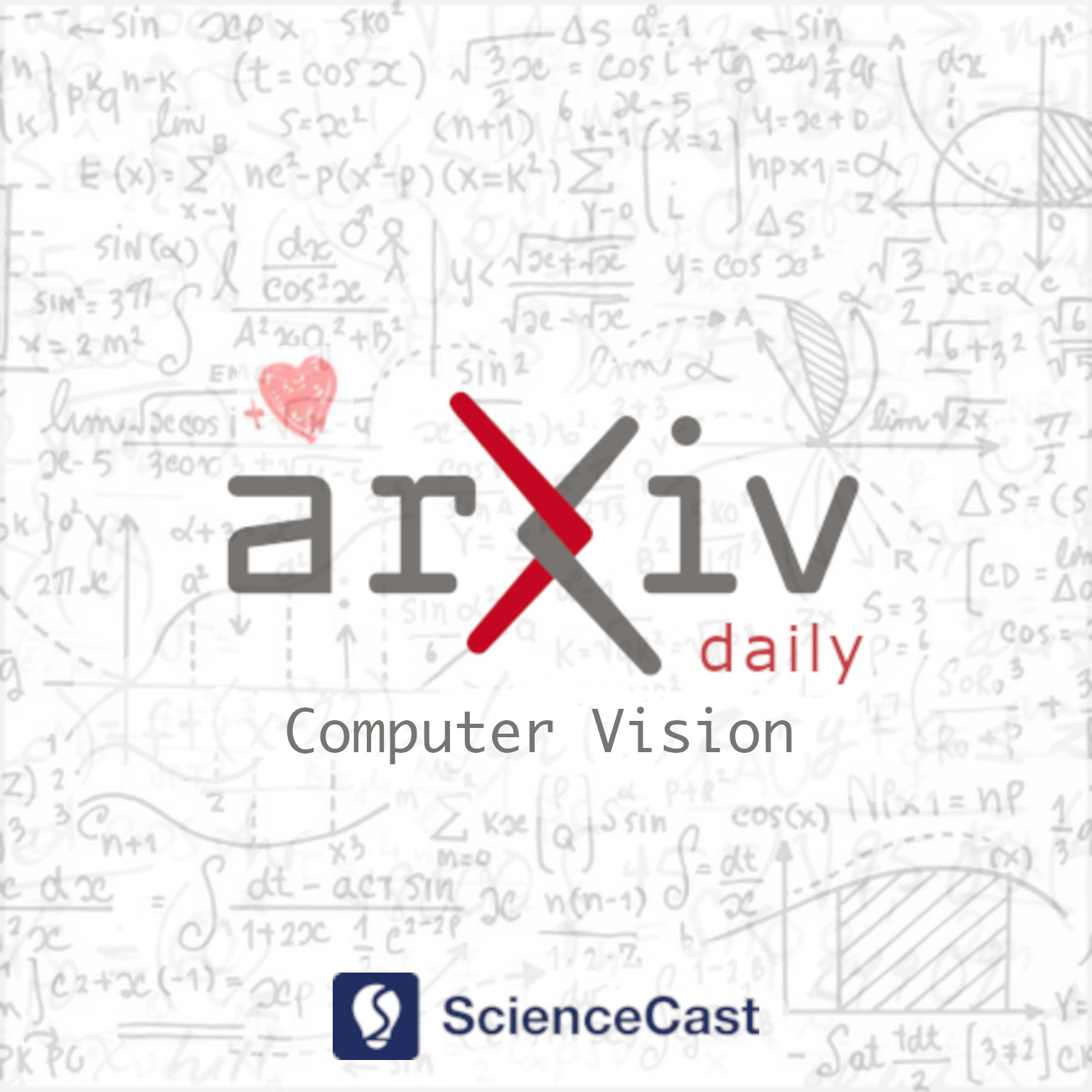
Computer Vision and Pattern Recognition (cs.CV)
Wed, 12 Jul 2023
1.Reading Radiology Imaging Like The Radiologist
Authors:Yuhao Wang
Abstract: Automated radiology report generation aims to generate radiology reports that contain rich, fine-grained descriptions of radiology imaging. Compared with image captioning in the natural image domain, medical images are very similar to each other, with only minor differences in the occurrence of diseases. Given the importance of these minor differences in the radiology report, it is crucial to encourage the model to focus more on the subtle regions of disease occurrence. Secondly, the problem of visual and textual data biases is serious. Not only do normal cases make up the majority of the dataset, but sentences describing areas with pathological changes also constitute only a small part of the paragraph. Lastly, generating medical image reports involves the challenge of long text generation, which requires more expertise and empirical training in medical knowledge. As a result, the difficulty of generating such reports is increased. To address these challenges, we propose a disease-oriented retrieval framework that utilizes similar reports as prior knowledge references. We design a factual consistency captioning generator to generate more accurate and factually consistent disease descriptions. Our framework can find most similar reports for a given disease from the CXR database by retrieving a disease-oriented mask consisting of the position and morphological characteristics. By referencing the disease-oriented similar report and the visual features, the factual consistency model can generate a more accurate radiology report.
