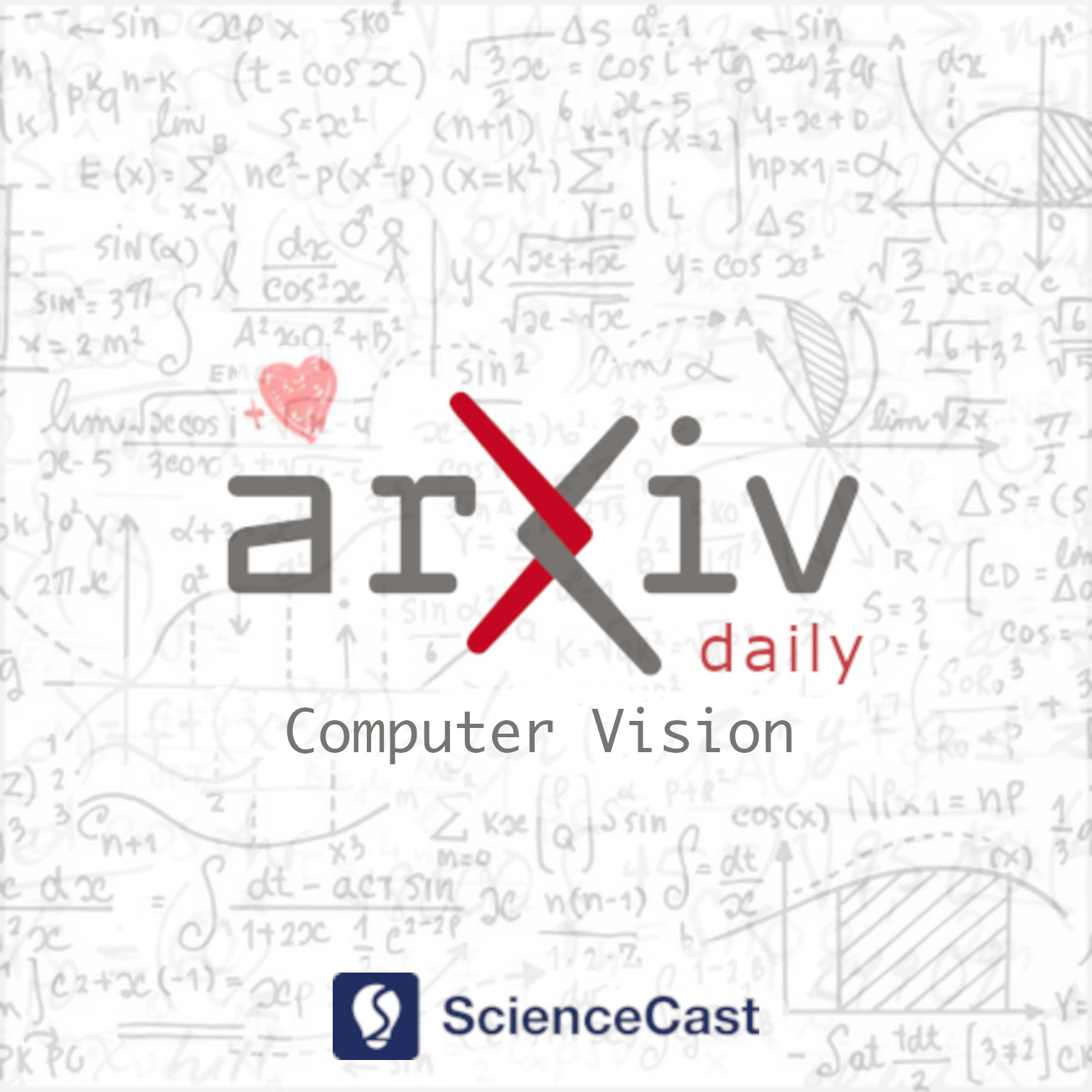
Computer Vision and Pattern Recognition (cs.CV)
Wed, 07 Jun 2023
1.BAA-NGP: Bundle-Adjusting Accelerated Neural Graphics Primitives
Authors:Sainan Liu, Shan Lin, Jingpei Lu, Shreya Saha, Alexey Supikov, Michael Yip
Abstract: Implicit neural representation has emerged as a powerful method for reconstructing 3D scenes from 2D images. Given a set of camera poses and associated images, the models can be trained to synthesize novel, unseen views. In order to expand the use cases for implicit neural representations, we need to incorporate camera pose estimation capabilities as part of the representation learning, as this is necessary for reconstructing scenes from real-world video sequences where cameras are generally not being tracked. Existing approaches like COLMAP and, most recently, bundle-adjusting neural radiance field methods often suffer from lengthy processing times. These delays ranging from hours to days, arise from laborious feature matching, hardware limitations, dense point sampling, and long training times required by a multi-layer perceptron structure with a large number of parameters. To address these challenges, we propose a framework called bundle-adjusting accelerated neural graphics primitives (BAA-NGP). Our approach leverages accelerated sampling and hash encoding to expedite both pose refinement/estimation and 3D scene reconstruction. Experimental results demonstrate that our method achieves a more than 10 to 20 $\times$ speed improvement in novel view synthesis compared to other bundle-adjusting neural radiance field methods without sacrificing the quality of pose estimation.
