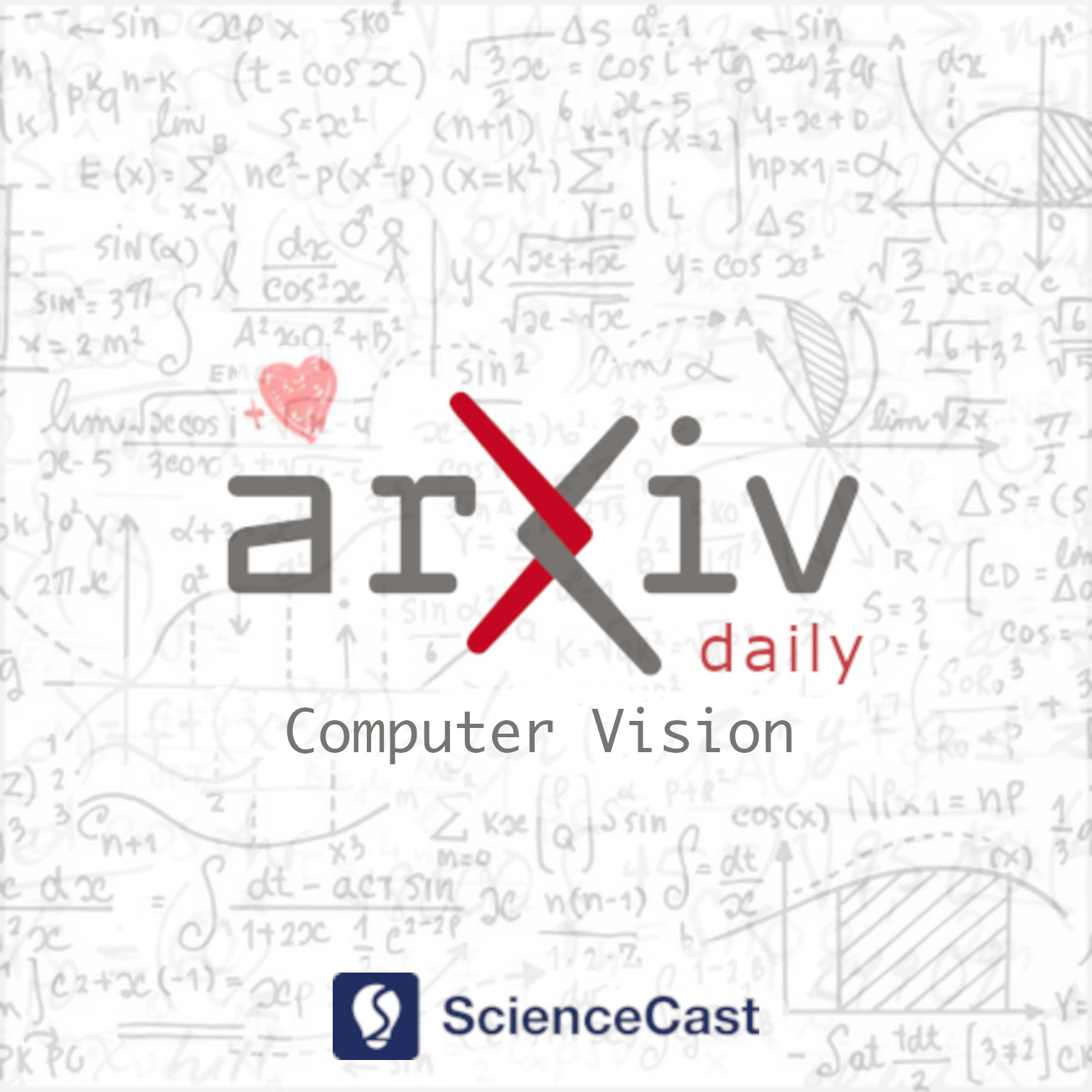
Computer Vision and Pattern Recognition (cs.CV)
Wed, 05 Jul 2023
1.Muti-scale Graph Neural Network with Signed-attention for Social Bot Detection: A Frequency Perspective
Authors:Shuhao Shi, Kai Qiao, Zhengyan Wang, Jie Yang, Baojie Song, Jian Chen, Bin Yan
Abstract: The presence of a large number of bots on social media has adverse effects. The graph neural network (GNN) can effectively leverage the social relationships between users and achieve excellent results in detecting bots. Recently, more and more GNN-based methods have been proposed for bot detection. However, the existing GNN-based bot detection methods only focus on low-frequency information and seldom consider high-frequency information, which limits the representation ability of the model. To address this issue, this paper proposes a Multi-scale with Signed-attention Graph Filter for social bot detection called MSGS. MSGS could effectively utilize both high and low-frequency information in the social graph. Specifically, MSGS utilizes a multi-scale structure to produce representation vectors at different scales. These representations are then combined using a signed-attention mechanism. Finally, multi-scale representations via MLP after polymerization to produce the final result. We analyze the frequency response and demonstrate that MSGS is a more flexible and expressive adaptive graph filter. MSGS can effectively utilize high-frequency information to alleviate the over-smoothing problem of deep GNNs. Experimental results on real-world datasets demonstrate that our method achieves better performance compared with several state-of-the-art social bot detection methods.
2.Multimodal Prompt Learning for Product Title Generation with Extremely Limited Labels
Authors:Bang Yang, Fenglin Liu, Zheng Li, Qingyu Yin, Chenyu You, Bing Yin, Yuexian Zou
Abstract: Generating an informative and attractive title for the product is a crucial task for e-commerce. Most existing works follow the standard multimodal natural language generation approaches, e.g., image captioning, and employ the large scale of human-labelled datasets to train desirable models. However, for novel products, especially in a different domain, there are few existing labelled data. In this paper, we propose a prompt-based approach, i.e., the Multimodal Prompt Learning framework, to accurately and efficiently generate titles for novel products with limited labels. We observe that the core challenges of novel product title generation are the understanding of novel product characteristics and the generation of titles in a novel writing style. To this end, we build a set of multimodal prompts from different modalities to preserve the corresponding characteristics and writing styles of novel products. As