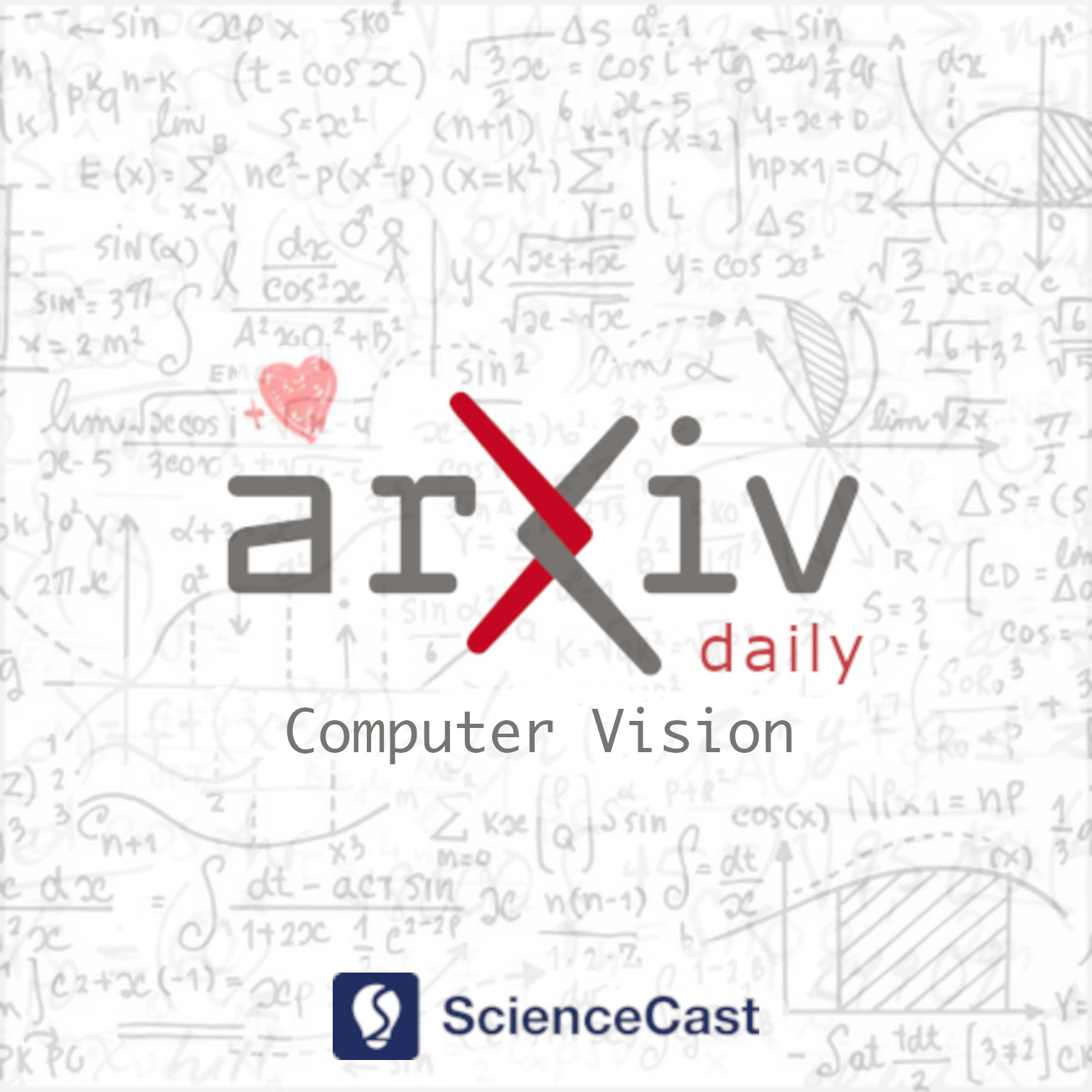
Computer Vision and Pattern Recognition (cs.CV)
Tue, 12 Sep 2023
1.Self-supervised Extraction of Human Motion Structures via Frame-wise Discrete Features
Authors:Tetsuya Abe, Ryusuke Sagawa, Ko Ayusawa, Wataru Takano
Abstract: The present paper proposes an encoder-decoder model for extracting the structures of human motions represented by frame-wise discrete features in a self-supervised manner. In the proposed method, features are extracted as codes in a motion codebook without the use of human knowledge, and the relationship between these codes can be visualized on a graph. Since the codes are expected to be temporally sparse compared to the captured frame rate and can be shared by multiple sequences, the proposed network model also addresses the need for training constraints. Specifically, the model consists of self-attention layers and a vector clustering block. The attention layers contribute to finding sparse keyframes and discrete features as motion codes, which are then extracted by vector clustering. The constraints are realized as training losses so that the same motion codes can be as contiguous as possible and can be shared by multiple sequences. In addition, we propose the use of causal self-attention as a method by which to calculate attention for long sequences consisting of numerous frames. In our experiments, the sparse structures of motion codes were used to compile a graph that facilitates visualization of the relationship between the codes and the differences between sequences. We then evaluated the effectiveness of the extracted motion codes by applying them to multiple recognition tasks and found that performance levels comparable to task-optimized methods could be achieved by linear probing.
2.FLDNet: A Foreground-Aware Network for Polyp Segmentation Leveraging Long-Distance Dependencies
Authors:Xuefeng Wei, Xuan Zhou
Abstract: Given the close association between colorectal cancer and polyps, the diagnosis and identification of colorectal polyps play a critical role in the detection and surgical intervention of colorectal cancer. In this context, the automatic detection and segmentation of polyps from various colonoscopy images has emerged as a significant problem that has attracted broad attention. Current polyp segmentation techniques face several challenges: firstly, polyps vary in size, texture, color, and pattern; secondly, the boundaries between polyps and mucosa are usually blurred, existing studies have focused on learning the local features of polyps while ignoring the long-range dependencies of the features, and also ignoring the local context and global contextual information of the combined features. To address these challenges, we propose FLDNet (Foreground-Long-Distance Network), a Transformer-based neural network that captures long-distance dependencies for accurate polyp segmentation. Specifically, the proposed model consists of three main modules: a pyramid-based Transformer encoder, a local context module, and a foreground-Aware module. Multilevel features with long-distance dependency information are first captured by the pyramid-based transformer encoder. On the high-level features, the local context module obtains the local characteristics related to the polyps by constructing different local context information. The coarse map obtained by decoding the reconstructed highest-level features guides the feature fusion process in the foreground-Aware module of the high-level features to achieve foreground enhancement of the polyps. Our proposed method, FLDNet, was evaluated using seven metrics on common datasets and demonstrated superiority over state-of-the-art methods on widely-used evaluation measures.
3.ATTA: Anomaly-aware Test-Time Adaptation for Out-of-Distribution Detection in Segmentation
Authors:Zhitong Gao, Shipeng Yan, Xuming He
Abstract: Recent advancements in dense out-of-distribution (OOD) detection have primarily focused on scenarios where the training and testing datasets share a similar domain, with the assumption that no domain shift exists between them. However, in real-world situations, domain shift often exits and significantly affects the accuracy of existing out-of-distribution (OOD) detection models. In this work, we propose a dual-level OOD detection framework to handle domain shift and semantic shift jointly. The first level distinguishes whether domain shift exists in the image by leveraging global low-level features, while the second level identifies pixels with semantic shift by utilizing dense high-level feature maps. In this way, we can selectively adapt the model to unseen domains as well as enhance model's capacity in detecting novel classes. We validate the efficacy of our proposed method on several OOD segmentation benchmarks, including those with significant domain shifts and those without, observing consistent performance improvements across various baseline models.