2.A New Dataset and Comparative Study for Aphid Cluster Detection
Authors:Tianxiao Zhang, Kaidong Li, Xiangyu Chen, Cuncong Zhong, Bo Luo, Ivan Grijalva Teran, Brian McCornack, Daniel Flippo, Ajay Sharda, Guanghui Wang
Abstract: Aphids are one of the main threats to crops, rural families, and global food security. Chemical pest control is a necessary component of crop production for maximizing yields, however, it is unnecessary to apply the chemical approaches to the entire fields in consideration of the environmental pollution and the cost. Thus, accurately localizing the aphid and estimating the infestation level is crucial to the precise local application of pesticides. Aphid detection is very challenging as each individual aphid is really small and all aphids are crowded together as clusters. In this paper, we propose to estimate the infection level by detecting aphid clusters. We have taken millions of images in the sorghum fields, manually selected 5,447 images that contain aphids, and annotated each aphid cluster in the image. To use these images for machine learning models, we crop the images into patches and created a labeled dataset with over 151,000 image patches. Then, we implement and compare the performance of four state-of-the-art object detection models.
3.Sem-CS: Semantic CLIPStyler for Text-Based Image Style Transfer
Authors:Chanda Grover Kamra, Indra Deep Mastan, Debayan Gupta
Abstract: CLIPStyler demonstrated image style transfer with realistic textures using only a style text description (instead of requiring a reference style image). However, the ground semantics of objects in the style transfer output is lost due to style spill-over on salient and background objects (content mismatch) or over-stylization. To solve this, we propose Semantic CLIPStyler (Sem-CS), that performs semantic style transfer. Sem-CS first segments the content image into salient and non-salient objects and then transfers artistic style based on a given style text description. The semantic style transfer is achieved using global foreground loss (for salient objects) and global background loss (for non-salient objects). Our empirical results, including DISTS, NIMA and user study scores, show that our proposed framework yields superior qualitative and quantitative performance. Our code is available at github.com/chandagrover/sem-cs.
4.YOGA: Deep Object Detection in the Wild with Lightweight Feature Learning and Multiscale Attention
Authors:Raja Sunkara, Tie Luo
Abstract: We introduce YOGA, a deep learning based yet lightweight object detection model that can operate on low-end edge devices while still achieving competitive accuracy. The YOGA architecture consists of a two-phase feature learning pipeline with a cheap linear transformation, which learns feature maps using only half of the convolution filters required by conventional convolutional neural networks. In addition, it performs multi-scale feature fusion in its neck using an attention mechanism instead of the naive concatenation used by conventional detectors. YOGA is a flexible model that can be easily scaled up or down by several orders of magnitude to fit a broad range of hardware constraints. We evaluate YOGA on COCO-val and COCO-testdev datasets with other over 10 state-of-the-art object detectors. The results show that YOGA strikes the best trade-off between model size and accuracy (up to 22% increase of AP and 23-34% reduction of parameters and FLOPs), making it an ideal choice for deployment in the wild on low-end edge devices. This is further affirmed by our hardware implementation and evaluation on NVIDIA Jetson Nano.
5.Towards Safe Self-Distillation of Internet-Scale Text-to-Image Diffusion Models
Authors:Sanghyun Kim, Seohyeon Jung, Balhae Kim, Moonseok Choi, Jinwoo Shin, Juho Lee
Abstract: Large-scale image generation models, with impressive quality made possible by the vast amount of data available on the Internet, raise social concerns that these models may generate harmful or copyrighted content. The biases and harmfulness arise throughout the entire training process and are hard to completely remove, which have become significant hurdles to the safe deployment of these models. In this paper, we propose a method called SDD to prevent problematic content generation in text-to-image diffusion models. We self-distill the diffusion model to guide the noise estimate conditioned on the target removal concept to match the unconditional one. Compared to the previous methods, our method eliminates a much greater proportion of harmful content from the generated images without degrading the overall image quality. Furthermore, our method allows the removal of multiple concepts at once, whereas previous works are limited to removing a single concept at a time.
6.Flexible and Fully Quantized Ultra-Lightweight TinyissimoYOLO for Ultra-Low-Power Edge Systems
Authors:Julian Moosmann, Hanna Mueller, Nicky Zimmerman, Georg Rutishauser, Luca Benini, Michele Magno
Abstract: This paper deploys and explores variants of TinyissimoYOLO, a highly flexible and fully quantized ultra-lightweight object detection network designed for edge systems with a power envelope of a few milliwatts. With experimental measurements, we present a comprehensive characterization of the network's detection performance, exploring the impact of various parameters, including input resolution, number of object classes, and hidden layer adjustments. We deploy variants of TinyissimoYOLO on state-of-the-art ultra-low-power extreme edge platforms, presenting an in-depth a comparison on latency, energy efficiency, and their ability to efficiently parallelize the workload. In particular, the paper presents a comparison between a novel parallel RISC-V processor (GAP9 from Greenwaves) with and without use of its on-chip hardware accelerator, an ARM Cortex-M7 core (STM32H7 from ST Microelectronics), two ARM Cortex-M4 cores (STM32L4 from STM and Apollo4b from Ambiq), and a multi-core platform with a CNN hardware accelerator (Analog Devices MAX78000). Experimental results show that the GAP9's hardware accelerator achieves the lowest inference latency and energy at 2.12ms and 150uJ respectively, which is around 2x faster and 20% more efficient than the next best platform, the MAX78000. The hardware accelerator of GAP9 can even run an increased resolution version of TinyissimoYOLO with 112x112 pixels and 10 detection classes within 3.2ms, consuming 245uJ. To showcase the competitiveness of a versatile general-purpose system we also deployed and profiled a multi-core implementation on GAP9 at different operating points, achieving 11.3ms with the lowest-latency and 490uJ with the most energy-efficient configuration. With this paper, we demonstrate the suitability and flexibility of TinyissimoYOLO on state-of-the-art detection datasets for real-time ultra-low-power edge inference.
