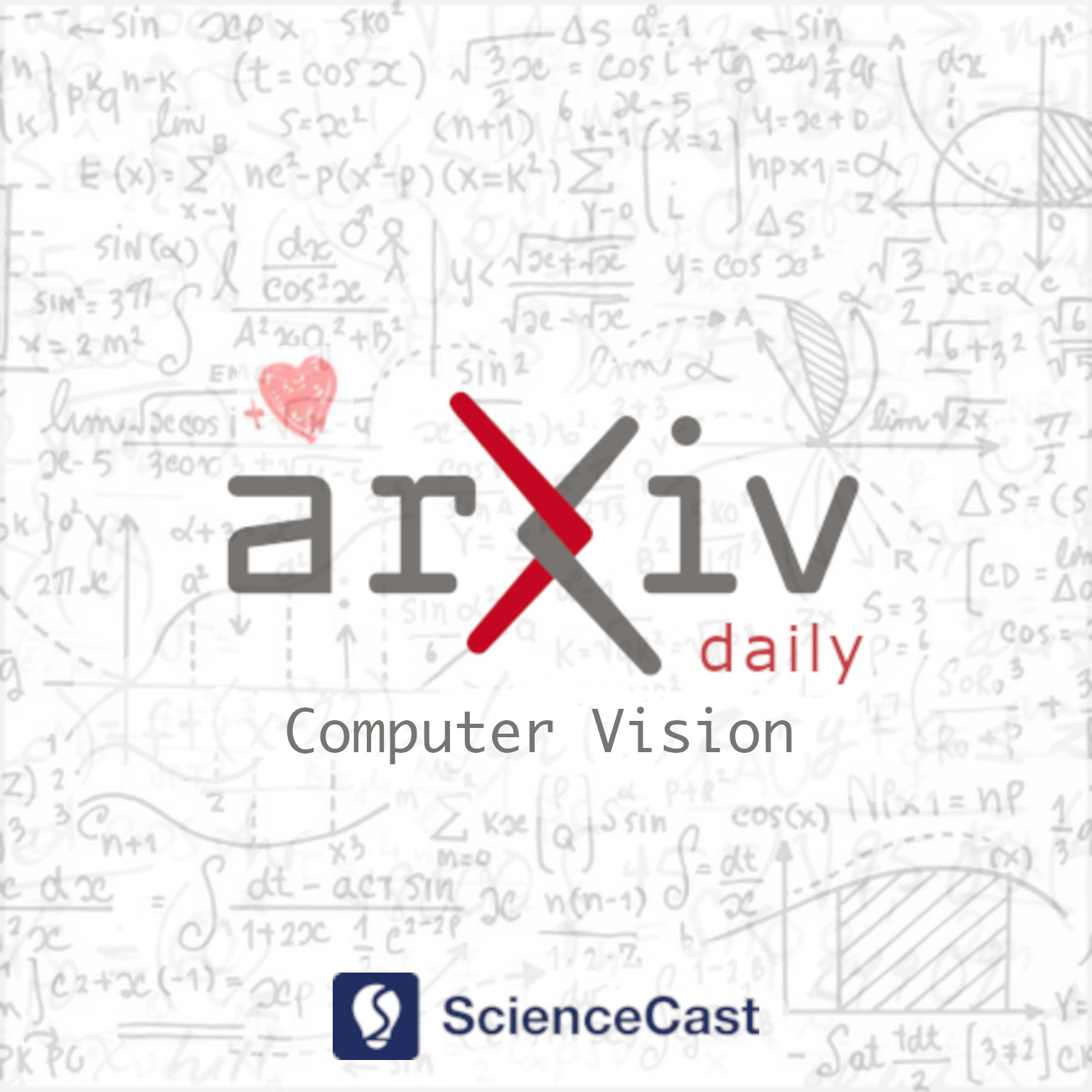
Computer Vision and Pattern Recognition (cs.CV)
Mon, 24 Apr 2023
1.Hierarchical Diffusion Autoencoders and Disentangled Image Manipulation
Authors:Zeyu Lu, Chengyue Wu, Xinyuan Chen, Yaohui Wang, Yu Qiao, Xihui Liu
Abstract: Diffusion models have attained impressive visual quality for image synthesis. However, how to interpret and manipulate the latent space of diffusion models has not been extensively explored. Prior work diffusion autoencoders encode the semantic representations into a semantic latent code, which fails to reflect the rich information of details and the intrinsic feature hierarchy. To mitigate those limitations, we propose Hierarchical Diffusion Autoencoders (HDAE) that exploit the fine-grained-to-abstract and lowlevel-to-high-level feature hierarchy for the latent space of diffusion models. The hierarchical latent space of HDAE inherently encodes different abstract levels of semantics and provides more comprehensive semantic representations. In addition, we propose a truncated-feature-based approach for disentangled image manipulation. We demonstrate the effectiveness of our proposed approach with extensive experiments and applications on image reconstruction, style mixing, controllable interpolation, detail-preserving and disentangled image manipulation, and multi-modal semantic image synthesis.
2.Function-Consistent Feature Distillation
Authors:Dongyang Liu, Meina Kan, Shiguang Shan, Xilin Chen
Abstract: Feature distillation makes the student mimic the intermediate features of the teacher. Nearly all existing feature-distillation methods use L2 distance or its slight variants as the distance metric between teacher and student features. However, while L2 distance is isotropic w.r.t. all dimensions, the neural network's operation on different dimensions is usually anisotropic, i.e., perturbations with the same 2-norm but in different dimensions of intermediate features lead to changes in the final output with largely different magnitude. Considering this, we argue that the similarity between teacher and student features should not be measured merely based on their appearance (i.e., L2 distance), but should, more importantly, be measured by their difference in function, namely how later layers of the network will read, decode, and process them. Therefore, we propose Function-Consistent Feature Distillation (FCFD), which explicitly optimizes the functional similarity between teacher and student features. The core idea of FCFD is to make teacher and student features not only numerically similar, but more importantly produce similar outputs when fed to the later part of the same network. With FCFD, the student mimics the teacher more faithfully and learns more from the teacher. Extensive experiments on image classification and object detection demonstrate the superiority of FCFD to existing methods. Furthermore, we can combine FCFD with many existing methods to obtain even higher accuracy. Our codes are available at https://github.com/LiuDongyang6/FCFD.
3.Auto-CARD: Efficient and Robust Codec Avatar Driving for Real-time Mobile Telepresence
Authors:Yonggan Fu, Yuecheng Li, Chenghui Li, Jason Saragih, Peizhao Zhang, Xiaoliang Dai, Yingyan Lin
Abstract: Real-time and robust photorealistic avatars for telepresence in AR/VR have been highly desired for enabling immersive photorealistic telepresence. However, there still exists one key bottleneck: the considerable computational expense needed to accurately infer facial expressions captured from headset-mounted cameras with a quality level that can match the realism of the avatar's human appearance. To this end, we propose a framework called Auto-CARD, which for the first time enables real-time and robust driving of Codec Avatars when exclusively using merely on-device computing resources. This is achieved by minimizing two sources of redundancy. First, we develop a dedicated neural architecture search technique called AVE-NAS for avatar encoding in AR/VR, which explicitly boosts both the searched architectures' robustness in the presence of extreme facial expressions and hardware friendliness on fast evolving AR/VR headsets. Second, we leverage the temporal redundancy in consecutively captured images during continuous rendering and develop a mechanism dubbed LATEX to skip the computation of redundant frames. Specifically, we first identify an opportunity from the linearity of the latent space derived by the avatar decoder and then propose to perform adaptive latent extrapolation for redundant frames. For evaluation, we demonstrate the efficacy of our Auto-CARD framework in real-time Codec Avatar driving settings, where we achieve a 5.05x speed-up on Meta Quest 2 while maintaining a comparable or even better animation quality than state-of-the-art avatar encoder designs.