4.TSSAT: Two-Stage Statistics-Aware Transformation for Artistic Style Transfer
Authors:Haibo Chen, Lei Zhao, Jun Li, Jian Yang
Abstract: Artistic style transfer aims to create new artistic images by rendering a given photograph with the target artistic style. Existing methods learn styles simply based on global statistics or local patches, lacking careful consideration of the drawing process in practice. Consequently, the stylization results either fail to capture abundant and diversified local style patterns, or contain undesired semantic information of the style image and deviate from the global style distribution. To address this issue, we imitate the drawing process of humans and propose a Two-Stage Statistics-Aware Transformation (TSSAT) module, which first builds the global style foundation by aligning the global statistics of content and style features and then further enriches local style details by swapping the local statistics (instead of local features) in a patch-wise manner, significantly improving the stylization effects. Moreover, to further enhance both content and style representations, we introduce two novel losses: an attention-based content loss and a patch-based style loss, where the former enables better content preservation by enforcing the semantic relation in the content image to be retained during stylization, and the latter focuses on increasing the local style similarity between the style and stylized images. Extensive qualitative and quantitative experiments verify the effectiveness of our method.
5.SoccerNet 2023 Challenges Results
Authors:Anthony Cioppa, Silvio Giancola, Vladimir Somers, Floriane Magera, Xin Zhou, Hassan Mkhallati, Adrien Deliège, Jan Held, Carlos Hinojosa, Amir M. Mansourian, Pierre Miralles, Olivier Barnich, Christophe De Vleeschouwer, Alexandre Alahi, Bernard Ghanem, Marc Van Droogenbroeck, Abdullah Kamal, Adrien Maglo, Albert Clapés, Amr Abdelaziz, Artur Xarles, Astrid Orcesi, Atom Scott, Bin Liu, Byoungkwon Lim, Chen Chen, Fabian Deuser, Feng Yan, Fufu Yu, Gal Shitrit, Guanshuo Wang, Gyusik Choi, Hankyul Kim, Hao Guo, Hasby Fahrudin, Hidenari Koguchi, Håkan Ardö, Ibrahim Salah, Ido Yerushalmy, Iftikar Muhammad, Ikuma Uchida, Ishay Be'ery, Jaonary Rabarisoa, Jeongae Lee, Jiajun Fu, Jianqin Yin, Jinghang Xu, Jongho Nang, Julien Denize, Junjie Li, Junpei Zhang, Juntae Kim, Kamil Synowiec, Kenji Kobayashi, Kexin Zhang, Konrad Habel, Kota Nakajima, Licheng Jiao, Lin Ma, Lizhi Wang, Luping Wang, Menglong Li, Mengying Zhou, Mohamed Nasr, Mohamed Abdelwahed, Mykola Liashuha, Nikolay Falaleev, Norbert Oswald, Qiong Jia, Quoc-Cuong Pham, Ran Song, Romain Hérault, Rui Peng, Ruilong Chen, Ruixuan Liu, Ruslan Baikulov, Ryuto Fukushima, Sergio Escalera, Seungcheon Lee, Shimin Chen, Shouhong Ding, Taiga Someya, Thomas B. Moeslund, Tianjiao Li, Wei Shen, Wei Zhang, Wei Li, Wei Dai, Weixin Luo, Wending Zhao, Wenjie Zhang, Xinquan Yang, Yanbiao Ma, Yeeun Joo, Yingsen Zeng, Yiyang Gan, Yongqiang Zhu, Yujie Zhong, Zheng Ruan, Zhiheng Li, Zhijian Huang, Ziyu Meng
Abstract: The SoccerNet 2023 challenges were the third annual video understanding challenges organized by the SoccerNet team. For this third edition, the challenges were composed of seven vision-based tasks split into three main themes. The first theme, broadcast video understanding, is composed of three high-level tasks related to describing events occurring in the video broadcasts: (1) action spotting, focusing on retrieving all timestamps related to global actions in soccer, (2) ball action spotting, focusing on retrieving all timestamps related to the soccer ball change of state, and (3) dense video captioning, focusing on describing the broadcast with natural language and anchored timestamps. The second theme, field understanding, relates to the single task of (4) camera calibration, focusing on retrieving the intrinsic and extrinsic camera parameters from images. The third and last theme, player understanding, is composed of three low-level tasks related to extracting information about the players: (5) re-identification, focusing on retrieving the same players across multiple views, (6) multiple object tracking, focusing on tracking players and the ball through unedited video streams, and (7) jersey number recognition, focusing on recognizing the jersey number of players from tracklets. Compared to the previous editions of the SoccerNet challenges, tasks (2-3-7) are novel, including new annotations and data, task (4) was enhanced with more data and annotations, and task (6) now focuses on end-to-end approaches. More information on the tasks, challenges, and leaderboards are available on https://www.soccer-net.org. Baselines and development kits can be found on https://github.com/SoccerNet.
6.Feature Aggregation Network for Building Extraction from High-resolution Remote Sensing Images
Authors:Xuan Zhou, Xuefeng Wei
Abstract: The rapid advancement in high-resolution satellite remote sensing data acquisition, particularly those achieving submeter precision, has uncovered the potential for detailed extraction of surface architectural features. However, the diversity and complexity of surface distributions frequently lead to current methods focusing exclusively on localized information of surface features. This often results in significant intraclass variability in boundary recognition and between buildings. Therefore, the task of fine-grained extraction of surface features from high-resolution satellite imagery has emerged as a critical challenge in remote sensing image processing. In this work, we propose the Feature Aggregation Network (FANet), concentrating on extracting both global and local features, thereby enabling the refined extraction of landmark buildings from high-resolution satellite remote sensing imagery. The Pyramid Vision Transformer captures these global features, which are subsequently refined by the Feature Aggregation Module and merged into a cohesive representation by the Difference Elimination Module. In addition, to ensure a comprehensive feature map, we have incorporated the Receptive Field Block and Dual Attention Module, expanding the receptive field and intensifying attention across spatial and channel dimensions. Extensive experiments on multiple datasets have validated the outstanding capability of FANet in extracting features from high-resolution satellite images. This signifies a major breakthrough in the field of remote sensing image processing. We will release our code soon.
7.Learning from History: Task-agnostic Model Contrastive Learning for Image Restoration
Authors:Gang Wu, Junjun Jiang, Kui Jiang, Xianming Liu
Abstract: Contrastive learning has emerged as a prevailing paradigm for high-level vision tasks, which, by introducing properly negative samples, has also been exploited for low-level vision tasks to achieve a compact optimization space to account for their ill-posed nature. However, existing methods rely on manually predefined, task-oriented negatives, which often exhibit pronounced task-specific biases. In this paper, we propose a innovative approach for the adaptive generation of negative samples directly from the target model itself, called ``learning from history``. We introduce the Self-Prior guided Negative loss for image restoration (SPNIR) to enable this approach. Our approach is task-agnostic and generic, making it compatible with any existing image restoration method or task. We demonstrate the effectiveness of our approach by retraining existing models with SPNIR. The results show significant improvements in image restoration across various tasks and architectures. For example, models retrained with SPNIR outperform the original FFANet and DehazeFormer by 3.41 dB and 0.57 dB on the RESIDE indoor dataset for image dehazing. Similarly, they achieve notable improvements of 0.47 dB on SPA-Data over IDT for image deraining and 0.12 dB on Manga109 for a 4x scale super-resolution over lightweight SwinIR, respectively. Code and retrained models are available at https://github.com/Aitical/Task-agnostic_Model_Contrastive_Learning_Image_Restoration.
