.
19.FlowIBR: Leveraging Pre-Training for Efficient Neural Image-Based Rendering of Dynamic Scenes
Authors:Marcel Büsching, Josef Bengtson, David Nilsson, Mårten Björkman
Abstract: We introduce a novel approach for monocular novel view synthesis of dynamic scenes. Existing techniques already show impressive rendering quality but tend to focus on optimization within a single scene without leveraging prior knowledge. This limitation has been primarily attributed to the lack of datasets of dynamic scenes available for training and the diversity of scene dynamics. Our method FlowIBR circumvents these issues by integrating a neural image-based rendering method, pre-trained on a large corpus of widely available static scenes, with a per-scene optimized scene flow field. Utilizing this flow field, we bend the camera rays to counteract the scene dynamics, thereby presenting the dynamic scene as if it were static to the rendering network. The proposed method reduces per-scene optimization time by an order of magnitude, achieving comparable results to existing methods - all on a single consumer-grade GPU.
20.Towards Content-based Pixel Retrieval in Revisited Oxford and Paris
Authors:Guoyuan An, Woo Jae Kim, Saelyne Yang, Rong Li, Yuchi Huo, Sung-Eui Yoon
Abstract: This paper introduces the first two pixel retrieval benchmarks. Pixel retrieval is segmented instance retrieval. Like semantic segmentation extends classification to the pixel level, pixel retrieval is an extension of image retrieval and offers information about which pixels are related to the query object. In addition to retrieving images for the given query, it helps users quickly identify the query object in true positive images and exclude false positive images by denoting the correlated pixels. Our user study results show pixel-level annotation can significantly improve the user experience. Compared with semantic and instance segmentation, pixel retrieval requires a fine-grained recognition capability for variable-granularity targets. To this end, we propose pixel retrieval benchmarks named PROxford and PRParis, which are based on the widely used image retrieval datasets, ROxford and RParis. Three professional annotators label 5,942 images with two rounds of double-checking and refinement. Furthermore, we conduct extensive experiments and analysis on the SOTA methods in image search, image matching, detection, segmentation, and dense matching using our pixel retrieval benchmarks. Results show that the pixel retrieval task is challenging to these approaches and distinctive from existing problems, suggesting that further research can advance the content-based pixel-retrieval and thus user search experience. The datasets can be downloaded from \href{https://github.com/anguoyuan/Pixel_retrieval-Segmented_instance_retrieval}{this link}.
21.Panoptic Vision-Language Feature Fields
Authors:Haoran Chen, Kenneth Blomqvist, Francesco Milano, Roland Siegwart
Abstract: Recently, methods have been proposed for 3D open-vocabulary semantic segmentation. Such methods are able to segment scenes into arbitrary classes given at run-time using their text description. In this paper, we propose to our knowledge the first algorithm for open-vocabulary panoptic segmentation, simultaneously performing both semantic and instance segmentation. Our algorithm, Panoptic Vision-Language Feature Fields (PVLFF) learns a feature field of the scene, jointly learning vision-language features and hierarchical instance features through a contrastive loss function from 2D instance segment proposals on input frames. Our method achieves comparable performance against the state-of-the-art close-set 3D panoptic systems on the HyperSim, ScanNet and Replica dataset and outperforms current 3D open-vocabulary systems in terms of semantic segmentation. We additionally ablate our method to demonstrate the effectiveness of our model architecture. Our code will be available at https://github.com/ethz-asl/autolabel.
22.Dual-view Curricular Optimal Transport for Cross-lingual Cross-modal Retrieval
Authors:Yabing Wang, Shuhui Wang, Hao Luo, Jianfeng Dong, Fan Wang, Meng Han, Xun Wang, Meng Wang
Abstract: Current research on cross-modal retrieval is mostly English-oriented, as the availability of a large number of English-oriented human-labeled vision-language corpora. In order to break the limit of non-English labeled data, cross-lingual cross-modal retrieval (CCR) has attracted increasing attention. Most CCR methods construct pseudo-parallel vision-language corpora via Machine Translation (MT) to achieve cross-lingual transfer. However, the translated sentences from MT are generally imperfect in describing the corresponding visual contents. Improperly assuming the pseudo-parallel data are correctly correlated will make the networks overfit to the noisy correspondence. Therefore, we propose Dual-view Curricular Optimal Transport (DCOT) to learn with noisy correspondence in CCR. In particular, we quantify the confidence of the sample pair correlation with optimal transport theory from both the cross-lingual and cross-modal views, and design dual-view curriculum learning to dynamically model the transportation costs according to the learning stage of the two views. Extensive experiments are conducted on two multilingual image-text datasets and one video-text dataset, and the results demonstrate the effectiveness and robustness of the proposed method. Besides, our proposed method also shows a good expansibility to cross-lingual image-text baselines and a decent generalization on out-of-domain data.