4.Robust and Efficient Memory Network for Video Object Segmentation
Authors:Yadang Chen, Dingwei Zhang, Zhi-xin Yang, Enhua Wu
Abstract: This paper proposes a Robust and Efficient Memory Network, referred to as REMN, for studying semi-supervised video object segmentation (VOS). Memory-based methods have recently achieved outstanding VOS performance by performing non-local pixel-wise matching between the query and memory. However, these methods have two limitations. 1) Non-local matching could cause distractor objects in the background to be incorrectly segmented. 2) Memory features with high temporal redundancy consume significant computing resources. For limitation 1, we introduce a local attention mechanism that tackles the background distraction by enhancing the features of foreground objects with the previous mask. For limitation 2, we first adaptively decide whether to update the memory features depending on the variation of foreground objects to reduce temporal redundancy. Second, we employ a dynamic memory bank, which uses a lightweight and differentiable soft modulation gate to decide how many memory features need to be removed in the temporal dimension. Experiments demonstrate that our REMN achieves state-of-the-art results on DAVIS 2017, with a $\mathcal{J\&F}$ score of 86.3% and on YouTube-VOS 2018, with a $\mathcal{G}$ over mean of 85.5%. Furthermore, our network shows a high inference speed of 25+ FPS and uses relatively few computing resources.
5.Gen-NeRF: Efficient and Generalizable Neural Radiance Fields via Algorithm-Hardware Co-Design
Authors:Yonggan Fu Celine, Zhifan Ye Celine, Jiayi Yuan Celine, Shunyao Zhang Celine, Sixu Li Celine, Haoran You Celine, Yingyan Celine, Lin
Abstract: Novel view synthesis is an essential functionality for enabling immersive experiences in various Augmented- and Virtual-Reality (AR/VR) applications, for which generalizable Neural Radiance Fields (NeRFs) have gained increasing popularity thanks to their cross-scene generalization capability. Despite their promise, the real-device deployment of generalizable NeRFs is bottlenecked by their prohibitive complexity due to the required massive memory accesses to acquire scene features, causing their ray marching process to be memory-bounded. To this end, we propose Gen-NeRF, an algorithm-hardware co-design framework dedicated to generalizable NeRF acceleration, which for the first time enables real-time generalizable NeRFs. On the algorithm side, Gen-NeRF integrates a coarse-then-focus sampling strategy, leveraging the fact that different regions of a 3D scene contribute differently to the rendered pixel, to enable sparse yet effective sampling. On the hardware side, Gen-NeRF highlights an accelerator micro-architecture to maximize the data reuse opportunities among different rays by making use of their epipolar geometric relationship. Furthermore, our Gen-NeRF accelerator features a customized dataflow to enhance data locality during point-to-hardware mapping and an optimized scene feature storage strategy to minimize memory bank conflicts. Extensive experiments validate the effectiveness of our proposed Gen-NeRF framework in enabling real-time and generalizable novel view synthesis.
6.Grad-PU: Arbitrary-Scale Point Cloud Upsampling via Gradient Descent with Learned Distance Functions
Authors:Yun He, Danhang Tang, Yinda Zhang, Xiangyang Xue, Yanwei Fu
Abstract: Most existing point cloud upsampling methods have roughly three steps: feature extraction, feature expansion and 3D coordinate prediction. However,they usually suffer from two critical issues: (1)fixed upsampling rate after one-time training, since the feature expansion unit is customized for each upsampling rate; (2)outliers or shrinkage artifact caused by the difficulty of precisely predicting 3D coordinates or residuals of upsampled points. To adress them, we propose a new framework for accurate point cloud upsampling that supports arbitrary upsampling rates. Our method first interpolates the low-res point cloud according to a given upsampling rate. And then refine the positions of the interpolated points with an iterative optimization process, guided by a trained model estimating the difference between the current point cloud and the high-res target. Extensive quantitative and qualitative results on benchmarks and downstream tasks demonstrate that our method achieves the state-of-the-art accuracy and efficiency.
7.Glocal Energy-based Learning for Few-Shot Open-Set Recognition
Authors:Haoyu Wang, Guansong Pang, Peng Wang, Lei Zhang, Wei Wei, Yanning Zhang
Abstract: Few-shot open-set recognition (FSOR) is a challenging task of great practical value. It aims to categorize a sample to one of the pre-defined, closed-set classes illustrated by few examples while being able to reject the sample from unknown classes. In this work, we approach the FSOR task by proposing a novel energy-based hybrid model. The model is composed of two branches, where a classification branch learns a metric to classify a sample to one of closed-set classes and the energy branch explicitly estimates the open-set probability. To achieve holistic detection of open-set samples, our model leverages both class-wise and pixel-wise features to learn a glocal energy-based score, in which a global energy score is learned using the class-wise features, while a local energy score is learned using the pixel-wise features. The model is enforced to assign large energy scores to samples that are deviated from the few-shot examples in either the class-wise features or the pixel-wise features, and to assign small energy scores otherwise. Experiments on three standard FSOR datasets show the superior performance of our model.
8.Accurate and Efficient Event-based Semantic Segmentation Using Adaptive Spiking Encoder-Decoder Network
Authors:Rui Zhang, Luziwei Leng, Kaiwei Che, Hu Zhang, Jie Cheng, Qinghai Guo, Jiangxing Liao, Ran Cheng
Abstract: Low-power event-driven computation and inherent temporal dynamics render spiking neural networks (SNNs) ideal candidates for processing highly dynamic and asynchronous signals from event-based sensors. However, due to the challenges in training and architectural design constraints, there is a scarcity of competitive demonstrations of SNNs in event-based dense prediction compared to artificial neural networks (ANNs). In this work, we construct an efficient spiking encoder-decoder network for large-scale event-based semantic segmentation tasks, optimizing the encoder with hierarchical search. To improve learning from highly dynamic event streams, we exploit the intrinsic adaptive threshold of spiking neurons to modulate network activation. Additionally, we develop a dual-path spiking spatially-adaptive modulation (SSAM) block to enhance the representation of sparse events, significantly improving network performance. Our network achieves 72.57% mean intersection over union (MIoU) on the DDD17 dataset and 57.22% MIoU on the newly proposed larger DSEC-Semantic dataset, surpassing current record ANNs by 4% while utilizing much lower computation costs. To the best of our knowledge, this is the first instance of SNNs outperforming ANNs in challenging event-based semantic segmentation tasks, demonstrating their immense potential in event-based vision. Our code will be publicly available.
9.Fitness-for-Duty Classification using Temporal Sequences of Iris Periocular images
Authors:Pamela C. Zurita, Daniel P. Benalcazar, Juan E. Tapia
Abstract: Fitness for Duty (FFD) techniques detects whether a subject is Fit to perform their work safely, which means no reduced alertness condition and security, or if they are Unfit, which means alertness condition reduced by sleepiness or consumption of alcohol and drugs. Human iris behaviour provides valuable information to predict FFD since pupil and iris movements are controlled by the central nervous system and are influenced by illumination, fatigue, alcohol, and drugs. This work aims to classify FFD using sequences of 8 iris images and to extract spatial and temporal information using Convolutional Neural Networks (CNN) and Long Short Term Memory Networks (LSTM). Our results achieved a precision of 81.4\% and 96.9\% for the prediction of Fit and Unfit subjects, respectively. The results also show that it is possible to determine if a subject is under alcohol, drug, and sleepiness conditions. Sleepiness can be identified as the most difficult condition to be determined. This system opens a different insight into iris biometric applications.