2.ScoreCL: Augmentation-Adaptive Contrastive Learning via Score-Matching Function
Authors:JinYoung Kim, Soonwoo Kwon, Hyojun Go, Yunsung Lee, Seungtaek Choi
Abstract: Self-supervised contrastive learning (CL) has achieved state-of-the-art performance in representation learning by minimizing the distance between positive pairs while maximizing that of negative ones. Recently, it has been verified that the model learns better representation with diversely augmented positive pairs because they enable the model to be more view-invariant. However, only a few studies on CL have considered the difference between augmented views, and have not gone beyond the hand-crafted findings. In this paper, we first observe that the score-matching function can measure how much data has changed from the original through augmentation. With the observed property, every pair in CL can be weighted adaptively by the difference of score values, resulting in boosting the performance of the existing CL method. We show the generality of our method, referred to as ScoreCL, by consistently improving various CL methods, SimCLR, SimSiam, W-MSE, and VICReg, up to 3%p in k-NN evaluation on CIFAR-10, CIFAR-100, and ImageNet-100. Moreover, we have conducted exhaustive experiments and ablations, including results on diverse downstream tasks, comparison with possible baselines, and improvement when used with other proposed augmentation methods. We hope our exploration will inspire more research in exploiting the score matching for CL.
3.FusedRF: Fusing Multiple Radiance Fields
Authors:Rahul Goel, Dhawal Sirikonda, Rajvi Shah, PJ Narayanan
Abstract: Radiance Fields (RFs) have shown great potential to represent scenes from casually captured discrete views. Compositing parts or whole of multiple captured scenes could greatly interest several XR applications. Prior works can generate new views of such scenes by tracing each scene in parallel. This increases the render times and memory requirements with the number of components. In this work, we provide a method to create a single, compact, fused RF representation for a scene composited using multiple RFs. The fused RF has the same render times and memory utilizations as a single RF. Our method distills information from multiple teacher RFs into a single student RF while also facilitating further manipulations like addition and deletion into the fused representation.
4.StructuredMesh: 3D Structured Optimization of Façade Components on Photogrammetric Mesh Models using Binary Integer Programming
Authors:Libin Wang, Han Hu, Qisen Shang, Bo Xu, Qing Zhu
Abstract: The lack of fa\c{c}ade structures in photogrammetric mesh models renders them inadequate for meeting the demands of intricate applications. Moreover, these mesh models exhibit irregular surfaces with considerable geometric noise and texture quality imperfections, making the restoration of structures challenging. To address these shortcomings, we present StructuredMesh, a novel approach for reconstructing fa\c{c}ade structures conforming to the regularity of buildings within photogrammetric mesh models. Our method involves capturing multi-view color and depth images of the building model using a virtual camera and employing a deep learning object detection pipeline to semi-automatically extract the bounding boxes of fa\c{c}ade components such as windows, doors, and balconies from the color image. We then utilize the depth image to remap these boxes into 3D space, generating an initial fa\c{c}ade layout. Leveraging architectural knowledge, we apply binary integer programming (BIP) to optimize the 3D layout's structure, encompassing the positions, orientations, and sizes of all components. The refined layout subsequently informs fa\c{c}ade modeling through instance replacement. We conducted experiments utilizing building mesh models from three distinct datasets, demonstrating the adaptability, robustness, and noise resistance of our proposed methodology. Furthermore, our 3D layout evaluation metrics reveal that the optimized layout enhances precision, recall, and F-score by 6.5%, 4.5%, and 5.5%, respectively, in comparison to the initial layout.
5.MultiSum: A Dataset for Multimodal Summarization and Thumbnail Generation of Videos
Authors:Jielin Qiu, Jiacheng Zhu, William Han, Aditesh Kumar, Karthik Mittal, Claire Jin, Zhengyuan Yang, Linjie Li, Jianfeng Wang, Bo Li, Ding Zhao, Lijuan Wang
Abstract: Multimodal summarization with multimodal output (MSMO) has emerged as a promising research direction. Nonetheless, numerous limitations exist within existing public MSMO datasets, including insufficient upkeep, data inaccessibility, limited size, and the absence of proper categorization, which pose significant challenges to effective research. To address these challenges and provide a comprehensive dataset for this new direction, we have meticulously curated the MultiSum dataset. Our new dataset features (1) Human-validated summaries for both video and textual content, providing superior human instruction and labels for multimodal learning. (2) Comprehensively and meticulously arranged categorization, spanning 17 principal categories and 170 subcategories to encapsulate a diverse array of real-world scenarios. (3) Benchmark tests performed on the proposed dataset to assess varied tasks and methods, including video temporal segmentation, video summarization, text summarization, and multimodal summarization. To champion accessibility and collaboration, we release the MultiSum dataset and the data collection tool as fully open-source resources, fostering transparency and accelerating future developments. Our project website can be found at https://multisum-dataset.github.io/.
6.Efficient Vision Transformer for Human Pose Estimation via Patch Selection
Authors:Kaleab A. Kinfu, René Vidal
Abstract: While Convolutional Neural Networks (CNNs) have been widely successful in 2D human pose estimation, Vision Transformers (ViTs) have emerged as a promising alternative to CNNs, boosting state-of-the-art performance. However, the quadratic computational complexity of ViTs has limited their applicability for processing high-resolution images and long videos. To address this challenge, we propose a simple method for reducing ViT's computational complexity based on selecting and processing a small number of most informative patches while disregarding others. We leverage a lightweight pose estimation network to guide the patch selection process, ensuring that the selected patches contain the most important information. Our experimental results on three widely used 2D pose estimation benchmarks, namely COCO, MPII and OCHuman, demonstrate the effectiveness of our proposed methods in significantly improving speed and reducing computational complexity with a slight drop in performance.