7.Unsupervised Optical Flow Estimation with Dynamic Timing Representation for Spike Camera
Authors:Lujie Xia, Ziluo Ding, Rui Zhao, Jiyuan Zhang, Lei Ma, Zhaofei Yu, Tiejun Huang, Ruiqin Xiong
Abstract: Efficiently selecting an appropriate spike stream data length to extract precise information is the key to the spike vision tasks. To address this issue, we propose a dynamic timing representation for spike streams. Based on multi-layers architecture, it applies dilated convolutions on temporal dimension to extract features on multi-temporal scales with few parameters. And we design layer attention to dynamically fuse these features. Moreover, we propose an unsupervised learning method for optical flow estimation in a spike-based manner to break the dependence on labeled data. In addition, to verify the robustness, we also build a spike-based synthetic validation dataset for extreme scenarios in autonomous driving, denoted as SSES dataset. It consists of various corner cases. Experiments show that our method can predict optical flow from spike streams in different high-speed scenes, including real scenes. For instance, our method gets $15\%$ and $19\%$ error reduction from the best spike-based work, SCFlow, in $\Delta t=10$ and $\Delta t=20$ respectively which are the same settings as the previous works.
8.What Happens During Finetuning of Vision Transformers: An Invariance Based Investigation
Authors:Gabriele Merlin, Vedant Nanda, Ruchit Rawal, Mariya Toneva
Abstract: The pretrain-finetune paradigm usually improves downstream performance over training a model from scratch on the same task, becoming commonplace across many areas of machine learning. While pretraining is empirically observed to be beneficial for a range of tasks, there is not a clear understanding yet of the reasons for this effect. In this work, we examine the relationship between pretrained vision transformers and the corresponding finetuned versions on several benchmark datasets and tasks. We present new metrics that specifically investigate the degree to which invariances learned by a pretrained model are retained or forgotten during finetuning. Using these metrics, we present a suite of empirical findings, including that pretraining induces transferable invariances in shallow layers and that invariances from deeper pretrained layers are compressed towards shallower layers during finetuning. Together, these findings contribute to understanding some of the reasons for the successes of pretrained models and the changes that a pretrained model undergoes when finetuned on a downstream task.
9.Pyramid Deep Fusion Network for Two-Hand Reconstruction from RGB-D Images
Authors:Jinwei Ren, Jianke Zhu
Abstract: Accurately recovering the dense 3D mesh of both hands from monocular images poses considerable challenges due to occlusions and projection ambiguity. Most of the existing methods extract features from color images to estimate the root-aligned hand meshes, which neglect the crucial depth and scale information in the real world. Given the noisy sensor measurements with limited resolution, depth-based methods predict 3D keypoints rather than a dense mesh. These limitations motivate us to take advantage of these two complementary inputs to acquire dense hand meshes on a real-world scale. In this work, we propose an end-to-end framework for recovering dense meshes for both hands, which employ single-view RGB-D image pairs as input. The primary challenge lies in effectively utilizing two different input modalities to mitigate the blurring effects in RGB images and noises in depth images. Instead of directly treating depth maps as additional channels for RGB images, we encode the depth information into the unordered point cloud to preserve more geometric details. Specifically, our framework employs ResNet50 and PointNet++ to derive features from RGB and point cloud, respectively. Additionally, we introduce a novel pyramid deep fusion network (PDFNet) to aggregate features at different scales, which demonstrates superior efficacy compared to previous fusion strategies. Furthermore, we employ a GCN-based decoder to process the fused features and recover the corresponding 3D pose and dense mesh. Through comprehensive ablation experiments, we have not only demonstrated the effectiveness of our proposed fusion algorithm but also outperformed the state-of-the-art approaches on publicly available datasets. To reproduce the results, we will make our source code and models publicly available at {\url{https://github.com/zijinxuxu/PDFNet}}.
10.Visualization for Multivariate Gaussian Anomaly Detection in Images
Authors:Joao P C Bertoldo, David Arrustico
Abstract: This paper introduces a simplified variation of the PaDiM (Pixel-Wise Anomaly Detection through Instance Modeling) method for anomaly detection in images, fitting a single multivariate Gaussian (MVG) distribution to the feature vectors extracted from a backbone convolutional neural network (CNN) and using their Mahalanobis distance as the anomaly score. We introduce an intermediate step in this framework by applying a whitening transformation to the feature vectors, which enables the generation of heatmaps capable of visually explaining the features learned by the MVG. The proposed technique is evaluated on the MVTec-AD dataset, and the results show the importance of visual model validation, providing insights into issues in this framework that were otherwise invisible. The visualizations generated for this paper are publicly available at https://doi.org/10.5281/zenodo.7937978.
