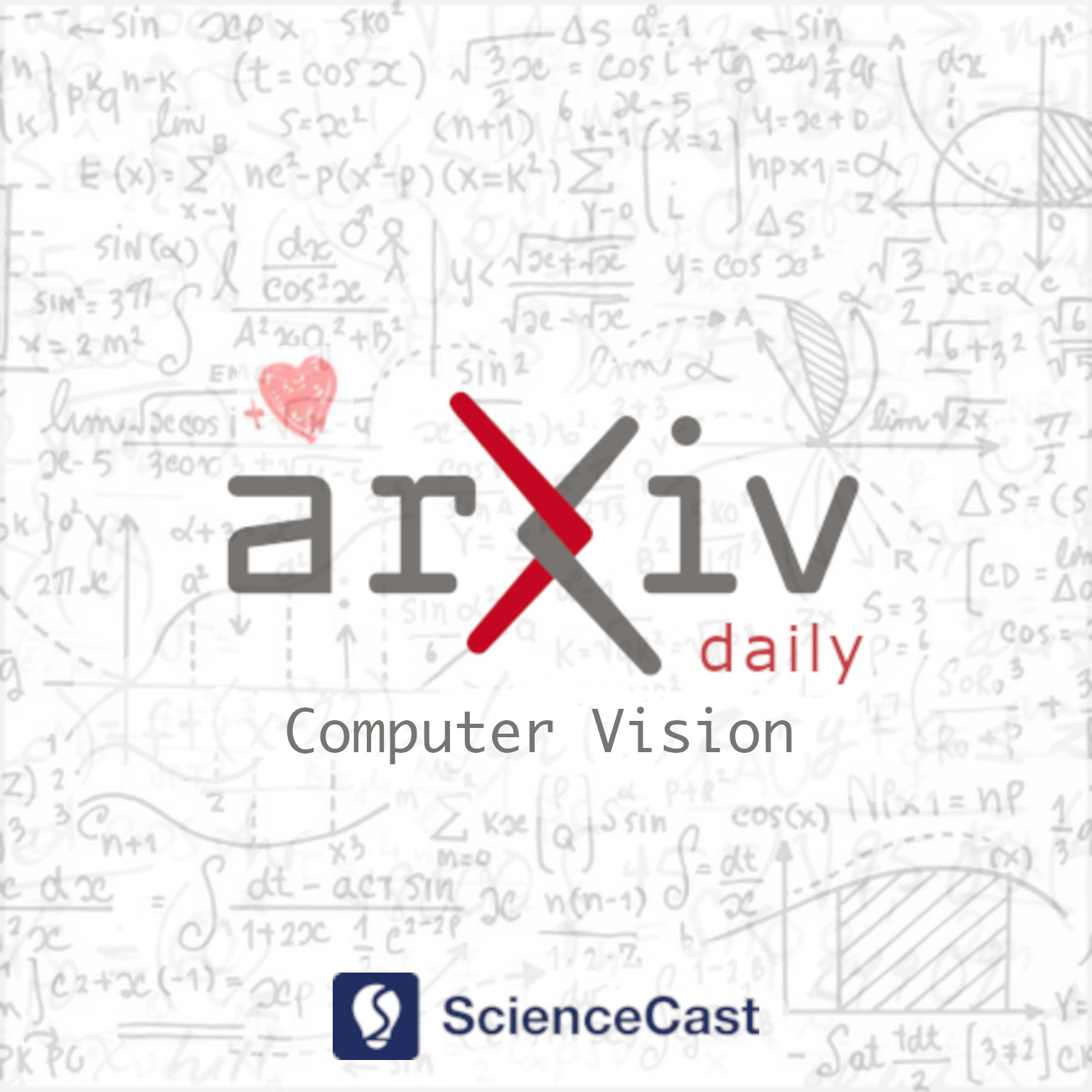
Computer Vision and Pattern Recognition (cs.CV)
Thu, 10 Aug 2023
1.TrainFors: A Large Benchmark Training Dataset for Image Manipulation Detection and Localization
Authors:Soumyaroop Nandi, Prem Natarajan, Wael Abd-Almageed
Abstract: The evaluation datasets and metrics for image manipulation detection and localization (IMDL) research have been standardized. But the training dataset for such a task is still nonstandard. Previous researchers have used unconventional and deviating datasets to train neural networks for detecting image forgeries and localizing pixel maps of manipulated regions. For a fair comparison, the training set, test set, and evaluation metrics should be persistent. Hence, comparing the existing methods may not seem fair as the results depend heavily on the training datasets as well as the model architecture. Moreover, none of the previous works release the synthetic training dataset used for the IMDL task. We propose a standardized benchmark training dataset for image splicing, copy-move forgery, removal forgery, and image enhancement forgery. Furthermore, we identify the problems with the existing IMDL datasets and propose the required modifications. We also train the state-of-the-art IMDL methods on our proposed TrainFors1 dataset for a fair evaluation and report the actual performance of these methods under similar conditions.