a result, with extremely limited labels for training, the proposed method can retrieve the multimodal prompts to generate desirable titles for novel products. The experiments and analyses are conducted on five novel product categories under both the in-domain and out-of-domain experimental settings. The results show that, with only 1% of downstream labelled data for training, our proposed approach achieves the best few-shot results and even achieves competitive results with fully-supervised methods trained on 100% of training data; With the full labelled data for training, our method achieves state-of-the-art results.
3.The KiTS21 Challenge: Automatic segmentation of kidneys, renal tumors, and renal cysts in corticomedullary-phase CT
Authors:Nicholas Heller, Fabian Isensee, Dasha Trofimova, Resha Tejpaul, Zhongchen Zhao, Huai Chen, Lisheng Wang, Alex Golts, Daniel Khapun, Daniel Shats, Yoel Shoshan, Flora Gilboa-Solomon, Yasmeen George, Xi Yang, Jianpeng Zhang, Jing Zhang, Yong Xia, Mengran Wu, Zhiyang Liu, Ed Walczak, Sean McSweeney, Ranveer Vasdev, Chris Hornung, Rafat Solaiman, Jamee Schoephoerster, Bailey Abernathy, David Wu, Safa Abdulkadir, Ben Byun, Justice Spriggs, Griffin Struyk, Alexandra Austin, Ben Simpson, Michael Hagstrom, Sierra Virnig, John French, Nitin Venkatesh, Sarah Chan, Keenan Moore, Anna Jacobsen, Susan Austin, Mark Austin, Subodh Regmi, Nikolaos Papanikolopoulos, Christopher Weight
Abstract: This paper presents the challenge report for the 2021 Kidney and Kidney Tumor Segmentation Challenge (KiTS21) held in conjunction with the 2021 international conference on Medical Image Computing and Computer Assisted Interventions (MICCAI). KiTS21 is a sequel to its first edition in 2019, and it features a variety of innovations in how the challenge was designed, in addition to a larger dataset. A novel annotation method was used to collect three separate annotations for each region of interest, and these annotations were performed in a fully transparent setting using a web-based annotation tool. Further, the KiTS21 test set was collected from an outside institution, challenging participants to develop methods that generalize well to new populations. Nonetheless, the top-performing teams achieved a significant improvement over the state of the art set in 2019, and this performance is shown to inch ever closer to human-level performance. An in-depth meta-analysis is presented describing which methods were used and how they faired on the leaderboard, as well as the characteristics of which cases generally saw good performance, and which did not. Overall KiTS21 facilitated a significant advancement in the state of the art in kidney tumor segmentation, and provides useful insights that are applicable to the field of semantic segmentation as a whole.
