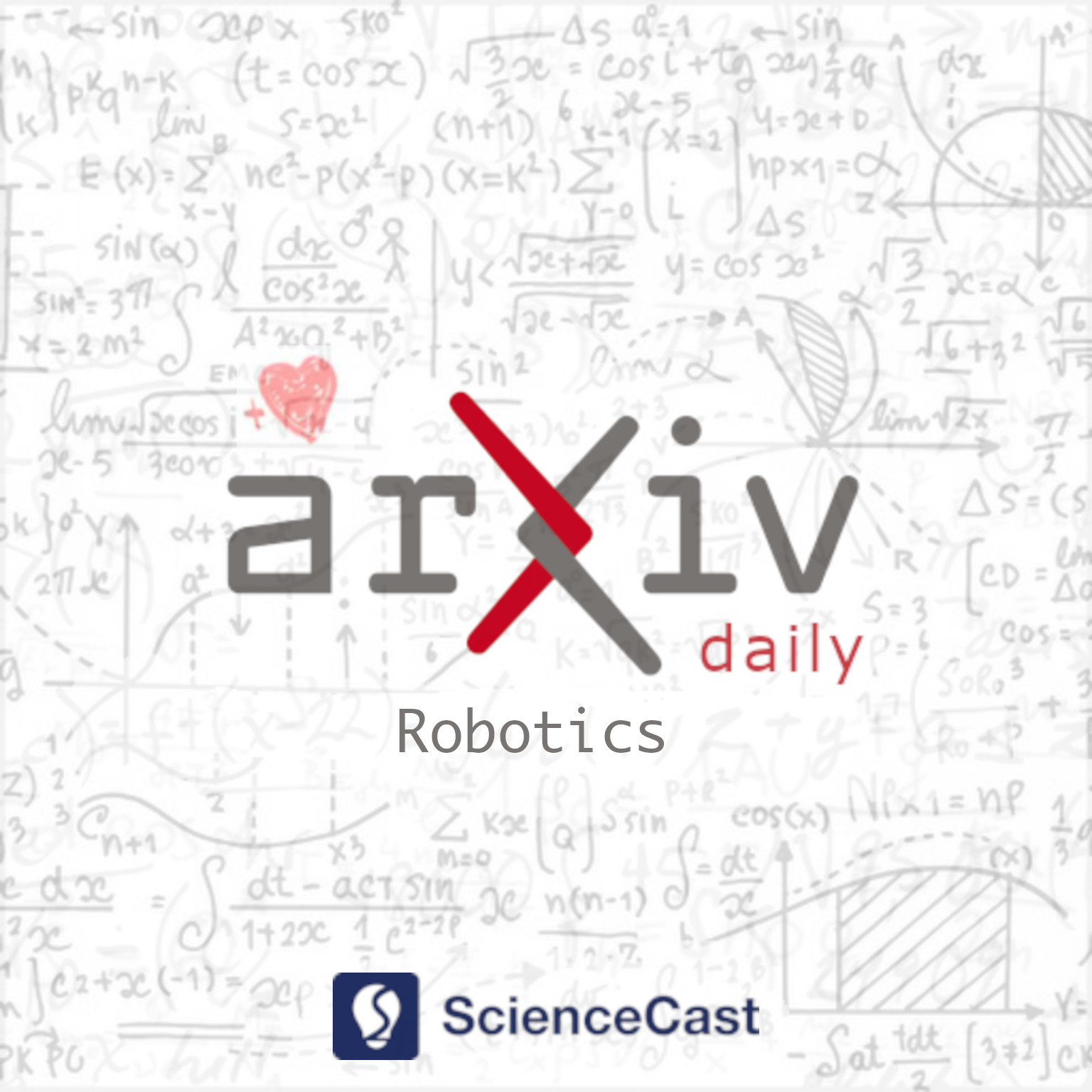
Robotics (cs.RO)
Wed, 13 Sep 2023
1.Solar-powered shape-changing origami microfliers
Authors:Kyle Johnson, Vicente Arroyos, Amélie Ferran, Tilboon Elberier, Raul Villanueva, Dennis Yin, Alberto Aliseda, Sawyer Fuller, Vikram Iyer, Shyamnath Gollakota
Abstract: Using wind to disperse microfliers that fall like seeds and leaves can help automate large-scale sensor deployments. Here, we present battery-free microfliers that can change shape in mid-air to vary their dispersal distance. We design origami microfliers using bi-stable leaf-out structures and uncover an important property: a simple change in the shape of these origami structures causes two dramatically different falling behaviors. When unfolded and flat, the microfliers exhibit a tumbling behavior that increases lateral displacement in the wind. When folded inward, their orientation is stabilized, resulting in a downward descent that is less influenced by wind. To electronically transition between these two shapes, we designed a low-power electromagnetic actuator that produces peak forces of up to 200 millinewtons within 25 milliseconds while powered by solar cells. We fabricated a circuit directly on the folded origami structure that includes a programmable microcontroller, Bluetooth radio, solar power harvesting circuit, a pressure sensor to estimate altitude and a temperature sensor. Outdoor evaluations show that our 414 milligram origami microfliers are able to electronically change their shape mid-air, travel up to 98 meters in a light breeze, and wirelessly transmit data via Bluetooth up to 60 meters away, using only power collected from the sun.