11.Operational Support Estimator Networks
Authors:Mete Ahishali, Mehmet Yamac, Serkan Kiranyaz, Moncef Gabbouj
Abstract: In this work, we propose a novel approach called Operational Support Estimator Networks (OSENs) for the support estimation task. Support Estimation (SE) is defined as finding the locations of non-zero elements in a sparse signal. By its very nature, the mapping between the measurement and sparse signal is a non-linear operation. Traditional support estimators rely on computationally expensive iterative signal recovery techniques to achieve such non-linearity. Contrary to the convolution layers, the proposed OSEN approach consists of operational layers that can learn such complex non-linearities without the need for deep networks. In this way, the performance of the non-iterative support estimation is greatly improved. Moreover, the operational layers comprise so-called generative \textit{super neurons} with non-local kernels. The kernel location for each neuron/feature map is optimized jointly for the SE task during the training. We evaluate the OSENs in three different applications: i. support estimation from Compressive Sensing (CS) measurements, ii. representation-based classification, and iii. learning-aided CS reconstruction where the output of OSENs is used as prior knowledge to the CS algorithm for an enhanced reconstruction. Experimental results show that the proposed approach achieves computational efficiency and outperforms competing methods, especially at low measurement rates by a significant margin. The software implementation is publicly shared at https://github.com/meteahishali/OSEN.
12.AICT: An Adaptive Image Compression Transformer
Authors:Ahmed Ghorbel, Wassim Hamidouche, Luce Morin
Abstract: Motivated by the efficiency investigation of the Tranformer-based transform coding framework, namely SwinT-ChARM, we propose to enhance the latter, as first, with a more straightforward yet effective Tranformer-based channel-wise auto-regressive prior model, resulting in an absolute image compression transformer (ICT). Current methods that still rely on ConvNet-based entropy coding are limited in long-range modeling dependencies due to their local connectivity and an increasing number of architectural biases and priors. On the contrary, the proposed ICT can capture both global and local contexts from the latent representations and better parameterize the distribution of the quantized latents. Further, we leverage a learnable scaling module with a sandwich ConvNeXt-based pre/post-processor to accurately extract more compact latent representation while reconstructing higher-quality images. Extensive experimental results on benchmark datasets showed that the proposed adaptive image compression transformer (AICT) framework significantly improves the trade-off between coding efficiency and decoder complexity over the versatile video coding (VVC) reference encoder (VTM-18.0) and the neural codec SwinT-ChARM.
13.RFENet: Towards Reciprocal Feature Evolution for Glass Segmentation
Authors:Ke Fan, Changan Wang, Yabiao Wang, Chengjie Wang, Ran Yi, Lizhuang Ma
Abstract: Glass-like objects are widespread in daily life but remain intractable to be segmented for most existing methods. The transparent property makes it difficult to be distinguished from background, while the tiny separation boundary further impedes the acquisition of their exact contour. In this paper, by revealing the key co-evolution demand of semantic and boundary learning, we propose a Selective Mutual Evolution (SME) module to enable the reciprocal feature learning between them. Then to exploit the global shape context, we propose a Structurally Attentive Refinement (SAR) module to conduct a fine-grained feature refinement for those ambiguous points around the boundary. Finally, to further utilize the multi-scale representation, we integrate the above two modules into a cascaded structure and then introduce a Reciprocal Feature Evolution Network (RFENet) for effective glass-like object segmentation. Extensive experiments demonstrate that our RFENet achieves state-of-the-art performance on three popular public datasets.
14.TreeFormer: a Semi-Supervised Transformer-based Framework for Tree Counting from a Single High Resolution Image
Authors:Hamed Amini Amirkolaee, Miaojing Shi, Mark Mulligan
Abstract: Automatic tree density estimation and counting using single aerial and satellite images is a challenging task in photogrammetry and remote sensing, yet has an important role in forest management. In this paper, we propose the first semisupervised transformer-based framework for tree counting which reduces the expensive tree annotations for remote sensing images. Our method, termed as TreeFormer, first develops a pyramid tree representation module based on transformer blocks to extract multi-scale features during the encoding stage. Contextual attention-based feature fusion and tree density regressor modules are further designed to utilize the robust features from the encoder to estimate tree density maps in the decoder. Moreover, we propose a pyramid learning strategy that includes local tree density consistency and local tree count ranking losses to utilize unlabeled images into the training process. Finally, the tree counter token is introduced to regulate the network by computing the global tree counts for both labeled and unlabeled images. Our model was evaluated on two benchmark tree counting datasets, Jiangsu, and Yosemite, as well as a new dataset, KCL-London, created by ourselves. Our TreeFormer outperforms the state of the art semi-supervised methods under the same setting and exceeds the fully-supervised methods using the same number of labeled images. The codes and datasets are available at https://github.com/HAAClassic/TreeFormer.