4.Task-Specific Alignment and Multiple Level Transformer for Few-Shot Action Recognition
Authors:Fei Guo, Li Zhu, YiWang Wang
Abstract: In the research field of few-shot learning, the main difference between image-based and video-based is the additional temporal dimension for videos. In recent years, many approaches for few-shot action recognition have followed the metric-based methods, especially, since some works use the Transformer to get the cross-attention feature of the videos or the enhanced prototype, and the results are competitive. However, they do not mine enough information from the Transformer because they only focus on the feature of a single level. In our paper, we have addressed this problem. We propose an end-to-end method named "Task-Specific Alignment and Multiple Level Transformer Network (TSA-MLT)". In our model, the Multiple Level Transformer focuses on the multiple-level feature of the support video and query video. Especially before Multiple Level Transformer, we use task-specific TSA to filter unimportant or misleading frames as a pre-processing. Furthermore, we adopt a fusion loss using two kinds of distance, the first is L2 sequence distance, which focuses on temporal order alignment. The second one is Optimal transport distance, which focuses on measuring the gap between the appearance and semantics of the videos. Using a simple fusion network, we fuse the two distances element-wise, then use the cross-entropy loss as our fusion loss. Extensive experiments show our method achieves state-of-the-art results on the HMDB51 and UCF101 datasets and a competitive result on the benchmark of Kinetics and something-2-something V2 datasets. Our code will be available at the URL: https://github.com/cofly2014/tsa-mlt.git
5.Multi-Modal Prototypes for Open-Set Semantic Segmentation
Authors:Yuhuan Yang, Chaofan Ma, Chen Ju, Ya Zhang, Yanfeng Wang
Abstract: In semantic segmentation, adapting a visual system to novel object categories at inference time has always been both valuable and challenging. To enable such generalization, existing methods rely on either providing several support examples as visual cues or class names as textual cues. Through the development is relatively optimistic, these two lines have been studied in isolation, neglecting the complementary intrinsic of low-level visual and high-level language information. In this paper, we define a unified setting termed as open-set semantic segmentation (O3S), which aims to learn seen and unseen semantics from both visual examples and textual names. Our pipeline extracts multi-modal prototypes for segmentation task, by first single modal self-enhancement and aggregation, then multi-modal complementary fusion. To be specific, we aggregate visual features into several tokens as visual prototypes, and enhance the class name with detailed descriptions for textual prototype generation. The two modalities are then fused to generate multi-modal prototypes for final segmentation. On both \pascal and \coco datasets, we conduct extensive experiments to evaluate the framework effectiveness. State-of-the-art results are achieved even on more detailed part-segmentation, Pascal-Animals, by only training on coarse-grained datasets. Thorough ablation studies are performed to dissect each component, both quantitatively and qualitatively.