8.A new meteor detection application robust to camera movements
Authors:Clara Ciocan ALSOC, Mathuran Kandeepan ALSOC, Adrien Cassagne ALSOC, Jeremie Vaubaillon IMCCE, Fabian Zander USQ, Lionel Lacassagne ALSOC
Abstract: This article presents a new tool for the automatic detection of meteors. Fast Meteor Detection Toolbox (FMDT) is able to detect meteor sightings by analyzing videos acquired by cameras onboard weather balloons or within airplane with stabilization. The challenge consists in designing a processing chain composed of simple algorithms, that are robust to the high fluctuation of the videos and that satisfy the constraints on power consumption (10 W) and real-time processing (25 frames per second).
9.Federated Learning for Large-Scale Scene Modeling with Neural Radiance Fields
Authors:Teppei Suzuki
Abstract: We envision a system to continuously build and maintain a map based on earth-scale neural radiance fields (NeRF) using data collected from vehicles and drones in a lifelong learning manner. However, existing large-scale modeling by NeRF has problems in terms of scalability and maintainability when modeling earth-scale environments. Therefore, to address these problems, we propose a federated learning pipeline for large-scale modeling with NeRF. We tailor the model aggregation pipeline in federated learning for NeRF, thereby allowing local updates of NeRF. In the aggregation step, the accuracy of the clients' global pose is critical. Thus, we also propose global pose alignment to align the noisy global pose of clients before the aggregation step. In experiments, we show the effectiveness of the proposed pose alignment and the federated learning pipeline on the large-scale scene dataset, Mill19.
10.Real-Time Semantic Segmentation: A Brief Survey & Comparative Study in Remote Sensing
Authors:Clifford Broni-Bediako, Junshi Xia, Naoto Yokoya
Abstract: Real-time semantic segmentation of remote sensing imagery is a challenging task that requires a trade-off between effectiveness and efficiency. It has many applications including tracking forest fires, detecting changes in land use and land cover, crop health monitoring, and so on. With the success of efficient deep learning methods (i.e., efficient deep neural networks) for real-time semantic segmentation in computer vision, researchers have adopted these efficient deep neural networks in remote sensing image analysis. This paper begins with a summary of the fundamental compression methods for designing efficient deep neural networks and provides a brief but comprehensive survey, outlining the recent developments in real-time semantic segmentation of remote sensing imagery. We examine several seminal efficient deep learning methods, placing them in a taxonomy based on the network architecture design approach. Furthermore, we evaluate the quality and efficiency of some existing efficient deep neural networks on a publicly available remote sensing semantic segmentation benchmark dataset, the OpenEarthMap. The experimental results of an extensive comparative study demonstrate that most of the existing efficient deep neural networks have good segmentation quality, but they suffer low inference speed (i.e., high latency rate), which may limit their capability of deployment in real-time applications of remote sensing image segmentation. We provide some insights into the current trend and future research directions for real-time semantic segmentation of remote sensing imagery.
11.Estimating exercise-induced fatigue from thermal facial images
Authors:Manuel Lage Cañellas, Constantino Álvarez Casado, Le Nguyen, Miguel Bordallo López
Abstract: Exercise-induced fatigue resulting from physical activity can be an early indicator of overtraining, illness, or other health issues. In this article, we present an automated method for estimating exercise-induced fatigue levels through the use of thermal imaging and facial analysis techniques utilizing deep learning models. Leveraging a novel dataset comprising over 400,000 thermal facial images of rested and fatigued users, our results suggest that exercise-induced fatigue levels could be predicted with only one static thermal frame with an average error smaller than 15\%. The results emphasize the viability of using thermal imaging in conjunction with deep learning for reliable exercise-induced fatigue estimation.
12.Can we predict the Most Replayed data of video streaming platforms?
Authors:Alessandro Duico, Ombretta Strafforello, Jan van Gemert
Abstract: Predicting which specific parts of a video users will replay is important for several applications, including targeted advertisement placement on video platforms and assisting video creators. In this work, we explore whether it is possible to predict the Most Replayed (MR) data from YouTube videos. To this end, we curate a large video benchmark, the YTMR500 dataset, which comprises 500 YouTube videos with MR data annotations. We evaluate Deep Learning (DL) models of varying complexity on our dataset and perform an extensive ablation study. In addition, we conduct a user study to estimate the human performance on MR data prediction. Our results show that, although by a narrow margin, all the evaluated DL models outperform random predictions. Additionally, they exceed human-level accuracy. This suggests that predicting the MR data is a difficult task that can be enhanced through the assistance of DL. Finally, we believe that DL performance on MR data prediction can be further improved, for example, by using multi-modal learning. We encourage the research community to use our benchmark dataset to further investigate automatic MR data prediction.
13.Towards Visual Taxonomy Expansion
Authors:Tinghui Zhu, Jingping Liu, Jiaqing Liang, Haiyun Jiang, Yanghua Xiao, Zongyu Wang, Rui Xie, Yunsen Xian
Abstract: Taxonomy expansion task is essential in organizing the ever-increasing volume of new concepts into existing taxonomies. Most existing methods focus exclusively on using textual semantics, leading to an inability to generalize to unseen terms and the "Prototypical Hypernym Problem." In this paper, we propose Visual Taxonomy Expansion (VTE), introducing visual features into the taxonomy expansion task. We propose a textual hypernymy learning task and a visual prototype learning task to cluster textual and visual semantics. In addition to the tasks on respective modalities, we introduce a hyper-proto constraint that integrates textual and visual semantics to produce fine-grained visual semantics. Our method is evaluated on two datasets, where we obtain compelling results. Specifically, on the Chinese taxonomy dataset, our method significantly improves accuracy by 8.75 %. Additionally, our approach performs better than ChatGPT on the Chinese taxonomy dataset.
14.HOC-Search: Efficient CAD Model and Pose Retrieval from RGB-D Scans
Authors:Stefan Ainetter, Sinisa Stekovic, Friedrich Fraundorfer, Vincent Lepetit
Abstract: We present an automated and efficient approach for retrieving high-quality CAD models of objects and their poses in a scene captured by a moving RGB-D camera. We first investigate various objective functions to measure similarity between a candidate CAD object model and the available data, and the best objective function appears to be a "render-and-compare" method comparing depth and mask rendering. We thus introduce a fast-search method that approximates an exhaustive search based on this objective function for simultaneously retrieving the object category, a CAD model, and the pose of an object given an approximate 3D bounding box. This method involves a search tree that organizes the CAD models and object properties including object category and pose for fast retrieval and an algorithm inspired by Monte Carlo Tree Search, that efficiently searches this tree. We show that this method retrieves CAD models that fit the real objects very well, with a speed-up factor of 10x to 120x compared to exhaustive search.