2.Hierarchical Time-Optimal Planning for Multi-Vehicle Racing
Authors:Georg Jank, Matthias Rowold, Boris Lohmann
Abstract: This paper presents a hierarchical planning algorithm for racing with multiple opponents. The two-stage approach consists of a high-level behavioral planning step and a low-level optimization step. By combining discrete and continuous planning methods, our algorithm encourages global time optimality without being limited by coarse discretization. In the behavioral planning step, the fastest behavior is determined with a low-resolution spatio-temporal visibility graph. Based on the selected behavior, we calculate maneuver envelopes that are subsequently applied as constraints in a time-optimal control problem. The performance of our method is comparable to a parallel approach that selects the fastest trajectory from multiple optimizations with different behavior classes. However, our algorithm can be executed on a single core. This significantly reduces computational requirements, especially when multiple opponents are involved. Therefore, the proposed method is an efficient and practical solution for real-time multi-vehicle racing scenarios.
3.Time-Optimal Gate-Traversing Planner for Autonomous Drone Racing
Authors:Chao Qin, Maxime S. J. Michet, Jingxiang Chen, Hugh H. -T. Liu
Abstract: Time-minimum trajectories through race tracks are determined by the drone's capability as well as the configuration of all gates (e.g., their shapes, sizes, and orientations). However, prior works neglect the impact of the gate configuration and formulate drone racing as a waypoint flight task, leading to conservative waypoint selection through each gate. We present a novel time-optimal planner that can account for gate constraints explicitly, enabling quadrotors to follow the most time-efficient waypoints at their single-rotor-thrust limits in tracks with hybrid gate types. Our approach provides comparable solution quality to the state-of-the-art but with a computation time orders of magnitude faster. Furthermore, the proposed framework allows users to customize gate constraints such as tunnels by concatenating existing gate classes, enabling high-fidelity race track modeling. Owing to the superior computation efficiency and flexibility, we can generate optimal racing trajectories for complex race tracks with tens or even hundreds of gates with distinct shapes. We validate our method in real-world flights and demonstrate that faster lap times can be produced by using gate constraints instead of waypoint constraints.
4.Stepwise Model Reconstruction of Robotic Manipulator Based on Data-Driven Method
Authors:Dingxu Guo, Jian xu, Shu Zhang
Abstract: Research on dynamics of robotic manipulators provides promising support for model-based control. In general, rigorous first-principles-based dynamics modeling and accurate identification of mechanism parameters are critical to achieving high precision in model-based control, while data-driven model reconstruction provides alternative approaches of the above process. Taking the level of activation of data as an indicator, this paper classifies the collected robotic manipulator data by means of K-means clustering algorithm. With the fundamental prior knowledge, we find the corresponding dynamical properties behind the classified data separately. Afterwards, the sparse identification of nonlinear dynamics (SINDy) method is used to reconstruct the dynamics model of the robotic manipulator step by step according to the activation level of the classified data. The simulation results show that the proposed method not only reduces the complexity of the basis function library, enabling the application of SINDy method to multi-degree-of-freedom robotic manipulators, but also decreases the influence of data noise on the regression results. Finally, the dynamic control based on the reconfigured model is deployed on the experimental platform, and the experimental results prove the effectiveness of the proposed method.