10.Universal Domain Adaptation via Compressive Attention Matching
Authors:Didi Zhu, Yincuan Li, Junkun Yuan, Zexi Li, Yunfeng Shao, Kun Kuang, Chao Wu
Abstract: Universal domain adaptation (UniDA) aims to transfer knowledge from the source domain to the target domain without any prior knowledge about the label set. The challenge lies in how to determine whether the target samples belong to common categories. The mainstream methods make judgments based on the sample features, which overemphasizes global information while ignoring the most crucial local objects in the image, resulting in limited accuracy. To address this issue, we propose a Universal Attention Matching (UniAM) framework by exploiting the self-attention mechanism in vision transformer to capture the crucial object information. The proposed framework introduces a novel Compressive Attention Matching (CAM) approach to explore the core information by compressively representing attentions. Furthermore, CAM incorporates a residual-based measurement to determine the sample commonness. By utilizing the measurement, UniAM achieves domain-wise and category-wise Common Feature Alignment (CFA) and Target Class Separation (TCS). Notably, UniAM is the first method utilizing the attention in vision transformer directly to perform classification tasks. Extensive experiments show that UniAM outperforms the current state-of-the-art methods on various benchmark datasets.
11.A Benchmark for Cycling Close Pass Near Miss Event Detection from Video Streams
Authors:Mingjie Li, Tharindu Rathnayake, Ben Beck, Lingheng Meng, Zijue Chen, Akansel Cosgun, Xiaojun Chang, Dana Kulić
Abstract: Cycling is a healthy and sustainable mode of transport. However, interactions with motor vehicles remain a key barrier to increased cycling participation. The ability to detect potentially dangerous interactions from on-bike sensing could provide important information to riders and policy makers. Thus, automated detection of conflict between cyclists and drivers has attracted researchers from both computer vision and road safety communities. In this paper, we introduce a novel benchmark, called Cyc-CP, towards cycling close pass near miss event detection from video streams. We first divide this task into scene-level and instance-level problems. Scene-level detection asks an algorithm to predict whether there is a close pass near miss event in the input video clip. Instance-level detection aims to detect which vehicle in the scene gives rise to a close pass near miss. We propose two benchmark models based on deep learning techniques for these two problems. For training and testing those models, we construct a synthetic dataset and also collect a real-world dataset. Our models can achieve 88.13% and 84.60% accuracy on the real-world dataset, respectively. We envision this benchmark as a test-bed to accelerate cycling close pass near miss detection and facilitate interaction between the fields of road safety, intelligent transportation systems and artificial intelligence. Both the benchmark datasets and detection models will be available at https://github.com/SustainableMobility/cyc-cp to facilitate experimental reproducibility and encourage more in-depth research in the field.
12.Underwater object classification combining SAS and transferred optical-to-SAS Imagery
Authors:Avi Abu, Roee Diamant
Abstract: Combining synthetic aperture sonar (SAS) imagery with optical images for underwater object classification has the potential to overcome challenges such as water clarity, the stability of the optical image analysis platform, and strong reflections from the seabed for sonar-based classification. In this work, we propose this type of multi-modal combination to discriminate between man-made targets and objects such as rocks or litter. We offer a novel classification algorithm that overcomes the problem of intensity and object formation differences between the two modalities. To this end, we develop a novel set of geometrical shape descriptors that takes into account the geometrical relation between the objects shadow and highlight. Results from 7,052 pairs of SAS and optical images collected during several sea experiments show improved classification performance compared to the state-of-the-art for better discrimination between different types of underwater objects. For reproducibility, we share our database.
13.Learning Visibility Field for Detailed 3D Human Reconstruction and Relighting
Authors:Ruichen Zheng, Peng Li, Haoqian Wang, Tao Yu
Abstract: Detailed 3D reconstruction and photo-realistic relighting of digital humans are essential for various applications. To this end, we propose a novel sparse-view 3d human reconstruction framework that closely incorporates the occupancy field and albedo field with an additional visibility field--it not only resolves occlusion ambiguity in multiview feature aggregation, but can also be used to evaluate light attenuation for self-shadowed relighting. To enhance its training viability and efficiency, we discretize visibility onto a fixed set of sample directions and supply it with coupled geometric 3D depth feature and local 2D image feature. We further propose a novel rendering-inspired loss, namely TransferLoss, to implicitly enforce the alignment between visibility and occupancy field, enabling end-to-end joint training. Results and extensive experiments demonstrate the effectiveness of the proposed method, as it surpasses state-of-the-art in terms of reconstruction accuracy while achieving comparably accurate relighting to ray-traced ground truth.
14.Transformer-based stereo-aware 3D object detection from binocular images
Authors:Hanqing Sun, Yanwei Pang, Jiale Cao, Jin Xie, Xuelong Li
Abstract: Vision Transformers have shown promising progress in various object detection tasks, including monocular 2D/3D detection and surround-view 3D detection. However, when used in essential and classic stereo 3D object detection, directly adopting those surround-view Transformers leads to slow convergence and significant precision drops. We argue that one of the causes of this defect is that the surround-view Transformers do not consider the stereo-specific image correspondence information. In a surround-view system, the overlapping areas are small, and thus correspondence is not a primary issue. In this paper, we explore the model design of vision Transformers in stereo 3D object detection, focusing particularly on extracting and encoding the task-specific image correspondence information. To achieve this goal, we present TS3D, a Transformer-based Stereo-aware 3D object detector. In the TS3D, a Disparity-Aware Positional Encoding (DAPE) model is proposed to embed the image correspondence information into stereo features. The correspondence is encoded as normalized disparity and is used in conjunction with sinusoidal 2D positional encoding to provide the location information of the 3D scene. To extract enriched multi-scale stereo features, we propose a Stereo Reserving Feature Pyramid Network (SRFPN). The SRFPN is designed to reserve the correspondence information while fusing intra-scale and aggregating cross-scale stereo features. Our proposed TS3D achieves a 41.29% Moderate Car detection average precision on the KITTI test set and takes 88 ms to detect objects from each binocular image pair. It is competitive with advanced counterparts in terms of both precision and inference speed.
15.Improving Knowledge Distillation Via Transferring Learning Ability
Authors:Long Liu, Tong Li, Hui Cheng
Abstract: Existing knowledge distillation methods generally use a teacher-student approach, where the student network solely learns from a well-trained teacher. However, this approach overlooks the inherent differences in learning abilities between the teacher and student networks, thus causing the capacity-gap problem. To address this limitation, we propose a novel method called SLKD.