7.Learning Probabilistic Coordinate Fields for Robust Correspondences
Authors:Weiyue Zhao, Hao Lu, Xinyi Ye, Zhiguo Cao, Xin Li
Abstract: We introduce Probabilistic Coordinate Fields (PCFs), a novel geometric-invariant coordinate representation for image correspondence problems. In contrast to standard Cartesian coordinates, PCFs encode coordinates in correspondence-specific barycentric coordinate systems (BCS) with affine invariance. To know \textit{when and where to trust} the encoded coordinates, we implement PCFs in a probabilistic network termed PCF-Net, which parameterizes the distribution of coordinate fields as Gaussian mixture models. By jointly optimizing coordinate fields and their confidence conditioned on dense flows, PCF-Net can work with various feature descriptors when quantifying the reliability of PCFs by confidence maps. An interesting observation of this work is that the learned confidence map converges to geometrically coherent and semantically consistent regions, which facilitates robust coordinate representation. By delivering the confident coordinates to keypoint/feature descriptors, we show that PCF-Net can be used as a plug-in to existing correspondence-dependent approaches. Extensive experiments on both indoor and outdoor datasets suggest that accurate geometric invariant coordinates help to achieve the state of the art in several correspondence problems, such as sparse feature matching, dense image registration, camera pose estimation, and consistency filtering. Further, the interpretable confidence map predicted by PCF-Net can also be leveraged to other novel applications from texture transfer to multi-homography classification.
8.Flare7K++: Mixing Synthetic and Real Datasets for Nighttime Flare Removal and Beyond
Authors:Yuekun Dai, Chongyi Li, Shangchen Zhou, Ruicheng Feng, Yihang Luo, Chen Change Loy
Abstract: Artificial lights commonly leave strong lens flare artifacts on the images captured at night, degrading both the visual quality and performance of vision algorithms. Existing flare removal approaches mainly focus on removing daytime flares and fail in nighttime cases. Nighttime flare removal is challenging due to the unique luminance and spectrum of artificial lights, as well as the diverse patterns and image degradation of the flares. The scarcity of the nighttime flare removal dataset constraints the research on this crucial task. In this paper, we introduce Flare7K++, the first comprehensive nighttime flare removal dataset, consisting of 962 real-captured flare images (Flare-R) and 7,000 synthetic flares (Flare7K). Compared to Flare7K, Flare7K++ is particularly effective in eliminating complicated degradation around the light source, which is intractable by using synthetic flares alone. Besides, the previous flare removal pipeline relies on the manual threshold and blur kernel settings to extract light sources, which may fail when the light sources are tiny or not overexposed. To address this issue, we additionally provide the annotations of light sources in Flare7K++ and propose a new end-to-end pipeline to preserve the light source while removing lens flares. Our dataset and pipeline offer a valuable foundation and benchmark for future investigations into nighttime flare removal studies. Extensive experiments demonstrate that Flare7K++ supplements the diversity of existing flare datasets and pushes the frontier of nighttime flare removal towards real-world scenarios.
9.Randomized 3D Scene Generation for Generalizable Self-supervised Pre-training
Authors:Lanxiao Li, Michael Heizmann
Abstract: Capturing and labeling real-world 3D data is laborious and time-consuming, which makes it costly to train strong 3D models. To address this issue, previous works generate randomized 3D scenes and pre-train models on generated data. Although the pre-trained models gain promising performance boosts, previous works have two major shortcomings. First, they focus on only one downstream task (i.e., object detection). Second, a fair comparison of generated data is still lacking. In this work, we systematically compare data generation methods using a unified setup. To clarify the generalization of the pre-trained models, we evaluate their performance in multiple tasks (e.g., object detection and semantic segmentation) and with different pre-training methods (e.g., masked autoencoder and contrastive learning). Moreover, we propose a new method to generate 3D scenes with spherical harmonics. It surpasses the previous formula-driven method with a clear margin and achieves on-par results with methods using real-world scans and CAD models.
10.T-ADAF: Adaptive Data Augmentation Framework for Image Classification Network based on Tensor T-product Operator
Authors:Feiyang Han, Yun Miao, Zhaoyi Sun, Yimin Wei
Abstract: Image classification is one of the most fundamental tasks in Computer Vision. In practical applications, the datasets are usually not as abundant as those in the laboratory and simulation, which is always called as Data Hungry. How to extract the information of data more completely and effectively is very important. Therefore, an Adaptive Data Augmentation Framework based on the tensor T-product Operator is proposed in this paper, to triple one image data to be trained and gain the result from all these three images together with only less than 0.1% increase in the number of parameters. At the same time, this framework serves the functions of column image embedding and global feature intersection, enabling the model to obtain information in not only spatial but frequency domain, and thus improving the prediction accuracy of the model. Numerical experiments have been designed for several models, and the results demonstrate the effectiveness of this adaptive framework. Numerical experiments show that our data augmentation framework can improve the performance of original neural network model by 2%, which provides competitive results to state-of-the-art methods.
11.Coarse Is Better? A New Pipeline Towards Self-Supervised Learning with Uncurated Images
Authors:Ke Zhu, Yin-Yin He, Jianxin Wu
Abstract: Most self-supervised learning (SSL) methods often work on curated datasets where the object-centric assumption holds. This assumption breaks down in uncurated scene images. Existing scene image SSL methods try to find the two views from original scene images that are well matched or dense, which is both complex and computationally heavy. This paper proposes a conceptually different pipeline: first find regions that are coarse objects (with adequate objectness), crop them out as pseudo object-centric images, then any SSL method can be directly applied as in a real object-centric dataset. That is, cropping benefits scene images SSL. A novel cropping strategy is proposed to find coarse object regions in scene images. The proposed pipeline and cropping strategy successfully learn high quality visual representation from uncurated scene datasets like MS-COCO, and the learning does not rely on external object-centric datasets such as ImageNet. Experiments show that our pipeline outperforms existing SSL methods on scene images, and is friendly for non-contrastive SSL methods like MAE. Ablations further verify that the proposed cropping strategy does not rely on pretrained SSL models, too.