15.C-RITNet: Set Infrared and Visible Image Fusion Free from Complementary Information Mining
Authors:Yafei Zhang, Keying Du, Huafeng Li, Zhengtao Yu, Yu Liu
Abstract: Infrared and visible image fusion (IVIF) aims to extract and integrate the complementary information in two different modalities to generate high-quality fused images with salient targets and abundant texture details. However, current image fusion methods go to great lengths to excavate complementary features, which is generally achieved through two efforts. On the one hand, the feature extraction network is expected to have excellent performance in extracting complementary information. On the other hand, complex fusion strategies are often designed to aggregate the complementary information. In other words, enabling the network to perceive and extract complementary information is extremely challenging. Complicated fusion strategies, while effective, still run the risk of losing weak edge details. To this end, this paper rethinks the IVIF outside the box, proposing a complementary-redundant information transfer network (C-RITNet). It reasonably transfers complementary information into redundant one, which integrates both the shared and complementary features from two modalities. Hence, the proposed method is able to alleviate the challenges posed by the complementary information extraction and reduce the reliance on sophisticated fusion strategies. Specifically, to skillfully sidestep aggregating complementary information in IVIF, we first design the mutual information transfer (MIT) module to mutually represent features from two modalities, roughly transferring complementary information into redundant one. Then, a redundant information acquisition supervised by source image (RIASSI) module is devised to further ensure the complementary-redundant information transfer after MIT. Meanwhile, we also propose a structure information preservation (SIP) module to guarantee that the edge structure information of the source images can be transferred to the fusion results.
16.Dynamic Visual Prompt Tuning for Parameter Efficient Transfer Learning
Authors:Chunqing Ruan, Hongjian Wang
Abstract: Parameter efficient transfer learning (PETL) is an emerging research spot that aims to adapt large-scale pre-trained models to downstream tasks. Recent advances have achieved great success in saving storage and computation costs. However, these methods do not take into account instance-specific visual clues for visual tasks. In this paper, we propose a Dynamic Visual Prompt Tuning framework (DVPT), which can generate a dynamic instance-wise token for each image. In this way, it can capture the unique visual feature of each image, which can be more suitable for downstream visual tasks. We designed a Meta-Net module that can generate learnable prompts based on each image, thereby capturing dynamic instance-wise visual features. Extensive experiments on a wide range of downstream recognition tasks show that DVPT achieves superior performance than other PETL methods. More importantly, DVPT even outperforms full fine-tuning on 17 out of 19 downstream tasks while maintaining high parameter efficiency. Our code will be released soon.
17.LEyes: A Lightweight Framework for Deep Learning-Based Eye Tracking using Synthetic Eye Images
Authors:sean anthony byrne, virmarie maquiling, marcus nyström, enkelejda kasneci, diederick c. niehorster
Abstract: Deep learning has bolstered gaze estimation techniques, but real-world deployment has been impeded by inadequate training datasets. This problem is exacerbated by both hardware-induced variations in eye images and inherent biological differences across the recorded participants, leading to both feature and pixel-level variance that hinders the generalizability of models trained on specific datasets. While synthetic datasets can be a solution, their creation is both time and resource-intensive. To address this problem, we present a framework called Light Eyes or "LEyes" which, unlike conventional photorealistic methods, only models key image features required for video-based eye tracking using simple light distributions. LEyes facilitates easy configuration for training neural networks across diverse gaze-estimation tasks. We demonstrate that models trained using LEyes outperform other state-of-the-art algorithms in terms of pupil and CR localization across well-known datasets. In addition, a LEyes trained model outperforms the industry standard eye tracker using significantly more cost-effective hardware. Going forward, we are confident that LEyes will revolutionize synthetic data generation for gaze estimation models, and lead to significant improvements of the next generation video-based eye trackers.
18.JOADAA: joint online action detection and action anticipation
Authors:Mohammed Guermal, Francois Bremond, Rui Dai, Abid Ali
Abstract: Action anticipation involves forecasting future actions by connecting past events to future ones. However, this reasoning ignores the real-life hierarchy of events which is considered to be composed of three main parts: past, present, and future. We argue that considering these three main parts and their dependencies could improve performance. On the other hand, online action detection is the task of predicting actions in a streaming manner. In this case, one has access only to the past and present information. Therefore, in online action detection (OAD) the existing approaches miss semantics or future information which limits their performance. To sum up, for both of these tasks, the complete set of knowledge (past-present-future) is missing, which makes it challenging to infer action dependencies, therefore having low performances. To address this limitation, we propose to fuse both tasks into a single uniform architecture. By combining action anticipation and online action detection, our approach can cover the missing dependencies of future information in online action detection. This method referred to as JOADAA, presents a uniform model that jointly performs action anticipation and online action detection. We validate our proposed model on three challenging datasets: THUMOS'14, which is a sparsely annotated dataset with one action per time step, CHARADES, and Multi-THUMOS, two densely annotated datasets with more complex scenarios. JOADAA achieves SOTA results on these benchmarks for both tasks.
19.Towards Reliable Domain Generalization: A New Dataset and Evaluations
Authors:Jiao Zhang, Xu-Yao Zhang, Cheng-Lin Liu
Abstract: There are ubiquitous distribution shifts in the real world. However, deep neural networks (DNNs) are easily biased towards the training set, which causes severe performance degradation when they receive out-of-distribution data. Many methods are studied to train models that generalize under various distribution shifts in the literature of domain generalization (DG). However, the recent DomainBed and WILDS benchmarks challenged the effectiveness of these methods. Aiming at the problems in the existing research, we propose a new domain generalization task for handwritten Chinese character recognition (HCCR) to enrich the application scenarios of DG method research. We evaluate eighteen DG methods on the proposed PaHCC (Printed and Handwritten Chinese Characters) dataset and show that the performance of existing methods on this dataset is still unsatisfactory. Besides, under a designed dynamic DG setting, we reveal more properties of DG methods and argue that only the leave-one-domain-out protocol is unreliable. We advocate that researchers in the DG community refer to dynamic performance of methods for more comprehensive and reliable evaluation. Our dataset and evaluations bring new perspectives to the community for more substantial progress. We will make our dataset public with the article published to facilitate the study of domain generalization.
20.Active Label Refinement for Semantic Segmentation of Satellite Images
Authors:Tuan Pham Minh, Jayan Wijesingha, Daniel Kottke, Marek Herde, Denis Huseljic, Bernhard Sick, Michael Wachendorf, Thomas Esch
Abstract: Remote sensing through semantic segmentation of satellite images contributes to the understanding and utilisation of the earth's surface. For this purpose, semantic segmentation networks are typically trained on large sets of labelled satellite images. However, obtaining expert labels for these images is costly. Therefore, we propose to rely on a low-cost approach, e.g. crowdsourcing or pretrained networks, to label the images in the first step. Since these initial labels are partially erroneous, we use active learning strategies to cost-efficiently refine the labels in the second step. We evaluate the active learning strategies using satellite images of Bengaluru in India, labelled with land cover and land use labels. Our experimental results suggest that an active label refinement to improve the semantic segmentation network's performance is beneficial.
21.Dual-Path Temporal Map Optimization for Make-up Temporal Video Grounding
Authors:Jiaxiu Li, Kun Li, Jia Li, Guoliang Chen, Dan Guo, Meng Wang
Abstract: Make-up temporal video grounding (MTVG) aims to localize the target video segment which is semantically related to a sentence describing a make-up activity, given a long video. Compared with the general video grounding task, MTVG focuses on meticulous actions and changes on the face. The make-up instruction step, usually involving detailed differences in products and facial areas, is more fine-grained than general activities (e.g, cooking activity and furniture assembly). Thus, existing general approaches cannot locate the target activity effectually. More specifically, existing proposal generation modules are not yet fully developed in providing semantic cues for the more fine-grained make-up semantic comprehension. To tackle this issue, we propose an effective proposal-based framework named Dual-Path Temporal Map Optimization Network (DPTMO) to capture fine-grained multimodal semantic details of make-up activities. DPTMO extracts both query-agnostic and query-guided features to construct two proposal sets and uses specific evaluation methods for the two sets. Different from the commonly used single structure in previous methods, our dual-path structure can mine more semantic information in make-up videos and distinguish fine-grained actions well. These two candidate sets represent the cross-modal makeup video-text similarity and multi-modal fusion relationship, complementing each other. Each set corresponds to its respective optimization perspective, and their joint prediction enhances the accuracy of video timestamp prediction. Comprehensive experiments on the YouMakeup dataset demonstrate our proposed dual structure excels in fine-grained semantic comprehension.
