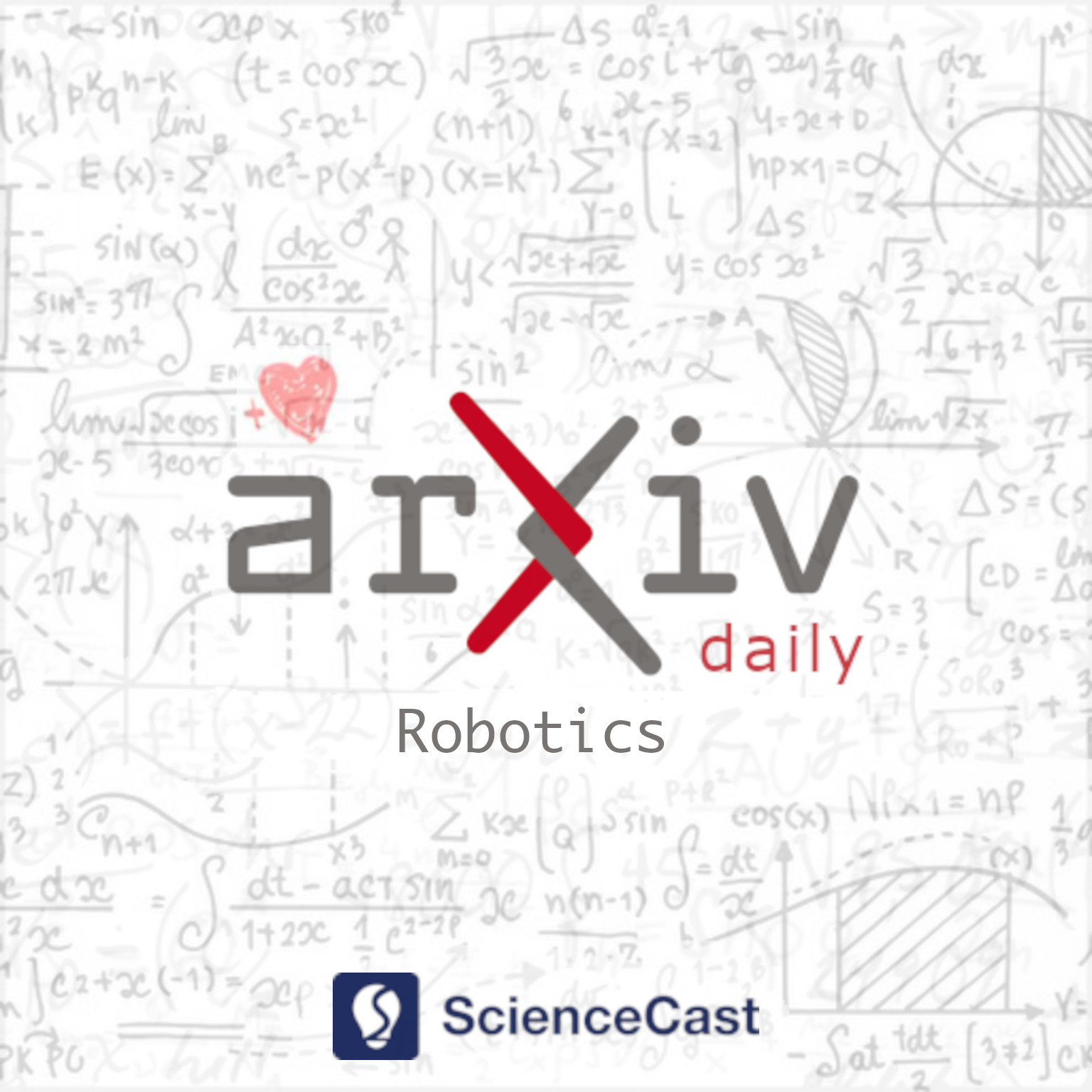
Robotics (cs.RO)
Mon, 03 Jul 2023
1.Surgical fine-tuning for Grape Bunch Segmentation under Visual Domain Shifts
Authors:Agnese Chiatti, Riccardo Bertoglio, Nico Catalano, Matteo Gatti, Matteo Matteucci
Abstract: Mobile robots will play a crucial role in the transition towards sustainable agriculture. To autonomously and effectively monitor the state of plants, robots ought to be equipped with visual perception capabilities that are robust to the rapid changes that characterise agricultural settings. In this paper, we focus on the challenging task of segmenting grape bunches from images collected by mobile robots in vineyards. In this context, we present the first study that applies surgical fine-tuning to instance segmentation tasks. We show how selectively tuning only specific model layers can support the adaptation of pre-trained Deep Learning models to newly-collected grape images that introduce visual domain shifts, while also substantially reducing the number of tuned parameters.