23.Learning Semantic Segmentation with Query Points Supervision on Aerial Images
Authors:Santiago Rivier, Carlos Hinojosa, Silvio Giancola, Bernard Ghanem
Abstract: Semantic segmentation is crucial in remote sensing, where high-resolution satellite images are segmented into meaningful regions. Recent advancements in deep learning have significantly improved satellite image segmentation. However, most of these methods are typically trained in fully supervised settings that require high-quality pixel-level annotations, which are expensive and time-consuming to obtain. In this work, we present a weakly supervised learning algorithm to train semantic segmentation algorithms that only rely on query point annotations instead of full mask labels. Our proposed approach performs accurate semantic segmentation and improves efficiency by significantly reducing the cost and time required for manual annotation. Specifically, we generate superpixels and extend the query point labels into those superpixels that group similar meaningful semantics. Then, we train semantic segmentation models, supervised with images partially labeled with the superpixels pseudo-labels. We benchmark our weakly supervised training approach on an aerial image dataset and different semantic segmentation architectures, showing that we can reach competitive performance compared to fully supervised training while reducing the annotation effort.
24.Zero-Shot Co-salient Object Detection Framework
Authors:Haoke Xiao, Lv Tang, Bo Li, Zhiming Luo, Shaozi Li
Abstract: Co-salient Object Detection (CoSOD) endeavors to replicate the human visual system's capacity to recognize common and salient objects within a collection of images. Despite recent advancements in deep learning models, these models still rely on training with well-annotated CoSOD datasets. The exploration of training-free zero-shot CoSOD frameworks has been limited. In this paper, taking inspiration from the zero-shot transfer capabilities of foundational computer vision models, we introduce the first zero-shot CoSOD framework that harnesses these models without any training process. To achieve this, we introduce two novel components in our proposed framework: the group prompt generation (GPG) module and the co-saliency map generation (CMP) module. We evaluate the framework's performance on widely-used datasets and observe impressive results. Our approach surpasses existing unsupervised methods and even outperforms fully supervised methods developed before 2020, while remaining competitive with some fully supervised methods developed before 2022.
25.Stream-based Active Learning by Exploiting Temporal Properties in Perception with Temporal Predicted Loss
Authors:Sebastian Schmidt BMW and TUM, Stephan Günnemann TUM
Abstract: Active learning (AL) reduces the amount of labeled data needed to train a machine learning model by intelligently choosing which instances to label. Classic pool-based AL requires all data to be present in a datacenter, which can be challenging with the increasing amounts of data needed in deep learning. However, AL on mobile devices and robots, like autonomous cars, can filter the data from perception sensor streams before reaching the datacenter. We exploited the temporal properties for such image streams in our work and proposed the novel temporal predicted loss (TPL) method. To evaluate the stream-based setting properly, we introduced the GTA V streets and the A2D2 streets dataset and made both publicly available. Our experiments showed that our approach significantly improves the diversity of the selection while being an uncertainty-based method. As pool-based approaches are more common in perception applications, we derived a concept for comparing pool-based and stream-based AL, where TPL out-performed state-of-the-art pool- or stream-based approaches for different models. TPL demonstrated a gain of 2.5 precept points (pp) less required data while being significantly faster than pool-based methods.
