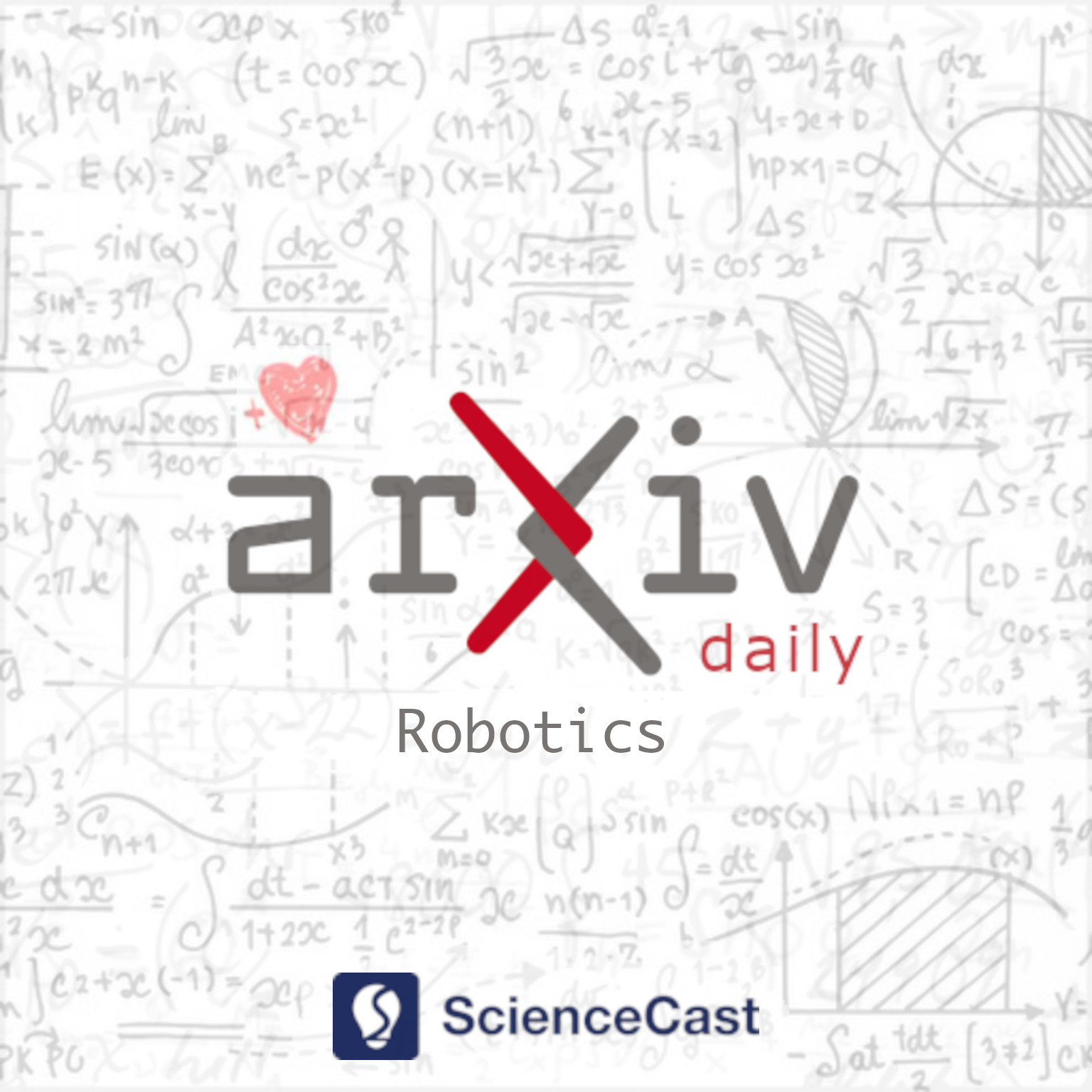
Robotics (cs.RO)
Wed, 30 Aug 2023
1.Learning the References of Online Model Predictive Control for Urban Self-Driving
Authors:Yubin Wang, Zengqi Peng, Hakim Ghazzai, Jun Ma
Abstract: In this work, we propose a novel learning-based online model predictive control (MPC) framework for motion synthesis of self-driving vehicles. In this framework, the decision variables are generated as instantaneous references to modulate the cost functions of online MPC, where the constraints of collision avoidance and drivable surface boundaries are latently represented in the soft form. Hence, the embodied maneuvers of the ego vehicle are empowered to adapt to complex and dynamic traffic environments, even with unmodeled uncertainties of other traffic participants. Furthermore, we implement a deep reinforcement learning (DRL) framework for policy search to cast the step actions as the decision variables, where the practical and lightweight observations are considered as the input features of the policy network. The proposed approach is implemented in the high-fidelity simulator involving compound-complex urban driving scenarios, and the results demonstrate that the proposed development manifests remarkable adaptiveness to complex and dynamic traffic environments with a success rate of 85%. Also, its advantages in terms of safety, maneuverability, and robustness are illustrated.