5.Lavender Autonomous Navigation with Semantic Segmentation at the Edge
Authors:Alessandro Navone, Fabrizio Romanelli, Marco Ambrosio, Mauro Martini, Simone Angarano, Marcello Chiaberge
Abstract: Achieving success in agricultural activities heavily relies on precise navigation in row crop fields. Recently, segmentation-based navigation has emerged as a reliable technique when GPS-based localization is unavailable or higher accuracy is needed due to vegetation or unfavorable weather conditions. It also comes in handy when plants are growing rapidly and require an online adaptation of the navigation algorithm. This work applies a segmentation-based visual agnostic navigation algorithm to lavender fields, considering both simulation and real-world scenarios. The effectiveness of this approach is validated through a wide set of experimental tests, which show the capability of the proposed solution to generalize over different scenarios and provide highly-reliable results.
6.Towards Connecting Control to Perception: High-Performance Whole-Body Collision Avoidance Using Control-Compatible Obstacles
Authors:Moritz Eckhoff, Dennis Knobbe, Henning Zwirnmann, Abdalla Swikir, Sami Haddadin
Abstract: One of the most important aspects of autonomous systems is safety. This includes ensuring safe human-robot and safe robot-environment interaction when autonomously performing complex tasks or in collaborative scenarios. Although several methods have been introduced to tackle this, most are unsuitable for real-time applications and require carefully hand-crafted obstacle descriptions. In this work, we propose a method combining high-frequency and real-time self and environment collision avoidance of a robotic manipulator with low-frequency, multimodal, and high-resolution environmental perceptions accumulated in a digital twin system. Our method is based on geometric primitives, so-called primitive skeletons. These, in turn, are information-compressed and real-time compatible digital representations of the robot's body and environment, automatically generated from ultra-realistic virtual replicas of the real world provided by the digital twin. Our approach is a key enabler for closing the loop between environment perception and robot control by providing the millisecond real-time control stage with a current and accurate world description, empowering it to react to environmental changes. We evaluate our whole-body collision avoidance on a 9-DOFs robot system through five experiments, demonstrating the functionality and efficiency of our framework.
7.Utilizing Hybrid Trajectory Prediction Models to Recognize Highly Interactive Traffic Scenarios
Authors:Maximilian Zipfl, Sven Spickermann, J. Marius Zöllner
Abstract: Autonomous vehicles hold great promise in improving the future of transportation. The driving models used in these vehicles are based on neural networks, which can be difficult to validate. However, ensuring the safety of these models is crucial. Traditional field tests can be costly, time-consuming, and dangerous. To address these issues, scenario-based closed-loop simulations can simulate many hours of vehicle operation in a shorter amount of time and allow for specific investigation of important situations. Nonetheless, the detection of relevant traffic scenarios that also offer substantial testing benefits remains a significant challenge. To address this need, in this paper we build an imitation learning based trajectory prediction for traffic participants. We combine an image-based (CNN) approach to represent spatial environmental factors and a graph-based (GNN) approach to specifically represent relations between traffic participants. In our understanding, traffic scenes that are highly interactive due to the network's significant utilization of the social component are more pertinent for a validation process. Therefore, we propose to use the activity of such sub networks as a measure of interactivity of a traffic scene. We evaluate our model using a motion dataset and discuss the value of the relationship information with respect to different traffic situations.
8.3D Active Metric-Semantic SLAM
Authors:Yuezhan Tao, Xu Liu, Igor Spasojevic, Saurav Agarwa, Vijay Kumar
Abstract: In this letter, we address the problem of exploration and metric-semantic mapping of multi-floor GPS-denied indoor environments using Size Weight and Power (SWaP) constrained aerial robots. Most previous work in exploration assumes that robot localization is solved. However, neglecting the state uncertainty of the agent can ultimately lead to cascading errors both in the resulting map and in the state of the agent itself. Furthermore, actions that reduce localization errors may be at direct odds with the exploration task. We propose a framework that balances the efficiency of exploration with actions that reduce the state uncertainty of the agent. In particular, our algorithmic approach for active metric-semantic SLAM is built upon sparse information abstracted from raw problem data, to make it suitable for SWaP-constrained robots. Furthermore, we integrate this framework within a fully autonomous aerial robotic system that achieves autonomous exploration in cluttered, 3D environments. From extensive real-world experiments, we showed that by including Semantic Loop Closure (SLC), we can reduce the robot pose estimation errors by over 90% in translation and approximately 75% in yaw, and the uncertainties in pose estimates and semantic maps by over 70% and 65%, respectively. Although discussed in the context of indoor multi-floor exploration, our system can be used for various other applications, such as infrastructure inspection and precision agriculture where reliable GPS data may not be available.