26.ReSimAD: Zero-Shot 3D Domain Transfer for Autonomous Driving with Source Reconstruction and Target Simulation
Authors:Bo Zhang, Xinyu Cai, Jiakang Yuan, Donglin Yang, Jianfei Guo, Renqiu Xia, Botian Shi, Min Dou, Tao Chen, Si Liu, Junchi Yan, Yu Qiao
Abstract: Domain shifts such as sensor type changes and geographical situation variations are prevalent in Autonomous Driving (AD), which poses a challenge since AD model relying on the previous-domain knowledge can be hardly directly deployed to a new domain without additional costs. In this paper, we provide a new perspective and approach of alleviating the domain shifts, by proposing a Reconstruction-Simulation-Perception (ReSimAD) scheme. Specifically, the implicit reconstruction process is based on the knowledge from the previous old domain, aiming to convert the domain-related knowledge into domain-invariant representations, \textit{e.g.}, 3D scene-level meshes. Besides, the point clouds simulation process of multiple new domains is conditioned on the above reconstructed 3D meshes, where the target-domain-like simulation samples can be obtained, thus reducing the cost of collecting and annotating new-domain data for the subsequent perception process. For experiments, we consider different cross-domain situations such as Waymo-to-KITTI, Waymo-to-nuScenes, Waymo-to-ONCE, \textit{etc}, to verify the \textbf{zero-shot} target-domain perception using ReSimAD. Results demonstrate that our method is beneficial to boost the domain generalization ability, even promising for 3D pre-training.
27.On the detection of Out-Of-Distribution samples in Multiple Instance Learning
Authors:Loïc Le Bescond, Maria Vakalopoulou, Stergios Christodoulidis, Fabrice André, Hugues Talbot
Abstract: The deployment of machine learning solutions in real-world scenarios often involves addressing the challenge of out-of-distribution (OOD) detection. While significant efforts have been devoted to OOD detection in classical supervised settings, the context of weakly supervised learning, particularly the Multiple Instance Learning (MIL) framework, remains under-explored. In this study, we tackle this challenge by adapting post-hoc OOD detection methods to the MIL setting while introducing a novel benchmark specifically designed to assess OOD detection performance in weakly supervised scenarios. Extensive experiments based on diverse public datasets do not reveal a single method with a clear advantage over the others. Although DICE emerges as the best-performing method overall, it exhibits significant shortcomings on some datasets, emphasizing the complexity of this under-explored and challenging topic. Our findings shed light on the complex nature of OOD detection under the MIL framework, emphasizing the importance of developing novel, robust, and reliable methods that can generalize effectively in a weakly supervised context. The code for the paper is available here: https://github.com/loic-lb/OOD_MIL.
28.Distance-Aware eXplanation Based Learning
Authors:Misgina Tsighe Hagos, Niamh Belton, Kathleen M. Curran, Brian Mac Namee
Abstract: eXplanation Based Learning (XBL) is an interactive learning approach that provides a transparent method of training deep learning models by interacting with their explanations. XBL augments loss functions to penalize a model based on deviation of its explanations from user annotation of image features. The literature on XBL mostly depends on the intersection of visual model explanations and image feature annotations. We present a method to add a distance-aware explanation loss to categorical losses that trains a learner to focus on important regions of a training dataset. Distance is an appropriate approach for calculating explanation loss since visual model explanations such as Gradient-weighted Class Activation Mapping (Grad-CAMs) are not strictly bounded as annotations and their intersections may not provide complete information on the deviation of a model's focus from relevant image regions. In addition to assessing our model using existing metrics, we propose an interpretability metric for evaluating visual feature-attribution based model explanations that is more informative of the model's performance than existing metrics. We demonstrate performance of our proposed method on three image classification tasks.
29.OpenFashionCLIP: Vision-and-Language Contrastive Learning with Open-Source Fashion Data
Authors:Giuseppe Cartella, Alberto Baldrati, Davide Morelli, Marcella Cornia, Marco Bertini, Rita Cucchiara
Abstract: The inexorable growth of online shopping and e-commerce demands scalable and robust machine learning-based solutions to accommodate customer requirements. In the context of automatic tagging classification and multimodal retrieval, prior works either defined a low generalizable supervised learning approach or more reusable CLIP-based techniques while, however, training on closed source data. In this work, we propose OpenFashionCLIP, a vision-and-language contrastive learning method that only adopts open-source fashion data stemming from diverse domains, and characterized by varying degrees of specificity. Our approach is extensively validated across several tasks and benchmarks, and experimental results highlight a significant out-of-domain generalization capability and consistent improvements over state-of-the-art methods both in terms of accuracy and recall. Source code and trained models are publicly available at: https://github.com/aimagelab/open-fashion-clip.