15.Recognizing student identification numbers from the matrix templates using a modified U-net architecture
Authors:Filip Pavičić
Abstract: This paper presents an innovative approach to student identification during exams and knowledge tests, which overcomes the limitations of the traditional personal information entry method. The proposed method employs a matrix template on the designated section of the exam, where squares containing numbers are selectively blackened. The methodology involves the development of a neural network specifically designed for recognizing students' personal identification numbers. The neural network utilizes a specially adapted U-Net architecture, trained on an extensive dataset comprising images of blackened tables. The network demonstrates proficiency in recognizing the patterns and arrangement of blackened squares, accurately interpreting the information inscribed within them. Additionally, the model exhibits high accuracy in correctly identifying entered student personal numbers and effectively detecting erroneous entries within the table. This approach offers multiple advantages. Firstly, it significantly accelerates the exam marking process by automatically extracting identifying information from the blackened tables, eliminating the need for manual entry and minimizing the potential for errors. Secondly, the method automates the identification process, thereby reducing administrative effort and expediting data processing. The introduction of this innovative identification system represents a notable advancement in the field of exams and knowledge tests, replacing the conventional manual entry of personal data with a streamlined, efficient, and accurate identification process.
16.Learning Kernel-Modulated Neural Representation for Efficient Light Field Compression
Authors:Jinglei Shi, Yihong Xu, Christine Guillemot
Abstract: Light field is a type of image data that captures the 3D scene information by recording light rays emitted from a scene at various orientations. It offers a more immersive perception than classic 2D images but at the cost of huge data volume. In this paper, we draw inspiration from the visual characteristics of Sub-Aperture Images (SAIs) of light field and design a compact neural network representation for the light field compression task. The network backbone takes randomly initialized noise as input and is supervised on the SAIs of the target light field. It is composed of two types of complementary kernels: descriptive kernels (descriptors) that store scene description information learned during training, and modulatory kernels (modulators) that control the rendering of different SAIs from the queried perspectives. To further enhance compactness of the network meanwhile retain high quality of the decoded light field, we accordingly introduce modulator allocation and kernel tensor decomposition mechanisms, followed by non-uniform quantization and lossless entropy coding techniques, to finally form an efficient compression pipeline. Extensive experiments demonstrate that our method outperforms other state-of-the-art (SOTA) methods by a significant margin in the light field compression task. Moreover, after aligning descriptors, the modulators learned from one light field can be transferred to new light fields for rendering dense views, indicating a potential solution for view synthesis task.
17.The IMPTC Dataset: An Infrastructural Multi-Person Trajectory and Context Dataset
Authors:Manuel Hetzel, Hannes Reichert, Günther Reitberger, Erich Fuchs, Konrad Doll, Bernhard Sick
Abstract: Inner-city intersections are among the most critical traffic areas for injury and fatal accidents. Automated vehicles struggle with the complex and hectic everyday life within those areas. Sensor-equipped smart infrastructures, which can cooperate with vehicles, can benefit automated traffic by extending the perception capabilities of drivers and vehicle perception systems. Additionally, they offer the opportunity to gather reproducible and precise data of a holistic scene understanding, including context information as a basis for training algorithms for various applications in automated traffic. Therefore, we introduce the Infrastructural Multi-Person Trajectory and Context Dataset (IMPTC). We use an intelligent public inner-city intersection in Germany with visual sensor technology. A multi-view camera and LiDAR system perceives traffic situations and road users' behavior. Additional sensors monitor contextual information like weather, lighting, and traffic light signal status. The data acquisition system focuses on Vulnerable Road Users (VRUs) and multi-agent interaction. The resulting dataset consists of eight hours of measurement data. It contains over 2,500 VRU trajectories, including pedestrians, cyclists, e-scooter riders, strollers, and wheelchair users, and over 20,000 vehicle trajectories at different day times, weather conditions, and seasons. In addition, to enable the entire stack of research capabilities, the dataset includes all data, starting from the sensor-, calibration- and detection data until trajectory and context data. The dataset is continuously expanded and is available online for non-commercial research at https://github.com/kav-institute/imptc-dataset.
18.Can Vision-Language Models be a Good Guesser? Exploring VLMs for Times and Location Reasoning
Authors:Gengyuan Zhang, Yurui Zhang, Kerui Zhang, Volker Tresp
Abstract: Vision-Language Models (VLMs) are expected to be capable of reasoning with commonsense knowledge as human beings. One example is that humans can reason where and when an image is taken based on their knowledge. This makes us wonder if, based on visual cues, Vision-Language Models that are pre-trained with large-scale image-text resources can achieve and even outperform human's capability in reasoning times and location. To address this question, we propose a two-stage \recognition\space and \reasoning\space probing task, applied to discriminative and generative VLMs to uncover whether VLMs can recognize times and location-relevant features and further reason about it. To facilitate the investigation, we introduce WikiTiLo, a well-curated image dataset compromising images with rich socio-cultural cues. In the extensive experimental studies, we find that although VLMs can effectively retain relevant features in visual encoders, they still fail to make perfect reasoning. We will release our dataset and codes to facilitate future studies.