12.ColNav: Real-Time Colon Navigation for Colonoscopy
Authors:Netanel Frank, Erez Posner, Emmanuelle Muhlethaler, Adi Zholkover, Moshe Bouhnik
Abstract: Colorectal cancer screening through colonoscopy continues to be the dominant global standard, as it allows identifying pre-cancerous or adenomatous lesions and provides the ability to remove them during the procedure itself. Nevertheless, failure by the endoscopist to identify such lesions increases the likelihood of lesion progression to subsequent colorectal cancer. Ultimately, colonoscopy remains operator-dependent, and the wide range of quality in colonoscopy examinations among endoscopists is influenced by variations in their technique, training, and diligence. This paper presents a novel real-time navigation guidance system for Optical Colonoscopy (OC). Our proposed system employs a real-time approach that displays both an unfolded representation of the colon and a local indicator directing to un-inspected areas. These visualizations are presented to the physician during the procedure, providing actionable and comprehensible guidance to un-surveyed areas in real-time, while seamlessly integrating into the physician's workflow. Through coverage experimental evaluation, we demonstrated that our system resulted in a higher polyp recall (PR) and high inter-rater reliability with physicians for coverage prediction. These results suggest that our real-time navigation guidance system has the potential to improve the quality and effectiveness of Optical Colonoscopy and ultimately benefit patient outcomes.
13.On the Generalization of Multi-modal Contrastive Learning
Authors:Qi Zhang, Yifei Wang, Yisen Wang
Abstract: Multi-modal contrastive learning (MMCL) has recently garnered considerable interest due to its superior performance in visual tasks, achieved by embedding multi-modal data, such as visual-language pairs. However, there still lack theoretical understandings of how MMCL extracts useful visual representation from multi-modal pairs, and particularly, how MMCL outperforms previous approaches like self-supervised contrastive learning (SSCL). In this paper, by drawing an intrinsic connection between MMCL and asymmetric matrix factorization, we establish the first generalization guarantees of MMCL for visual downstream tasks. Based on this framework, we further unify MMCL and SSCL by showing that MMCL implicitly performs SSCL with (pseudo) positive pairs induced by text pairs. Through this unified perspective, we characterize the advantage of MMCL by showing that text pairs induce more semantically consistent and diverse positive pairs, which, according to our analysis, provably benefit downstream generalization. Inspired by this finding, we propose CLIP-guided resampling methods to significantly improve the downstream performance of SSCL on ImageNet by leveraging multi-modal information. Code is available at https://github.com/PKU-ML/CLIP-Help-SimCLR.
14.CorrMatch: Label Propagation via Correlation Matching for Semi-Supervised Semantic Segmentation
Authors:Boyuan Sun, Yuqi Yang, Le Zhang, Ming-Ming Cheng, Qibin Hou
Abstract: In this paper, we present a simple but performant semi-supervised semantic segmentation approach, termed CorrMatch. Our goal is to mine more high-quality regions from the unlabeled images to leverage the unlabeled data more efficiently via consistency regularization. The key contributions of our CorrMatch are two novel and complementary strategies. First, we introduce an adaptive threshold updating strategy with a relaxed initialization to expand the high-quality regions. Furthermore, we propose to propagate high-confidence predictions through measuring the pairwise similarities between pixels. Despite its simplicity, we show that CorrMatch achieves great performance on popular semi-supervised semantic segmentation benchmarks. Taking the DeepLabV3+ framework with ResNet-101 backbone as our segmentation model, we receive a 76%+ mIoU score on the Pascal VOC 2012 segmentation benchmark with only 92 annotated images provided. We also achieve a consistent improvement over previous semi-supervised semantic segmentation models. Code will be made publicly available.
15.ViDA: Homeostatic Visual Domain Adapter for Continual Test Time Adaptation
Authors:Jiaming Liu, Senqiao Yang, Peidong Jia, Ming Lu, Yandong Guo, Wei Xue, Shanghang Zhang
Abstract: Since real-world machine systems are running in non-stationary and continually changing environments, Continual Test-Time Adaptation (CTTA) task is proposed to adapt the pre-trained model to continually changing target domains. Recently, existing methods mainly focus on model-based adaptation, which aims to leverage a self-training manner to extract the target domain knowledge. However, pseudo labels can be noisy and the updated model parameters are uncertain under dynamic data distributions, leading to error accumulation and catastrophic forgetting in the continual adaptation process. To tackle these challenges and maintain the model plasticity, we tactfully design a Visual Domain Adapter (ViDA) for CTTA, explicitly handling both domain-specific and domain-agnostic knowledge. Specifically, we first comprehensively explore the different domain representations of the adapters with trainable high and low-rank embedding space. Then we inject ViDAs into the pre-trained model, which leverages high-rank and low-rank prototypes to adapt the current domain distribution and maintain the continual domain-shared knowledge, respectively. To adapt to the various distribution shifts of each sample in target domains, we further propose a Homeostatic Knowledge Allotment (HKA) strategy, which adaptively merges knowledge from each ViDA with different rank prototypes. Extensive experiments conducted on four widely-used benchmarks demonstrate that our proposed method achieves state-of-the-art performance in both classification and segmentation CTTA tasks. In addition, our method can be regarded as a novel transfer paradigm and showcases promising results in zero-shot adaptation of foundation models to continual downstream tasks and distributions.
16.An Overview of Challenges in Egocentric Text-Video Retrieval
Authors:Burak Satar, Hongyuan Zhu, Hanwang Zhang, Joo Hwee Lim
Abstract: Text-video retrieval contains various challenges, including biases coming from diverse sources. We highlight some of them supported by illustrations to open a discussion. Besides, we address one of the biases, frame length bias, with a simple method which brings a very incremental but promising increase. We conclude with future directions.