30.ITI-GEN: Inclusive Text-to-Image Generation
Authors:Cheng Zhang, Xuanbai Chen, Siqi Chai, Chen Henry Wu, Dmitry Lagun, Thabo Beeler, Fernando De la Torre
Abstract: Text-to-image generative models often reflect the biases of the training data, leading to unequal representations of underrepresented groups. This study investigates inclusive text-to-image generative models that generate images based on human-written prompts and ensure the resulting images are uniformly distributed across attributes of interest. Unfortunately, directly expressing the desired attributes in the prompt often leads to sub-optimal results due to linguistic ambiguity or model misrepresentation. Hence, this paper proposes a drastically different approach that adheres to the maxim that "a picture is worth a thousand words". We show that, for some attributes, images can represent concepts more expressively than text. For instance, categories of skin tones are typically hard to specify by text but can be easily represented by example images. Building upon these insights, we propose a novel approach, ITI-GEN, that leverages readily available reference images for Inclusive Text-to-Image GENeration. The key idea is learning a set of prompt embeddings to generate images that can effectively represent all desired attribute categories. More importantly, ITI-GEN requires no model fine-tuning, making it computationally efficient to augment existing text-to-image models. Extensive experiments demonstrate that ITI-GEN largely improves over state-of-the-art models to generate inclusive images from a prompt. Project page: https://czhang0528.github.io/iti-gen.
31.UniSeg: A Unified Multi-Modal LiDAR Segmentation Network and the OpenPCSeg Codebase
Authors:Youquan Liu, Runnan Chen, Xin Li, Lingdong Kong, Yuchen Yang, Zhaoyang Xia, Yeqi Bai, Xinge Zhu, Yuexin Ma, Yikang Li, Yu Qiao, Yuenan Hou
Abstract: Point-, voxel-, and range-views are three representative forms of point clouds. All of them have accurate 3D measurements but lack color and texture information. RGB images are a natural complement to these point cloud views and fully utilizing the comprehensive information of them benefits more robust perceptions. In this paper, we present a unified multi-modal LiDAR segmentation network, termed UniSeg, which leverages the information of RGB images and three views of the point cloud, and accomplishes semantic segmentation and panoptic segmentation simultaneously. Specifically, we first design the Learnable cross-Modal Association (LMA) module to automatically fuse voxel-view and range-view features with image features, which fully utilize the rich semantic information of images and are robust to calibration errors. Then, the enhanced voxel-view and range-view features are transformed to the point space,where three views of point cloud features are further fused adaptively by the Learnable cross-View Association module (LVA). Notably, UniSeg achieves promising results in three public benchmarks, i.e., SemanticKITTI, nuScenes, and Waymo Open Dataset (WOD); it ranks 1st on two challenges of two benchmarks, including the LiDAR semantic segmentation challenge of nuScenes and panoptic segmentation challenges of SemanticKITTI. Besides, we construct the OpenPCSeg codebase, which is the largest and most comprehensive outdoor LiDAR segmentation codebase. It contains most of the popular outdoor LiDAR segmentation algorithms and provides reproducible implementations. The OpenPCSeg codebase will be made publicly available at https://github.com/PJLab-ADG/PCSeg.
32.Temporal Action Localization with Enhanced Instant Discriminability
Authors:Dingfeng Shi, Qiong Cao, Yujie Zhong, Shan An, Jian Cheng, Haogang Zhu, Dacheng Tao
Abstract: Temporal action detection (TAD) aims to detect all action boundaries and their corresponding categories in an untrimmed video. The unclear boundaries of actions in videos often result in imprecise predictions of action boundaries by existing methods. To resolve this issue, we propose a one-stage framework named TriDet. First, we propose a Trident-head to model the action boundary via an estimated relative probability distribution around the boundary. Then, we analyze the rank-loss problem (i.e. instant discriminability deterioration) in transformer-based methods and propose an efficient scalable-granularity perception (SGP) layer to mitigate this issue. To further push the limit of instant discriminability in the video backbone, we leverage the strong representation capability of pretrained large models and investigate their performance on TAD. Last, considering the adequate spatial-temporal context for classification, we design a decoupled feature pyramid network with separate feature pyramids to incorporate rich spatial context from the large model for localization. Experimental results demonstrate the robustness of TriDet and its state-of-the-art performance on multiple TAD datasets, including hierarchical (multilabel) TAD datasets.