2.Advantages of Multimodal versus Verbal-Only Robot-to-Human Communication with an Anthropomorphic Robotic Mock Driver
Authors:Tim Schreiter, Lucas Morillo-Mendez, Ravi T. Chadalavada, Andrey Rudenko, Erik Billing, Martin Magnusson, Kai O. Arras, Achim J. Lilienthal
Abstract: Robots are increasingly used in shared environments with humans, making effective communication a necessity for successful human-robot interaction. In our work, we study a crucial component: active communication of robot intent. Here, we present an anthropomorphic solution where a humanoid robot communicates the intent of its host robot acting as an "Anthropomorphic Robotic Mock Driver" (ARMoD). We evaluate this approach in two experiments in which participants work alongside a mobile robot on various tasks, while the ARMoD communicates a need for human attention, when required, or gives instructions to collaborate on a joint task. The experiments feature two interaction styles of the ARMoD: a verbal-only mode using only speech and a multimodal mode, additionally including robotic gaze and pointing gestures to support communication and register intent in space. Our results show that the multimodal interaction style, including head movements and eye gaze as well as pointing gestures, leads to more natural fixation behavior. Participants naturally identified and fixated longer on the areas relevant for intent communication, and reacted faster to instructions in collaborative tasks. Our research further indicates that the ARMoD intent communication improves engagement and social interaction with mobile robots in workplace settings.
3.Perch a quadrotor on planes by the ceiling effect
Authors:Yuying Zou, Haotian Li, Yunfan Ren, Wei Xu, Yihang Li, Yixi Cai, Shenji Zhou, Fu Zhang
Abstract: Perching is a promising solution for a small unmanned aerial vehicle (UAV) to save energy and extend operation time. This paper proposes a quadrotor that can perch on planar structures using the ceiling effect. Compared with the existing work, this perching method does not require any claws, hooks, or adhesive pads, leading to a simpler system design. This method does not limit the perching by surface angle or material either. The design of the quadrotor that only uses its propeller guards for surface contact is presented in this paper. We also discussed the automatic perching strategy including trajectory generation and power management. Experiments are conducted to verify that the approach is practical and the UAV can perch on planes with different angles. Energy consumption in the perching state is assessed, showing that more than 30% of power can be saved. Meanwhile, the quadrotor exhibits improved stability while perching compared to when it is hovering.
4.A Biomimetic Fingerprint for Robotic Tactile Sensing
Authors:Oscar Alberto Juiña Quilachamín, Nicolás Navarro-Guerrero
Abstract: Tactile sensors have been developed since the early '70s and have greatly improved, but there are still no widely adopted solutions. Various technologies, such as capacitive, piezoelectric, piezoresistive, optical, and magnetic, are used in haptic sensing. However, most sensors are not mechanically robust for many applications and cannot cope well with curved or sizeable surfaces. Aiming to address this problem, we present a 3D-printed fingerprint pattern to enhance the body-borne vibration signal for dynamic tactile feedback. The 3D-printed fingerprint patterns were designed and tested for an RH8D Adult size Robot Hand. The patterns significantly increased the signal's power to over 11 times the baseline. A public haptic dataset including 52 objects of several materials was created using the best fingerprint pattern and material.