6.Remote Sensing Image Change Detection with Graph Interaction
Authors:Chenglong Liu
Abstract: Modern remote sensing image change detection has witnessed substantial advancements by harnessing the potent feature extraction capabilities of CNNs and Transforms.Yet,prevailing change detection techniques consistently prioritize extracting semantic features related to significant alterations,overlooking the viability of directly interacting with bitemporal image features.In this letter,we propose a bitemporal image graph Interaction network for remote sensing change detection,namely BGINet-CD. More specifically,by leveraging the concept of non-local operations and mapping the features obtained from the backbone network to the graph structure space,we propose a unified self-focus mechanism for bitemporal images.This approach enhances the information coupling between the two temporal images while effectively suppressing task-irrelevant interference,Based on a streamlined backbone architecture,namely ResNet18,our model demonstrates superior performance compared to other state-of-the-art methods (SOTA) on the GZ CD dataset. Moreover,the model exhibits an enhanced trade-off between accuracy and computational efficiency,further improving its overall effectiveness
7.ZJU ReLER Submission for EPIC-KITCHEN Challenge 2023: Semi-Supervised Video Object Segmentation
Authors:Jiahao Li, Yuanyou Xu, Zongxin Yang, Yi Yang, Yueting Zhuang
Abstract: The Associating Objects with Transformers (AOT) framework has exhibited exceptional performance in a wide range of complex scenarios for video object segmentation. In this study, we introduce MSDeAOT, a variant of the AOT series that incorporates transformers at multiple feature scales. Leveraging the hierarchical Gated Propagation Module (GPM), MSDeAOT efficiently propagates object masks from previous frames to the current frame using a feature scale with a stride of 16. Additionally, we employ GPM in a more refined feature scale with a stride of 8, leading to improved accuracy in detecting and tracking small objects. Through the implementation of test-time augmentations and model ensemble techniques, we achieve the top-ranking position in the EPIC-KITCHEN VISOR Semi-supervised Video Object Segmentation Challenge.
8.ZJU ReLER Submission for EPIC-KITCHEN Challenge 2023: TREK-150 Single Object Tracking
Authors:Yuanyou Xu, Jiahao Li, Zongxin Yang, Yi Yang, Yueting Zhuang
Abstract: The Associating Objects with Transformers (AOT) framework has exhibited exceptional performance in a wide range of complex scenarios for video object tracking and segmentation. In this study, we convert the bounding boxes to masks in reference frames with the help of the Segment Anything Model (SAM) and Alpha-Refine, and then propagate the masks to the current frame, transforming the task from Video Object Tracking (VOT) to video object segmentation (VOS). Furthermore, we introduce MSDeAOT, a variant of the AOT series that incorporates transformers at multiple feature scales. MSDeAOT efficiently propagates object masks from previous frames to the current frame using two feature scales of 16 and 8. As a testament to the effectiveness of our design, we achieved the 1st place in the EPIC-KITCHENS TREK-150 Object Tracking Challenge.
9.Generative Adversarial Networks for Dental Patient Identity Protection in Orthodontic Educational Imaging
Authors:Mingchuan Tian, Wilson Weixun Lu, Kelvin Weng Chiong Foong, Eugene Loh
Abstract: Objectives: This research introduces a novel area-preserving Generative Adversarial Networks (GAN) inversion technique for effectively de-identifying dental patient images. This innovative method addresses privacy concerns while preserving key dental features, thereby generating valuable resources for dental education and research. Methods: We enhanced the existing GAN Inversion methodology to maximize the preservation of dental characteristics within the synthesized images. A comprehensive technical framework incorporating several deep learning models was developed to provide end-to-end development guidance and practical application for image de-identification. Results: Our approach was assessed with varied facial pictures, extensively used for diagnosing skeletal asymmetry and facial anomalies. Results demonstrated our model's ability to adapt the context from one image to another, maintaining compatibility, while preserving dental features essential for oral diagnosis and dental education. A panel of five clinicians conducted an evaluation on a set of original and GAN-processed images. The generated images achieved effective de-identification, maintaining the realism of important dental features and were deemed useful for dental diagnostics and education. Clinical Significance: Our GAN model and the encompassing framework can streamline the de-identification process of dental patient images, enhancing efficiency in dental education. This method improves students' diagnostic capabilities by offering more exposure to orthodontic malocclusions. Furthermore, it facilitates the creation of de-identified datasets for broader 2D image research at major research institutions.