17.Fine-Grained Visual Prompting
Authors:Lingfeng Yang, Yueze Wang, Xiang Li, Xinlong Wang, Jian Yang
Abstract: Vision-Language Models (VLMs), such as CLIP, have demonstrated impressive zero-shot transfer capabilities in image-level visual perception. However, these models have shown limited performance in instance-level tasks that demand precise localization and recognition. Previous works have suggested that incorporating visual prompts, such as colorful boxes or circles, can improve the ability of models to recognize objects of interest. Nonetheless, compared to language prompting, visual prompting designs are rarely explored. Existing approaches, which employ coarse visual cues such as colorful boxes or circles, often result in sub-optimal performance due to the inclusion of irrelevant and noisy pixels. In this paper, we carefully study the visual prompting designs by exploring more fine-grained markings, such as segmentation masks and their variations. In addition, we introduce a new zero-shot framework that leverages pixel-level annotations acquired from a generalist segmentation model for fine-grained visual prompting. Consequently, our investigation reveals that a straightforward application of blur outside the target mask, referred to as the Blur Reverse Mask, exhibits exceptional effectiveness. This proposed prompting strategy leverages the precise mask annotations to reduce focus on weakly related regions while retaining spatial coherence between the target and the surrounding background. Our Fine-Grained Visual Prompting (FGVP) demonstrates superior performance in zero-shot comprehension of referring expressions on the RefCOCO, RefCOCO+, and RefCOCOg benchmarks. It outperforms prior methods by an average margin of 3.0% to 4.6%, with a maximum improvement of 12.5% on the RefCOCO+ testA subset. The part detection experiments conducted on the PACO dataset further validate the preponderance of FGVP over existing visual prompting techniques. Code and models will be made available.
18.Youku-mPLUG: A 10 Million Large-scale Chinese Video-Language Dataset for Pre-training and Benchmarks
Authors:Haiyang Xu, Qinghao Ye, Xuan Wu, Ming Yan, Yuan Miao, Jiabo Ye, Guohai Xu, Anwen Hu, Yaya Shi, Guangwei Xu, Chenliang Li, Qi Qian, Maofei Que, Ji Zhang, Xiao Zeng, Fei Huang
Abstract: To promote the development of Vision-Language Pre-training (VLP) and multimodal Large Language Model (LLM) in the Chinese community, we firstly release the largest public Chinese high-quality video-language dataset named Youku-mPLUG, which is collected from Youku, a well-known Chinese video-sharing website, with strict criteria of safety, diversity, and quality. Youku-mPLUG contains 10 million Chinese video-text pairs filtered from 400 million raw videos across a wide range of 45 diverse categories for large-scale pre-training. In addition, to facilitate a comprehensive evaluation of video-language models, we carefully build the largest human-annotated Chinese benchmarks covering three popular video-language tasks of cross-modal retrieval, video captioning, and video category classification. Youku-mPLUG can enable researchers to conduct more in-depth multimodal research and develop better applications in the future. Furthermore, we release popular video-language pre-training models, ALPRO and mPLUG-2, and our proposed modularized decoder-only model mPLUG-video pre-trained on Youku-mPLUG. Experiments show that models pre-trained on Youku-mPLUG gain up to 23.1% improvement in video category classification. Besides, mPLUG-video achieves a new state-of-the-art result on these benchmarks with 80.5% top-1 accuracy in video category classification and 68.9 CIDEr score in video captioning, respectively. Finally, we scale up mPLUG-video based on the frozen Bloomz with only 1.7% trainable parameters as Chinese multimodal LLM, and demonstrate impressive instruction and video understanding ability. The zero-shot instruction understanding experiment indicates that pretraining with Youku-mPLUG can enhance the ability to comprehend overall and detailed visual semantics, recognize scene text, and leverage open-domain knowledge.
19.SF-FSDA: Source-Free Few-Shot Domain Adaptive Object Detection with Efficient Labeled Data Factory
Authors:Han Sun, Rui Gong, Konrad Schindler, Luc Van Gool
Abstract: Domain adaptive object detection aims to leverage the knowledge learned from a labeled source domain to improve the performance on an unlabeled target domain. Prior works typically require the access to the source domain data for adaptation, and the availability of sufficient data on the target domain. However, these assumptions may not hold due to data privacy and rare data collection. In this paper, we propose and investigate a more practical and challenging domain adaptive object detection problem under both source-free and few-shot conditions, named as SF-FSDA. To overcome this problem, we develop an efficient labeled data factory based approach. Without accessing the source domain, the data factory renders i) infinite amount of synthesized target-domain like images, under the guidance of the few-shot image samples and text description from the target domain; ii) corresponding bounding box and category annotations, only demanding minimum human effort, i.e., a few manually labeled examples. On the one hand, the synthesized images mitigate the knowledge insufficiency brought by the few-shot condition. On the other hand, compared to the popular pseudo-label technique, the generated annotations from data factory not only get rid of the reliance on the source pretrained object detection model, but also alleviate the unavoidably pseudo-label noise due to domain shift and source-free condition. The generated dataset is further utilized to adapt the source pretrained object detection model, realizing the robust object detection under SF-FSDA. The experiments on different settings showcase that our proposed approach outperforms other state-of-the-art methods on SF-FSDA problem. Our codes and models will be made publicly available.
20.M$^3$IT: A Large-Scale Dataset towards Multi-Modal Multilingual Instruction Tuning
Authors:Lei Li, Yuwei Yin, Shicheng Li, Liang Chen, Peiyi Wang, Shuhuai Ren, Mukai Li, Yazheng Yang, Jingjing Xu, Xu Sun, Lingpeng Kong, Qi Liu
Abstract: Instruction tuning has significantly advanced large language models (LLMs) such as ChatGPT, enabling them to align with human instructions across diverse tasks. However, progress in open vision-language models (VLMs) has been limited due to the scarcity of high-quality instruction datasets. To tackle this challenge and promote research in the vision-language field, we introduce the Multi-Modal, Multilingual Instruction Tuning (M$^3$IT) dataset, designed to optimize VLM alignment with human instructions. Our M$^3$IT dataset comprises 40 carefully curated datasets, including 2.4 million instances and 400 manually written task instructions, reformatted into a vision-to-text structure. Key tasks are translated into 80 languages with an advanced translation system, ensuring broader accessibility. M$^3$IT surpasses previous datasets regarding task coverage, instruction number and instance scale. Moreover, we develop Ying-VLM, a VLM model trained on our M$^3$IT dataset, showcasing its potential to answer complex questions requiring world knowledge, generalize to unseen video tasks, and comprehend unseen instructions in Chinese. To encourage further research, we have open-sourced both the dataset and trained models.