16.Survey on Unsupervised Domain Adaptation for Semantic Segmentation for Visual Perception in Automated Driving
Authors:Manuel Schwonberg, Joshua Niemeijer, Jan-Aike Termöhlen, Jörg P. Schäfer, Nico M. Schmidt, Hanno Gottschalk, Tim Fingscheidt
Abstract: Deep neural networks (DNNs) have proven their capabilities in many areas in the past years, such as robotics, or automated driving, enabling technological breakthroughs. DNNs play a significant role in environment perception for the challenging application of automated driving and are employed for tasks such as detection, semantic segmentation, and sensor fusion. Despite this progress and tremendous research efforts, several issues still need to be addressed that limit the applicability of DNNs in automated driving. The bad generalization of DNNs to new, unseen domains is a major problem on the way to a safe, large-scale application, because manual annotation of new domains is costly, particularly for semantic segmentation. For this reason, methods are required to adapt DNNs to new domains without labeling effort. The task, which these methods aim to solve is termed unsupervised domain adaptation (UDA). While several different domain shifts can challenge DNNs, the shift between synthetic and real data is of particular importance for automated driving, as it allows the use of simulation environments for DNN training. In this work, we present an overview of the current state of the art in this field of research. We categorize and explain the different approaches for UDA. The number of considered publications is larger than any other survey on this topic. The scope of this survey goes far beyond the description of the UDA state-of-the-art. Based on our large data and knowledge base, we present a quantitative comparison of the approaches and use the observations to point out the latest trends in this field. In the following, we conduct a critical analysis of the state-of-the-art and highlight promising future research directions. With this survey, we aim to facilitate UDA research further and encourage scientists to exploit novel research directions to generalize DNNs better.
17.UTSGAN: Unseen Transition Suss GAN for Transition-Aware Image-to-image Translation
Authors:Yaxin Shi, Xiaowei Zhou, Ping Liu, Ivor W. Tsang
Abstract: In the field of Image-to-Image (I2I) translation, ensuring consistency between input images and their translated results is a key requirement for producing high-quality and desirable outputs. Previous I2I methods have relied on result consistency, which enforces consistency between the translated results and the ground truth output, to achieve this goal. However, result consistency is limited in its ability to handle complex and unseen attribute changes in translation tasks. To address this issue, we introduce a transition-aware approach to I2I translation, where the data translation mapping is explicitly parameterized with a transition variable, allowing for the modelling of unobserved translations triggered by unseen transitions. Furthermore, we propose the use of transition consistency, defined on the transition variable, to enable regularization of consistency on unobserved translations, which is omitted in previous works. Based on these insights, we present Unseen Transition Suss GAN (UTSGAN), a generative framework that constructs a manifold for the transition with a stochastic transition encoder and coherently regularizes and generalizes result consistency and transition consistency on both training and unobserved translations with tailor-designed constraints. Extensive experiments on four different I2I tasks performed on five different datasets demonstrate the efficacy of our proposed UTSGAN in performing consistent translations.
18.Few-shot Class-incremental Pill Recognition
Authors:Jinghua Zhang, Li Liu, Kai Gao, Dewen Hu
Abstract: The automatic pill recognition system is of great significance in improving the efficiency of the hospital, helping people with visual impairment, and avoiding cross-infection. However, most existing pill recognition systems based on deep learning can merely perform pill classification on the learned pill categories with sufficient training data. In practice, the expensive cost of data annotation and the continuously increasing categories of new pills make it meaningful to develop a few-shot class-incremental pill recognition system. In this paper, we develop the first few-shot class-incremental pill recognition system, which adopts decoupled learning strategy of representations and classifiers. In learning representations, we propose the novel Center-Triplet loss function, which can promote intra-class compactness and inter-class separability. In learning classifiers, we propose a specialized pseudo pill image construction strategy to train the Graph Attention Network to obtain the adaptation model. Moreover, we construct two new pill image datasets for few-shot class-incremental learning. The experimental results show that our framework outperforms the state-of-the-art methods.
19.3rd Place Solution to Meta AI Video Similarity Challenge
Authors:Shuhei Yokoo, Peifei Zhu, Junki Ishikawa, Rintaro Hasegawa
Abstract: This paper presents our 3rd place solution in both Descriptor Track and Matching Track of the Meta AI Video Similarity Challenge (VSC2022), a competition aimed at detecting video copies. Our approach builds upon existing image copy detection techniques and incorporates several strategies to exploit on the properties of video data, resulting in a simple yet powerful solution. By employing our proposed method, we achieved substantial improvements in accuracy compared to the baseline results (Descriptor Track: 41% improvement, Matching Track: 76% improvement). Our code is publicly available here: https://github.com/line/Meta-AI-Video-Similarity-Challenge-3rd-Place-Solution
20.ICDAR 2023 Competition on Reading the Seal Title
Authors:Wenwen Yu, Mingyu Liu, Mingrui Chen, Ning Lu, Yinlong Wen, Yuliang Liu, Dimosthenis Karatzas, Xiang Bai
Abstract: Reading seal title text is a challenging task due to the variable shapes of seals, curved text, background noise, and overlapped text. However, this important element is commonly found in official and financial scenarios, and has not received the attention it deserves in the field of OCR technology. To promote research in this area, we organized ICDAR 2023 competition on reading the seal title (ReST), which included two tasks: seal title text detection (Task 1) and end-to-end seal title recognition (Task 2). We constructed a dataset of 10,000 real seal data, covering the most common classes of seals, and labeled all seal title texts with text polygons and text contents. The competition opened on 30th December, 2022 and closed on 20th March, 2023. The competition attracted 53 participants from academia and industry including 28 submissions for Task 1 and 25 submissions for Task 2, which demonstrated significant interest in this challenging task. In this report, we present an overview of the competition, including the organization, challenges, and results. We describe the dataset and tasks, and summarize the submissions and evaluation results. The results show that significant progress has been made in the field of seal title text reading, and we hope that this competition will inspire further research and development in this important area of OCR technology.
21.Track Anything: Segment Anything Meets Videos
Authors:Jinyu Yang, Mingqi Gao, Zhe Li, Shang Gao, Fangjing Wang, Feng Zheng
Abstract: Recently, the Segment Anything Model (SAM) gains lots of attention rapidly due to its impressive segmentation performance on images. Regarding its strong ability on image segmentation and high interactivity with different prompts, we found that it performs poorly on consistent segmentation in videos. Therefore, in this report, we propose Track Anything Model (TAM), which achieves high-performance interactive tracking and segmentation in videos. To be detailed, given a video sequence, only with very little human participation, \textit{i.e.}, several clicks, people can track anything they are interested in, and get satisfactory results in one-pass inference. Without additional training, such an interactive design performs impressively on video object tracking and segmentation. All resources are available on \url{https://github.com/gaomingqi/Track-Anything}. We hope this work can facilitate related research.
22.gSDF: Geometry-Driven Signed Distance Functions for 3D Hand-Object Reconstruction
Authors:Zerui Chen, Shizhe Chen, Cordelia Schmid, Ivan Laptev
Abstract: Signed distance functions (SDFs) is an attractive framework that has recently shown promising results for 3D shape reconstruction from images. SDFs seamlessly generalize to different shape resolutions and topologies but lack explicit modelling of the underlying 3D geometry. In this work, we exploit the hand structure and use it as guidance for SDF-based shape reconstruction. In particular, we address reconstruction of hands and manipulated objects from monocular RGB images. To this end, we estimate poses of hands and objects and use them to guide 3D reconstruction. More specifically, we predict kinematic chains of pose transformations and align SDFs with highly-articulated hand poses. We improve the visual features of 3D points with geometry alignment and further leverage temporal information to enhance the robustness to occlusion and motion blurs. We conduct extensive experiments on the challenging ObMan and DexYCB benchmarks and demonstrate significant improvements of the proposed method over the state of the art.