22.Computer Vision Pipeline for Automated Antarctic Krill Analysis
Authors:Mazvydas Gudelis, Michal Mackiewicz, Julie Bremner, Sophie Fielding
Abstract: British Antarctic Survey (BAS) researchers launch annual expeditions to the Antarctic in order to estimate Antarctic Krill biomass and assess the change from previous years. These comparisons provide insight into the effects of the current environment on this key component of the marine food chain. In this work we have developed tools for automating the data collection and analysis process, using web-based image annotation tools and deep learning image classification and regression models. We achieve highly accurate krill instance segmentation results with an average 77.28% AP score, as well as separate maturity stage and length estimation of krill specimens with 62.99% accuracy and a 1.96 mm length error respectively.
23.A 3M-Hybrid Model for the Restoration of Unique Giant Murals: A Case Study on the Murals of Yongle Palace
Authors:Jing Yang, Nur Intan Raihana Ruhaiyem, Chichun Zhou
Abstract: The Yongle Palace murals, as valuable cultural heritage, have suffered varying degrees of damage, making their restoration of significant importance. However, the giant size and unique data of Yongle Palace murals present challenges for existing deep-learning based restoration methods: 1) The distinctive style introduces domain bias in traditional transfer learning-based restoration methods, while the scarcity of mural data further limits the applicability of these methods. 2) Additionally, the giant size of these murals results in a wider range of defect types and sizes, necessitating models with greater adaptability. Consequently, there is a lack of focus on deep learning-based restoration methods for the unique giant murals of Yongle Palace. Here, a 3M-Hybrid model is proposed to address these challenges. Firstly, based on the characteristic that the mural data frequency is prominent in the distribution of low and high frequency features, high and low frequency features are separately abstracted for complementary learning. Furthermore, we integrate a pre-trained Vision Transformer model (VIT) into the CNN module, allowing us to leverage the benefits of a large model while mitigating domain bias. Secondly, we mitigate seam and structural distortion issues resulting from the restoration of large defects by employing a multi-scale and multi-perspective strategy, including data segmentation and fusion. Experimental results demonstrate the efficacy of our proposed model. In regular-sized mural restoration, it improves SSIM and PSNR by 14.61% and 4.73%, respectively, compared to the best model among four representative CNN models. Additionally, it achieves favorable results in the final restoration of giant murals.
24.360$^\circ$ from a Single Camera: A Few-Shot Approach for LiDAR Segmentation
Authors:Laurenz Reichardt, Nikolas Ebert, Oliver Wasenmüller
Abstract: Deep learning applications on LiDAR data suffer from a strong domain gap when applied to different sensors or tasks. In order for these methods to obtain similar accuracy on different data in comparison to values reported on public benchmarks, a large scale annotated dataset is necessary. However, in practical applications labeled data is costly and time consuming to obtain. Such factors have triggered various research in label-efficient methods, but a large gap remains to their fully-supervised counterparts. Thus, we propose ImageTo360, an effective and streamlined few-shot approach to label-efficient LiDAR segmentation. Our method utilizes an image teacher network to generate semantic predictions for LiDAR data within a single camera view. The teacher is used to pretrain the LiDAR segmentation student network, prior to optional fine-tuning on 360$^\circ$ data. Our method is implemented in a modular manner on the point level and as such is generalizable to different architectures. We improve over the current state-of-the-art results for label-efficient methods and even surpass some traditional fully-supervised segmentation networks.
25.SCP: Scene Completion Pre-training for 3D Object Detection
Authors:Yiming Shan, Yan Xia, Yuhong Chen, Daniel Cremers
Abstract: 3D object detection using LiDAR point clouds is a fundamental task in the fields of computer vision, robotics, and autonomous driving. However, existing 3D detectors heavily rely on annotated datasets, which are both time-consuming and prone to errors during the process of labeling 3D bounding boxes. In this paper, we propose a Scene Completion Pre-training (SCP) method to enhance the performance of 3D object detectors with less labeled data. SCP offers three key advantages: (1) Improved initialization of the point cloud model. By completing the scene point clouds, SCP effectively captures the spatial and semantic relationships among objects within urban environments. (2) Elimination of the need for additional datasets. SCP serves as a valuable auxiliary network that does not impose any additional efforts or data requirements on the 3D detectors. (3) Reduction of the amount of labeled data for detection. With the help of SCP, the existing state-of-the-art 3D detectors can achieve comparable performance while only relying on 20% labeled data.
26.Fast Sparse PCA via Positive Semidefinite Projection for Unsupervised Feature Selection
Authors:Junjing Zheng, Xinyu Zhang, Yongxiang Liu, Weidong Jiang, Kai Huo, Li Liu
Abstract: In the field of unsupervised feature selection, sparse principal component analysis (SPCA) methods have attracted more and more attention recently. Compared to spectral-based methods, SPCA methods don't rely on the construction of a similarity matrix and show better feature selection ability on real-world data. The original SPCA formulates a nonconvex optimization problem. Existing convex SPCA methods reformulate SPCA as a convex model by regarding the reconstruction matrix as an optimization variable. However, they are lack of constraints equivalent to the orthogonality restriction in SPCA, leading to larger solution space. In this paper, it's proved that the optimal solution to a convex SPCA model falls onto the Positive Semidefinite (PSD) cone. A standard convex SPCA-based model with PSD constraint for unsupervised feature selection is proposed. Further, a two-step fast optimization algorithm via PSD projection is presented to solve the proposed model. Two other existing convex SPCA-based models are also proven to have their solutions optimized on the PSD cone in this paper. Therefore, the PSD versions of these two models are proposed to accelerate their convergence as well. We also provide a regularization parameter setting strategy for our proposed method. Experiments on synthetic and real-world datasets demonstrate the effectiveness and efficiency of the proposed methods.
27.SGFeat: Salient Geometric Feature for Point Cloud Registration
Authors:Qianliang Wu, Yaqing Ding, Lei Luo, Chuanwei Zhou, Jin Xie, Jian Yang
Abstract: Point Cloud Registration (PCR) is a critical and challenging task in computer vision. One of the primary difficulties in PCR is identifying salient and meaningful points that exhibit consistent semantic and geometric properties across different scans. Previous methods have encountered challenges with ambiguous matching due to the similarity among patch blocks throughout the entire point cloud and the lack of consideration for efficient global geometric consistency. To address these issues, we propose a new framework that includes several novel techniques. Firstly, we introduce a semantic-aware geometric encoder that combines object-level and patch-level semantic information. This encoder significantly improves registration recall by reducing ambiguity in patch-level superpoint matching. Additionally, we incorporate a prior knowledge approach that utilizes an intrinsic shape signature to identify salient points. This enables us to extract the most salient super points and meaningful dense points in the scene. Secondly, we introduce an innovative transformer that encodes High-Order (HO) geometric features. These features are crucial for identifying salient points within initial overlap regions while considering global high-order geometric consistency. To optimize this high-order transformer further, we introduce an anchor node selection strategy. By encoding inter-frame triangle or polyhedron consistency features based on these anchor nodes, we can effectively learn high-order geometric features of salient super points. These high-order features are then propagated to dense points and utilized by a Sinkhorn matching module to identify key correspondences for successful registration. In our experiments conducted on well-known datasets such as 3DMatch/3DLoMatch and KITTI, our approach has shown promising results, highlighting the effectiveness of our novel method.