2.Local-Global Information Interaction Debiasing for Dynamic Scene Graph Generation
Authors:Xinyu Lyu, Jingwei Liu, Yuyu Guo, Lianli Gao
Abstract: The task of dynamic scene graph generation (DynSGG) aims to generate scene graphs for given videos, which involves modeling the spatial-temporal information in the video. However, due to the long-tailed distribution of samples in the dataset, previous DynSGG models fail to predict the tail predicates. We argue that this phenomenon is due to previous methods that only pay attention to the local spatial-temporal information and neglect the consistency of multiple frames. To solve this problem, we propose a novel DynSGG model based on multi-task learning, DynSGG-MTL, which introduces the local interaction information and global human-action interaction information. The interaction between objects and frame features makes the model more fully understand the visual context of the single image. Long-temporal human actions supervise the model to generate multiple scene graphs that conform to the global constraints and avoid the model being unable to learn the tail predicates. Extensive experiments on Action Genome dataset demonstrate the efficacy of our proposed framework, which not only improves the dynamic scene graph generation but also alleviates the long-tail problem.
3.Informative Scene Graph Generation via Debiasing
Authors:Lianli Gao, Xinyu Lyu, Yuyu Guo, Yuxuan Hu, Yuan-Fang Li, Lu Xu, Heng Tao Shen, Jingkuan Song
Abstract: Scene graph generation aims to detect visual relationship triplets, (subject, predicate, object). Due to biases in data, current models tend to predict common predicates, e.g. "on" and "at", instead of informative ones, e.g. "standing on" and "looking at". This tendency results in the loss of precise information and overall performance. If a model only uses "stone on road" rather than "stone blocking road" to describe an image, it may be a grave misunderstanding. We argue that this phenomenon is caused by two imbalances: semantic space level imbalance and training sample level imbalance. For this problem, we propose DB-SGG, an effective framework based on debiasing but not the conventional distribution fitting. It integrates two components: Semantic Debiasing (SD) and Balanced Predicate Learning (BPL), for these imbalances. SD utilizes a confusion matrix and a bipartite graph to construct predicate relationships. BPL adopts a random undersampling strategy and an ambiguity removing strategy to focus on informative predicates. Benefiting from the model-agnostic process, our method can be easily applied to SGG models and outperforms Transformer by 136.3%, 119.5%, and 122.6% on mR@20 at three SGG sub-tasks on the SGG-VG dataset. Our method is further verified on another complex SGG dataset (SGG-GQA) and two downstream tasks (sentence-to-graph retrieval and image captioning).
4.Double-chain Constraints for 3D Human Pose Estimation in Images and Videos
Authors:Hongbo Kang, Yong Wang, Mengyuan Liu, Doudou Wu, Peng Liu, Wenming Yang
Abstract: Reconstructing 3D poses from 2D poses lacking depth information is particularly challenging due to the complexity and diversity of human motion. The key is to effectively model the spatial constraints between joints to leverage their inherent dependencies. Thus, we propose a novel model, called Double-chain Graph Convolutional Transformer (DC-GCT), to constrain the pose through a double-chain design consisting of local-to-global and global-to-local chains to obtain a complex representation more suitable for the current human pose. Specifically, we combine the advantages of GCN and Transformer and design a Local Constraint Module (LCM) based on GCN and a Global Constraint Module (GCM) based on self-attention mechanism as well as a Feature Interaction Module (FIM). The proposed method fully captures the multi-level dependencies between human body joints to optimize the modeling capability of the model. Moreover, we propose a method to use temporal information into the single-frame model by guiding the video sequence embedding through the joint embedding of the target frame, with negligible increase in computational cost. Experimental results demonstrate that DC-GCT achieves state-of-the-art performance on two challenging datasets (Human3.6M and MPI-INF-3DHP). Notably, our model achieves state-of-the-art performance on all action categories in the Human3.6M dataset using detected 2D poses from CPN, and our code is available at: https://github.com/KHB1698/DC-GCT.