21.Improving Diffusion-based Image Translation using Asymmetric Gradient Guidance
Authors:Gihyun Kwon, Jong Chul Ye
Abstract: Diffusion models have shown significant progress in image translation tasks recently. However, due to their stochastic nature, there's often a trade-off between style transformation and content preservation. Current strategies aim to disentangle style and content, preserving the source image's structure while successfully transitioning from a source to a target domain under text or one-shot image conditions. Yet, these methods often require computationally intense fine-tuning of diffusion models or additional neural networks. To address these challenges, here we present an approach that guides the reverse process of diffusion sampling by applying asymmetric gradient guidance. This results in quicker and more stable image manipulation for both text-guided and image-guided image translation. Our model's adaptability allows it to be implemented with both image- and latent-diffusion models. Experiments show that our method outperforms various state-of-the-art models in image translation tasks.
22.FoSp: Focus and Separation Network for Early Smoke Segmentation
Authors:Lujian Yao, Haitao Zhao, Jingchao Peng, Zhongze Wang, Kaijie Zhao
Abstract: Early smoke segmentation (ESS) enables the accurate identification of smoke sources, facilitating the prompt extinguishing of fires and preventing large-scale gas leaks. But ESS poses greater challenges than conventional object and regular smoke segmentation due to its small scale and transparent appearance, which can result in high miss detection rate and low precision. To address these issues, a Focus and Separation Network (FoSp) is proposed. We first introduce a Focus module employing bidirectional cascade which guides low-resolution and high-resolution features towards mid-resolution to locate and determine the scope of smoke, reducing the miss detection rate. Next, we propose a Separation module that separates smoke images into a pure smoke foreground and a smoke-free background, enhancing the contrast between smoke and background fundamentally, improving segmentation precision. Finally, a Domain Fusion module is developed to integrate the distinctive features of the two modules which can balance recall and precision to achieve high F_beta. Futhermore, to promote the development of ESS, we introduce a high-quality real-world dataset called SmokeSeg, which contains more small and transparent smoke than the existing datasets. Experimental results show that our model achieves the best performance on three available datasets: SYN70K (mIoU: 83.00%), SMOKE5K (F_beta: 81.6%) and SmokeSeg (F_beta: 72.05%). Especially, our FoSp outperforms SegFormer by 7.71% (F_beta) for early smoke segmentation on SmokeSeg.
23.Defocus to focus: Photo-realistic bokeh rendering by fusing defocus and radiance priors
Authors:Xianrui Luo, Juewen Peng, Ke Xian, Zijin Wu, Zhiguo Cao
Abstract: We consider the problem of realistic bokeh rendering from a single all-in-focus image. Bokeh rendering mimics aesthetic shallow depth-of-field (DoF) in professional photography, but these visual effects generated by existing methods suffer from simple flat background blur and blurred in-focus regions, giving rise to unrealistic rendered results. In this work, we argue that realistic bokeh rendering should (i) model depth relations and distinguish in-focus regions, (ii) sustain sharp in-focus regions, and (iii) render physically accurate Circle of Confusion (CoC). To this end, we present a Defocus to Focus (D2F) framework to learn realistic bokeh rendering by fusing defocus priors with the all-in-focus image and by implementing radiance priors in layered fusion. Since no depth map is provided, we introduce defocus hallucination to integrate depth by learning to focus. The predicted defocus map implies the blur amount of bokeh and is used to guide weighted layered rendering. In layered rendering, we fuse images blurred by different kernels based on the defocus map. To increase the reality of the bokeh, we adopt radiance virtualization to simulate scene radiance. The scene radiance used in weighted layered rendering reassigns weights in the soft disk kernel to produce the CoC. To ensure the sharpness of in-focus regions, we propose to fuse upsampled bokeh images and original images. We predict the initial fusion mask from our defocus map and refine the mask with a deep network. We evaluate our model on a large-scale bokeh dataset. Extensive experiments show that our approach is capable of rendering visually pleasing bokeh effects in complex scenes. In particular, our solution receives the runner-up award in the AIM 2020 Rendering Realistic Bokeh Challenge.
24.Improving neural network representations using human similarity judgments
Authors:Lukas Muttenthaler, Lorenz Linhardt, Jonas Dippel, Robert A. Vandermeulen, Katherine Hermann, Andrew K. Lampinen, Simon Kornblith
Abstract: Deep neural networks have reached human-level performance on many computer vision tasks. However, the objectives used to train these networks enforce only that similar images are embedded at similar locations in the representation space, and do not directly constrain the global structure of the resulting space. Here, we explore the impact of supervising this global structure by linearly aligning it with human similarity judgments. We find that a naive approach leads to large changes in local representational structure that harm downstream performance. Thus, we propose a novel method that aligns the global structure of representations while preserving their local structure. This global-local transform considerably improves accuracy across a variety of few-shot learning and anomaly detection tasks. Our results indicate that human visual representations are globally organized in a way that facilitates learning from few examples, and incorporating this global structure into neural network representations improves performance on downstream tasks.