2.Learning to Navigate from Scratch using World Models and Curiosity: the Good, the Bad, and the Ugly
Authors:Daria de Tinguy, Sven Remmery, Pietro Mazzaglia, Tim Verbelen, Bart Dhoedt
Abstract: Learning to navigate unknown environments from scratch is a challenging problem. This work presents a system that integrates world models with curiosity-driven exploration for autonomous navigation in new environments. We evaluate performance through simulations and real-world experiments of varying scales and complexities. In simulated environments, the approach rapidly and comprehensively explores the surroundings. Real-world scenarios introduce additional challenges. Despite demonstrating promise in a small controlled environment, we acknowledge that larger and dynamic environments can pose challenges for the current system. Our analysis emphasizes the significance of developing adaptable and robust world models that can handle environmental changes to prevent repetitive exploration of the same areas.
3.Sarrus-inspired Deployable Polyhedral Mechanisms
Authors:Yuanqing Gu, Xiao Zhang, Guowu Wei, Yan Chen
Abstract: Deployable polyhedral mechanisms (DPMs) have witnessed flourishing growth in recent years because of their potential applications in robotics, space exploration, structure engineering, etc. This paper firstly presents the construction, mobility and kinematics of a family of Sarrus-inspired deployable polyhedral mechanisms. By carrying out expansion operation and implanting Sarrus linkages along the straight-line motion paths, deployable tetrahedral, cubic and dodecahedral mechanisms are identified and constructed following tetrahedral, octahedral and icosahedral symmetry, respectively. Three paired transformations with synchronized radial motion between Platonic and Archimedean polyhedrons are revealed, and their significant symmetric properties are perfectly remained in each work configuration. Subsequently, with assistant of equivalent prismatic joints, the equivalent analysis strategy for mobility of multiloop polyhedral mechanisms is proposed to significantly simplify the calculation process. This paper hence presents the construction method and equivalent analysis of the Sarrus-inspired DPMs that are not only valuable in theoretical investigation, but also have great potential in practical applications such as mechanical metamaterials, deployable architectures and space exploration.
4.Sparse Waypoint Validity Checking for Self-Entanglement-Free Tethered Path Planning
Authors:Tong Yang, Jiangpin Liu, Yue Wang, Rong Xiong
Abstract: A novel mechanism to derive self-entanglement-free (SEF) path for tethered differential-driven robots is proposed in this work. The problem is tailored to the deployment of tethered differential-driven robots in situations where an omni-directional tether re-tractor is not available. This is frequently encountered when it is impractical to concurrently equip an omni-directional tether retracting mechanism with other geometrically intricate devices, such as a manipulator, which is notably relevant in applications like disaster recovery, spatial exploration, etc. Without specific attention to the spatial relation between the shape of the tether and the pose of the mobile unit, the issue of self-entanglement arises when the robot moves, resulting in unsafe robot movements and the risk of damaging the tether. In this paper, the SEF constraint is first formulated as the boundedness of a relative angle function which characterises the angular difference between the tether stretching direction and the robot's heading direction. Then, a constrained searching-based path planning algorithm is proposed which produces a path that is sub-optimal whilst ensuring the avoidance of tether self-entanglement. Finally, the algorithmic efficiency of the proposed path planner is further enhanced by proving the conditioned sparsity of the primitive path validity checking module. The effectiveness of the proposed algorithm is assessed through case studies, comparing its performance against untethered differential-driven planners in challenging planning scenarios. A comparative analysis is further conducted between the normal node expansion module and the improved node expansion module which incorporates sparse waypoint validity checking. Real-world tests are also conducted to validate the algorithm's performance. An open-source implementation has also made available for the benefit of the robotics community.