23.MRSN: Multi-Relation Support Network for Video Action Detection
Authors:Yin-Dong Zheng, Guo Chen, Minglei Yuan, Tong Lu
Abstract: Action detection is a challenging video understanding task, requiring modeling spatio-temporal and interaction relations. Current methods usually model actor-actor and actor-context relations separately, ignoring their complementarity and mutual support. To solve this problem, we propose a novel network called Multi-Relation Support Network (MRSN). In MRSN, Actor-Context Relation Encoder (ACRE) and Actor-Actor Relation Encoder (AARE) model the actor-context and actor-actor relation separately. Then Relation Support Encoder (RSE) computes the supports between the two relations and performs relation-level interactions. Finally, Relation Consensus Module (RCM) enhances two relations with the long-term relations from the Long-term Relation Bank (LRB) and yields a consensus. Our experiments demonstrate that modeling relations separately and performing relation-level interactions can achieve and outperformer state-of-the-art results on two challenging video datasets: AVA and UCF101-24.
24.MMC: Multi-Modal Colorization of Images using Textual Descriptions
Authors:Subhankar Ghosh, Prasun Roy, Saumik Bhattacharya, Umapada Pal, Michael Blumenstein
Abstract: Handling various objects with different colors is a significant challenge for image colorization techniques. Thus, for complex real-world scenes, the existing image colorization algorithms often fail to maintain color consistency. In this work, we attempt to integrate textual descriptions as an auxiliary condition, along with the grayscale image that is to be colorized, to improve the fidelity of the colorization process. To do so, we have proposed a deep network that takes two inputs (grayscale image and the respective encoded text description) and tries to predict the relevant color components. Also, we have predicted each object in the image and have colorized them with their individual description to incorporate their specific attributes in the colorization process. After that, a fusion model fuses all the image objects (segments) to generate the final colorized image. As the respective textual descriptions contain color information of the objects present in the image, text encoding helps to improve the overall quality of predicted colors. In terms of performance, the proposed method outperforms existing colorization techniques in terms of LPIPS, PSNR and SSIM metrics.
25.Diabetic Foot Ulcer Grand Challenge 2022 Summary
Authors:Connah Kendrick, Bill Cassidy, Neil D. Reeves, Joseph M. Pappachan, Claire O'Shea, Vishnu Chandrabalan, Moi Hoon Yap
Abstract: The Diabetic Foot Ulcer Challenge 2022 focused on the task of diabetic foot ulcer segmentation, based on the work completed in previous DFU challenges. The challenge provided 4000 images of full-view foot ulcer images together with corresponding delineation of ulcer regions. This paper provides an overview of the challenge, a summary of the methods proposed by the challenge participants, the results obtained from each technique, and a comparison of the challenge results. The best-performing network was a modified HarDNet-MSEG, with a Dice score of 0.7287.
26.D2NT: A High-Performing Depth-to-Normal Translator
Authors:Yi Feng, Bohuan Xue, Ming Liu, Qijun Chen, Rui Fan
Abstract: Surface normal holds significant importance in visual environmental perception, serving as a source of rich geometric information. However, the state-of-the-art (SoTA) surface normal estimators (SNEs) generally suffer from an unsatisfactory trade-off between efficiency and accuracy. To resolve this dilemma, this paper first presents a superfast depth-to-normal translator (D2NT), which can directly translate depth images into surface normal maps without calculating 3D coordinates. We then propose a discontinuity-aware gradient (DAG) filter, which adaptively generates gradient convolution kernels to improve depth gradient estimation. Finally, we propose a surface normal refinement module that can easily be integrated into any depth-to-normal SNEs, substantially improving the surface normal estimation accuracy. Our proposed algorithm demonstrates the best accuracy among all other existing real-time SNEs and achieves the SoTA trade-off between efficiency and accuracy.
27.GRIG: Few-Shot Generative Residual Image Inpainting
Authors:Wanglong Lu, Xianta Jiang, Xiaogang Jin, Yong-Liang Yang, Minglun Gong, Tao Wang, Kaijie Shi, Hanli Zhao
Abstract: Image inpainting is the task of filling in missing or masked region of an image with semantically meaningful contents. Recent methods have shown significant improvement in dealing with large-scale missing regions. However, these methods usually require large training datasets to achieve satisfactory results and there has been limited research into training these models on a small number of samples. To address this, we present a novel few-shot generative residual image inpainting method that produces high-quality inpainting results. The core idea is to propose an iterative residual reasoning method that incorporates Convolutional Neural Networks (CNNs) for feature extraction and Transformers for global reasoning within generative adversarial networks, along with image-level and patch-level discriminators. We also propose a novel forgery-patch adversarial training strategy to create faithful textures and detailed appearances. Extensive evaluations show that our method outperforms previous methods on the few-shot image inpainting task, both quantitatively and qualitatively.
28.MixPro: Data Augmentation with MaskMix and Progressive Attention Labeling for Vision Transformer
Authors:Qihao Zhao, Yangyu Huang, Wei Hu, Fan Zhang, Jun Liu
Abstract: The recently proposed data augmentation TransMix employs attention labels to help visual transformers (ViT) achieve better robustness and performance. However, TransMix is deficient in two aspects: 1) The image cropping method of TransMix may not be suitable for vision transformer. 2) At the early stage of training, the model produces unreliable attention maps. TransMix uses unreliable attention maps to compute mixed attention labels that can affect the model. To address the aforementioned issues, we propose MaskMix and Progressive Attention Labeling (PAL) in image and label space, respectively. In detail, from the perspective of image space, we design MaskMix, which mixes two images based on a patch-like grid mask. In particular, the size of each mask patch is adjustable and is a multiple of the image patch size, which ensures each image patch comes from only one image and contains more global contents. From the perspective of label space, we design PAL, which utilizes a progressive factor to dynamically re-weight the attention weights of the mixed attention label. Finally, we combine MaskMix and Progressive Attention Labeling as our new data augmentation method, named MixPro. The experimental results show that our method can improve various ViT-based models at scales on ImageNet classification (73.8\% top-1 accuracy based on DeiT-T for 300 epochs). After being pre-trained with MixPro on ImageNet, the ViT-based models also demonstrate better transferability to semantic segmentation, object detection, and instance segmentation. Furthermore, compared to TransMix, MixPro also shows stronger robustness on several benchmarks. The code will be released at https://github.com/fistyee/MixPro.
29.VR Facial Animation for Immersive Telepresence Avatars
Authors:Andre Rochow, Max Schwarz, Michael Schreiber, Sven Behnke
Abstract: VR Facial Animation is necessary in applications requiring clear view of the face, even though a VR headset is worn. In our case, we aim to animate the face of an operator who is controlling our robotic avatar system. We propose a real-time capable pipeline with very fast adaptation for specific operators. In a quick enrollment step, we capture a sequence of source images from the operator without the VR headset which contain all the important operator-specific appearance information. During inference, we then use the operator keypoint information extracted from a mouth camera and two eye cameras to estimate the target expression and head pose, to which we map the appearance of a source still image. In order to enhance the mouth expression accuracy, we dynamically select an auxiliary expression frame from the captured sequence. This selection is done by learning to transform the current mouth keypoints into the source camera space, where the alignment can be determined accurately. We, furthermore, demonstrate an eye tracking pipeline that can be trained in less than a minute, a time efficient way to train the whole pipeline given a dataset that includes only complete faces, show exemplary results generated by our method, and discuss performance at the ANA Avatar XPRIZE semifinals.