5.A Data-Driven Approach to Geometric Modeling of Systems with Low-Bandwidth Actuator Dynamics
Authors:Siming Deng, Junning Liu, Bibekananda Datta, Aishwarya Pantula, David H. Gracias, Thao D. Nguyen, Brian A. Bittner, Noah J. Cowan
Abstract: It is challenging to perform identification on soft robots due to their underactuated, high dimensional dynamics. In this work, we present a data-driven modeling framework, based on geometric mechanics (also known as gauge theory), that can be applied to systems with low-bandwidth actuation of the shape space. By exploiting temporal asymmetries in actuator dynamics, our approach enables the design of robots that can be driven by a single control input. We present a method for constructing a series connected model comprising actuator and locomotor dynamics based on data points from stochastically perturbed, repeated behaviors around the observed limit cycle. We demonstrate our methods on a real-world example of a soft crawler made by stimuli-responsive hydrogels that locomotes on merely one cycling control signal by utilizing its geometric and temporal asymmetry. For systems with first-order, low-pass actuator dynamics, such as swelling-driven actuators used in hydrogel crawlers, we show that first order Taylor approximations can well capture the dynamics of the system shape as well as its movements. Finally, we propose an approach of numerically optimizing control signals by iteratively refining models and optimizing the input waveform.
6.Scenario-Based Motion Planning with Bounded Probability of Collision
Authors:Oscar de Groot, Laura Ferranti, Dariu Gavrila, Javier Alonso-Mora
Abstract: Robots will increasingly operate near humans that introduce uncertainties in the motion planning problem due to their complex nature. Typically, chance constraints are introduced in the planner to optimize performance while guaranteeing probabilistic safety. However, existing methods do not consider the actual probability of collision for the planned trajectory, but rather its marginalization, that is, the independent collision probabilities for each planning step and/or dynamic obstacle, resulting in conservative trajectories. To address this issue, we introduce a novel real-time capable method termed Safe Horizon MPC, that explicitly constrains the joint probability of collision with all obstacles over the duration of the motion plan. This is achieved by reformulating the chance-constrained planning problem using scenario optimization and predictive control. Our method is less conservative than state-of-the-art approaches, applicable to arbitrary probability distributions of the obstacles' trajectories, computationally tractable and scalable. We demonstrate our proposed approach using a mobile robot and an autonomous vehicle in an environment shared with humans.
7.Assessment of the Utilization of Quadruped Robots in Pharmaceutical Research and Development Laboratories
Authors:Brian Parkinson, Ádám Wolf, Péter Galambos, Károly Széll
Abstract: Drug development is becoming more and more complex and resource-intensive. To reduce the costs and the time-to-market, the pharmaceutical industry employs cutting-edge automation solutions. Supportive robotics technologies, such as stationary and mobile manipulators, exist in various laboratory settings. However, they still lack the mobility and dexterity to navigate and operate in human-centered environments. We evaluate the feasibility of quadruped robots for the specific use case of remote inspection, utilizing the out-of-the-box capabilities of Boston Dynamics' Spot platform. We also provide an outlook on the newest technological advancements and the future applications these are anticipated to enable.
8.Artifacts Mapping: Multi-Modal Semantic Mapping for Object Detection and 3D Localization
Authors:Federico Rollo, Gennaro Raiola, Andrea Zunino, Nikolaos Tsagarakis, Arash Ajoudani
Abstract: Geometric navigation is nowadays a well-established field of robotics and the research focus is shifting towards higher-level scene understanding, such as Semantic Mapping. When a robot needs to interact with its environment, it must be able to comprehend the contextual information of its surroundings. This work focuses on classifying and localising objects within a map, which is under construction (SLAM) or already built. To further explore this direction, we propose a framework that can autonomously detect and localize predefined objects in a known environment using a multi-modal sensor fusion approach (combining RGB and depth data from an RGB-D camera and a lidar). The framework consists of three key elements: understanding the environment through RGB data, estimating depth through multi-modal sensor fusion, and managing artifacts (i.e., filtering and stabilizing measurements). The experiments show that the proposed framework can accurately detect 98% of the objects in the real sample environment, without post-processing, while 85% and 80% of the objects were mapped using the single RGBD camera or RGB + lidar setup respectively. The comparison with single-sensor (camera or lidar) experiments is performed to show that sensor fusion allows the robot to accurately detect near and far obstacles, which would have been noisy or imprecise in a purely visual or laser-based approach.