5.High Performance Networking Layer for Simulation Applications
Authors:Amir Mohammad Zarif Shahsavan Nejad, Amir Mahdi Zarif Shahsavan Nejad, Amirali Setayeshi, Soroush Sadeghnejad
Abstract: Autonomous vehicles are one of the most popular and also fast-growing technologies in the world. As we go further, there are still a lot of challenges that are unsolved and may cause problems in the future when it comes to testing in real world. Simulations on the other hand have always had a huge impact in the fields of science, technology, physics, etc. The simulation also powers real-world Autonomous Vehicles nowadays. Therefore, We have built an Autonomous Vehicle Simulation Software - called AVIS Engine - that provides tools and features that help develop autonomous vehicles in various environments. AVIS Engine features an advanced input and output system for the vehicle and includes a traffic system and vehicle sensor system which can be communicated using the fast networking system and ROS Bridge.
6.WALL-E: Embodied Robotic WAiter Load Lifting with Large Language Model
Authors:Tianyu Wang, Yifan Li, Haitao Lin, Xiangyang Xue, Yanwei Fu
Abstract: Enabling robots to understand language instructions and react accordingly to visual perception has been a long-standing goal in the robotics research community. Achieving this goal requires cutting-edge advances in natural language processing, computer vision, and robotics engineering. Thus, this paper mainly investigates the potential of integrating the most recent Large Language Models (LLMs) and existing visual grounding and robotic grasping system to enhance the effectiveness of the human-robot interaction. We introduce the WALL-E (Embodied Robotic WAiter load lifting with Large Language model) as an example of this integration. The system utilizes the LLM of ChatGPT to summarize the preference object of the users as a target instruction via the multi-round interactive dialogue. The target instruction is then forwarded to a visual grounding system for object pose and size estimation, following which the robot grasps the object accordingly. We deploy this LLM-empowered system on the physical robot to provide a more user-friendly interface for the instruction-guided grasping task. The further experimental results on various real-world scenarios demonstrated the feasibility and efficacy of our proposed framework.
7.RoboTAP: Tracking Arbitrary Points for Few-Shot Visual Imitation
Authors:Mel Vecerik, Carl Doersch, Yi Yang, Todor Davchev, Yusuf Aytar, Guangyao Zhou, Raia Hadsell, Lourdes Agapito, Jon Scholz
Abstract: For robots to be useful outside labs and specialized factories we need a way to teach them new useful behaviors quickly. Current approaches lack either the generality to onboard new tasks without task-specific engineering, or else lack the data-efficiency to do so in an amount of time that enables practical use. In this work we explore dense tracking as a representational vehicle to allow faster and more general learning from demonstration. Our approach utilizes Track-Any-Point (TAP) models to isolate the relevant motion in a demonstration, and parameterize a low-level controller to reproduce this motion across changes in the scene configuration. We show this results in robust robot policies that can solve complex object-arrangement tasks such as shape-matching, stacking, and even full path-following tasks such as applying glue and sticking objects together, all from demonstrations that can be collected in minutes.