19.Smart Infrastructure: A Research Junction
Authors:Manuel Hetzel, Hannes Reichert, Konrad Doll, Bernhard Sick
Abstract: Complex inner-city junctions are among the most critical traffic areas for injury and fatal accidents. The development of highly automated driving (HAD) systems struggles with the complex and hectic everyday life within those areas. Sensor-equipped smart infrastructures, which can communicate and cooperate with vehicles, are essential to enable a holistic scene understanding to resolve occlusions drivers and vehicle perception systems for themselves can not cover. We introduce an intelligent research infrastructure equipped with visual sensor technology, located at a public inner-city junction in Aschaffenburg, Germany. A multiple-view camera system monitors the traffic situation to perceive road users' behavior. Both motorized and non-motorized traffic is considered. The system is used for research in data generation, evaluating new HAD sensors systems, algorithms, and Artificial Intelligence (AI) training strategies using real-, synthetic- and augmented data. In addition, the junction features a highly accurate digital twin. Real-world data can be taken into the digital twin for simulation purposes and synthetic data generation.
20.Large Class Separation is not what you need for Relational Reasoning-based OOD Detection
Authors:Lorenzo Li Lu, Giulia D'Ascenzi, Francesco Cappio Borlino, Tatiana Tommasi
Abstract: Standard recognition approaches are unable to deal with novel categories at test time. Their overconfidence on the known classes makes the predictions unreliable for safety-critical applications such as healthcare or autonomous driving. Out-Of-Distribution (OOD) detection methods provide a solution by identifying semantic novelty. Most of these methods leverage a learning stage on the known data, which means training (or fine-tuning) a model to capture the concept of normality. This process is clearly sensitive to the amount of available samples and might be computationally expensive for on-board systems. A viable alternative is that of evaluating similarities in the embedding space produced by large pre-trained models without any further learning effort. We focus exactly on such a fine-tuning-free OOD detection setting. This works presents an in-depth analysis of the recently introduced relational reasoning pre-training and investigates the properties of the learned embedding, highlighting the existence of a correlation between the inter-class feature distance and the OOD detection accuracy. As the class separation depends on the chosen pre-training objective, we propose an alternative loss function to control the inter-class margin, and we show its advantage with thorough experiments.
21.CellGAN: Conditional Cervical Cell Synthesis for Augmenting Cytopathological Image Classification
Authors:Zhenrong Shen, Maosong Cao, Sheng Wang, Lichi Zhang, Qian Wang
Abstract: Automatic examination of thin-prep cytologic test (TCT) slides can assist pathologists in finding cervical abnormality for accurate and efficient cancer screening. Current solutions mostly need to localize suspicious cells and classify abnormality based on local patches, concerning the fact that whole slide images of TCT are extremely large. It thus requires many annotations of normal and abnormal cervical cells, to supervise the training of the patch-level classifier for promising performance. In this paper, we propose CellGAN to synthesize cytopathological images of various cervical cell types for augmenting patch-level cell classification. Built upon a lightweight backbone, CellGAN is equipped with a non-linear class mapping network to effectively incorporate cell type information into image generation. We also propose the Skip-layer Global Context module to model the complex spatial relationship of the cells, and attain high fidelity of the synthesized images through adversarial learning. Our experiments demonstrate that CellGAN can produce visually plausible TCT cytopathological images for different cell types. We also validate the effectiveness of using CellGAN to greatly augment patch-level cell classification performance.
22.SepVAE: a contrastive VAE to separate pathological patterns from healthy ones
Authors:Robin Louiset, Edouard Duchesnay, Antoine Grigis, Benoit Dufumier, Pietro Gori
Abstract: Contrastive Analysis VAE (CA-VAEs) is a family of Variational auto-encoders (VAEs) that aims at separating the common factors of variation between a background dataset (BG) (i.e., healthy subjects) and a target dataset (TG) (i.e., patients) from the ones that only exist in the target dataset. To do so, these methods separate the latent space into a set of salient features (i.e., proper to the target dataset) and a set of common features (i.e., exist in both datasets). Currently, all models fail to prevent the sharing of information between latent spaces effectively and to capture all salient factors of variation. To this end, we introduce two crucial regularization losses: a disentangling term between common and salient representations and a classification term between background and target samples in the salient space. We show a better performance than previous CA-VAEs methods on three medical applications and a natural images dataset (CelebA). Code and datasets are available on GitHub https://github.com/neurospin-projects/2023_rlouiset_sepvae.
23.UGCANet: A Unified Global Context-Aware Transformer-based Network with Feature Alignment for Endoscopic Image Analysis
Authors:Pham Vu Hung, Nguyen Duy Manh, Nguyen Thi Oanh, Nguyen Thi Thuy, Dinh Viet Sang
Abstract: Gastrointestinal endoscopy is a medical procedure that utilizes a flexible tube equipped with a camera and other instruments to examine the digestive tract. This minimally invasive technique allows for diagnosing and managing various gastrointestinal conditions, including inflammatory bowel disease, gastrointestinal bleeding, and colon cancer. The early detection and identification of lesions in the upper gastrointestinal tract and the identification of malignant polyps that may pose a risk of cancer development are critical components of gastrointestinal endoscopy's diagnostic and therapeutic applications. Therefore, enhancing the detection rates of gastrointestinal disorders can significantly improve a patient's prognosis by increasing the likelihood of timely medical intervention, which may prolong the patient's lifespan and improve overall health outcomes. This paper presents a novel Transformer-based deep neural network designed to perform multiple tasks simultaneously, thereby enabling accurate identification of both upper gastrointestinal tract lesions and colon polyps. Our approach proposes a unique global context-aware module and leverages the powerful MiT backbone, along with a feature alignment block, to enhance the network's representation capability. This novel design leads to a significant improvement in performance across various endoscopic diagnosis tasks. Extensive experiments demonstrate the superior performance of our method compared to other state-of-the-art approaches.