30.Improving Synthetically Generated Image Detection in Cross-Concept Settings
Authors:Pantelis Dogoulis, Giorgos Kordopatis-Zilos, Ioannis Kompatsiaris, Symeon Papadopoulos
Abstract: New advancements for the detection of synthetic images are critical for fighting disinformation, as the capabilities of generative AI models continuously evolve and can lead to hyper-realistic synthetic imagery at unprecedented scale and speed. In this paper, we focus on the challenge of generalizing across different concept classes, e.g., when training a detector on human faces and testing on synthetic animal images - highlighting the ineffectiveness of existing approaches that randomly sample generated images to train their models. By contrast, we propose an approach based on the premise that the robustness of the detector can be enhanced by training it on realistic synthetic images that are selected based on their quality scores according to a probabilistic quality estimation model. We demonstrate the effectiveness of the proposed approach by conducting experiments with generated images from two seminal architectures, StyleGAN2 and Latent Diffusion, and using three different concepts for each, so as to measure the cross-concept generalization ability. Our results show that our quality-based sampling method leads to higher detection performance for nearly all concepts, improving the overall effectiveness of the synthetic image detectors.
31.Occlusion Robust 3D Human Pose Estimation with StridedPoseGraphFormer and Data Augmentation
Authors:Soubarna Banik, Patricia Gschoßmann, Alejandro Mendoza Garcia, Alois Knoll
Abstract: Occlusion is an omnipresent challenge in 3D human pose estimation (HPE). In spite of the large amount of research dedicated to 3D HPE, only a limited number of studies address the problem of occlusion explicitly. To fill this gap, we propose to combine exploitation of spatio-temporal features with synthetic occlusion augmentation during training to deal with occlusion. To this end, we build a spatio-temporal 3D HPE model, StridedPoseGraphFormer based on graph convolution and transformers, and train it using occlusion augmentation. Unlike the existing occlusion-aware methods, that are only tested for limited occlusion, we extensively evaluate our method for varying degrees of occlusion. We show that our proposed method compares favorably with the state-of-the-art (SoA). Our experimental results also reveal that in the absence of any occlusion handling mechanism, the performance of SoA 3D HPE methods degrades significantly when they encounter occlusion.
32.Augmentation-based Domain Generalization for Semantic Segmentation
Authors:Manuel Schwonberg, Fadoua El Bouazati, Nico M. Schmidt, Hanno Gottschalk
Abstract: Unsupervised Domain Adaptation (UDA) and domain generalization (DG) are two research areas that aim to tackle the lack of generalization of Deep Neural Networks (DNNs) towards unseen domains. While UDA methods have access to unlabeled target images, domain generalization does not involve any target data and only learns generalized features from a source domain. Image-style randomization or augmentation is a popular approach to improve network generalization without access to the target domain. Complex methods are often proposed that disregard the potential of simple image augmentations for out-of-domain generalization. For this reason, we systematically study the in- and out-of-domain generalization capabilities of simple, rule-based image augmentations like blur, noise, color jitter and many more. Based on a full factorial design of experiment design we provide a systematic statistical evaluation of augmentations and their interactions. Our analysis provides both, expected and unexpected, outcomes. Expected, because our experiments confirm the common scientific standard that combination of multiple different augmentations out-performs single augmentations. Unexpected, because combined augmentations perform competitive to state-of-the-art domain generalization approaches, while being significantly simpler and without training overhead. On the challenging synthetic-to-real domain shift between Synthia and Cityscapes we reach 39.5% mIoU compared to 40.9% mIoU of the best previous work. When additionally employing the recent vision transformer architecture DAFormer we outperform these benchmarks with a performance of 44.2% mIoU
33.Exploring shared memory architectures for end-to-end gigapixel deep learning
Authors:Lucas W. Remedios, Leon Y. Cai, Samuel W. Remedios, Karthik Ramadass, Aravind Krishnan, Ruining Deng, Can Cui, Shunxing Bao, Lori A. Coburn, Yuankai Huo, Bennett A. Landman
Abstract: Deep learning has made great strides in medical imaging, enabled by hardware advances in GPUs. One major constraint for the development of new models has been the saturation of GPU memory resources during training. This is especially true in computational pathology, where images regularly contain more than 1 billion pixels. These pathological images are traditionally divided into small patches to enable deep learning due to hardware limitations. In this work, we explore whether the shared GPU/CPU memory architecture on the M1 Ultra systems-on-a-chip (SoCs) recently released by Apple, Inc. may provide a solution. These affordable systems (less than \$5000) provide access to 128 GB of unified memory (Mac Studio with M1 Ultra SoC). As a proof of concept for gigapixel deep learning, we identified tissue from background on gigapixel areas from whole slide images (WSIs). The model was a modified U-Net (4492 parameters) leveraging large kernels and high stride. The M1 Ultra SoC was able to train the model directly on gigapixel images (16000$\times$64000 pixels, 1.024 billion pixels) with a batch size of 1 using over 100 GB of unified memory for the process at an average speed of 1 minute and 21 seconds per batch with Tensorflow 2/Keras. As expected, the model converged with a high Dice score of 0.989 $\pm$ 0.005. Training up until this point took 111 hours and 24 minutes over 4940 steps. Other high RAM GPUs like the NVIDIA A100 (largest commercially accessible at 80 GB, $\sim$\$15000) are not yet widely available (in preview for select regions on Amazon Web Services at \$40.96/hour as a group of 8). This study is a promising step towards WSI-wise end-to-end deep learning with prevalent network architectures.
34.Efficient Halftoning via Deep Reinforcement Learning
Authors:Haitian Jiang, Dongliang Xiong, Xiaowen Jiang, Li Ding, Liang Chen, Kai Huang
Abstract: Halftoning aims to reproduce a continuous-tone image with pixels whose intensities are constrained to two discrete levels. This technique has been deployed on every printer, and the majority of them adopt fast methods (e.g., ordered dithering, error diffusion) that fail to render structural details, which determine halftone's quality. Other prior methods of pursuing visual pleasure by searching for the optimal halftone solution, on the contrary, suffer from their high computational cost. In this paper, we propose a fast and structure-aware halftoning method via a data-driven approach. Specifically, we formulate halftoning as a reinforcement learning problem, in which each binary pixel's value is regarded as an action chosen by a virtual agent with a shared fully convolutional neural network (CNN) policy. In the offline phase, an effective gradient estimator is utilized to train the agents in producing high-quality halftones in one action step. Then, halftones can be generated online by one fast CNN inference. Besides, we propose a novel anisotropy suppressing loss function, which brings the desirable blue-noise property. Finally, we find that optimizing SSIM could result in holes in flat areas, which can be avoided by weighting the metric with the contone's contrast map. Experiments show that our framework can effectively train a light-weight CNN, which is 15x faster than previous structure-aware methods, to generate blue-noise halftones with satisfactory visual quality. We also present a prototype of deep multitoning to demonstrate the extensibility of our method.
35.End-to-End Spatio-Temporal Action Localisation with Video Transformers
Authors:Alexey Gritsenko, Xuehan Xiong, Josip Djolonga, Mostafa Dehghani, Chen Sun, Mario Lučić, Cordelia Schmid, Anurag Arnab
Abstract: The most performant spatio-temporal action localisation models use external person proposals and complex external memory banks. We propose a fully end-to-end, purely-transformer based model that directly ingests an input video, and outputs tubelets -- a sequence of bounding boxes and the action classes at each frame. Our flexible model can be trained with either sparse bounding-box supervision on individual frames, or full tubelet annotations. And in both cases, it predicts coherent tubelets as the output. Moreover, our end-to-end model requires no additional pre-processing in the form of proposals, or post-processing in terms of non-maximal suppression. We perform extensive ablation experiments, and significantly advance the state-of-the-art results on four different spatio-temporal action localisation benchmarks with both sparse keyframes and full tubelet annotations.