9.Real-Time Motion Planning for In-Hand Manipulation with a Multi-Fingered Hand
Authors:Xiao Gao, Kunpeng Yao, Farshad Khadivar, Aude Billard
Abstract: Dexterous manipulation of objects once held in hand remains a challenge. Such skills are, however, necessary for robotics to move beyond gripper-based manipulation and use all the dexterity offered by anthropomorphic robotic hands. One major challenge when manipulating an object within the hand is that fingers must move around the object while avoiding collision with other fingers or the object. Such collision-free paths must be computed in real-time, as the smallest deviation from the original plan can easily lead to collisions. We present a real-time approach to computing collision-free paths in a high-dimensional space. To guide the exploration, we learn an explicit representation of the free space, retrievable in real-time. We further combine this representation with closed-loop control via dynamical systems and sampling-based motion planning and show that the combination increases performance compared to alternatives, offering efficient search of feasible paths and real-time obstacle avoidance in a multi-fingered robotic hand.
10.Learning to Explore Indoor Environments using Autonomous Micro Aerial Vehicles
Authors:Yuezhan Tao, Eran Iceland, Beiming Li, Elchanan Zwecher, Uri Heinemann, Avraham Cohen, Amir Avni, Oren Gal, Ariel Barel, Vijay Kumar
Abstract: In this paper, we address the challenge of exploring unknown indoor aerial environments using autonomous aerial robots with Size Weight and Power (SWaP) constraints. The SWaP constraints induce limits on mission time requiring efficiency in exploration. We present a novel exploration framework that uses Deep Learning (DL) to predict the most likely indoor map given the previous observations, and Deep Reinforcement Learning (DRL) for exploration, designed to run on modern SWaP constraints neural processors. The DL-based map predictor provides a prediction of the occupancy of the unseen environment while the DRL-based planner determines the best navigation goals that can be safely reached to provide the most information. The two modules are tightly coupled and run onboard allowing the vehicle to safely map an unknown environment. Extensive experimental and simulation results show that our approach surpasses state-of-the-art methods by 50-60% in efficiency, which we measure by the fraction of the explored space as a function of the length of the trajectory traveled.
11.Using Lidar Intensity for Robot Navigation
Authors:Adarsh Jagan Sathyamoorthy, Kasun Weerakoon, Mohamed Elnoor, Dinesh Manocha
Abstract: We present Multi-Layer Intensity Map, a novel 3D object representation for robot perception and autonomous navigation. They consist of multiple stacked layers of 2D grid maps each derived from reflected point cloud intensities corresponding to a certain height interval. The different layers of the intensity maps can be used to simultaneously estimate obstacles' height, solidity/density, and opacity. We demonstrate that they can help accurately differentiate obstacles that are safe to navigate through (e.g. beaded/string curtains, pliable tall grass), from ones that must be avoided (e.g. transparent surfaces such as glass walls, bushes, trees, etc.) in indoor and outdoor environments. Further, to handle narrow passages, and navigate through non-solid obstacles in dense environments, we propose an approach to adaptively inflate or enlarge the obstacles detected on intensity maps based on their solidity, and the robot's preferred velocity direction. We demonstrate these improved navigation capabilities in real-world narrow, dense environments using a real Turtlebot and Boston Dynamics Spot. We observe significant increases in success rates (up to 50%), a 9.55% decrease in trajectory length, and up to a 10.9% increase in the F-score compared to current navigation methods using other sensor modalities.