9.Soft Gripping: Specifying for Trustworthiness
Authors:Dhaminda B. Abeywickrama, Nguyen Hao Le, Greg Chance, Peter D. Winter, Arianna Manzini, Alix J. Partridge, Jonathan Ives, John Downer, Graham Deacon, Jonathan Rossiter, Kerstin Eder, Shane Windsor
Abstract: Soft robotics is an emerging technology in which engineers create flexible devices for use in a variety of applications. In order to advance the wide adoption of soft robots, ensuring their trustworthiness is essential; if soft robots are not trusted, they will not be used to their full potential. In order to demonstrate trustworthiness, a specification needs to be formulated to define what is trustworthy. However, even for soft robotic grippers, which is one of the most mature areas in soft robotics, the soft robotics community has so far given very little attention to formulating specifications. In this work, we discuss the importance of developing specifications during development of soft robotic systems, and present an extensive example specification for a soft gripper for pick-and-place tasks for grocery items. The proposed specification covers both functional and non-functional requirements, such as reliability, safety, adaptability, predictability, ethics, and regulations. We also highlight the need to promote verifiability as a first-class objective in the design of a soft gripper.
10.Social Impressions of the NAO Robot and its Impact on Physiology
Authors:Ruchik Mishra, Karla Conn Welch
Abstract: The social applications of robots possess intrinsic challenges with respect to social paradigms and heterogeneity of different groups. These challenges can be in the form of social acceptability, anthropomorphism, likeability, past experiences with robots etc. In this paper, we have considered a group of neurotypical adults to describe how different voices and motion types of the NAO robot can have effect on the perceived safety, anthropomorphism, likeability, animacy, and perceived intelligence of the robot. In addition, prior robot experience has also been taken into consideration to perform this analysis using a one-way Analysis of Variance (ANOVA). Further, we also demonstrate that these different modalities instigate different physiological responses in the person. This classification has been done using two different deep learning approaches, 1) Convolutional Neural Network (CNN), and 2) Gramian Angular Fields on the Blood Volume Pulse (BVP) data recorded. Both of these approaches achieve better than chance accuracy 25% for a 4 class classification.
11.Towards Safe Autonomous Driving Policies using a Neuro-Symbolic Deep Reinforcement Learning Approach
Authors:Iman Sharifi, Mustafa Yildirim, Saber Fallah
Abstract: The dynamic nature of driving environments and the presence of diverse road users pose significant challenges for decision-making in autonomous driving. Deep reinforcement learning (DRL) has emerged as a popular approach to tackle this problem. However, the application of existing DRL solutions is mainly confined to simulated environments due to safety concerns, impeding their deployment in real-world. To overcome this limitation, this paper introduces a novel neuro-symbolic model-free DRL approach, called DRL with Symbolic Logics (DRLSL) that combines the strengths of DRL (learning from experience) and symbolic first-order logics knowledge-driven reasoning) to enable safe learning in real-time interactions of autonomous driving within real environments. This innovative approach provides a means to learn autonomous driving policies by actively engaging with the physical environment while ensuring safety. We have implemented the DRLSL framework in autonomous driving using the highD dataset and demonstrated that our method successfully avoids unsafe actions during both the training and testing phases. Furthermore, our results indicate that DRLSL achieves faster convergence during training and exhibits better generalizability to new driving scenarios compared to traditional DRL methods.