25.NeMO: Neural Map Growing System for Spatiotemporal Fusion in Bird's-Eye-View and BDD-Map Benchmark
Authors:Xi Zhu, Xiya Cao, Zhiwei Dong, Caifa Zhou, Qiangbo Liu, Wei Li, Yongliang Wang
Abstract: Vision-centric Bird's-Eye View (BEV) representation is essential for autonomous driving systems (ADS). Multi-frame temporal fusion which leverages historical information has been demonstrated to provide more comprehensive perception results. While most research focuses on ego-centric maps of fixed settings, long-range local map generation remains less explored. This work outlines a new paradigm, named NeMO, for generating local maps through the utilization of a readable and writable big map, a learning-based fusion module, and an interaction mechanism between the two. With an assumption that the feature distribution of all BEV grids follows an identical pattern, we adopt a shared-weight neural network for all grids to update the big map. This paradigm supports the fusion of longer time series and the generation of long-range BEV local maps. Furthermore, we release BDD-Map, a BDD100K-based dataset incorporating map element annotations, including lane lines, boundaries, and pedestrian crossing. Experiments on the NuScenes and BDD-Map datasets demonstrate that NeMO outperforms state-of-the-art map segmentation methods. We also provide a new scene-level BEV map evaluation setting along with the corresponding baseline for a more comprehensive comparison.
26.PhenoBench -- A Large Dataset and Benchmarks for Semantic Image Interpretation in the Agricultural Domain
Authors:Jan Weyler, Federico Magistri, Elias Marks, Yue Linn Chong, Matteo Sodano, Gianmarco Roggiolani, Nived Chebrolu, Cyrill Stachniss, Jens Behley
Abstract: The production of food, feed, fiber, and fuel is a key task of agriculture. Especially crop production has to cope with a multitude of challenges in the upcoming decades caused by a growing world population, climate change, the need for sustainable production, lack of skilled workers, and generally the limited availability of arable land. Vision systems could help cope with these challenges by offering tools to make better and more sustainable field management decisions and support the breeding of new varieties of crops by allowing temporally dense and reproducible measurements. Recently, tackling perception tasks in the agricultural domain got increasing interest in the computer vision and robotics community since agricultural robotics are one promising solution for coping with the lack of workers and enable a more sustainable agricultural production at the same time. While large datasets and benchmarks in other domains are readily available and have enabled significant progress toward more reliable vision systems, agricultural datasets and benchmarks are comparably rare. In this paper, we present a large dataset and benchmarks for the semantic interpretation of images of real agricultural fields. Our dataset recorded with a UAV provides high-quality, dense annotations of crops and weeds, but also fine-grained labels of crop leaves at the same time, which enable the development of novel algorithms for visual perception in the agricultural domain. Together with the labeled data, we provide novel benchmarks for evaluating different visual perception tasks on a hidden test set comprised of different fields: known fields covered by the training data and a completely unseen field. The tasks cover semantic segmentation, panoptic segmentation of plants, leaf instance segmentation, detection of plants and leaves, and hierarchical panoptic segmentation for jointly identifying plants and leaves.
27.MarineVRS: Marine Video Retrieval System with Explainability via Semantic Understanding
Authors:Tan-Sang Ha, Hai Nguyen-Truong, Tuan-Anh Vu, Sai-Kit Yeung
Abstract: Building a video retrieval system that is robust and reliable, especially for the marine environment, is a challenging task due to several factors such as dealing with massive amounts of dense and repetitive data, occlusion, blurriness, low lighting conditions, and abstract queries. To address these challenges, we present MarineVRS, a novel and flexible video retrieval system designed explicitly for the marine domain. MarineVRS integrates state-of-the-art methods for visual and linguistic object representation to enable efficient and accurate search and analysis of vast volumes of underwater video data. In addition, unlike the conventional video retrieval system, which only permits users to index a collection of images or videos and search using a free-form natural language sentence, our retrieval system includes an additional Explainability module that outputs the segmentation masks of the objects that the input query referred to. This feature allows users to identify and isolate specific objects in the video footage, leading to more detailed analysis and understanding of their behavior and movements. Finally, with its adaptability, explainability, accuracy, and scalability, MarineVRS is a powerful tool for marine researchers and scientists to efficiently and accurately process vast amounts of data and gain deeper insights into the behavior and movements of marine species.
28.Integrating Geometric Control into Text-to-Image Diffusion Models for High-Quality Detection Data Generation via Text Prompt
Authors:Kai Chen, Enze Xie, Zhe Chen, Lanqing Hong, Zhenguo Li, Dit-Yan Yeung
Abstract: Diffusion models have attracted significant attention due to their remarkable ability to create content and generate data for tasks such as image classification. However, the usage of diffusion models to generate high-quality object detection data remains an underexplored area, where not only the image-level perceptual quality but also geometric conditions such as bounding boxes and camera views are essential. Previous studies have utilized either copy-paste synthesis or layout-to-image (L2I) generation with specifically designed modules to encode semantic layouts. In this paper, we propose GeoDiffusion, a simple framework that can flexibly translate various geometric conditions into text prompts and empower the pre-trained text-to-image (T2I) diffusion models for high-quality detection data generation. Unlike previous L2I methods, our GeoDiffusion is able to encode not only bounding boxes but also extra geometric conditions such as camera views in self-driving scenes. Extensive experiments demonstrate GeoDiffusion outperforms previous L2I methods while maintaining 4x training time faster. To the best of our knowledge, this is the first work to adopt diffusion models for layout-to-image generation with geometric conditions and demonstrate that L2I-generated images can be beneficial for improving the performance of object detectors.
29.ICON$^2$: Reliably Benchmarking Predictive Inequity in Object Detection
Authors:Sruthi Sudhakar, Viraj Prabhu, Olga Russakovsky, Judy Hoffman
Abstract: As computer vision systems are being increasingly deployed at scale in high-stakes applications like autonomous driving, concerns about social bias in these systems are rising. Analysis of fairness in real-world vision systems, such as object detection in driving scenes, has been limited to observing predictive inequity across attributes such as pedestrian skin tone, and lacks a consistent methodology to disentangle the role of confounding variables e.g. does my model perform worse for a certain skin tone, or are such scenes in my dataset more challenging due to occlusion and crowds? In this work, we introduce ICON$^2$, a framework for robustly answering this question. ICON$^2$ leverages prior knowledge on the deficiencies of object detection systems to identify performance discrepancies across sub-populations, compute correlations between these potential confounders and a given sensitive attribute, and control for the most likely confounders to obtain a more reliable estimate of model bias. Using our approach, we conduct an in-depth study on the performance of object detection with respect to income from the BDD100K driving dataset, revealing useful insights.