36.Meta-tuning Loss Functions and Data Augmentation for Few-shot Object Detection
Authors:Berkan Demirel, Orhun Buğra Baran, Ramazan Gokberk Cinbis
Abstract: Few-shot object detection, the problem of modelling novel object detection categories with few training instances, is an emerging topic in the area of few-shot learning and object detection. Contemporary techniques can be divided into two groups: fine-tuning based and meta-learning based approaches. While meta-learning approaches aim to learn dedicated meta-models for mapping samples to novel class models, fine-tuning approaches tackle few-shot detection in a simpler manner, by adapting the detection model to novel classes through gradient based optimization. Despite their simplicity, fine-tuning based approaches typically yield competitive detection results. Based on this observation, we focus on the role of loss functions and augmentations as the force driving the fine-tuning process, and propose to tune their dynamics through meta-learning principles. The proposed training scheme, therefore, allows learning inductive biases that can boost few-shot detection, while keeping the advantages of fine-tuning based approaches. In addition, the proposed approach yields interpretable loss functions, as opposed to highly parametric and complex few-shot meta-models. The experimental results highlight the merits of the proposed scheme, with significant improvements over the strong fine-tuning based few-shot detection baselines on benchmark Pascal VOC and MS-COCO datasets, in terms of both standard and generalized few-shot performance metrics.
37.Multi-crop Contrastive Learning for Unsupervised Image-to-Image Translation
Authors:Chen Zhao, Wei-Ling Cai, Zheng Yuan, Cheng-Wei Hu
Abstract: Recently, image-to-image translation methods based on contrastive learning achieved state-of-the-art results in many tasks. However, the negatives are sampled from the input feature spaces in the previous work, which makes the negatives lack diversity. Moreover, in the latent space of the embedings,the previous methods ignore domain consistency between the generated image and the real images of target domain. In this paper, we propose a novel contrastive learning framework for unpaired image-to-image translation, called MCCUT. We utilize the multi-crop views to generate the negatives via the center-crop and the random-crop, which can improve the diversity of negatives and meanwhile increase the quality of negatives. To constrain the embedings in the deep feature space,, we formulate a new domain consistency loss function, which encourages the generated images to be close to the real images in the embedding space of same domain. Furthermore, we present a dual coordinate channel attention network by embedding positional information into SENet, which called DCSE module. We employ the DCSE module in the design of generator, which makes the generator pays more attention to channels with greater weight. In many image-to-image translation tasks, our method achieves state-of-the-art results, and the advantages of our method have been proved through extensive comparison experiments and ablation research.
38.HOSNeRF: Dynamic Human-Object-Scene Neural Radiance Fields from a Single Video
Authors:Jia-Wei Liu, Yan-Pei Cao, Tianyuan Yang, Eric Zhongcong Xu, Jussi Keppo, Ying Shan, Xiaohu Qie, Mike Zheng Shou
Abstract: We introduce HOSNeRF, a novel 360{\deg} free-viewpoint rendering method that reconstructs neural radiance fields for dynamic human-object-scene from a single monocular in-the-wild video. Our method enables pausing the video at any frame and rendering all scene details (dynamic humans, objects, and backgrounds) from arbitrary viewpoints. The first challenge in this task is the complex object motions in human-object interactions, which we tackle by introducing the new object bones into the conventional human skeleton hierarchy to effectively estimate large object deformations in our dynamic human-object model. The second challenge is that humans interact with different objects at different times, for which we introduce two new learnable object state embeddings that can be used as conditions for learning our human-object representation and scene representation, respectively. Extensive experiments show that HOSNeRF significantly outperforms SOTA approaches on two challenging datasets by a large margin of 40% ~ 50% in terms of LPIPS. The code, data, and compelling examples of 360{\deg} free-viewpoint renderings from single videos will be released in https://showlab.github.io/HOSNeRF.
39.Moving Forward by Moving Backward: Embedding Action Impact over Action Semantics
Authors:Kuo-Hao Zeng, Luca Weihs, Roozbeh Mottaghi, Ali Farhadi
Abstract: A common assumption when training embodied agents is that the impact of taking an action is stable; for instance, executing the "move ahead" action will always move the agent forward by a fixed distance, perhaps with some small amount of actuator-induced noise. This assumption is limiting; an agent may encounter settings that dramatically alter the impact of actions: a move ahead action on a wet floor may send the agent twice as far as it expects and using the same action with a broken wheel might transform the expected translation into a rotation. Instead of relying that the impact of an action stably reflects its pre-defined semantic meaning, we propose to model the impact of actions on-the-fly using latent embeddings. By combining these latent action embeddings with a novel, transformer-based, policy head, we design an Action Adaptive Policy (AAP). We evaluate our AAP on two challenging visual navigation tasks in the AI2-THOR and Habitat environments and show that our AAP is highly performant even when faced, at inference-time with missing actions and, previously unseen, perturbed action space. Moreover, we observe significant improvement in robustness against these actions when evaluating in real-world scenarios.
40.Explicit Correspondence Matching for Generalizable Neural Radiance Fields
Authors:Yuedong Chen, Haofei Xu, Qianyi Wu, Chuanxia Zheng, Tat-Jen Cham, Jianfei Cai
Abstract: We present a new generalizable NeRF method that is able to directly generalize to new unseen scenarios and perform novel view synthesis with as few as two source views. The key to our approach lies in the explicitly modeled correspondence matching information, so as to provide the geometry prior to the prediction of NeRF color and density for volume rendering. The explicit correspondence matching is quantified with the cosine similarity between image features sampled at the 2D projections of a 3D point on different views, which is able to provide reliable cues about the surface geometry. Unlike previous methods where image features are extracted independently for each view, we consider modeling the cross-view interactions via Transformer cross-attention, which greatly improves the feature matching quality. Our method achieves state-of-the-art results on different evaluation settings, with the experiments showing a strong correlation between our learned cosine feature similarity and volume density, demonstrating the effectiveness and superiority of our proposed method. Code is at https://github.com/donydchen/matchnerf
41.Large-capacity and Flexible Video Steganography via Invertible Neural Network
Authors:Chong Mou, Youmin Xu, Jiechong Song, Chen Zhao, Bernard Ghanem, Jian Zhang
Abstract: Video steganography is the art of unobtrusively concealing secret data in a cover video and then recovering the secret data through a decoding protocol at the receiver end. Although several attempts have been made, most of them are limited to low-capacity and fixed steganography. To rectify these weaknesses, we propose a Large-capacity and Flexible Video Steganography Network (LF-VSN) in this paper. For large-capacity, we present a reversible pipeline to perform multiple videos hiding and recovering through a single invertible neural network (INN). Our method can hide/recover 7 secret videos in/from 1 cover video with promising performance. For flexibility, we propose a key-controllable scheme, enabling different receivers to recover particular secret videos from the same cover video through specific keys. Moreover, we further improve the flexibility by proposing a scalable strategy in multiple videos hiding, which can hide variable numbers of secret videos in a cover video with a single model and a single training session. Extensive experiments demonstrate that with the significant improvement of the video steganography performance, our proposed LF-VSN has high security, large hiding capacity, and flexibility. The source code is available at https://github.com/MC-E/LF-VSN.