24.Exposing the Fake: Effective Diffusion-Generated Images Detection
Authors:Ruipeng Ma, Jinhao Duan, Fei Kong, Xiaoshuang Shi, Kaidi Xu
Abstract: Image synthesis has seen significant advancements with the advent of diffusion-based generative models like Denoising Diffusion Probabilistic Models (DDPM) and text-to-image diffusion models. Despite their efficacy, there is a dearth of research dedicated to detecting diffusion-generated images, which could pose potential security and privacy risks. This paper addresses this gap by proposing a novel detection method called Stepwise Error for Diffusion-generated Image Detection (SeDID). Comprising statistical-based $\text{SeDID}_{\text{Stat}}$ and neural network-based $\text{SeDID}_{\text{NNs}}$, SeDID exploits the unique attributes of diffusion models, namely deterministic reverse and deterministic denoising computation errors. Our evaluations demonstrate SeDID's superior performance over existing methods when applied to diffusion models. Thus, our work makes a pivotal contribution to distinguishing diffusion model-generated images, marking a significant step in the domain of artificial intelligence security.
25.Stochastic Light Field Holography
Authors:Florian Schiffers, Praneeth Chakravarthula, Nathan Matsuda, Grace Kuo, Ethan Tseng, Douglas Lanman, Felix Heide, Oliver Cossairt
Abstract: The Visual Turing Test is the ultimate goal to evaluate the realism of holographic displays. Previous studies have focused on addressing challenges such as limited \'etendue and image quality over a large focal volume, but they have not investigated the effect of pupil sampling on the viewing experience in full 3D holograms. In this work, we tackle this problem with a novel hologram generation algorithm motivated by matching the projection operators of incoherent Light Field and coherent Wigner Function light transport. To this end, we supervise hologram computation using synthesized photographs, which are rendered on-the-fly using Light Field refocusing from stochastically sampled pupil states during optimization. The proposed method produces holograms with correct parallax and focus cues, which are important for passing the Visual Turing Test. We validate that our approach compares favorably to state-of-the-art CGH algorithms that use Light Field and Focal Stack supervision. Our experiments demonstrate that our algorithm significantly improves the realism of the viewing experience for a variety of different pupil states.
26.MMBench: Is Your Multi-modal Model an All-around Player?
Authors:Yuan Liu, Haodong Duan, Yuanhan Zhang, Bo Li, Songyang Zhang, Wangbo Zhao, Yike Yuan, Jiaqi Wang, Conghui He, Ziwei Liu, Kai Chen, Dahua Lin
Abstract: Large vision-language models have recently achieved remarkable progress, exhibiting great perception and reasoning abilities concerning visual information. However, how to effectively evaluate these large vision-language models remains a major obstacle, hindering future model development. Traditional benchmarks like VQAv2 or COCO Caption provide quantitative performance measurements but suffer from a lack of fine-grained ability assessment and non-robust evaluation metrics. Recent subjective benchmarks, such as OwlEval, offer comprehensive evaluations of a model's abilities by incorporating human labor, but they are not scalable and display significant bias. In response to these challenges, we propose MMBench, a novel multi-modality benchmark. MMBench methodically develops a comprehensive evaluation pipeline, primarily comprised of two elements. The first element is a meticulously curated dataset that surpasses existing similar benchmarks in terms of the number and variety of evaluation questions and abilities. The second element introduces a novel CircularEval strategy and incorporates the use of ChatGPT. This implementation is designed to convert free-form predictions into pre-defined choices, thereby facilitating a more robust evaluation of the model's predictions. MMBench is a systematically-designed objective benchmark for robustly evaluating the various abilities of vision-language models. We hope MMBench will assist the research community in better evaluating their models and encourage future advancements in this domain. Project page: https://opencompass.org.cn/mmbench.
27.Improved Real-time Image Smoothing with Weak Structures Preserved and High-contrast Details Removed
Authors:Shengchun Wang, Wencheng Wang, Fei Hou
Abstract: Image smoothing is by reducing pixel-wise gradients to smooth out details. As existing methods always rely on gradients to determine smoothing manners, it is difficult to distinguish structures and details to handle distinctively due to the overlapped ranges of gradients for structures and details. Thus, it is still challenging to achieve high-quality results, especially on preserving weak structures and removing high-contrast details. In this paper, we address this challenge by improving the real-time optimization-based method via iterative least squares (called ILS). We observe that 1) ILS uses gradients as the independent variable in its penalty function for determining smoothing manners, and 2) the framework of ILS can still work for image smoothing when we use some values instead of gradients in the penalty function. Thus, corresponding to the properties of pixels on structures or not, we compute some values to use in the penalty function to determine smoothing manners, and so we can handle structures and details distinctively, no matter whether their gradients are high or low. As a result, we can conveniently remove high-contrast details while preserving weak structures. Moreover, such values can be adjusted to accelerate optimization computation, so that we can use fewer iterations than the original ILS method for efficiency. This also reduces the changes onto structures to help structure preservation. Experimental results show our advantages over existing methods on efficiency and quality.