30.ARTIC3D: Learning Robust Articulated 3D Shapes from Noisy Web Image Collections
Authors:Chun-Han Yao, Amit Raj, Wei-Chih Hung, Yuanzhen Li, Michael Rubinstein, Ming-Hsuan Yang, Varun Jampani
Abstract: Estimating 3D articulated shapes like animal bodies from monocular images is inherently challenging due to the ambiguities of camera viewpoint, pose, texture, lighting, etc. We propose ARTIC3D, a self-supervised framework to reconstruct per-instance 3D shapes from a sparse image collection in-the-wild. Specifically, ARTIC3D is built upon a skeleton-based surface representation and is further guided by 2D diffusion priors from Stable Diffusion. First, we enhance the input images with occlusions/truncation via 2D diffusion to obtain cleaner mask estimates and semantic features. Second, we perform diffusion-guided 3D optimization to estimate shape and texture that are of high-fidelity and faithful to input images. We also propose a novel technique to calculate more stable image-level gradients via diffusion models compared to existing alternatives. Finally, we produce realistic animations by fine-tuning the rendered shape and texture under rigid part transformations. Extensive evaluations on multiple existing datasets as well as newly introduced noisy web image collections with occlusions and truncation demonstrate that ARTIC3D outputs are more robust to noisy images, higher quality in terms of shape and texture details, and more realistic when animated. Project page: https://chhankyao.github.io/artic3d/
31.Designing a Better Asymmetric VQGAN for StableDiffusion
Authors:Zixin Zhu, Xuelu Feng, Dongdong Chen, Jianmin Bao, Le Wang, Yinpeng Chen, Lu Yuan, Gang Hua
Abstract: StableDiffusion is a revolutionary text-to-image generator that is causing a stir in the world of image generation and editing. Unlike traditional methods that learn a diffusion model in pixel space, StableDiffusion learns a diffusion model in the latent space via a VQGAN, ensuring both efficiency and quality. It not only supports image generation tasks, but also enables image editing for real images, such as image inpainting and local editing. However, we have observed that the vanilla VQGAN used in StableDiffusion leads to significant information loss, causing distortion artifacts even in non-edited image regions. To this end, we propose a new asymmetric VQGAN with two simple designs. Firstly, in addition to the input from the encoder, the decoder contains a conditional branch that incorporates information from task-specific priors, such as the unmasked image region in inpainting. Secondly, the decoder is much heavier than the encoder, allowing for more detailed recovery while only slightly increasing the total inference cost. The training cost of our asymmetric VQGAN is cheap, and we only need to retrain a new asymmetric decoder while keeping the vanilla VQGAN encoder and StableDiffusion unchanged. Our asymmetric VQGAN can be widely used in StableDiffusion-based inpainting and local editing methods. Extensive experiments demonstrate that it can significantly improve the inpainting and editing performance, while maintaining the original text-to-image capability. The code is available at \url{https://github.com/buxiangzhiren/Asymmetric_VQGAN}.
32.Contrastive Lift: 3D Object Instance Segmentation by Slow-Fast Contrastive Fusion
Authors:Yash Bhalgat, Iro Laina, João F. Henriques, Andrew Zisserman, Andrea Vedaldi
Abstract: Instance segmentation in 3D is a challenging task due to the lack of large-scale annotated datasets. In this paper, we show that this task can be addressed effectively by leveraging instead 2D pre-trained models for instance segmentation. We propose a novel approach to lift 2D segments to 3D and fuse them by means of a neural field representation, which encourages multi-view consistency across frames. The core of our approach is a slow-fast clustering objective function, which is scalable and well-suited for scenes with a large number of objects. Unlike previous approaches, our method does not require an upper bound on the number of objects or object tracking across frames. To demonstrate the scalability of the slow-fast clustering, we create a new semi-realistic dataset called the Messy Rooms dataset, which features scenes with up to 500 objects per scene. Our approach outperforms the state-of-the-art on challenging scenes from the ScanNet, Hypersim, and Replica datasets, as well as on our newly created Messy Rooms dataset, demonstrating the effectiveness and scalability of our slow-fast clustering method.
33.GP-UNIT: Generative Prior for Versatile Unsupervised Image-to-Image Translation
Authors:Shuai Yang, Liming Jiang, Ziwei Liu, Chen Change Loy
Abstract: Recent advances in deep learning have witnessed many successful unsupervised image-to-image translation models that learn correspondences between two visual domains without paired data. However, it is still a great challenge to build robust mappings between various domains especially for those with drastic visual discrepancies. In this paper, we introduce a novel versatile framework, Generative Prior-guided UNsupervised Image-to-image Translation (GP-UNIT), that improves the quality, applicability and controllability of the existing translation models. The key idea of GP-UNIT is to distill the generative prior from pre-trained class-conditional GANs to build coarse-level cross-domain correspondences, and to apply the learned prior to adversarial translations to excavate fine-level correspondences. With the learned multi-level content correspondences, GP-UNIT is able to perform valid translations between both close domains and distant domains. For close domains, GP-UNIT can be conditioned on a parameter to determine the intensity of the content correspondences during translation, allowing users to balance between content and style consistency. For distant domains, semi-supervised learning is explored to guide GP-UNIT to discover accurate semantic correspondences that are hard to learn solely from the appearance. We validate the superiority of GP-UNIT over state-of-the-art translation models in robust, high-quality and diversified translations between various domains through extensive experiments.