33.Learning the Geodesic Embedding with Graph Neural Networks
Authors:Bo Pang, Zhongtian Zheng, Guoping Wang, Peng-Shuai Wang
Abstract: We present GeGnn, a learning-based method for computing the approximate geodesic distance between two arbitrary points on discrete polyhedra surfaces with constant time complexity after fast precomputation. Previous relevant methods either focus on computing the geodesic distance between a single source and all destinations, which has linear complexity at least or require a long precomputation time. Our key idea is to train a graph neural network to embed an input mesh into a high-dimensional embedding space and compute the geodesic distance between a pair of points using the corresponding embedding vectors and a lightweight decoding function. To facilitate the learning of the embedding, we propose novel graph convolution and graph pooling modules that incorporate local geodesic information and are verified to be much more effective than previous designs. After training, our method requires only one forward pass of the network per mesh as precomputation. Then, we can compute the geodesic distance between a pair of points using our decoding function, which requires only several matrix multiplications and can be massively parallelized on GPUs. We verify the efficiency and effectiveness of our method on ShapeNet and demonstrate that our method is faster than existing methods by orders of magnitude while achieving comparable or better accuracy. Additionally, our method exhibits robustness on noisy and incomplete meshes and strong generalization ability on out-of-distribution meshes. The code and pretrained model can be found on https://github.com/IntelligentGeometry/GeGnn.
34.CitDet: A Benchmark Dataset for Citrus Fruit Detection
Authors:Jordan A. James, Heather K. Manching, Matthew R. Mattia, Kim D. Bowman, Amanda M. Hulse-Kemp, William J. Beksi
Abstract: In this letter, we present a new dataset to advance the state of the art in detecting citrus fruit and accurately estimate yield on trees affected by the Huanglongbing (HLB) disease in orchard environments via imaging. Despite the fact that significant progress has been made in solving the fruit detection problem, the lack of publicly available datasets has complicated direct comparison of results. For instance, citrus detection has long been of interest in the agricultural research community, yet there is an absence of work, particularly involving public datasets of citrus affected by HLB. To address this issue, we enhance state-of-the-art object detection methods for use in typical orchard settings. Concretely, we provide high-resolution images of citrus trees located in an area known to be highly affected by HLB, along with high-quality bounding box annotations of citrus fruit. Fruit on both the trees and the ground are labeled to allow for identification of fruit location, which contributes to advancements in yield estimation and potential measure of HLB impact via fruit drop. The dataset consists of over 32,000 bounding box annotations for fruit instances contained in 579 high-resolution images. In summary, our contributions are the following: (i) we introduce a novel dataset along with baseline performance benchmarks on multiple contemporary object detection algorithms, (ii) we show the ability to accurately capture fruit location on tree or on ground, and finally (ii) we present a correlation of our results with yield estimations.
35.An Effective Two-stage Training Paradigm Detector for Small Dataset
Authors:Zheng Wang, Dong Xie, Hanzhi Wang, Jiang Tian
Abstract: Learning from the limited amount of labeled data to the pre-train model has always been viewed as a challenging task. In this report, an effective and robust solution, the two-stage training paradigm YOLOv8 detector (TP-YOLOv8), is designed for the object detection track in VIPriors Challenge 2023. First, the backbone of YOLOv8 is pre-trained as the encoder using the masked image modeling technique. Then the detector is fine-tuned with elaborate augmentations. During the test stage, test-time augmentation (TTA) is used to enhance each model, and weighted box fusion (WBF) is implemented to further boost the performance. With the well-designed structure, our approach has achieved 30.4% average precision from 0.50 to 0.95 on the DelftBikes test set, ranking 4th on the leaderboard.
36.Diffusion-Guided Reconstruction of Everyday Hand-Object Interaction Clips
Authors:Yufei Ye, Poorvi Hebbar, Abhinav Gupta, Shubham Tulsiani
Abstract: We tackle the task of reconstructing hand-object interactions from short video clips. Given an input video, our approach casts 3D inference as a per-video optimization and recovers a neural 3D representation of the object shape, as well as the time-varying motion and hand articulation. While the input video naturally provides some multi-view cues to guide 3D inference, these are insufficient on their own due to occlusions and limited viewpoint variations. To obtain accurate 3D, we augment the multi-view signals with generic data-driven priors to guide reconstruction. Specifically, we learn a diffusion network to model the conditional distribution of (geometric) renderings of objects conditioned on hand configuration and category label, and leverage it as a prior to guide the novel-view renderings of the reconstructed scene. We empirically evaluate our approach on egocentric videos across 6 object categories, and observe significant improvements over prior single-view and multi-view methods. Finally, we demonstrate our system's ability to reconstruct arbitrary clips from YouTube, showing both 1st and 3rd person interactions.