5.DAOT: Domain-Agnostically Aligned Optimal Transport for Domain-Adaptive Crowd Counting
Authors:Huilin Zhu, Jingling Yuan, Xian Zhong, Zhengwei Yang, Zheng Wang, Shengfeng He
Abstract: Domain adaptation is commonly employed in crowd counting to bridge the domain gaps between different datasets. However, existing domain adaptation methods tend to focus on inter-dataset differences while overlooking the intra-differences within the same dataset, leading to additional learning ambiguities. These domain-agnostic factors, e.g., density, surveillance perspective, and scale, can cause significant in-domain variations, and the misalignment of these factors across domains can lead to a drop in performance in cross-domain crowd counting. To address this issue, we propose a Domain-agnostically Aligned Optimal Transport (DAOT) strategy that aligns domain-agnostic factors between domains. The DAOT consists of three steps. First, individual-level differences in domain-agnostic factors are measured using structural similarity (SSIM). Second, the optimal transfer (OT) strategy is employed to smooth out these differences and find the optimal domain-to-domain misalignment, with outlier individuals removed via a virtual "dustbin" column. Third, knowledge is transferred based on the aligned domain-agnostic factors, and the model is retrained for domain adaptation to bridge the gap across domains. We conduct extensive experiments on five standard crowd-counting benchmarks and demonstrate that the proposed method has strong generalizability across diverse datasets. Our code will be available at: https://github.com/HopooLinZ/DAOT/.
6.Deep Semantic Graph Matching for Large-scale Outdoor Point Clouds Registration
Authors:Shaocong Liu, Tao Wang, Yan Zhang, Ruqin Zhou, Li Li, Chenguang Dai, Yongsheng Zhang, Hanyun Wang
Abstract: The current point cloud registration methods are mainly based on geometric information and usually ignore the semantic information in the point clouds. In this paper, we treat the point cloud registration problem as semantic instance matching and registration task, and propose a deep semantic graph matching method for large-scale outdoor point cloud registration. Firstly, the semantic category labels of 3D point clouds are obtained by utilizing large-scale point cloud semantic segmentation network. The adjacent points with the same category labels are then clustered together by using Euclidean clustering algorithm to obtain the semantic instances. Secondly, the semantic adjacency graph is constructed based on the spatial adjacency relation of semantic instances. Three kinds of high-dimensional features including geometric shape features, semantic categorical features and spatial distribution features are learned through graph convolutional network, and enhanced based on attention mechanism. Thirdly, the semantic instance matching problem is modeled as an optimal transport problem, and solved through an optimal matching layer. Finally, according to the matched semantic instances, the geometric transformation matrix between two point clouds is first obtained by SVD algorithm and then refined by ICP algorithm. The experiments are cconducted on the KITTI Odometry dataset, and the average relative translation error and average relative rotation error of the proposed method are 6.6cm and 0.229{\deg} respectively.
7.RLSAC: Reinforcement Learning enhanced Sample Consensus for End-to-End Robust Estimation
Authors:Chang Nie, Guangming Wang, Zhe Liu, Luca Cavalli, Marc Pollefeys, Hesheng Wang
Abstract: Robust estimation is a crucial and still challenging task, which involves estimating model parameters in noisy environments. Although conventional sampling consensus-based algorithms sample several times to achieve robustness, these algorithms cannot use data features and historical information effectively. In this paper, we propose RLSAC, a novel Reinforcement Learning enhanced SAmple Consensus framework for end-to-end robust estimation. RLSAC employs a graph neural network to utilize both data and memory features to guide exploring directions for sampling the next minimum set. The feedback of downstream tasks serves as the reward for unsupervised training. Therefore, RLSAC can avoid differentiating to learn the features and the feedback of downstream tasks for end-to-end robust estimation. In addition, RLSAC integrates a state transition module that encodes both data and memory features. Our experimental results demonstrate that RLSAC can learn from features to gradually explore a better hypothesis. Through analysis, it is apparent that RLSAC can be easily transferred to other sampling consensus-based robust estimation tasks. To the best of our knowledge, RLSAC is also the first method that uses reinforcement learning to sample consensus for end-to-end robust estimation. We release our codes at https://github.com/IRMVLab/RLSAC.
8.Adv-Inpainting: Generating Natural and Transferable Adversarial Patch via Attention-guided Feature Fusion
Authors:Yanjie Li, Mingxing Duan, Bin Xiao
Abstract: The rudimentary adversarial attacks utilize additive noise to attack facial recognition (FR) models. However, because manipulating the total face is impractical in the physical setting, most real-world FR attacks are based on adversarial patches, which limit perturbations to a small area. Previous adversarial patch attacks often resulted in unnatural patterns and clear boundaries that were easily noticeable. In this paper, we argue that generating adversarial patches with plausible content can result in stronger transferability than using additive noise or directly sampling from the latent space. To generate natural-looking and highly transferable adversarial patches, we propose an innovative two-stage coarse-to-fine attack framework called Adv-Inpainting. In the first stage, we propose an attention-guided StyleGAN (Att-StyleGAN) that adaptively combines texture and identity features based on the attention map to generate high-transferable and natural adversarial patches. In the second stage, we design a refinement network with a new boundary variance loss to further improve the coherence between the patch and its surrounding area. Experiment results demonstrate that Adv-Inpainting is stealthy and can produce adversarial patches with stronger transferability and improved visual quality than previous adversarial patch attacks.