28.Patch n' Pack: NaViT, a Vision Transformer for any Aspect Ratio and Resolution
Authors:Mostafa Dehghani, Basil Mustafa, Josip Djolonga, Jonathan Heek, Matthias Minderer, Mathilde Caron, Andreas Steiner, Joan Puigcerver, Robert Geirhos, Ibrahim Alabdulmohsin, Avital Oliver, Piotr Padlewski, Alexey Gritsenko, Mario Lučić, Neil Houlsby
Abstract: The ubiquitous and demonstrably suboptimal choice of resizing images to a fixed resolution before processing them with computer vision models has not yet been successfully challenged. However, models such as the Vision Transformer (ViT) offer flexible sequence-based modeling, and hence varying input sequence lengths. We take advantage of this with NaViT (Native Resolution ViT) which uses sequence packing during training to process inputs of arbitrary resolutions and aspect ratios. Alongside flexible model usage, we demonstrate improved training efficiency for large-scale supervised and contrastive image-text pretraining. NaViT can be efficiently transferred to standard tasks such as image and video classification, object detection, and semantic segmentation and leads to improved results on robustness and fairness benchmarks. At inference time, the input resolution flexibility can be used to smoothly navigate the test-time cost-performance trade-off. We believe that NaViT marks a departure from the standard, CNN-designed, input and modelling pipeline used by most computer vision models, and represents a promising direction for ViTs.
29.Facial Reenactment Through a Personalized Generator
Authors:Ariel Elazary, Yotam Nitzan, Daniel Cohen-Or
Abstract: In recent years, the role of image generative models in facial reenactment has been steadily increasing. Such models are usually subject-agnostic and trained on domain-wide datasets. The appearance of the reenacted individual is learned from a single image, and hence, the entire breadth of the individual's appearance is not entirely captured, leading these methods to resort to unfaithful hallucination. Thanks to recent advancements, it is now possible to train a personalized generative model tailored specifically to a given individual. In this paper, we propose a novel method for facial reenactment using a personalized generator. We train the generator using frames from a short, yet varied, self-scan video captured using a simple commodity camera. Images synthesized by the personalized generator are guaranteed to preserve identity. The premise of our work is that the task of reenactment is thus reduced to accurately mimicking head poses and expressions. To this end, we locate the desired frames in the latent space of the personalized generator using carefully designed latent optimization. Through extensive evaluation, we demonstrate state-of-the-art performance for facial reenactment. Furthermore, we show that since our reenactment takes place in a semantic latent space, it can be semantically edited and stylized in post-processing.
30.Correlation-Aware Mutual Learning for Semi-supervised Medical Image Segmentation
Authors:Shengbo Gao, Ziji Zhang, Jiechao Ma, Zihao Li, Shu Zhang
Abstract: Semi-supervised learning has become increasingly popular in medical image segmentation due to its ability to leverage large amounts of unlabeled data to extract additional information. However, most existing semi-supervised segmentation methods only focus on extracting information from unlabeled data, disregarding the potential of labeled data to further improve the performance of the model. In this paper, we propose a novel Correlation Aware Mutual Learning (CAML) framework that leverages labeled data to guide the extraction of information from unlabeled data. Our approach is based on a mutual learning strategy that incorporates two modules: the Cross-sample Mutual Attention Module (CMA) and the Omni-Correlation Consistency Module (OCC). The CMA module establishes dense cross-sample correlations among a group of samples, enabling the transfer of label prior knowledge to unlabeled data. The OCC module constructs omni-correlations between the unlabeled and labeled datasets and regularizes dual models by constraining the omni-correlation matrix of each sub-model to be consistent. Experiments on the Atrial Segmentation Challenge dataset demonstrate that our proposed approach outperforms state-of-the-art methods, highlighting the effectiveness of our framework in medical image segmentation tasks. The codes, pre-trained weights, and data are publicly available.
31.Deep Learning of Crystalline Defects from TEM images: A Solution for the Problem of "Never Enough Training Data"
Authors:Kishan Govind, Daniela Oliveros, Antonin Dlouhy, Marc Legros, Stefan Sandfeld
Abstract: Crystalline defects, such as line-like dislocations, play an important role for the performance and reliability of many metallic devices. Their interaction and evolution still poses a multitude of open questions to materials science and materials physics. In-situ TEM experiments can provide important insights into how dislocations behave and move. During such experiments, the dislocation microstructure is captured in form of videos. The analysis of individual video frames can provide useful insights but is limited by the capabilities of automated identification, digitization, and quantitative extraction of the dislocations as curved objects. The vast amount of data also makes manual annotation very time consuming, thereby limiting the use of Deep Learning-based, automated image analysis and segmentation of the dislocation microstructure. In this work, a parametric model for generating synthetic training data for segmentation of dislocations is developed. Even though domain scientists might dismiss synthetic training images sometimes as too artificial, our findings show that they can result in superior performance, particularly regarding the generalizing of the Deep Learning models with respect to different microstructures and imaging conditions. Additionally, we propose an enhanced deep learning method optimized for segmenting overlapping or intersecting dislocation lines. Upon testing this framework on four distinct real datasets, we find that our synthetic training data are able to yield high-quality results also on real images-even more so if fine-tune on a few real images was done.