42.AssemblyHands: Towards Egocentric Activity Understanding via 3D Hand Pose Estimation
Authors:Takehiko Ohkawa, Kun He, Fadime Sener, Tomas Hodan, Luan Tran, Cem Keskin
Abstract: We present AssemblyHands, a large-scale benchmark dataset with accurate 3D hand pose annotations, to facilitate the study of egocentric activities with challenging hand-object interactions. The dataset includes synchronized egocentric and exocentric images sampled from the recent Assembly101 dataset, in which participants assemble and disassemble take-apart toys. To obtain high-quality 3D hand pose annotations for the egocentric images, we develop an efficient pipeline, where we use an initial set of manual annotations to train a model to automatically annotate a much larger dataset. Our annotation model uses multi-view feature fusion and an iterative refinement scheme, and achieves an average keypoint error of 4.20 mm, which is 85% lower than the error of the original annotations in Assembly101. AssemblyHands provides 3.0M annotated images, including 490K egocentric images, making it the largest existing benchmark dataset for egocentric 3D hand pose estimation. Using this data, we develop a strong single-view baseline of 3D hand pose estimation from egocentric images. Furthermore, we design a novel action classification task to evaluate predicted 3D hand poses. Our study shows that having higher-quality hand poses directly improves the ability to recognize actions.
43.Segment Anything in 3D with NeRFs
Authors:Jiazhong Cen, Zanwei Zhou, Jiemin Fang, Wei Shen, Lingxi Xie, Xiaopeng Zhang, Qi Tian
Abstract: The Segment Anything Model (SAM) has demonstrated its effectiveness in segmenting any object/part in various 2D images, yet its ability for 3D has not been fully explored. The real world is composed of numerous 3D scenes and objects. Due to the scarcity of accessible 3D data and high cost of its acquisition and annotation, lifting SAM to 3D is a challenging but valuable research avenue. With this in mind, we propose a novel framework to Segment Anything in 3D, named SA3D. Given a neural radiance field (NeRF) model, SA3D allows users to obtain the 3D segmentation result of any target object via only one-shot manual prompting in a single rendered view. With input prompts, SAM cuts out the target object from the according view. The obtained 2D segmentation mask is projected onto 3D mask grids via density-guided inverse rendering. 2D masks from other views are then rendered, which are mostly uncompleted but used as cross-view self-prompts to be fed into SAM again. Complete masks can be obtained and projected onto mask grids. This procedure is executed via an iterative manner while accurate 3D masks can be finally learned. SA3D can adapt to various radiance fields effectively without any additional redesigning. The entire segmentation process can be completed in approximately two minutes without any engineering optimization. Our experiments demonstrate the effectiveness of SA3D in different scenes, highlighting the potential of SAM in 3D scene perception. The project page is at https://jumpat.github.io/SA3D/.
44.Fully Sparse Fusion for 3D Object Detection
Authors:Yingyan Li, Lue Fan, Yang Liu, Zehao Huang, Yuntao Chen, Naiyan Wang, Zhaoxiang Zhang, Tieniu Tan
Abstract: Currently prevalent multimodal 3D detection methods are built upon LiDAR-based detectors that usually use dense Bird's-Eye-View (BEV) feature maps. However, the cost of such BEV feature maps is quadratic to the detection range, making it not suitable for long-range detection. Fully sparse architecture is gaining attention as they are highly efficient in long-range perception. In this paper, we study how to effectively leverage image modality in the emerging fully sparse architecture. Particularly, utilizing instance queries, our framework integrates the well-studied 2D instance segmentation into the LiDAR side, which is parallel to the 3D instance segmentation part in the fully sparse detector. This design achieves a uniform query-based fusion framework in both the 2D and 3D sides while maintaining the fully sparse characteristic. Extensive experiments showcase state-of-the-art results on the widely used nuScenes dataset and the long-range Argoverse 2 dataset. Notably, the inference speed of the proposed method under the long-range LiDAR perception setting is 2.7 $\times$ faster than that of other state-of-the-art multimodal 3D detection methods. Code will be released at \url{https://github.com/BraveGroup/FullySparseFusion}.
45.Distilling from Similar Tasks for Transfer Learning on a Budget
Authors:Kenneth Borup, Cheng Perng Phoo, Bharath Hariharan
Abstract: We address the challenge of getting efficient yet accurate recognition systems with limited labels. While recognition models improve with model size and amount of data, many specialized applications of computer vision have severe resource constraints both during training and inference. Transfer learning is an effective solution for training with few labels, however often at the expense of a computationally costly fine-tuning of large base models. We propose to mitigate this unpleasant trade-off between compute and accuracy via semi-supervised cross-domain distillation from a set of diverse source models. Initially, we show how to use task similarity metrics to select a single suitable source model to distill from, and that a good selection process is imperative for good downstream performance of a target model. We dub this approach DistillNearest. Though effective, DistillNearest assumes a single source model matches the target task, which is not always the case. To alleviate this, we propose a weighted multi-source distillation method to distill multiple source models trained on different domains weighted by their relevance for the target task into a single efficient model (named DistillWeighted). Our methods need no access to source data, and merely need features and pseudo-labels of the source models. When the goal is accurate recognition under computational constraints, both DistillNearest and DistillWeighted approaches outperform both transfer learning from strong ImageNet initializations as well as state-of-the-art semi-supervised techniques such as FixMatch. Averaged over 8 diverse target tasks our multi-source method outperforms the baselines by 5.6%-points and 4.5%-points, respectively.
46.Once Detected, Never Lost: Surpassing Human Performance in Offline LiDAR based 3D Object Detection
Authors:Lue Fan, Yuxue Yang, Yiming Mao, Feng Wang, Yuntao Chen, Naiyan Wang, Zhaoxiang Zhang
Abstract: This paper aims for high-performance offline LiDAR-based 3D object detection. We first observe that experienced human annotators annotate objects from a track-centric perspective. They first label the objects with clear shapes in a track, and then leverage the temporal coherence to infer the annotations of obscure objects. Drawing inspiration from this, we propose a high-performance offline detector in a track-centric perspective instead of the conventional object-centric perspective. Our method features a bidirectional tracking module and a track-centric learning module. Such a design allows our detector to infer and refine a complete track once the object is detected at a certain moment. We refer to this characteristic as "onCe detecTed, neveR Lost" and name the proposed system CTRL. Extensive experiments demonstrate the remarkable performance of our method, surpassing the human-level annotating accuracy and the previous state-of-the-art methods in the highly competitive Waymo Open Dataset without model ensemble. The code will be made publicly available at https://github.com/tusen-ai/SST.
47.Total-Recon: Deformable Scene Reconstruction for Embodied View Synthesis
Authors:Chonghyuk Song, Gengshan Yang, Kangle Deng, Jun-Yan Zhu, Deva Ramanan
Abstract: We explore the task of embodied view synthesis from monocular videos of deformable scenes. Given a minute-long RGBD video of people interacting with their pets, we render the scene from novel camera trajectories derived from in-scene motion of actors: (1) egocentric cameras that simulate the point of view of a target actor and (2) 3rd-person cameras that follow the actor. Building such a system requires reconstructing the root-body and articulated motion of each actor in the scene, as well as a scene representation that supports free-viewpoint synthesis. Longer videos are more likely to capture the scene from diverse viewpoints (which helps reconstruction) but are also more likely to contain larger motions (which complicates reconstruction). To address these challenges, we present Total-Recon, the first method to photorealistically reconstruct deformable scenes from long monocular RGBD videos. Crucially, to scale to long videos, our method hierarchically decomposes the scene motion into the motion of each object, which itself is decomposed into global root-body motion and local articulations. To quantify such "in-the-wild" reconstruction and view synthesis, we collect ground-truth data from a specialized stereo RGBD capture rig for 11 challenging videos, significantly outperforming prior art. Code, videos, and data can be found at https://andrewsonga.github.io/totalrecon .