8.Predicting Energy Consumption and Traversal Time of Ground Robots for Outdoor Navigation on Multiple Types of Terrain
Authors:Matthias Eder, Gerald Steinbauer-Wagner
Abstract: The outdoor navigation capabilities of ground robots have improved significantly in recent years, opening up new potential applications in a variety of settings. Cost-based representations of the environment are frequently used in the path planning domain to obtain an optimized path based on various objectives, such as traversal time or energy consumption. However, obtaining such cost representations is still cumbersome, particularly in outdoor settings with diverse terrain types and slope angles. In this paper, we address this problem by using a data-driven approach to develop a cost representation for various outdoor terrain types that supports two optimization objectives, namely energy consumption and traversal time. We train a supervised machine learning model whose inputs consists of extracted environment data along a path and whose outputs are the predicted energy consumption and traversal time. The model is based on a ResNet neural network architecture and trained using field-recorded data. The error of the proposed method on different types of terrain is within 11\% of the ground truth data. To show that it performs and generalizes better than currently existing approaches on various types of terrain, a comparison to a baseline method is made.
9.DRL-Based Trajectory Tracking for Motion-Related Modules in Autonomous Driving
Authors:Yinda Xu, Lidong Yu
Abstract: Autonomous driving systems are always built on motion-related modules such as the planner and the controller. An accurate and robust trajectory tracking method is indispensable for these motion-related modules as a primitive routine. Current methods often make strong assumptions about the model such as the context and the dynamics, which are not robust enough to deal with the changing scenarios in a real-world system. In this paper, we propose a Deep Reinforcement Learning (DRL)-based trajectory tracking method for the motion-related modules in autonomous driving systems. The representation learning ability of DL and the exploration nature of RL bring strong robustness and improve accuracy. Meanwhile, it enhances versatility by running the trajectory tracking in a model-free and data-driven manner. Through extensive experiments, we demonstrate both the efficiency and effectiveness of our method compared to current methods.
10.EnsembleFollower: A Hybrid Car-Following Framework Based On Reinforcement Learning and Hierarchical Planning
Authors:Xu Han, Xianda Chen, Meixin Zhu, Pinlong Cai, Jianshan Zhou, Xiaowen Chu
Abstract: Car-following models have made significant contributions to our understanding of longitudinal driving behavior. However, they often exhibit limited accuracy and flexibility, as they cannot fully capture the complexity inherent in car-following processes, or may falter in unseen scenarios due to their reliance on confined driving skills present in training data. It is worth noting that each car-following model possesses its own strengths and weaknesses depending on specific driving scenarios. Therefore, we propose EnsembleFollower, a hierarchical planning framework for achieving advanced human-like car-following. The EnsembleFollower framework involves a high-level Reinforcement Learning-based agent responsible for judiciously managing multiple low-level car-following models according to the current state, either by selecting an appropriate low-level model to perform an action or by allocating different weights across all low-level components. Moreover, we propose a jerk-constrained kinematic model for more convincing car-following simulations. We evaluate the proposed method based on real-world driving data from the HighD dataset. The experimental results illustrate that EnsembleFollower yields improved accuracy of human-like behavior and achieves effectiveness in combining hybrid models, demonstrating that our proposed framework can handle diverse car-following conditions by leveraging the strengths of various low-level models.
11.Learning Vision-based Pursuit-Evasion Robot Policies
Authors:Andrea Bajcsy, Antonio Loquercio, Ashish Kumar, Jitendra Malik
Abstract: Learning strategic robot behavior -- like that required in pursuit-evasion interactions -- under real-world constraints is extremely challenging. It requires exploiting the dynamics of the interaction, and planning through both physical state and latent intent uncertainty. In this paper, we transform this intractable problem into a supervised learning problem, where a fully-observable robot policy generates supervision for a partially-observable one. We find that the quality of the supervision signal for the partially-observable pursuer policy depends on two key factors: the balance of diversity and optimality of the evader's behavior and the strength of the modeling assumptions in the fully-observable policy. We deploy our policy on a physical quadruped robot with an RGB-D camera on pursuit-evasion interactions in the wild. Despite all the challenges, the sensing constraints bring about creativity: the robot is pushed to gather information when uncertain, predict intent from noisy measurements, and anticipate in order to intercept. Project webpage: https://abajcsy.github.io/vision-based-pursuit/