12.Density-based Feasibility Learning with Normalizing Flows for Introspective Robotic Assembly
Authors:Jianxiang Feng, Matan Atad, Ismael Rodríguez, Maximilian Durner, Stephan Günnemann, Rudolph Triebel
Abstract: Machine Learning (ML) models in Robotic Assembly Sequence Planning (RASP) need to be introspective on the predicted solutions, i.e. whether they are feasible or not, to circumvent potential efficiency degradation. Previous works need both feasible and infeasible examples during training. However, the infeasible ones are hard to collect sufficiently when re-training is required for swift adaptation to new product variants. In this work, we propose a density-based feasibility learning method that requires only feasible examples. Concretely, we formulate the feasibility learning problem as Out-of-Distribution (OOD) detection with Normalizing Flows (NF), which are powerful generative models for estimating complex probability distributions. Empirically, the proposed method is demonstrated on robotic assembly use cases and outperforms other single-class baselines in detecting infeasible assemblies. We further investigate the internal working mechanism of our method and show that a large memory saving can be obtained based on an advanced variant of NF.
13.Dynamic Mobile Manipulation via Whole-Body Bilateral Teleoperation of a Wheeled Humanoid
Authors:Amartya Purushottam, Yeongtae Jung, Christopher Xu, Joao Ramos
Abstract: Humanoid robots have the potential to help human workers by realizing physically demanding manipulation tasks such as moving large boxes within warehouses. We define such tasks as Dynamic Mobile Manipulation (DMM). This paper presents a framework for DMM via whole-body teleoperation, built upon three key contributions: Firstly, a teleoperation framework employing a Human Machine Interface (HMI) and a bi-wheeled humanoid, SATYRR, is proposed. Secondly, the study introduces a dynamic locomotion mapping, utilizing human-robot reduced order models, and a kinematic retargeting strategy for manipulation tasks. Additionally, the paper discusses the role of whole-body haptic feedback for wheeled humanoid control. Finally, the system's effectiveness and mappings for DMM are validated through locomanipulation experiments and heavy box pushing tasks. Here we show two forms of DMM: grasping a target moving at an average speed of 0.4 m/s, and pushing boxes weighing up to 105\% of the robot's weight. By simultaneously adjusting their pitch and using their arms, the pilot adjusts the robot pose to apply larger contact forces and move a heavy box at a constant velocity of 0.2 m/s.
14.Efficient Determination of Safety Requirements for Perception Systems
Authors:Sydney M. Katz, Anthony L. Corso, Esen Yel, Mykel J. Kochenderfer
Abstract: Perception systems operate as a subcomponent of the general autonomy stack, and perception system designers often need to optimize performance characteristics while maintaining safety with respect to the overall closed-loop system. For this reason, it is useful to distill high-level safety requirements into component-level requirements on the perception system. In this work, we focus on efficiently determining sets of safe perception system performance characteristics given a black-box simulator of the fully-integrated, closed-loop system. We combine the advantages of common black-box estimation techniques such as Gaussian processes and threshold bandits to develop a new estimation method, which we call smoothing bandits. We demonstrate our method on a vision-based aircraft collision avoidance problem and show improvements in terms of both accuracy and efficiency over the Gaussian process and threshold bandit baselines.
15.Multi-Predictor Fusion: Combining Learning-based and Rule-based Trajectory Predictors
Authors:Sushant Veer, Apoorva Sharma, Marco Pavone
Abstract: Trajectory prediction modules are key enablers for safe and efficient planning of autonomous vehicles (AVs), particularly in highly interactive traffic scenarios. Recently, learning-based trajectory predictors have experienced considerable success in providing state-of-the-art performance due to their ability to learn multimodal behaviors of other agents from data. In this paper, we present an algorithm called multi-predictor fusion (MPF) that augments the performance of learning-based predictors by imbuing them with motion planners that are tasked with satisfying logic-based rules. MPF probabilistically combines learning- and rule-based predictors by mixing trajectories from both standalone predictors in accordance with a belief distribution that reflects the online performance of each predictor. In our results, we show that MPF outperforms the two standalone predictors on various metrics and delivers the most consistent performance.