28.Human Action Co-occurrence in Lifestyle Vlogs using Graph Link Prediction
Authors:Oana Ignat, Santiago Castro, Weiji Li, Rada Mihalcea
Abstract: We introduce the task of automatic human action co-occurrence identification, i.e., determine whether two human actions can co-occur in the same interval of time. We create and make publicly available the ACE (Action Co-occurrencE) dataset, consisting of a large graph of ~12k co-occurring pairs of visual actions and their corresponding video clips. We describe graph link prediction models that leverage visual and textual information to automatically infer if two actions are co-occurring. We show that graphs are particularly well suited to capture relations between human actions, and the learned graph representations are effective for our task and capture novel and relevant information across different data domains. The ACE dataset and the code introduced in this paper are publicly available at https://github.com/MichiganNLP/vlog_action_co-occurrence.
29.Use neural networks to recognize students' handwritten letters and incorrect symbols
Authors:JiaJun Zhu, Zichuan Yang, Binjie Hong, Jiacheng Song, Jiwei Wang, Tianhao Chen, Shuilan Yang, Zixun Lan, Fei Ma
Abstract: Correcting students' multiple-choice answers is a repetitive and mechanical task that can be considered an image multi-classification task. Assuming possible options are 'abcd' and the correct option is one of the four, some students may write incorrect symbols or options that do not exist. In this paper, five classifications were set up - four for possible correct options and one for other incorrect writing. This approach takes into account the possibility of non-standard writing options.
30.Enhancing Multi-modal Cooperation via Fine-grained Modality Valuation
Authors:Yake Wei, Ruoxuan Feng, Zihe Wang, Di Hu
Abstract: One primary topic of multi-modal learning is to jointly incorporate heterogeneous information from different modalities. However, most models often suffer from unsatisfactory multi-modal cooperation, which could not jointly utilize all modalities well. Some methods are proposed to identify and enhance the worse learnt modality, but are often hard to provide the fine-grained observation of multi-modal cooperation at sample-level with theoretical support. Hence, it is essential to reasonably observe and improve the fine-grained cooperation between modalities, especially when facing realistic scenarios where the modality discrepancy could vary across different samples. To this end, we introduce a fine-grained modality valuation metric to evaluate the contribution of each modality at sample-level. Via modality valuation, we regretfully observe that the multi-modal model tends to rely on one specific modality, resulting in other modalities being low-contributing. We further analyze this issue and improve cooperation between modalities by enhancing the discriminative ability of low-contributing modalities in a targeted manner. Overall, our methods reasonably observe the fine-grained uni-modal contribution at sample-level and achieve considerable improvement on different multi-modal models.
31.Modality Unifying Network for Visible-Infrared Person Re-Identification
Authors:Hao Yu, Xu Cheng, Wei Peng, Weihao Liu, Guoying Zhao
Abstract: Visible-infrared person re-identification (VI-ReID) is a challenging task due to large cross-modality discrepancies and intra-class variations. Existing methods mainly focus on learning modality-shared representations by embedding different modalities into the same feature space. As a result, the learned feature emphasizes the common patterns across modalities while suppressing modality-specific and identity-aware information that is valuable for Re-ID. To address these issues, we propose a novel Modality Unifying Network (MUN) to explore a robust auxiliary modality for VI-ReID. First, the auxiliary modality is generated by combining the proposed cross-modality learner and intra-modality learner, which can dynamically model the modality-specific and modality-shared representations to alleviate both cross-modality and intra-modality variations. Second, by aligning identity centres across the three modalities, an identity alignment loss function is proposed to discover the discriminative feature representations. Third, a modality alignment loss is introduced to consistently reduce the distribution distance of visible and infrared images by modality prototype modeling. Extensive experiments on multiple public datasets demonstrate that the proposed method surpasses the current state-of-the-art methods by a significant margin.
32.OTAS: Unsupervised Boundary Detection for Object-Centric Temporal Action Segmentation
Authors:Yuerong Li, Zhengrong Xue, Huazhe Xu
Abstract: Temporal action segmentation is typically achieved by discovering the dramatic variances in global visual descriptors. In this paper, we explore the merits of local features by proposing the unsupervised framework of Object-centric Temporal Action Segmentation (OTAS). Broadly speaking, OTAS consists of self-supervised global and local feature extraction modules as well as a boundary selection module that fuses the features and detects salient boundaries for action segmentation. As a second contribution, we discuss the pros and cons of existing frame-level and boundary-level evaluation metrics. Through extensive experiments, we find OTAS is superior to the previous state-of-the-art method by $41\%$ on average in terms of our recommended F1 score. Surprisingly, OTAS even outperforms the ground-truth human annotations in the user study. Moreover, OTAS is efficient enough to allow real-time inference.
33.IBAFormer: Intra-batch Attention Transformer for Domain Generalized Semantic Segmentation
Authors:Qiyu Sun, Huilin Chen, Meng Zheng, Ziyan Wu, Michael Felsberg, Yang Tang
Abstract: Domain generalized semantic segmentation (DGSS) is a critical yet challenging task, where the model is trained only on source data without access to any target data. Despite the proposal of numerous DGSS strategies, the generalization capability remains limited in CNN architectures. Though some Transformer-based segmentation models show promising performance, they primarily focus on capturing intra-sample attentive relationships, disregarding inter-sample correlations which can potentially benefit DGSS. To this end, we enhance the attention modules in Transformer networks for improving DGSS by incorporating information from other independent samples in the same batch, enriching contextual information, and diversifying the training data for each attention block. Specifically, we propose two alternative intra-batch attention mechanisms, namely mean-based intra-batch attention (MIBA) and element-wise intra-batch attention (EIBA), to capture correlations between different samples, enhancing feature representation and generalization capabilities. Building upon intra-batch attention, we introduce IBAFormer, which integrates self-attention modules with the proposed intra-batch attention for DGSS. Extensive experiments demonstrate that IBAFormer achieves SOTA performance in DGSS, and ablation studies further confirm the effectiveness of each introduced component.
34.Fg-T2M: Fine-Grained Text-Driven Human Motion Generation via Diffusion Model
Authors:Yin Wang, Zhiying Leng, Frederick W. B. Li, Shun-Cheng Wu, Xiaohui Liang
Abstract: Text-driven human motion generation in computer vision is both significant and challenging. However, current methods are limited to producing either deterministic or imprecise motion sequences, failing to effectively control the temporal and spatial relationships required to conform to a given text description. In this work, we propose a fine-grained method for generating high-quality, conditional human motion sequences supporting precise text description. Our approach consists of two key components: 1) a linguistics-structure assisted module that constructs accurate and complete language feature to fully utilize text information; and 2) a context-aware progressive reasoning module that learns neighborhood and overall semantic linguistics features from shallow and deep graph neural networks to achieve a multi-step inference. Experiments show that our approach outperforms text-driven motion generation methods on HumanML3D and KIT test sets and generates better visually confirmed motion to the text conditions.