12.Efficient Reinforcement Learning for Jumping Monopods
Authors:Riccardo Bussola, Michele Focchi, Andrea Del Prete, Daniele Fontanelli, Luigi Palopoli
Abstract: In this work, we consider the complex control problem of making a monopod reach a target with a jump. The monopod can jump in any direction and the terrain underneath its foot can be uneven. This is a template of a much larger class of problems, which are extremely challenging and computationally expensive to solve using standard optimisation-based techniques. Reinforcement Learning (RL) could be an interesting alternative, but the application of an end-to-end approach in which the controller must learn everything from scratch, is impractical. The solution advocated in this paper is to guide the learning process within an RL framework by injecting physical knowledge. This expedient brings to widespread benefits, such as a drastic reduction of the learning time, and the ability to learn and compensate for possible errors in the low-level controller executing the motion. We demonstrate the advantage of our approach with respect to both optimization-based and end-to-end RL approaches.
13.Multi-Robot Informative Path Planning from Regression with Sparse Gaussian Processes
Authors:Kalvik Jakkala, Srinivas Akella
Abstract: This paper addresses multi-robot informative path planning (IPP) for environmental monitoring. The problem involves determining informative regions in the environment that should be visited by robots in order to gather the most information about the environment. We propose an efficient sparse Gaussian process-based approach that uses gradient descent to optimize paths in continuous environments. Our approach efficiently scales to both spatially and spatio-temporally correlated environments. Moreover, our approach can simultaneously optimize the informative paths while accounting for routing constraints, such as a distance budget and limits on the robot's velocity and acceleration. Our approach can be used for IPP with both discrete and continuous sensing robots, with point and non-point field-of-view sensing shapes, and for multi-robot IPP. The proposed approach is demonstrated to be fast and accurate on real-world data.
14.CLiFF-LHMP: Using Spatial Dynamics Patterns for Long-Term Human Motion Prediction
Authors:Yufei Zhu, Andrey Rudenko, Tomasz P. Kucner, Luigi Palmieri, Kai O. Arras, Achim J. Lilienthal, Martin Magnusson
Abstract: Human motion prediction is important for mobile service robots and intelligent vehicles to operate safely and smoothly around people. The more accurate predictions are, particularly over extended periods of time, the better a system can, e.g., assess collision risks and plan ahead. In this paper, we propose to exploit maps of dynamics (MoDs, a class of general representations of place-dependent spatial motion patterns, learned from prior observations) for long-term human motion prediction (LHMP). We present a new MoD-informed human motion prediction approach, named CLiFF-LHMP, which is data efficient, explainable, and insensitive to errors from an upstream tracking system. Our approach uses CLiFF-map, a specific MoD trained with human motion data recorded in the same environment. We bias a constant velocity prediction with samples from the CLiFF-map to generate multi-modal trajectory predictions. In two public datasets we show that this algorithm outperforms the state of the art for predictions over very extended periods of time, achieving 45% more accurate prediction performance at 50s compared to the baseline.
15.RadarLCD: Learnable Radar-based Loop Closure Detection Pipeline
Authors:Mirko Usuelli, Matteo Frosi, Paolo Cudrano, Simone Mentasti, Matteo Matteucci
Abstract: Loop Closure Detection (LCD) is an essential task in robotics and computer vision, serving as a fundamental component for various applications across diverse domains. These applications encompass object recognition, image retrieval, and video analysis. LCD consists in identifying whether a robot has returned to a previously visited location, referred to as a loop, and then estimating the related roto-translation with respect to the analyzed location. Despite the numerous advantages of radar sensors, such as their ability to operate under diverse weather conditions and provide a wider range of view compared to other commonly used sensors (e.g., cameras or LiDARs), integrating radar data remains an arduous task due to intrinsic noise and distortion. To address this challenge, this research introduces RadarLCD, a novel supervised deep learning pipeline specifically designed for Loop Closure Detection using the FMCW Radar (Frequency Modulated Continuous Wave) sensor. RadarLCD, a learning-based LCD methodology explicitly designed for radar systems, makes a significant contribution by leveraging the pre-trained HERO (Hybrid Estimation Radar Odometry) model. Being originally developed for radar odometry, HERO's features are used to select key points crucial for LCD tasks. The methodology undergoes evaluation across a variety of FMCW Radar dataset scenes, and it is compared to state-of-the-art systems such as Scan Context for Place Recognition and ICP for Loop Closure. The results demonstrate that RadarLCD surpasses the alternatives in multiple aspects of Loop Closure Detection.