35.Jersey Number Recognition using Keyframe Identification from Low-Resolution Broadcast Videos
Authors:Bavesh Balaji, Jerrin Bright, Harish Prakash, Yuhao Chen, David A Clausi, John Zelek
Abstract: Player identification is a crucial component in vision-driven soccer analytics, enabling various downstream tasks such as player assessment, in-game analysis, and broadcast production. However, automatically detecting jersey numbers from player tracklets in videos presents challenges due to motion blur, low resolution, distortions, and occlusions. Existing methods, utilizing Spatial Transformer Networks, CNNs, and Vision Transformers, have shown success in image data but struggle with real-world video data, where jersey numbers are not visible in most of the frames. Hence, identifying frames that contain the jersey number is a key sub-problem to tackle. To address these issues, we propose a robust keyframe identification module that extracts frames containing essential high-level information about the jersey number. A spatio-temporal network is then employed to model spatial and temporal context and predict the probabilities of jersey numbers in the video. Additionally, we adopt a multi-task loss function to predict the probability distribution of each digit separately. Extensive evaluations on the SoccerNet dataset demonstrate that incorporating our proposed keyframe identification module results in a significant 37.81% and 37.70% increase in the accuracies of 2 different test sets with domain gaps. These results highlight the effectiveness and importance of our approach in tackling the challenges of automatic jersey number detection in sports videos.
36.Self-Training and Multi-Task Learning for Limited Data: Evaluation Study on Object Detection
Authors:Hoàng-Ân Lê, Minh-Tan Pham
Abstract: Self-training allows a network to learn from the predictions of a more complicated model, thus often requires well-trained teacher models and mixture of teacher-student data while multi-task learning jointly optimizes different targets to learn salient interrelationship and requires multi-task annotations for each training example. These frameworks, despite being particularly data demanding have potentials for data exploitation if such assumptions can be relaxed. In this paper, we compare self-training object detection under the deficiency of teacher training data where students are trained on unseen examples by the teacher, and multi-task learning with partially annotated data, i.e. single-task annotation per training example. Both scenarios have their own limitation but potentially helpful with limited annotated data. Experimental results show the improvement of performance when using a weak teacher with unseen data for training a multi-task student. Despite the limited setup we believe the experimental results show the potential of multi-task knowledge distillation and self-training, which could be beneficial for future study. Source code is at https://lhoangan.github.io/multas.
37.Towards High-Quality Specular Highlight Removal by Leveraging Large-Scale Synthetic Data
Authors:Gang Fu, Qing Zhang, Lei Zhu, Chunxia Xiao, Ping Li
Abstract: This paper aims to remove specular highlights from a single object-level image. Although previous methods have made some progresses, their performance remains somewhat limited, particularly for real images with complex specular highlights. To this end, we propose a three-stage network to address them. Specifically, given an input image, we first decompose it into the albedo, shading, and specular residue components to estimate a coarse specular-free image. Then, we further refine the coarse result to alleviate its visual artifacts such as color distortion. Finally, we adjust the tone of the refined result to match that of the input as closely as possible. In addition, to facilitate network training and quantitative evaluation, we present a large-scale synthetic dataset of object-level images, covering diverse objects and illumination conditions. Extensive experiments illustrate that our network is able to generalize well to unseen real object-level images, and even produce good results for scene-level images with multiple background objects and complex lighting.
38.AI4Food-NutritionFW: A Novel Framework for the Automatic Synthesis and Analysis of Eating Behaviours
Authors:Sergio Romero-Tapiador, Ruben Tolosana, Aythami Morales, Isabel Espinosa-Salinas, Gala Freixer, Julian Fierrez, Ruben Vera-Rodriguez, Enrique Carrillo de Santa Pau, Ana Ramírez de Molina, Javier Ortega-Garcia
Abstract: Nowadays millions of images are shared on social media and web platforms. In particular, many of them are food images taken from a smartphone over time, providing information related to the individual's diet. On the other hand, eating behaviours are directly related to some of the most prevalent diseases in the world. Exploiting recent advances in image processing and Artificial Intelligence (AI), this scenario represents an excellent opportunity to: i) create new methods that analyse the individuals' health from what they eat, and ii) develop personalised recommendations to improve nutrition and diet under specific circumstances (e.g., obesity or COVID). Having tunable tools for creating food image datasets that facilitate research in both lines is very much needed. This paper proposes AI4Food-NutritionFW, a framework for the creation of food image datasets according to configurable eating behaviours. AI4Food-NutritionFW simulates a user-friendly and widespread scenario where images are taken using a smartphone. In addition to the framework, we also provide and describe a unique food image dataset that includes 4,800 different weekly eating behaviours from 15 different profiles and 1,200 subjects. Specifically, we consider profiles that comply with actual lifestyles from healthy eating behaviours (according to established knowledge), variable profiles (e.g., eating out, holidays), to unhealthy ones (e.g., excess of fast food or sweets). Finally, we automatically evaluate a healthy index of the subject's eating behaviours using multidimensional metrics based on guidelines for healthy diets proposed by international organisations, achieving promising results (99.53% and 99.60% accuracy and sensitivity, respectively). We also release to the research community a software implementation of our proposed AI4Food-NutritionFW and the mentioned food image dataset created with it.
39.Semantic and Articulated Pedestrian Sensing Onboard a Moving Vehicle
Authors:Maria Priisalu
Abstract: It is difficult to perform 3D reconstruction from on-vehicle gathered video due to the large forward motion of the vehicle. Even object detection and human sensing models perform significantly worse on onboard videos when compared to standard benchmarks because objects often appear far away from the camera compared to the standard object detection benchmarks, image quality is often decreased by motion blur and occlusions occur often. This has led to the popularisation of traffic data-specific benchmarks. Recently Light Detection And Ranging (LiDAR) sensors have become popular to directly estimate depths without the need to perform 3D reconstructions. However, LiDAR-based methods still lack in articulated human detection at a distance when compared to image-based methods. We hypothesize that benchmarks targeted at articulated human sensing from LiDAR data could bring about increased research in human sensing and prediction in traffic and could lead to improved traffic safety for pedestrians.
40.SAMPLING: Scene-adaptive Hierarchical Multiplane Images Representation for Novel View Synthesis from a Single Image
Authors:Xiaoyu Zhou, Zhiwei Lin, Xiaojun Shan, Yongtao Wang, Deqing Sun, Ming-Hsuan Yang
Abstract: Recent novel view synthesis methods obtain promising results for relatively small scenes, e.g., indoor environments and scenes with a few objects, but tend to fail for unbounded outdoor scenes with a single image as input. In this paper, we introduce SAMPLING, a Scene-adaptive Hierarchical Multiplane Images Representation for Novel View Synthesis from a Single Image based on improved multiplane images (MPI). Observing that depth distribution varies significantly for unbounded outdoor scenes, we employ an adaptive-bins strategy for MPI to arrange planes in accordance with each scene image. To represent intricate geometry and multi-scale details, we further introduce a hierarchical refinement branch, which results in high-quality synthesized novel views. Our method demonstrates considerable performance gains in synthesizing large-scale unbounded outdoor scenes using a single image on the KITTI dataset and generalizes well to the unseen Tanks and Temples dataset. The code and models will be made public.
41.Grounded Language Acquisition From Object and Action Imagery
Authors:James Robert Kubricht, Zhaoyuan Yang, Jianwei Qiu, Peter Henry Tu
Abstract: Deep learning approaches to natural language processing have made great strides in recent years. While these models produce symbols that convey vast amounts of diverse knowledge, it is unclear how such symbols are grounded in data from the world. In this paper, we explore the development of a private language for visual data representation by training emergent language (EL) encoders/decoders in both i) a traditional referential game environment and ii) a contrastive learning environment utilizing a within-class matching training paradigm. An additional classification layer utilizing neural machine translation and random forest classification was used to transform symbolic representations (sequences of integer symbols) to class labels. These methods were applied in two experiments focusing on object recognition and action recognition. For object recognition, a set of sketches produced by human participants from real imagery was used (Sketchy dataset) and for action recognition, 2D trajectories were generated from 3D motion capture systems (MOVI dataset). In order to interpret the symbols produced for data in each experiment, gradient-weighted class activation mapping (Grad-CAM) methods were used to identify pixel regions indicating semantic features which contribute evidence towards symbols in learned languages. Additionally, a t-distributed stochastic neighbor embedding (t-SNE) method was used to investigate embeddings learned by CNN feature extractors.
42.Exploring Flat Minima for Domain Generalization with Large Learning Rates
Authors:Jian Zhang, Lei Qi, Yinghuan Shi, Yang Gao
Abstract: Domain Generalization (DG) aims to generalize to arbitrary unseen domains. A promising approach to improve model generalization in DG is the identification of flat minima. One typical method for this task is SWAD, which involves averaging weights along the training trajectory. However, the success of weight averaging depends on the diversity of weights, which is limited when training with a small learning rate. Instead, we observe that leveraging a large learning rate can simultaneously promote weight diversity and facilitate the identification of flat regions in the loss landscape. However, employing a large learning rate suffers from the convergence problem, which cannot be resolved by simply averaging the training weights. To address this issue, we introduce a training strategy called Lookahead which involves the weight interpolation, instead of average, between fast and slow weights. The fast weight explores the weight space with a large learning rate, which is not converged while the slow weight interpolates with it to ensure the convergence. Besides, weight interpolation also helps identify flat minima by implicitly optimizing the local entropy loss that measures flatness. To further prevent overfitting during training, we propose two variants to regularize the training weight with weighted averaged weight or with accumulated history weight. Taking advantage of this new perspective, our methods achieve state-of-the-art performance on both classification and semantic segmentation domain generalization benchmarks. The code is available at https://github.com/koncle/DG-with-Large-LR.
43.Padding-free Convolution based on Preservation of Differential Characteristics of Kernels
Authors:Kuangdai Leng, Jeyan Thiyagalingam
Abstract: Convolution is a fundamental operation in image processing and machine learning. Aimed primarily at maintaining image size, padding is a key ingredient of convolution, which, however, can introduce undesirable boundary effects. We present a non-padding-based method for size-keeping convolution based on the preservation of differential characteristics of kernels. The main idea is to make convolution over an incomplete sliding window "collapse" to a linear differential operator evaluated locally at its central pixel, which no longer requires information from the neighbouring missing pixels. While the underlying theory is rigorous, our final formula turns out to be simple: the convolution over an incomplete window is achieved by convolving its nearest complete window with a transformed kernel. This formula is computationally lightweight, involving neither interpolation or extrapolation nor restrictions on image and kernel sizes. Our method favours data with smooth boundaries, such as high-resolution images and fields from physics. Our experiments include: i) filtering analytical and non-analytical fields from computational physics and, ii) training convolutional neural networks (CNNs) for the tasks of image classification, semantic segmentation and super-resolution reconstruction. In all these experiments, our method has exhibited visible superiority over the compared ones.
44.Exploring Non-additive Randomness on ViT against Query-Based Black-Box Attacks
Authors:Jindong Gu, Fangyun Wei, Philip Torr, Han Hu
Abstract: Deep Neural Networks can be easily fooled by small and imperceptible perturbations. The query-based black-box attack (QBBA) is able to create the perturbations using model output probabilities of image queries requiring no access to the underlying models. QBBA poses realistic threats to real-world applications. Recently, various types of robustness have been explored to defend against QBBA. In this work, we first taxonomize the stochastic defense strategies against QBBA. Following our taxonomy, we propose to explore non-additive randomness in models to defend against QBBA. Specifically, we focus on underexplored Vision Transformers based on their flexible architectures. Extensive experiments show that the proposed defense approach achieves effective defense, without much sacrifice in performance.
45.Attention De-sparsification Matters: Inducing Diversity in Digital Pathology Representation Learning
Authors:Saarthak Kapse, Srijan Das, Jingwei Zhang, Rajarsi R. Gupta, Joel Saltz, Dimitris Samaras, Prateek Prasanna
Abstract: We propose DiRL, a Diversity-inducing Representation Learning technique for histopathology imaging. Self-supervised learning techniques, such as contrastive and non-contrastive approaches, have been shown to learn rich and effective representations of digitized tissue samples with limited pathologist supervision. Our analysis of vanilla SSL-pretrained models' attention distribution reveals an insightful observation: sparsity in attention, i.e, models tends to localize most of their attention to some prominent patterns in the image. Although attention sparsity can be beneficial in natural images due to these prominent patterns being the object of interest itself, this can be sub-optimal in digital pathology; this is because, unlike natural images, digital pathology scans are not object-centric, but rather a complex phenotype of various spatially intermixed biological components. Inadequate diversification of attention in these complex images could result in crucial information loss. To address this, we leverage cell segmentation to densely extract multiple histopathology-specific representations, and then propose a prior-guided dense pretext task for SSL, designed to match the multiple corresponding representations between the views. Through this, the model learns to attend to various components more closely and evenly, thus inducing adequate diversification in attention for capturing context rich representations. Through quantitative and qualitative analysis on multiple tasks across cancer types, we demonstrate the efficacy of our method and observe that the attention is more globally distributed.
46.Learning Disentangled Avatars with Hybrid 3D Representations
Authors:Yao Feng, Weiyang Liu, Timo Bolkart, Jinlong Yang, Marc Pollefeys, Michael J. Black
Abstract: Tremendous efforts have been made to learn animatable and photorealistic human avatars. Towards this end, both explicit and implicit 3D representations are heavily studied for a holistic modeling and capture of the whole human (e.g., body, clothing, face and hair), but neither representation is an optimal choice in terms of representation efficacy since different parts of the human avatar have different modeling desiderata. For example, meshes are generally not suitable for modeling clothing and hair. Motivated by this, we present Disentangled Avatars~(DELTA), which models humans with hybrid explicit-implicit 3D representations. DELTA takes a monocular RGB video as input, and produces a human avatar with separate body and clothing/hair layers. Specifically, we demonstrate two important applications for DELTA. For the first one, we consider the disentanglement of the human body and clothing and in the second, we disentangle the face and hair. To do so, DELTA represents the body or face with an explicit mesh-based parametric 3D model and the clothing or hair with an implicit neural radiance field. To make this possible, we design an end-to-end differentiable renderer that integrates meshes into volumetric rendering, enabling DELTA to learn directly from monocular videos without any 3D supervision. Finally, we show that how these two applications can be easily combined to model full-body avatars, such that the hair, face, body and clothing can be fully disentangled yet jointly rendered. Such a disentanglement enables hair and clothing transfer to arbitrary body shapes. We empirically validate the effectiveness of DELTA's disentanglement by demonstrating its promising performance on disentangled reconstruction, virtual clothing try-on and hairstyle transfer. To facilitate future research, we also release an open-sourced pipeline for the study of hybrid human avatar modeling.